Genomics of lung cancer
Multi-platform investigation of non-small cell lung cancer has the capability of discovering clinically important biological pathways involved in cancer development, progression, prognosis and response to treatment in patients with lung cancer. These two complementary publications from this productive research group describe genomic analyses of the two commonest subtypes of lung cancer: lung adenocarcinoma (LUAD) and lung squamous cell carcinoma (LUSC). The investigators analyse primary whole exome sequencing (WES) data from resected early stage lung cancers, supplemented by combinatorial comparative analyses of available landmark data: The Cancer Genome Atlas (TCGA) (1,2).
In addition to describing the types and frequency of mutations, they provide a subset analysis of clinical relevance to survival and response to adjuvant therapy, as well as correlation with a panel of selected immune response biomarkers. The latter analyses are of topical interest given emerging evidence of clinical benefit from immunotherapies designed to address the immune checkpoints that tumours hijack to suppress host anti-cancer immunity.
The results are summarised in Table 1, which also provides a narrative comparison of the two lung cancer subtypes.
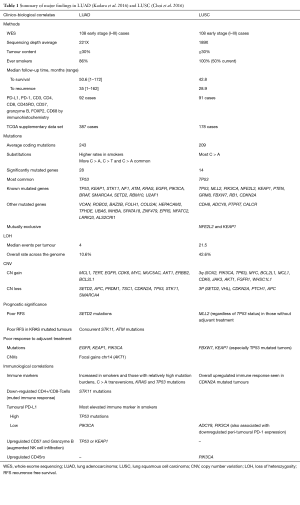
Full table
Briefly, in LUAD they found an average of 243 coding mutations, with 28 genes associated with an increased mutation burden based on a genome-wide threshold of P<2.4×10-6. Copy number variations (CNVs) were also identified, with gains in putative oncogenes and losses in tumour suppressor genes, as expected. Correlation between the primary data set and the publicly available TCGA data is reassuring as to face-validity. The observations between genomic changes and immune profiles by immunohistochemistry (IHC) and some clinical parameters (prognosis and response to adjuvant treatment) are interesting, and add to the accumulating knowledge of potential actionable biomarkers in this cancer phenotype.
In early stage LUSC, there were a mean of 209 exonic mutations, with 14 reaching statistically significance. Recurrent CNVs were also reported including gain of chromosome 3q, reaching a frequency of 70.4% in this sample-set. Mutations (apart from CDKN2 and MLL2) and CNVs appeared consistent between this cohort and TCGA dataset. Again there were correlations between genomic changes and immunoprofiling by IHC and certain clinical characteristics as summarised in Table 1. In contrast to LUAD where a high mutational burden corresponded with elevated immune markers including PD-L1, only CD57 expression was correlated in LUSC.
Strengths of these studies include technology platforms, depth of sequencing, and demonstration of general concordance with TCGA datasets. Many known genes were replicated and some new candidates have emerged. Functional correlation is of value in helping to decipher the meaning of genomic landscape aberrations in these cancers.
The relationship to prognosis and response to adjuvant therapy is interesting, but due to the relatively small sample size and absence of control data, it is mainly hypothesis generating, rather than a definitive demonstration of prognostic or predictive power. Also, since not every case was analysed for immune molecules, there is a possibility of selection bias, so it would be informative to know whether the cases were consecutive or a convenience sample, to judge the risk of such bias. Nonetheless, these data provide a rational basis for validation studies.
In summary, these companion papers report high quality data in modestly sized lung cancer subsets and add to the pivotal data generated by TCGA. They report some new gene candidates, and potentially useful biomarkers predictive of response to therapy. Furthermore, there were correlations with immune IHC biomarkers, some of which are known to differentially affect responses to emerging immunotherapies. The future addition of multi-omic comparison, e.g., epigenomics, RNA-Seq, proteomic, as well as multi-region sampling would add further value to these data helping to better understand the increasingly recognised complexities of intra- and inter-tumoural genomic heterogeneity (3-5).
Acknowledgements
Funding: This work was supported by a NHMRC Practitioner Fellowship 1019891 (KF), and NHMRC Career Development Fellowship 1026215 (IY).
Footnote
Conflicts of Interest: The authors have no conflicts of interest to declare.
References
- Cancer Genome Atlas Research Network. Comprehensive genomic characterization of squamous cell lung cancers. Nature 2012;489:519-25. [Crossref] [PubMed]
- Cancer Genome Atlas Research Network. Comprehensive molecular profiling of lung adenocarcinoma. Nature 2014;511:543-50. [Crossref] [PubMed]
- de Bruin EC, McGranahan N, Mitter R, et al. Spatial and temporal diversity in genomic instability processes defines lung cancer evolution. Science 2014;346:251-6. [Crossref] [PubMed]
- Zhang J, Fujimoto J, Zhang J, et al. Intratumor heterogeneity in localized lung adenocarcinomas delineated by multiregion sequencing. Science 2014;346:256-9. [Crossref] [PubMed]
- Gerlinger M, Rowan AJ, Horswell S, et al. Intratumor heterogeneity and branched evolution revealed by multiregion sequencing. N Engl J Med 2012;366:883-92. [Crossref] [PubMed]