Exhaled breath analysis for lung cancer
Lung cancer is the most common cause of cancer deaths worldwide, accounting for 1.37 million deaths in 2008 (1,2). Early diagnosis (Stage I) is associated with far better survival (67% 5-year survival rate) than later stage disease (Stage III, 23% 5-year survival rate) (3). Symptoms alone cannot be relied upon to indicate the presence of lung cancer as they often do not appear until the cancer is relatively advanced. New techniques to detect disease earlier in high risk populations of asymptomatic individuals would be expected to significantly improve survival. The aim of this review was to examine the scientific evidence relating result of the analyses of exhaled breath and exhaled breath condensate (EBC) to the presence of lung cancer.
Background
As early as Roman times, the smell of a person’s breath has assisted physicians with the diagnosis of a disease, e.g., uncontrolled diabetes was associated with a sweet, acetone odour; liver failure produced a fish-like smell; and renal failure was identified by a urine-like smell (4).
McCulloch (5) demonstrated that dogs could be trained to detect lung cancer and breast cancer in subjects with various stages of disease with almost 100% accuracy, merely by smelling the subject’s breath. These observations suggest that there are biomarkers in exhaled breath that are potentially useful for diagnosing disease.
Over the last 40 years there have been many studies aiming to characterise these biomarkers. In 1971, Pauling et al. (6), using a gas chromatograph (GC), measured 250 different compounds in human breath samples. Since then Phillips measured 1,259 compounds in normal subjects in 1997 (7), and over 3,000 compounds in 1999 (8).
Analysis of exhaled breath
The compounds in exhaled breath may be useful indicators of a disease process in the lung. They have been classified as:
- Inorganic compounds, e.g., carbon dioxide, oxygen, and nitric oxide;
- Non-volatile compounds measured in EBC, e.g., isoprostanes, cytokines, leukotrienes and hydrogen peroxide (4).
- Volatile organic compounds (VOCs) which can be divided into different classes, e.g., saturated hydrocarbons (ethane, pentane, aldehydes), unsaturated hydrocarbons (isoprene), oxygen containing (acetone), sulphur containing (ethyl mercaptane, dimethylsulfide) and nitrogen containing (dimethylamine, ammonia) (9). The most commonly identified VOCs are isoprene, acetone, ethanol, methanol, other alcohols and alkanes (8).
VOCs
Little is known about the genesis of exhaled breath VOCs. Some are thought to be endogenous, that is produced by the body as end-products of metabolic pathways, e.g., isoprene, an unsaturated hydrocarbon, formed along the mevalonic acid pathway of cholesterol synthesis (10); acetone, an oxygen containing compound produced from glucose metabolism; And saturated hydrocarbons or alkanes such as ethane and pentane produced from oxygen free radical-mediated lipid peroxidation of fatty acid components of cell membranes (11). The latter compounds are thought to be markers of oxidative stress. VOCs are also components of exogenous contaminants from the external environment that have been inhaled and absorbed through the lungs or skin. Apart from lung excretion, VOCs can be catabolized and excreted through the liver or kidney (12).
There have been studies published on the VOCs detected and measured in various respiratory disease states including asthma, COPD, cystic fibrosis, and lung cancer.
Table 1 summarizes the VOCs detected in the different lung diseases. It appears that there is not one VOC that is diagnostic for a condition but rather a combination of VOCs (15,22).
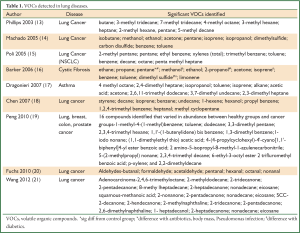
Full table
Exhaled breath VOC analysers
GC and mass spectrometry (GC-MS)
Because of the low concentrations of VOCs (parts per billion, ppb) (14,16) in exhaled breath, sensitive and highly accurate GCs and mass spectrometers have been utilized. Table 2 summarizes some of the published results of GC/mass spectrometric analysis of VOCs in exhaled breath. GCs and mass spectrometers have limited application in a clinical setting because of their expense, difficulty of use, and the need for highly experienced analysts to operate them and interpret the results.
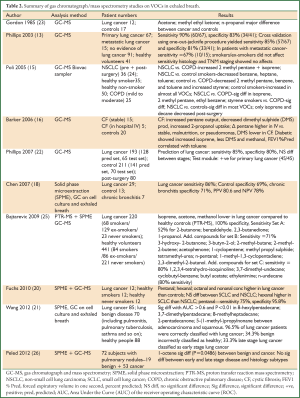
Full table
Portable/inexpensive devices
Several technologies, more portable and relatively inexpensive, have been developed and adapted to analyze exhaled breath samples. These include ion mobility spectrometers, and electronic nose instruments such as the Cyranose 320, the quartz microbalance, colorimeters, and gold particle nanosensors. Table 3 summarises published sensitivity and specificity results of electronic nose devices in the analysis of VOCs in exhaled breath of control and lung cancer subjects.
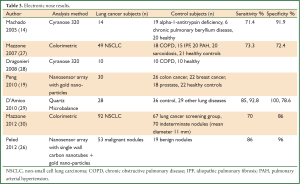
Full table
Ion mobility spectrometry (IMS)
The principle of the IMS system is a 550 MBq 63Ni β-radiation ionising source (Ni) which breaks down analytes from exhaled breath into ions. The ions separate and travel down a chamber at speeds that are related to their size, mass and geometry hitting a Faraday plate at the end of the chamber. As each ion hits the plate an electrical signal is generated which, when combined, produce an ion spectrum which is a fingerprint for the exhaled breath. Westhoff et al. (31) in 2009 was able to discriminate between 32 patients with lung cancer and 54 healthy subjects including both non-smokers and smokers in the group with 100% accuracy.
Electronic noses
Advances in technology have produced small, portable array type devices to detect and identify chemicals in gaseous samples. They are designed to respond to the mix of compounds in the sample rather than identify individual compounds. The principle behind the devices is that the VOCs adsorb onto a sensor producing a change in conductivity, color or oscillation of a crystal. Output is usually a pattern representing the mix of VOCs.
Quartz microbalance
The quartz microbalance is an 8 sensor array of oscillating quartz crystals coated with varied metalloporphyrins to which VOCs adsorb, changing the mass of the sensors and their oscillation frequency. The change in the oscillation frequency is recorded for each sensor.
Di Natale et al. (32) used the quartz microbalance to demonstrate a 90.3% accuracy in discriminating between subjects with lung cancer (n=42), healthy volunteers (n=18) and post-surgery lung cancer patients (n=9, two tested pre and post-surgery). All lung cancer subjects were correctly identified, and overlap was reported between the healthy control group and the post-surgery group. In 2009 D’Amico et al. (29) demonstrated 85% sensitivity and 100% specificity in discriminating lung cancer from healthy non-smokers and 93% sensitivity and 79% specificity when compared to subjects with other lung diseases.
Cyranose 320
The Cyranose 320 is a portable analyzer with 32 built-in carbon-black polymer composite chemiresistors in an array format. The polymer matrix adsorbs VOCs in exhaled breath, and swells causing an increase in electrical resistance. Each polymer chemoresistor has different properties which absorb VOCs to varying degrees producing a differential response across the array. The combined results from the sensors produce a sample ‘smellprint’.
The statistical algorithms for the analysis of patterns include Principal Component Analysis (PCA) to reduce the initial data set from the 32 sensors to a set of 4 principal components that capture the greatest variance of the data. Canonical Discriminate Analysis (CDA) using the factors that demonstrated a significant difference between the patients groups is used to create a model that maximizes the ratio of between-group distance to within-group distance. Wilks’ lambda with a P value <0.05 is utilized to demonstrate a statistically significant difference between patient groups. The distance between group means is quantified with a number called Mahalanobis distance (M-distance). M-distance >3 indicates a high probability of difference between groups (P<0.1). Accuracy or capacity of the prediction model to completely distinguish all members of a clinical group is assessed using the “leave one-out method”. The cross validated value (CVV) is the percentage of participants that were correctly classified to a particular participant group.
Machado et al. (14) demonstrated a difference between the exhaled breath VOC profile of a lung cancer group (n=14) and a control group (n=20) with M-distance of 3.25 and cross validated accuracy (CVA) of 71.6%. However there was no difference between stages or between histopathology subtypes of the lung cancer. When the model created from the training set was validated with a new group of lung cancer subjects (n=14) and control subjects (n=62) the electronic nose was able to discriminate between the two groups with sensitivity 71.4% and specificity 91.9%.
In 2008 Dragonieri et al. (28) also found that it was possible to use the Cyranose 320 to distinguish patients with lung cancer (n=10) and COPD (n=10) with accuracy of 85% (M-distance 3.73), and healthy control subjects (n=10), 90%, (M-distance 2.96).
Colorimetry
The colorimetric analyzer has dots impregnated with chemically sensitive compounds, e.g., metalloporphyrins on a cartridge. Each dot is sensitive to a broad range of VOCs but with varying sensitivity. Adsorption of VOCs to the dots causes them to change color. The cartridges are scanned before and after exposure to the sample, and the change in color of the spots is measured and converted to a number.
Two studies published by Mazzone et al. (27,30) used a colorimetric analyzer to compare the exhaled breath VOCs of subjects with lung cancer and control groups. The first, in 2007, (27) described a model discriminating between VOCs of subjects with lung cancer and control subjects [IPF, PAH, COPD, sarcoidosis, healthy subjects (smokers and non-smokers)] with an error rate of 14.1%. Using an independent validation set of subjects the sensitivity of the model in diagnosing lung cancer was 73.3% and specificity 72.4%. These results were not influenced by the patients’ smoking history (P=0.87), histology (P=0.49), cancer stage (P=0.79) or size of the tumour (P=0.69). In 2012, Mazzone et al. (30) found that a combination of the breath profile and clinical risk factors including age, sex, smoking status and COPD improved the accuracy of the model in discriminating between patients with and without lung cancer [Area Under the Curve (AUC) of the receiver operating characteristic curve (ROC) 0.811]. The same model showed a difference between exhaled breath of subjects with squamous and adenocarcinomas (AUC =0.864), early (I and II) and late stage disease (III and IV) (AUC 0.784) and survival <12 months compared to survival >12 months (AUC 0.770).
Gold particle nanosensor
Peng et al. (19) developed a nanosensor array with 14 gold nanoparticle electrodes overlaid with a mixture of compounds including dodecanethiol, 4-methoxy-toluenethiol, hexanethiol, 11-mercapto-1-undecanol, decanethiol, octadecanethiol, tert-dodecanethiol, 1-butanethiol, 2-ethyl-hexanethiol, 3-methyl-1-butanethiol, 2-mercaptobenzoxazole, 11-mercapto-1-undecanol, 2-mercapto-benzyl alcohol, and 3-methyl-1-butanethiol. When exposed to a breath sample the 14 sensors undergo a reversible change in resistance and are analyzed using principal component and cluster analysis. The 2010 study demonstrated a difference between patterns of healthy subjects and patients with lung, colon and breast cancers, but an overlap was found with patients with prostate cancer. Distinct patient groups were identified in one plot when patient groups were analyzed together.
The study also investigated the VOC composition of exhaled breath for each patient group using a GC-MS. They identified a total of 16 VOCs that varied in concentration between healthy and patient groups; six for lung cancer, six for colon cancer, five for breast cancer, and four in prostate cancer. However, there was overlap in abundance of compounds for each of the cancer groups. Peled et al. (26) used a tailor-made chemical nanoarray of 18 cross-reactive sensors, 2 based on random networks of single-wall carbon nanotubes, and 16 based on spherical gold nanoparticles. The accuracy of the nanoarray in discriminating between malignant and benign disease was 88% with an AUC of 0.986. It was also able to discriminate between early and late disease (accuracy 88% AUC 0.961) and adenocarcinoma and squamous (accuracy 88% AUC 0.974).
Canine detection
Dogs have a highly developed sense of smell with a detection threshold at several parts per trillion. Table 4 summarizes the findings of canine detection of cancer.
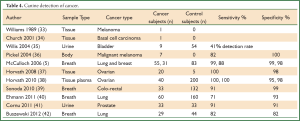
Full table
The premise that dogs may be able to detect cancer by smell was first described in 1989 by Williams (33) who reported the case of a patient’s dog showing an interest in one mole (later identified as melanoma) but not others. In 2001, Church (34) also described the case of a dog sniffing a skin lesion later identified as a basal cell carcinoma. In each case the dogs showed no further interest after the lesions were excised.
The first published research study in 2004 by Willis et al. (35) demonstrated that dogs were able to be trained to detect bladder cancer (1 positive sample in 7) by smelling urine samples. Dogs were successful in 22 out 54 cases (41%) compared with 14% expected by chance. Sonoda et al. (39) trained a dog to detect colorectal cancer in exhaled breath of 33 patients with sensitivity and specificity of 91% and 99%. In 2010 Horvath et al. (38) demonstrated that dogs were able to detect ovarian cancer from tissue and plasma with sensitivity and specificity of 100% and 95%, and 100% and 98% respectively. The dogs were also able to detect early cancer and discriminate between ovarian and other gynaecological malignancies such as endometrial, cervical and vulvar carcinomas. The first study to use dogs to detect lung cancer was by McCulloch et al. (5) in 2006. He trained five dogs to identify exhaled breath samples of subjects with lung cancer and breast cancer. The sensitivity of the canine detection technique for biopsy-confirmed lung cancer (n=55) was 99%, with 99% specificity, while in breast cancer (n=31) the sensitivity was 88% and specificity 98%, with equal accuracy scored by all dogs. The results were different to those of Ehmann et al. (40) who showed that dogs were able to identify lung cancer with sensitivity 71% and specificity 93%. Buszewski et al. (42) compared the chromatographic VOC content of exhaled breath samples of lung cancer subjects (n=29) with canine recognition of lung cancer, and found positive correlations of r=0.85 and 0.97 for ethyl acetate and 2-pentanone while acetonitrile, propanal and 1-propanol were negatively correlated with the dogs’ response to breath samples (r=–0.78, –0.87 and –0.98). He concluded that dogs are probably discriminating between breath samples based on a specific breath odour but it is still unknown what odour or mix of compounds dogs detect.
Although canine scent detection by trained dogs seemed relatively simple and inexpensive, apart from high quality studies performed (43), relatively few published data in general or lung cancer in particular have been reported.
EBC analysis
EBC consists of approximately 99% water vapor (44) as well as a small fraction of respiratory airway lining fluid droplets (45). EBC collection is a simple, safe, non-invasive technique for investigating inflammation and oxidative stress. The EBC can be collected by the subject breathing through a tube inserted in either a metal tube cooled to 0 degrees (R Tube) or through a condenser (Ecoscreen). They breathe tidally for 10-20 minutes through the system and at the end of the time the condensate is collected and analyzed. Studies have shown elevated levels of inflammatory and oxidative stress biomarkers such as hydrogen peroxide, leukotrienes, isoprostanes, hydrogen ions, prostaglandins, and nitrogen oxides (46) in EBC of patients with asthma, COPD, bronchiectasis and cystic fibrosis (47). Studies by Carpagnano et al. (48-53) and Gessner et al. (54) demonstrated they were able to detect human DNA in EBC. A p53 gene mutation (54) was found in 36% of NSCLC subjects while none of the control subjects showed the mutation. Carpagnano et al. (51) demonstrated that microsatellite alterations in EBC-DNA were more frequent in subjects with NSCLC (89%) compared with healthy control subjects (35%). A recent publication from Carpagnano et al. (48) demonstrated a significant difference (P<0.001) in EBC matrix metalloproteinase 9 (MMP-9) concentrations between NSCLC and control subjects with transudative pleural effusion (25 vs. 2.6 ng/mL). The authors also described positive correlations between MMP-9 concentration and pack years smoking history (r=0.8, P<0.0001) and stage of lung cancer (r=0.6, P<0.01). However there was no correlation with the histopathological type of lung cancer.
Methodological limitations
The main limitation of exhaled breath analysis is the lack of recommended guidelines in the sampling of exhaled breath. As seen in Table 5 there have been many techniques used. The methods vary on whether the inhaled room air is filtered (14,19), or unfiltered (5,27-30,40); the period of tidal breathing prior to collecting breath samples (0-12 minutes); the technique used to collect the breath sample, e.g., tidal breath sample (27,29,30,40), vital capacity (5,14,28), or alveolar breath sample (19); and what system was used to collect the sample, one (14,27-30), two bag sampling system (19) or cylindrical polypropylene sampling tubes (5,40). There are also many variations on the statistical analysis. Finally, there has been no comparison between different equipment.
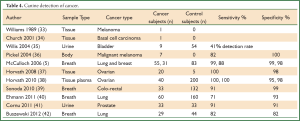
Full table
Applying VOCs analysis in lung cancer
Post lung cancer resection results
Phillips et al. (22) used GC-MS to analyze the exhaled breath of untreated lung cancer subjects and healthy controls from which he developed a mathematical model to discriminate between the two subject groups. The model was tested on 80 post-surgical patients and found that it was positive in 77/80 subjects indicating that the VOC profile was unchanged post-surgical resection. These findings suggested that the VOCs do not come from the tumor itself but rather from other tissues. Poli et al. (15) selected thirteen VOCs using GC-MS that discriminated between lung cancer subjects and control groups. He retested 26 subjects 15-30 days post-surgery and found that two of the thirteen previously identified VOCs, isoprene and decane, altered significantly. When retested at 1 month (n=21), Poli et al. (55) showed that isoprene continued to be significantly decreased from
pre-surgery levels while at 3 years (n=10), 5 VOCs were significantly different; isoprene and benzene were decreased while pentane, toluene and ethylene benzene levels were increased. When compared to control subjects, post-surgical subjects at three years had no significant difference in levels of benzene, heptanes and octanes. These findings again suggest that the exhaled breath VOCs measured in lung cancer subjects are not produced by the tumor.
Validation studies
Machado (14), Phillips (22), and Mazzone (27) have performed validation studies using an independent group of subjects to test the model developed from their training set of subjects. See Table 6 for summary of results. Machado et al. (14) tested the exhaled breath of lung cancer subjects, healthy control subjects and subjects with other respiratory conditions including asthma, COPD and pulmonary hypertension with the Cyranose 320 analyzer. He used support vector machine (SVM) analysis, a learning algorithm, to discriminate between exhaled breath samples of different subject groups. The developed model was accurate in 85% of the subjects. The analyzer had a sensitivity of 71.4%, a specificity of 91.9%, a positive predicted value (PPV) for lung cancer of 66.6% and a 93.4% negative predictive value (NPV). In 2007, Phillips (22) published a study using fuzzy logic to develop a model of breath biomarkers for lung cancer. He tested this model using GC-MS on the exhaled breath of 135 subjects, 65 lung cancer subjects and 70 healthy control subjects. It had 84.6% sensitivity, 80% specificity and 0.88 AUC. Mazzone (27) when using the colorimetric analyzer produced lower sensitivity and specificity values (73.3%, 72.4%) when testing his model on an independent group of subjects with lung cancer, sarcoidosis, IPF, PAH, COPD and healthy subjects. However, the sensitivity was 100% and specificity 60% when a group of subjects (n=29) with indeterminate pulmonary nodules <30 mm was tested.

Full table
Factors which may confound VOC analysis results
Age
Using GC-MS Phillips et al. in 2000 (56) investigated the effect of age on alkane contours in 102 normal subjects. He found that there was a statistical significant difference between age groups 9-31 and 46-89. This was different to Dragonieri et al. (17), Mazzone et al. (27), and Wehinger et al's. (57) findings who used Cyranose 320, colorimetry and proton transfer reaction MS. Dragonieri et al. demonstrated that there was no significant difference in smellprints between age groups <45 and >45 years in 20 normal subjects. Mazzone et al. also demonstrated no difference in results (27) with age (P=0.96). Wehinger et al. using PTR-MS for VOC 31 (believed to be formaldehyde) and 43 (believed to be isopropanol) showed no differences with age. Peng et al. (19) again found no difference with age using the gold particle nanosensor.
Airway calibre
Lazar et al. (58) found with the Cyranose 320 that the exhaled breath profile in 10 asthmatics was altered by nebulisation of methacholine and isotonic saline but was not altered by the airway calibre.
Smoking
Gordon et al. (59) demonstrated with GC-MS in 5 smokers and 5 non-smokers that cigarette smoke affected the volatile organic composition of their exhaled breath. However, the level of the measured VOCs returned to an approximate baseline after 15 minutes. As part of a study of lung cancer and healthy controls using the Cyranose 320, the exhaled breath profiles of current and non-smokers in both healthy subjects and those with disease were found by Machado et al. (14) not to be different and he concluded that the difference between the subject groups was most likely due to the disease process and not to smoking. This was supported by Mazzone et al. (27) whose colorimetry results were not affected by the patients’ smoking history, (current, former or non-smoker) (P=0.87). Peng et al. (19) also found that the subjects’ smoking habits did not affect the results using the gold particle nanosensor. Phillips et al. (22,60) used GC-MS to analyze the exhaled breath of subjects with lung cancer and subjects with a smoking history and a negative CT for lung cancer and developed a model to discriminate between the two patient groups. The accuracy of the model was tested on an independent group of subjects. On evaluating the ROC curves for current and former smokers he found no difference between the two groups. Fens et al. (61) findings in a COPD and asthma study using the Cyranose 320 also showed no difference in breathprints between current and ex-smokers (P=0.16).
Lung function severity
Machado et al. (14) used the Cyranose 320 in his breath analysis and indicated that the severity of lung dysfunction did not affect the clustering of samples.
Gender
Several studies have showed that gender has no effect on the profile (19,27,57).
Conclusions
This review has demonstrated a consistent association between patterns of VOCs in exhaled breath, and genetic markers in EBC, and the presence of lung cancer.
Historically, canine detection of lung cancer was reported to be highly sensitive and specific but it still requires further validation and replication in larger trials to establish its accuracy. Studies examining exhaled breath using GC and mass spectrometers have identified individual chemical compounds associated with lung cancer and confirmed that there is not one VOC but rather a combination of VOCs that are either increased or decreased in concentration. These techniques have limited applicability in the clinical setting because of their expense, difficulty of use, and the need for highly experienced analysts to operate and interpret the results. Electronic noses and related instruments are simpler, cheaper and easier to use, facilitating their utilization in the clinical setting. These instruments employ different technologies to identify VOC patterns. Studies using a variety of these instruments, sampling techniques, and different statistical analyses have consistently discriminated between groups of patients with lung cancer and control subjects. No combination of the instruments, methodologies or statistical analysis has yet been shown to reliably predict which patients in an at risk population are likely to have lung cancer. There will obviously need to be some consensus regarding the most appropriate instruments, collection techniques and statistical methods to optimise the accuracy of the identification of at risk patients for lung cancer. Further validation of the models developed to discriminate between lung cancer and control groups will also be required to determine the sensitivity and specificity of the techniques. With these limitations, exhaled breath analysis does hold great promise because of its simplicity and low cost as a new screening and diagnostic technique in lung cancer.
EBC, a technique which can allow quantification of genetic markers, also shows promise, but does not at this stage have an established place in the screening of lung cancer primarily because it requires a sophisticated genetics laboratory to analyze the samples.
Acknowledgements
Disclosure: The authors declare no conflict of interest.
References
- Jemal A, Center MM, DeSantis C, et al. Global patterns of cancer incidence and mortality rates and trends. Cancer Epidemiol Biomarkers Prev 2010;19:1893-907. [PubMed]
- Parkin DM, Bray F, Ferlay J, et al. Global cancer statistics, 2002. CA Cancer J Clin 2005;55:74-108. [PubMed]
- Mountain CF. Revisions in the international system for staging lung cancer. Chest 1997;111:1710-7. [PubMed]
- Buszewski B, Kesy M, Ligor T, et al. Human exhaled air analytics: biomarkers of diseases. Biomed Chromatogr 2007;21:553-66. [PubMed]
- McCulloch M, Jezierski T, Broffman M, et al. Diagnostic accuracy of canine scent detection in early- and late-stage lung and breast cancers. Integr Cancer Ther 2006;5:30-9. [PubMed]
- Pauling L, Robinson AB, Teranishi R, et al. Quantitative analysis of urine vapor and breath by gas-liquid partition chromatography. Proc Natl Acad Sci U S A 1971;68:2374-6. [PubMed]
- Phillips M. Method for the collection and assay of volatile organic compounds in breath. Anal Biochem 1997;247:272-8. [PubMed]
- Phillips M, Gleeson K, Hughes JM, et al. Volatile organic compounds in breath as markers of lung cancer: a cross-sectional study. Lancet 1999;353:1930-3. [PubMed]
- Mazzone PJ. Analysis of volatile organic compounds in the exhaled breath for the diagnosis of lung cancer. J Thorac Oncol 2008;3:774-80. [PubMed]
- Stone BG, Besse TJ, Duane WC, et al. Effect of regulating cholesterol biosynthesis on breath isoprene excretion in men. Lipids 1993;28:705-8. [PubMed]
- Kneepkens CM, Lepage G, Roy CC. The potential of the hydrocarbon breath test as a measure of lipid peroxidation. Free Radic Biol Med 1994;17:127-60. [PubMed]
- Phillips M, Herrera J, Krishnan S, et al. Variation in volatile organic compounds in the breath of normal humans. J Chromatogr B Biomed Sci Appl 1999;729:75-88. [PubMed]
- Phillips M, Cataneo RN, Cummin AR, et al. Detection of lung cancer with volatile markers in the breath. Chest 2003;123:2115-23. [PubMed]
- Machado RF, Laskowski D, Deffenderfer O, et al. Detection of lung cancer by sensor array analyses of exhaled breath. Am J Respir Crit Care Med 2005;171:1286-91. [PubMed]
- Poli D, Carbognani P, Corradi M, et al. Exhaled volatile organic compounds in patients with non-small cell lung cancer: cross sectional and nested short-term follow-up study. Respir Res 2005;6:71. [PubMed]
- Barker M, Hengst M, Schmid J, et al. Volatile organic compounds in the exhaled breath of young patients with cystic fibrosis. Eur Respir J 2006;27:929-36. [PubMed]
- Dragonieri S, Schot R, Mertens BJ, et al. An electronic nose in the discrimination of patients with asthma and controls. J Allergy Clin Immunol 2007;120:856-62. [PubMed]
- Chen X, Xu F, Wang Y, et al. A study of the volatile organic compounds exhaled by lung cancer cells in vitro for breath diagnosis. Cancer 2007;110:835-44. [PubMed]
- Peng G, Hakim M, Broza YY, et al. Detection of lung, breast, colorectal, and prostate cancers from exhaled breath using a single array of nanosensors. Br J Cancer 2010;103:542-51. [PubMed]
- Fuchs P, Loeseken C, Schubert JK, et al. Breath gas aldehydes as biomarkers of lung cancer. Int J Cancer 2010;126:2663-70. [PubMed]
- Wang Y, Hu Y, Wang D, et al. The analysis of volatile organic compounds biomarkers for lung cancer in exhaled breath, tissues and cell lines. Cancer Biomark 2012;11:129-37. [PubMed]
- Phillips M, Altorki N, Austin JH, et al. Prediction of lung cancer using volatile biomarkers in breath. Cancer Biomark 2007;3:95-109. [PubMed]
- Gordon SM, Szidon JP, Krotoszynski BK, et al. Volatile organic compounds in exhaled air from patients with lung cancer. Clin Chem 1985;31:1278-82. [PubMed]
- Ellis JR, Gleeson FV. Lung cancer screening. Br J Radiol 2001;74:478-85. [PubMed]
- Bajtarevic A, Ager C, Pienz M, et al. Noninvasive detection of lung cancer by analysis of exhaled breath. BMC Cancer 2009;9:348. [PubMed]
- Peled N, Hakim M, Bunn PA Jr, et al. Non-invasive breath analysis of pulmonary nodules. J Thorac Oncol 2012;7:1528-33. [PubMed]
- Mazzone PJ, Hammel J, Dweik R, et al. Diagnosis of lung cancer by the analysis of exhaled breath with a colorimetric sensor array. Thorax 2007;62:565-8. [PubMed]
- Dragonieri S, Annema JT, Schot R, et al. An electronic nose in the discrimination of patients with non-small cell lung cancer and COPD. Lung Cancer 2009;64:166-70. [PubMed]
- D'Amico A, Pennazza G, Santonico M, et al. An investigation on electronic nose diagnosis of lung cancer. Lung Cancer 2010;68:170-6. [PubMed]
- Mazzone PJ, Wang XF, Xu Y, et al. Exhaled breath analysis with a colorimetric sensor array for the identification and characterization of lung cancer. J Thorac Oncol 2012;7:137-42. [PubMed]
- Westhoff M, Litterst P, Freitag L, et al. Ion mobility spectrometry for the detection of volatile organic compounds in exhaled breath of patients with lung cancer: results of a pilot study. Thorax 2009;64:744-8. [PubMed]
- Di Natale C, Macagnano A, Martinelli E, et al. Lung cancer identification by the analysis of breath by means of an array of non-selective gas sensors. Biosens Bioelectron 2003;18:1209-18. [PubMed]
- Williams H, Pembroke A. Sniffer dogs in the melanoma clinic? Lancet 1989;1:734. [PubMed]
- Church J, Williams H. Another sniffer dog for the clinic? Lancet 2001;358:930. [PubMed]
- Willis CM, Church SM, Guest CM, et al. Olfactory detection of human bladder cancer by dogs: proof of principle study. BMJ 2004;329:712. [PubMed]
- Pickel D. Evidence for canine olfactory detection of melanoma. Appl Anim Behav Sci 2004;89:107-16.
- Horvath G, Jarverud GA, Jarverud S, et al. Human ovarian carcinomas detected by specific odor. Integr Cancer Ther 2008;7:76-80. [PubMed]
- Horvath G, Andersson H, Paulsson G. Characteristic odour in the blood reveals ovarian carcinoma. BMC Cancer 2010;10:643. [PubMed]
- Sonoda H, Kohnoe S, Yamazato T, et al. Colorectal cancer screening with odour material by canine scent detection. Gut 2011;60:814-9. [PubMed]
- Ehmann R, Boedeker E, Friedrich U, et al. Canine scent detection in the diagnosis of lung cancer: revisiting a puzzling phenomenon. Eur Respir J 2012;39:669-76. [PubMed]
- Cornu JN, Cancel-Tassin G, Ondet V, et al. Olfactory detection of prostate cancer by dogs sniffing urine: a step forward in early diagnosis. Eur Urol 2011;59:197-201. [PubMed]
- Buszewski B, Ligor T, Jezierski T, et al. Identification of volatile lung cancer markers by gas chromatography-mass spectrometry: comparison with discrimination by canines. Anal Bioanal Chem 2012;404:141-6. [PubMed]
- McCulloch M, Turner K, Broffman M. Lung cancer detection by canine scent: will there be a lab in the lab? Eur Respir J 2012;39:511-2. [PubMed]
- Effros RM, Hoagland KW, Bosbous M, et al. Dilution of respiratory solutes in exhaled condensates. Am J Respir Crit Care Med 2002;165:663-9. [PubMed]
- Effros RM, Dunning MB 3rd, Biller J, et al. The promise and perils of exhaled breath condensates. Am J Physiol Lung Cell Mol Physiol 2004;287:L1073-80. [PubMed]
- Montuschi P. Analysis of exhaled breath condensate in respiratory medicine: methodological aspects and potential clinical applications. Ther Adv Respir Dis 2007;1:5-23. [PubMed]
- Grob NM, Aytekin M, Dweik RA. Biomarkers in exhaled breath condensate: a review of collection, processing and analysis. J Breath Res 2008;2:037004. [PubMed]
- Carpagnano GE, Costantino E, Palladino GP, et al. Microsatellite alterations and cell-free DNA analysis: could they increase the cytology sensitivity in the diagnosis of malignant pleural effusion? Rejuvenation Res 2012;15:265-73. [PubMed]
- Carpagnano GE, Foschino-Barbaro MP, Mule G, et al. 3p microsatellite alterations in exhaled breath condensate from patients with non-small cell lung cancer. Am J Respir Crit Care Med 2005;172:738-44. [PubMed]
- Carpagnano GE, Foschino-Barbaro MP, Resta O, et al. Endothelin-1 is increased in the breath condensate of patients with non-small-cell lung cancer. Oncology 2004;66:180-4. [PubMed]
- Carpagnano GE, Foschino-Barbaro MP, Spanevello A, et al. 3p microsatellite signature in exhaled breath condensate and tumor tissue of patients with lung cancer. Am J Respir Crit Care Med 2008;177:337-41. [PubMed]
- Carpagnano GE, Palladino GP, Martinelli D, et al. Exhaled matrix metalloproteinase-9 in lung cancer. Rejuvenation Res 2012;15:359-65. [PubMed]
- Carpagnano GE, Spanevello A, Carpagnano F, et al. Prognostic value of exhaled microsatellite alterations at 3p in NSCLC patients. Lung Cancer 2009;64:334-40. [PubMed]
- Gessner C, Kuhn H, Toepfer K, et al. Detection of p53 gene mutations in exhaled breath condensate of non-small cell lung cancer patients. Lung Cancer 2004;43:215-22. [PubMed]
- Poli D, Goldoni M, Caglieri A, et al. Breath analysis in non small cell lung cancer patients after surgical tumour resection. Acta Biomed 2008;79:64-72. [PubMed]
- Phillips M, Cataneo RN, Greenberg J, et al. Effect of age on the breath methylated alkane contour, a display of apparent new markers of oxidative stress. J Lab Clin Med 2000;136:243-9. [PubMed]
- Wehinger A. Lung cancer detection by proton transfer reaction mass-spectrometric analysis of human breath gas. Int J Mass Spectrom 2007;265:49-59.
- Lazar Z, Fens N, van der Maten J, et al. Electronic nose breathprints are independent of acute changes in airway caliber in asthma. Sensors (Basel) 2010;10:9127-38. [PubMed]
- Gordon SM, Wallace LA, Brinkman MC, et al. Volatile organic compounds as breath biomarkers for active and passive smoking. Environ Health Perspect 2002;110:689-98. [PubMed]
- Phillips M, Altorki N, Austin JH, et al. Detection of lung cancer using weighted digital analysis of breath biomarkers. Clin Chim Acta 2008;393:76-84. [PubMed]
- Fens N, Zwinderman AH, van der Schee MP, et al. Exhaled breath profiling enables discrimination of chronic obstructive pulmonary disease and asthma. Am J Respir Crit Care Med 2009;180:1076-82. [PubMed]