Signal stratification of autoantibody levels in serum samples and its application to the early detection of lung cancer
Introduction
The technical and clinical validation of an autoantibody (AAb) assay for the early detection of lung cancer (EarlyCDT®-Lung) has recently been described (1-3). In a series of case-control studies, where the cases were newly diagnosed lung cancer patients, circulating AAbs to a panel of tumor-associated antigens (TAAs) were measured in serum samples. Validation of the 7 AAb panel showed that EarlyCDT®-Lung can, with a specificity of 93%, detect elevated levels of AAbs in peripheral blood samples for up to 41% of all primary lung cancers (3). In combination with imaging techniques, the test is now commercially available to assist clinicians in the early detection of lung cancer in a high-risk population.
Currently a single test threshold (“cut-off”) for each AAb measured in the panel classifies the samples into two strata, i.e., positive or negative for AAbs associated with lung cancer. This two-stratum test yields a useful binary classification, but given the range of intervention options available to the clinician, refinement of the result is desirable. A four-stratum test is therefore now proposed with additional sets of low and high cut-offs to classify the results into high positive, positive, negative and low negative strata indicating relatively very high, high, low and very low levels of AAbs, respectively; the level of AAbs measured relates to the probability of lung cancer (i.e., risk of having the disease) (Table 1). This allows more refined intervention for different sub-groups of patients.
Materials and methods
Assay procedure
AAbs to seven TAAs (p53, NY-ESO-1, CAGE, GBU4-5, SOX2, MAGE A4, and HuD) were measured using EarlyCDT®-Lung (Oncimmune USA LLC, De Soto, KS, USA), a commercially available blood test based on indirect enzyme-linked immunosorbent assay (ELISA) methods, that uses microtiter plates coated with semi-log serial dilutions of recombinant antigens (1). AAb levels were measured as optical density units, background-corrected and then converted to calibrated reference units (RU). Each patient serum sample was assayed in duplicate on each plate and a titration curve obtained for each antigen. A sample was declared positive if there was a clear titration curve, and if the RU at either of the two highest points on the titration curve was above its respective cut-off for at least one antigen. Quality control samples were interspersed in the sample order.
Patient samples
Three separate sets of serum samples were used in this work, two case-control sets described previously, and a new population-based set. All patients provided written informed consent for their samples to be used in this study.
Sample set A (Validation case-control)
This set comprised 235 patients with lung cancer from UK, US, Ukraine, and Russia (obtained at or just after diagnosis) representing 87% of the cancers in a previously published dataset (Group 3, n=269) (2) for which enough volume was available to complete the panel of seven AAbs (3). There were 179 non-small-cell lung cancers (NSCLCs, 76%), 53 small-cell lung cancers (SCLCs, 23%), and three others (1%). The controls, all recruited in the US from the general population, came from the same sample set and comprised 266 healthy volunteers with no history of cancer, 235 of whom were matched to the cases by age, gender, and smoking history (2).
Sample set B (Post-validation case-control)
Four groups of patients (Groups 1-4) with newly diagnosed lung cancer, but prior to treatment, plus controls matched by gender, age (±4 years) and smoking history (as far as possible), were combined into a single dataset, as previously reported (4). Group 1 comprised 32 cases with SCLC from a single UK center and Group 2 comprised 161 cases from multiple European centers. Controls (±4 years) came from a prospective collection of cancer-free smokers in the Midlands of England and the Midwest of America. Group 3 comprised 120 cases from a single center in Vancouver, Canada, matched to 113 high-risk lung-cancer-free controls. Group 4 comprised 23 cases matched to 109 controls. The total sample set comprised 336 lung cancer cases, including 301 NSCLC (90%) and 35 SCLC (10%), and 415 normal control sera. The incomplete matching in Groups 2 to 4 was mainly due to controls being excluded if they had been used for another group or if sample volume was insufficient.
Sample set C (Population)
This set comprised 847 commercially-derived samples collected consecutively between November 2010 and February 2012 from individuals deemed by their clinicians as being at high risk of developing lung cancer. Clinical follow-up information available through a prospective audit is known for all these individuals of whom 36/847 (4.3%) were diagnosed [using computed tomography (CT) and/or biopsy] with lung cancer within 6 months after taking the test. Ethnicity was known for 823 (97%) of patients.
Derivation of cut-offs
The current test cut-offs divide the samples into two strata, positive or negative, corresponding to high and low lung cancer risk respectively, so as to maximize the sensitivity for a specificity of about 90% (2). As previously reported (2,3), the specificity was also adjusted for the presence of an estimated small number of undiagnosed cancers in the control group. In the Population dataset, individuals were defined as ‘cancer-free’ if a lung cancer diagnosis was not obtained within six months after testing (manuscript in preparation).
Using sample set A, a new set of high cut-offs, splitting the two-stratum positives, was derived by adding a multiple of the standard deviation of the distribution of controls to the current cut-off for each autoantibody to optimize specificity and sensitivity to yield a high positive predictive value (PPV). Similarly, a set of low cut-offs, splitting the two-stratum negatives, was derived by subtracting multiples of standard deviations to yield a high negative predictive value (NPV). The main calculations were performed assuming a cancer prevalence of 2.7% (2), but tabulation for 4%, being the typical five-year lung cancer risk for an average smoker, was also carried out. All analyses were carried out using SAS® (Version 9.1.3, Cary, NC, USA).
Statistical analysis
The new cut-offs were applied to all datasets, separately and combined, thus sorting patients into four strata on the basis of their EarlyCDT®-Lung AAb levels (Table 1). To check the consistency of the classification, the percentages of samples within the new strata were compared across datasets for cases and controls separately using Fisher Exact tests (5).
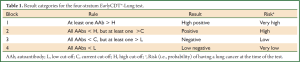
Full Table
Using the specificity and sensitivity for each stratum, the PPV and NPV were then derived using the number of samples in the stratum versus their complement, the samples not in the stratum. A continuous estimate of five-year demographic risk based on gender, age and, where available, smoking history was also derived using a modified version of the Spitz model (6). The demographic factors and staging on the stratification were investigated using multinomial modelling (SAS®, Proc GENMOD and Proc FREQ).
A further analysis compared subjects who could or could not be classified according to the main National Lung Screening Trial (NLST) trial inclusion criteria, i.e., age (55-74 years old) and smoking history (≥30 pack years and quit <15 years ago) (7). In the combined dataset (n=1,802), 531 subjects met the NLST criteria (29%) 116 of whom (22%) were lung cancers, while 1,271 did not (non-NLST) (71%) 328 of whom (26%) were lung cancers (Tables 2,3). There were more under-age subjects than over-age for sets A & B: 21% under-age, 68% NLST and 13% over-age. For sample set C (population set), the figures were 31%, 58% and 11%, respectively. Also, Non-NLST subjects had all smoked less. Two associations were tested (χ2 tests): (I) between cancer status and NLST eligibility for each separate test stratum, and (II) between EarlyCDT-Lung positivity and NLST eligibility for cancers and non-cancer subjects separately.
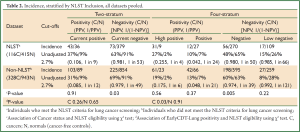
Full Table
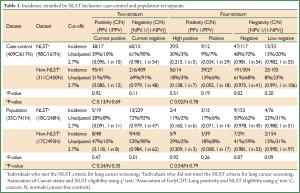
Full Table
Results
Patient samples
The patient demographics were summarized for the sample sets A, B & C separately (Table 4, full details in Table 5). Demographics for sample sets A & B were representative of patients with lung cancer, with more males than females, age ranging from 23 to 90 years and more than half of patients being at least 60 years old. In sample set C (the Population dataset), however, there was a higher percentage of females, suggesting that females are more likely to be proactive about their health, and with a median age of the cases about 10 years older than for the controls. The pattern of smoking was similar over all three datasets, although with a tendency for the cases to be current smokers and controls to be ex-smokers. Mean demographic risk in sample set C was higher for cases than for controls, reflecting the differences noted above.
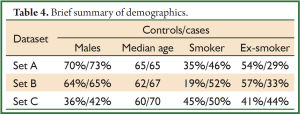
Full Table
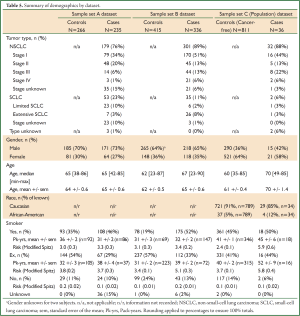
Full Table
Analysis using the current and newly defined test cut-offs
For both the current two-stratum test and the new four-stratum test, using the cut-offs derived from sample set A, the sensitivity, specificity, PPV and NPV were calculated for each dataset and for all datasets combined (Table 6, full details in Table 7). For convenience the NPV is also presented in its reciprocal form (1 in X), i.e., the probability of cancer given a negative result.
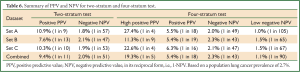
Full Table
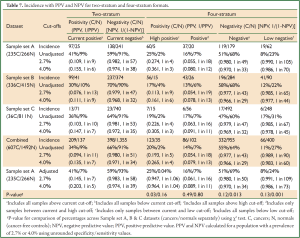
Full Table
Sample set A
The two-stratum test gave a cancer/normal positivity of 41%/9% (PPV =0.109, 1 in 9), and the four-stratum test high positive stratum improved this to 25%/2% (PPV =0.274, 1 in 4) (Tables 6,7). The two-stratum test also gave a cancer/normal negativity of 59%/91% (NPV =0.982, 1 in 57), and the four-stratum test low negative stratum improved this to 8%/23% (NPV =0.990, 1 in 105). For the demographic split, no difference (5% level) between strata was seen for gender (P=0.99), age category (P=0.053) or smoking status (P=0.37), similarly for staging profile (P=0.16).
Sample set B
The two-stratum test gave a cancer/normal positivity of 30%/10% (PPV =0.076, 1 in 13), and the high positive stratum improved this to 17%/4% (PPV =0.113, 1 in 9). The two-stratum test also gave a cancer/normal negativity of 70%/90% (NPV =0.979, 1 in 47), and the low negative stratum improved this to 12%/22% (NPV =0.985, 1 in 65) (Table 6). No difference between strata was seen for gender (P=0.88), age category (P=0.62) or smoking status (P=0.57), similarly for staging profile (P=0.21).
Sample set C
The two-stratum test gave a cancer/normal positivity of 36%/9% (PPV =0.103, 1 in 10), and the high positive stratum improved this to 19%/2% (PPV =0.226, 1 in 4). The two-stratum test also gave a cancer/normal negativity of 64%/91% (NPV =0.981, 1 in 53), and the low negative stratum improved this slightly to 17%/31% (NPV =0.985, 1 in 67) (Table 6). Again, no difference between strata was seen for gender (P=0.20), age category (P=0.07) or smoking status (P=0.51), similarly for the proportion of Caucasians (P=0.22). There were too few cancers to investigate staging.
Combined set
The three datasets were pooled into a single Combined dataset, with 607 cases and 1,492 controls. The two-stratum test gave a cancer/normal positivity of 34%/9% (PPV =0.094, 1 in 11) and the high positive stratum improved this to 20%/2% (PPV =0.193, 1 in 5). The two-stratum cut-offs also gave a cancer/normal negativity of 66%/91% (NPV =0.980, 1 in 51) and the low negative stratum improved this to 11%/27% (NPV =0.989, 1 in 90) (Table 6). Further analysis showed clear consistency of the EarlyCDT-Lung risk profile across age decades (Tables 8,9).
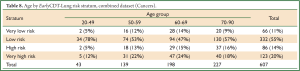
Full Table
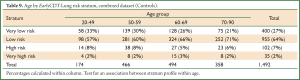
Full Table
Comparison across datasets
The positivity percentages were consistent across the three datasets for both cases and controls (Table 6). For the low negative stratum, the percentage of negatives in sample set C ‘controls’ (31%) was higher than for the other two datasets (23% and 22% respectively, P=0.001), which could reflect the higher number of younger cancer-free individuals in the population sample set C. Even despite the age difference, some dataset-to-dataset variation is to be expected, and the difference was not great. This consistency confirmed that the new sample set A cut-offs were directly applicable to sample sets B & C.
Risk analysis
In standard demographic models (e.g., Spitz) (6), risk increases with age and degree of smoking. To assess the independence of demographic risk and EarlyCDT-Lung result, a single threshold was applied to demographic risk to classify samples into low and high risk. This allowed 2×2×2 tables of positivity (demographic risk, EarlyCDT-Lung result, cancer status) to be compiled, bearing in mind the matching in the case-control sets. No evidence was found for a departure from independence (proportionality) of demographic risk and EarlyCDT-Lung.
The modification of the personalized continuous demographic risk by the four-stratum test is also under investigation. Based on DLR (diagnostic likelihood ratio) calculations (8) for typical cases (e.g., middle-aged moderate smokers), going from a positive result in the two-stratum test to a high positive result in the four-stratum test changed the risk increase from 4.3- to 12.7-fold. Similarly, going from a two-stratum negative result to a four-stratum low negative result changed the risk decrease from 1.5- to 2.9-fold.
EarlyCDT-Lung and NLST criteria
There was no evidence that the cancer rate differed between NLST and Non-NLST cohorts at the positive end of the test (Table 2). At the negative end, there were some small differences, but these were not consistent across sample sets A & B (more cancers in the Non-NLST cohort) and sample set C (Population dataset) (more cancers in the NLST cohort). Statistical significance was generally only seen when the table frequencies were high, and in fact, the differences were not large in the NPV estimates.
There was also little evidence for a difference between NLST and Non-NLST cohorts in their positivity profile. The only comparison significant at 5% was for the sample sets A & B cancers in the four-stratum test where there were more high positives in the NLST cohort (30%) than in the Non-NLST (18%) (Table 3), but this finding was not repeated in sample set C (Population dataset).
Discussion
Improvements in diagnostic test sensitivity and specificity, and hence PPV and NPV, facilitate clinical intervention decisions. This report confirms that the addition of high and low cut-offs to EarlyCDT®-Lung enables stratification of patients into very high risk for lung cancer, with improved PPV, or very low risk, with improved NPV.
Three lung cancer case-control sets were assessed. The case demographics were representative of patients with lung cancer: a predominance of males, more than half of patients >60 years of age, and over half the patients with early-stage lung cancer (i.e., NSCLC stages 1 or 2 or limited SCLC).
For the high positive stratum the specificity was set at 98%. In sample set A, this lowered the sensitivity from 41% for the positive stratum to 25%, but overall the PPV was greatly increased from 10.9% (1 in 9) to 27.4% (1 in 4). Similarly, for the low negative stratum the NPV increased from 98.2% (1 in 57) to 99.0% (1 in 105). The cost for this improvement is reduced performance for the two intermediate strata; for the positive stratum the PPV fell to 5.5% (1 in 18), whilst for the negative stratum the NPV fell to 98.0% (1 in 49).
Importantly, the consistency of performance when applied to sample sets B and C was found to be excellent (Tables 6,7). These data suggest that the EarlyCDT®-Lung measurements may provide a continuous variable in terms of lung cancer risk. We term this the Occurrence ScoreTM and it is under development.
There was no evidence for an association between demographic factors and EarlyCDT-Lung strata. The analysis clearly suggested that EarlyCDT®-Lung is adding to demographic risk independently.
The varied origin of the sample sets supports the general applicability of the results. Nodule data was not available for the case-control datasets. In the Population dataset, however, a positive EarlyCDT®-Lung result did add to the risk of a lung nodule being cancer (manuscript in preparation). The described AAb technology and CT imaging are potentially additive rather than competitive since the presence of AAbs provides an opportunity for early detection of lung cancer, even in early-stage disease, and may therefore be useful in the management of high-risk individuals. Thus, for example, combining a low negative EarlyCDT®-Lung result with a negative CT scan would lead to a very high NPV (manuscript in preparation).
Finally, this study compared the EarlyCDT-Lung strata with whether or not patients met the entry criteria for the NLST study. Only 65% of participants in the Prostate, Lung, Colorectal and Ovarian Cancer Screening Trial (PLCO) who developed lung cancers met the NLST criteria (9), and in another recent US study of early stage lung cancer patients (n=267) fewer than half met the criteria and would not be covered under current screening paradigms (10). In our analysis of EarlyCDT-Lung, little evidence was found that the cancer rate differed between NLST and Non-NLST cohorts, indicating that EarlyCDT-Lung provides similar risk stratification for these cohorts. Thus we can now identify individuals initially deemed at a risk lower than the NLST criteria whose risk after EarlyCDT-Lung is equivalent to the entry criteria for the NLST. This provides a rationale for identification and CT screening of individuals who fall outside the NLST criteria.
Conclusions
EarlyCDT-Lung is recommended as a tool for physicians to assess a patient’s probability of lung cancer thereby facilitating the early detection of lung cancer. By applying two additional cut-offs, we have converted the test to a four-stratum version to allow further stratification of patients into different risk categories. This enhanced stratification can be used on a population that fulfills the NLST criteria to identify super high risk sub-groups. In addition, we have shown that EarlyCDT-Lung can increase the risk estimates for certain Non-NLST patients, and bring them into the NLST range, thus facilitating more appropriate intervention for such patients.
Acknowledgements
Funding: This work was supported by the University of Nottingham and Oncimmune Ltd.
The manuscript was drafted by the authors. However, the authors would like to acknowledge and thank Varinia Munoz, from Complete Medical Communications, who provided editorial assistance funded by Oncimmune Ltd. Thanks also to Jared Allen, Oncimmune Ltd., for data-processing and IT support.
Disclosure: JFRR is Chief Scientific Officer and a shareholder in Oncimmune Ltd, a University of Nottingham spinout company; G. Hamilton-Fairley is a shareholder and Executive Chairman of Oncimmune Ltd; G. Healey is an employee of Oncimmune Ltd.; L. J. Peek is an employee of Oncimmune LLC. All remaining authors have declared no conflicts of interest.
References
- Murray A, Chapman CJ, Healey G, et al. Technical validation of an autoantibody test for lung cancer. Ann Oncol 2010;21:1687-93. [PubMed]
- Boyle P, Chapman CJ, Holdenrieder S, et al. Clinical validation of an autoantibody test for lung cancer. Ann Oncol 2011;22:383-9. [PubMed]
- Chapman CJ, Healey GF, Murray A, et al. EarlyCDT®-Lung test: improved clinical utility through additional autoantibody assays. Tumour Biol 2012;33:1319-26. [PubMed]
- Lam S, Boyle P, Healey GF, et al. EarlyCDT-Lung: an immunobiomarker test as an aid to early detection of lung cancer. Cancer Prev Res (Phila) 2011;4:1126-34. [PubMed]
- Armitage P, Berry G, Matthews JNS. Analysing means and proportions. In: Statistical methods in medical research, 4th edition. Oxford: John Wiley and Sons, 2001:83-146.
- Spitz MR, Hong WK, Amos CI, et al. A risk model for prediction of lung cancer. J Natl Cancer Inst 2007;99:715-26. [PubMed]
- National Lung Screening Trial Research Team, Aberle DR, Adams AM, et al. Reduced lung-cancer mortality with low-dose computed tomographic screening. N Engl J Med 2011;365:395-409. [PubMed]
- Pepe MS. eds. The statistical evaluation of medical tests for classification and prediction. Oxford: Oxford University Press, 2003.
- Tammemagi CM, Pinsky PF, Caporaso NE, et al. Lung cancer risk prediction: Prostate, Lung, Colorectal And Ovarian Cancer Screening Trial models and validation. J Natl Cancer Inst 2011;103:1058-68. [PubMed]
- Taiwo EO, Yorio JT, Yan J, et al. How have we diagnosed early-stage lung cancer without radiographic screening? A contemporary single-center experience. PLoS One 2012;7:e52313. [PubMed]