A new prediction score for critically ill patients—do we need an Apgar score for acute respiratory distress syndrome?
A score to quickly summarize the health of newborns
Back in 1952 the anesthesiologist Virginia Apgar invented a score to quickly summarize the health of neonates (1). The scale of the score was determined by evaluating a neonate on five simple criteria on a scale from zero to two, then summing up the five values thus obtained, at 1 and 5 minutes after birth. Some 10 years after initial publication, a backronym for the score was coined in the United States as a mnemonic learning aid: appearance (skin color), pulse (heart rate), grimace (reflex irritability), activity (muscle tone), and respiration, or “Apgar score”. Of note, the purpose of the “Apgar score” was to determine quickly whether a newborn needed immediate medical care—it was not designed to predict long-term outcome; nevertheless, a score that remains <3 at later time points may indicate longer term neurological damage.
A score to quickly predict outcome in patients with acute respiratory distress syndrome (ARDS)
The “American-European Consensus Criteria of ARDS” as well as the more recent “Berlin definition for ARDS” both used the PaO2/FiO2 for classification of ARDS patients (2). The validity of using PaO2/FiO2 for classification of ARDS was recently criticized by a group of Spanish researchers who found the predictive accuracy of classification based on PaO2/FiO2 to be low, particularly when (I) calculated early after the initial diagnosis of ARDS; and (II) when calculated while not using predefined ventilator settings (3). They argued that there is need for better prediction scores for ARDS patients.
The same Spanish group of investigators recently reported on the “age, plateau pressure, and PaO2/FiO2 score”, in short APPS, a simple nine-point score to predict outcome of ARDS 24 hours after the diagnosis (4). Using tertiles of the three above described variables, they were able to predict hospital mortality with an area under the receiver operating characteristics curve of 0.76 in a training cohort, and of 0.80 in a validation cohort. The APPS was perfectly calibrated, thus an increase in APPS was linearly associated with an increase in mortality (Figure 1). The good results can be seen as remarkable, and the comparison with the “Apgar score” for neonates was swiftly made (5).
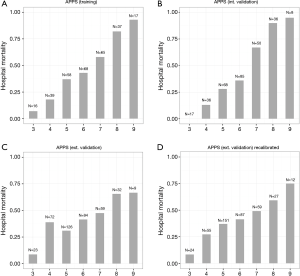
External validity
There are, however, some reservations with the validity of the APPS. A group of investigators in the Netherlands recently validated the new score (6). As patients in their centers were ventilated using pressure-controlled modes of ventilation, it was not possible to use plateau pressures, and they had to use maximum airway pressures instead. The predictive accuracy of the APPS for hospital mortality was moderate in their cohort of patients, with an area under the receiver operating characteristics curve of only 0.62, much lower than in the two cohorts from Spain.
Also calibration was disturbed in this external validation cohort (Figure 1). Of the three measures included in the score, only the PaO2/FiO2 showed a comparable predictive accuracy. Even when cutoffs for age and maximal airway pressure were adjusted, the predictive value for mortality remained low (Figure 1). The distribution of age is not the same in every ICU and largely depends on the geographical location of the hospital and the specialized care provided in the hospital. Additionally, age is also related to mortality outside of the hospital, but this is not a simple linear relation (7). The exact strength of this association per age naturally depends on the life expectance in the population (8). Therefore age cutoffs can and should not be translated blindly between countries in a general population, and probably also not in cohorts of critically ill patients.
PaO2/FiO2 could change depending on ventilator settings. Indeed, the Spanish consortium showed before that use of “standard” ventilator settings (tidal volumes of 6 mL/kg predicted body weight; PEEP according to pre-defined guideline) increases the predictive accuracy of PaO2/FiO2 for mortality (3). The same approach was used in their training and validation cohorts for the APPS, and this may explain the comparable association of PaO2/FiO2 with outcome. We may need to appreciate that patients in other centers are frequently not ventilated according to the guideline used in Spanish centers (9).
The plateau pressure suffers from the similar disadvantages. Use of higher levels of PEEP, for instance, increases plateau pressure levels without changing driving pressure levels. Use of recruitment maneuvers may decrease plateau pressure levels in patients with recruitable lung parts. Even the ventilator mode may influence the plateau pressure, as in a volume-controlled mode the plateau pressure may change with changes in lung compliance, while in a pressure-controlled mode the maximal airway pressure is fixed and therefore more constant.
Towards better prediction in ARDS patients
The central argument for using the APPS is the speed and simplicity with which it is calculated. The score is calculated swiftly at the bedside without difficult or complex calculations. This benefit may outweigh the disadvantages as described above. However, the APPS is meant to be calculated 24 hours after the initial diagnosis of ARDS and it is far from sure whether it qualifies as a fast score when this delay is inherent to the score. Also, with computers available at the bedside in almost every intensive care unit, and pocket computers in the hand of almost every physician, it is questionable if a score needs to be simple. It is not without reasons that APACHE scores are used worldwide, despite the fact that they take time to calculate and are complex: they are extremely accurate for mortality prediction (10).
If speed and simplicity can be ignored, a first suggestion could be re-inclusion of items that were excluded by the investigators of the APPS. These were excluded because they lacked statistical significance in the univariate comparison, but it is here where “P values” are frequently wrongly interpreted (11,12): P values do not provide information on added predictive accuracy of combination of variables. Indeed, predictors should always be evaluated in multivariable prediction models to obtain the optimal predictive accuracy, eliminating the least predictive factor step by step (13). This approach could result in a score with better predictive accuracy, and even could be less biased when tested in an external cohort.
Another step could be the addition of biological variables on top of the physiological parameters used in the APPS. The combination of clinical data with five biological markers (surfactant protein D, soluble tumor necrosis factor receptor 1, Von Willebrand factor, soluble intercellular adhesion molecule 1 and interleukin 8) had a higher predictive accuracy for mortality in ARDS patients, than a combination of clinical data alone (14). This was also shown for another biological marker, the soluble urokinase plasminogen activator receptor (15). The major disadvantage is that these markers are almost never routinely available, at least for now. However, also routinely available biological markers like plasma bicarbonate show an independent association with outcome in ARDS patients (16). Because biological markers are not directly influenced by the physician, the combination of physiological parameters with biological markers could be less biased when tested in an external cohort.
Conclusions
The APPS certainly is a promising next step in outcome prediction in ARDS patients. However, additional effort is needed. We suggest the addition of more physiological parameters and also biological variables.
Acknowledgements
Funding: Dr. Bos is supported by a junior investigator grant from the Dutch lung foundation (Longfonds; 4.2.16.132JO) and previously received a European Respiratory Society short-term fellowship award. Dr. Bos and Prof. Schultz were supported by the Center of Translational Molecular Medicine for the MARS (grant number 04I-201).
Footnote
Conflicts of Interest: The authors have no conflicts of interest to declare.
References
- Apgar V. A proposal for a new method of evaluation of the newborn infant. Curr Res Anesth Analg 1953;32:260-7. [Crossref] [PubMed]
- ARDS Definition Task Force, Ranieri VM, Rubenfeld GD, et al. Acute respiratory distress syndrome: the Berlin Definition. JAMA 2012;307:2526-33. [PubMed]
- Villar J, Blanco J, del Campo R, et al. Assessment of PaO2/FiO2 for stratification of patients with moderate and severe acute respiratory distress syndrome. BMJ Open 2015;5:e006812. [Crossref] [PubMed]
- Villar J, Ambrós A, Soler JA, et al. Age, PaO2/FIO2, and Plateau Pressure Score: A Proposal for a Simple Outcome Score in Patients With the Acute Respiratory Distress Syndrome. Crit Care Med 2016;44:1361-9. [Crossref] [PubMed]
- Villar J, Kacmarek RM. The APPS: an outcome score for the acute respiratory distress syndrome. J Thorac Dis 2016;8:E1343-E1347. [Crossref] [PubMed]
- Bos LD, Schouten LR, Cremer OL, et al. External validation of the APPS, a new and simple outcome prediction score in patients with the acute respiratory distress syndrome. Ann Intensive Care 2016;6:89. [Crossref] [PubMed]
- Kesteloot H, Huang X. On the relationship between human all-cause mortality and age. Eur J Epidemiol 2003;18:503-11. [Crossref] [PubMed]
- Wilmoth JR, Boe C, Barbieri M. Geographic Differences in Life Expectancy at Age 50 in the United States Compared with Other High-Income Countries. In: Crimmins EM, Preston SH, Cohen B. editors. International Differences in Mortality at Older Ages: Dimensions and Sources. The National Academies Press, 2010:333-66.
- Bellani G, Laffey JG, Pham T, et al. Epidemiology, Patterns of Care, and Mortality for Patients With Acute Respiratory Distress Syndrome in Intensive Care Units in 50 Countries. JAMA 2016;315:788-800. [Crossref] [PubMed]
- Knaus WA, Draper EA, Wagner DP, et al. APACHE II: a severity of disease classification system. Crit Care Med 1985;13:818-29. [Crossref] [PubMed]
- Nuzzo R. Scientific method: statistical errors. Nature 2014;506:150-2. [Crossref] [PubMed]
- Greenland S, Senn SJ, Rothman KJ, et al. Statistical tests, P values, confidence intervals, and power: a guide to misinterpretations. Eur J Epidemiol 2016;31:337-50. [Crossref] [PubMed]
- Moons KG, Kengne AP, Woodward M, et al. Risk prediction models: I. Development, internal validation, and assessing the incremental value of a new (bio)marker. Heart 2012;98:683-90. [Crossref] [PubMed]
- Calfee CS, Ware LB, Glidden DV, et al. Use of risk reclassification with multiple biomarkers improves mortality prediction in acute lung injury. Crit Care Med 2011;39:711-7. [Crossref] [PubMed]
- Geboers DG, de Beer FM, Tuip-de Boer AM, et al. Plasma suPAR as a prognostic biological marker for ICU mortality in ARDS patients. Intensive Care Med 2015;41:1281-90. [Crossref] [PubMed]
- Famous KR, Delucchi K, Ware LB, et al. Acute Respiratory Distress Syndrome Subphenotypes Respond Differently to Randomized Fluid Management Strategy. Am J Respir Crit Care Med 2017;195:331-8. [PubMed]