A richer understanding of intratumoral heterogeneity: single-cell genomics put it within reach
The advent of targeted therapeutics has brought unprecedented benefit to selected patients and pushed anti-cancer treatment shifting towards personalized and precise approaches (1). At the same time, their inherited limitations, mainly referring to acquired resistance, have largely increased our appreciation of intratumoral heterogeneity. Taking EGFR inhibitors as an example, these molecular agents block EGFR signals, significantly increase median progression-free survival (PFS) and improve response rate in NSCLC patients whose tumors have key genetic alterations or ‘oncogenic drivers’ (2). However, despite remarkable initial response, almost all patients develop at certain point resistance to the agents. This nature of cancer response to targeted therapies reinforced the notion that a bulk tumor is molecularly heterogeneous and consists of transformed cells harboring distinct genetic profiles which predispose cells with different sensitivity to therapeutics. This type of tumor heterogeneity is designated as “intratumoral heterogeneity”, in contrast to “intertumoral heterogeneity” which describes the difference in morphology and expression of histopathological markers in different subtypes of cancer (3).
Intratumoral heterogeneity is defined as an uneven distribution, spatially or temporally, of genomic diversification in an individual tumor, fostered by accumulated genetic mutations. It is understood based on knowledge attained from in vitro or small retrospective cohort studies that the oncogenesis occurs through a sequential acquisition of genetic, transcriptomics, and epigenetic alterations that configure uncontrolled and destabilized cellular programs, such as evasion of cell cycle control, enhanced cellular proliferation, over-induced angiogenesis (4). Transformed cells possessing these conferred growth advantages subsequently acquire an elevated spontaneous mutation rate leading to increased levels of genomic instability, thus the expanding tumor population are impelled to create additional genetic alterations. A direct consequence of this multi-step transformation procedure is the genetically abnormal and heterogeneous multiple clones, including primary clones and subclones (5). Distinct subclones can evolve at different stages during oncogenesis (temporal), and can also reside at different regions (spatial) (6,7). These stochastic genetic alterations are driving forces behind intratumoral heterogeneity, and the latter is further augmented and maintained by selective pressures, e.g. therapeutic pressures. Under pressures, certain subclones can acquire an accelerated mutation rate; some subclones harboring sensitive mutations to a certain therapeutics might develop de novo resistance mutations which further prevail to be dominant mutations (8). As a result, cancers generally evolve into a more heterogeneous state during the course of oncogenesis.
Recently, powered by advances in sequencing techniques, a longitudinal study was initiated to investigate intratumor heterogeneity in lung cancer (9). This study, entitled the TRACERx (Tracking Non-Small-Cell Lung Cancer Evolution through Therapy), prospectively profiled using whole-exome sequencing multiregion biopsies of treatment naïve NSCLC and analyzed somatic mutation and chromosomal instability in multiple tumor regions. By defining the clonal and subclonal events, tumor evolutionary processes were determined and correlated with clinical outcome. This prospective study established the proof for the first time in a large NSCLC cohort that intratumor heterogeneity and branched evolution is a universal event and is associated with an increased risk of recurrence or death. Moreover, this study demonstrated the superior ability of multiregion profiling to single tumor sampling in understanding tumor biology, especially driver mutations and tumor evolution (9). This study excels itself as a milestone in delineating tumor heterogeneity. Nevertheless, future similar studies are expected to inspect tumor microenvironment, yet another important component, in relation to tumor cell genetics in shaping the heterogeneous intrinsicality of cancer, favorably at single cell level.
Tumor microenvironment and its interaction with tumor cells make individual tumors more heterogeneous. The number of locally infiltrated immune cells, the function of infiltrated immune cells, the relative composition of different immune cells around tumor cells, and local concentration of immune molecules such as cytokines, together with malignant cells, make up individual and distinct ecosystems within a tumor. The dynamic interactions between these cells shape the ecosystems to a either immunoactive or immunosuppressive status, which is not only imperative for tumor evolutionary fitness but determinant for keeping tumor cells in check and unleashing potential of immunotherapy (10). In nearly all clinical trials, PD-1– and PD-L1–blocking antibodies in advanced NSCLC obtained an objective response rate (ORR) of ~20%, leaving much room for improvement. The unsatisfied ORR mainly roots from inferior correlation between current biomarkers for the selection of patients to receive immune checkpoint inhibitors and intrinsic tumor immunogenomic landscape. The current predictive marker for anti-PD-1/PD-L1 response is PD-L1 expression. However the expression of PD-L1 is detectable on tumor cells as well as tumor infiltrating immune cells. Moreover PD-L1 expression might vary among different sites of the same tumor, as demonstrated by a recent study in which co-existence of PD-1 high and PD-1 low T cells were detected in a tumor, which might confer reverse anti-tumor immune effect (11). More importantly, diverse factors are engaged within tumor microenvironment and T cell exhaustion, including PD-1/PD-L1 but also the number of Tregs and the expression level of other immune checkpoints. These hallmarks highly suggest that the local immune contexture of tumors is profoundly heterogeneous and dynamic. To dissect this multicellular local ecosystem and decode the complicated communication between tumor cells and immune cells as well between different immune cells are the keys to improve the efficacy of immunotherapy.
In the past decades, cancer genomes have been intensively investigated at multiple-omics levels, with considerable novel insight obtained with respect to cancer development, progression, tumor intratumoral heterogeneity, as well as response to therapeutics intervention (12,13). Nevertheless, these studies are all conducted with methodologies developed for analyzing “bulk” populations of cells, including the prospective study in NSCLC published recently (9). The molecular profiles derived from such mixed cell populations are exclusively an average scenery of the studied tumor, no information about the inherent variation within the tumor. In case other cells, for example immune cells or stroma cells, co-exist in the profiled “bulk” population—which is unfortunately inevitably in most cases, the cancer signatures are to certain extent diluted or contaminated. The “bulk” cell molecular profiles therefore are not able to draw maps of heterogeneous tumor cells and their states, and to depict how the changes in the map underlie the cancer response to the therapy.
Sampling at multiple sites can only partially overcome this problem. The clustering and evolutionary study of subclones within same regions revealed that most tumor regions (86%) were found to carry subclones evolved from the same ascendant branch in the phylogenetic tree, indicating genetic diversification is a spatially common event within a tumor (14). As indicated by this study, bulk tumor cell profiles sampled from multiple sites definitely enable us gain deeper insight into heterogeneity of tumor genomes. Yet this strategy is only applicable to tumors whose spatial heterogeneity is characterized by uneven distribution of key molecular alterations across different regions of the tumors. For the tumors which have ubiquitous presence of key molecular driver alterations, but an unequal distribution of additional molecular alterations or passenger mutations, multiregion sampling is not able to provide in-depth insight into the full extent of intratumoral heterogeneity (15). In addition, multiregion sampling is yet a population-based study on a mixture of different cells. If no isolation of individual cells follows the yielded profile resolution is insufficient to unlock cell type specific contribution to the ecosystems. The underestimation of intratumoral heterogeneity is remaining unsolved and still the major challenges to personalized-medicine and biomarker development.
In parallel, cancer local immune status has been studied with increasing resolution, attributing to advances in cellular biology, for instance the application of flow cytometry. With these techniques, the quantitative presence of certain infiltrating immune cells can be assessed by detecting surface markers expressed on that sort of immune cells, such as CD3, CD4, and CD8 for T cells, and CD19 for B cells (16). Estimating local immunoprofiles through measuring limited number of markers has apparent disadvantages. First, most surface markers lack cell specificity and are expressed in multiple cell populations. Second, this type of measurement is phenotypic, leading to divergences between marker expression and cell functionality as well as discrepancies in existing studies. Third, there are a large number of immune cell subtypes, and distinguishing subtypes requires a sequential detection of multiple markers, which is highly labor-, time-, and cost-inefficient. The application of next generation sequencing (NGS) has largely facilitated the understanding of immunome. The utility of flow cytometry and NGS in combination has made it possible to obtain genetic, transcriptomic, and epigenetic profiles of purified immune cells (16). Still, the isolation of all immune cell subtypes by flow cytometry for subsequent DNA- or RNA-sequencing is tremendous effort and cannot be widely implemented.
Despite substantial advances made, we are still at the beginning of understanding multiple dimensions of a tumor and dissecting the interactive cellular ecosystems, mainly local immunomes, in a tumor. The single-cell-resolution information is greatly demanded to an unprecedented extent.
The cutting-edge single-cell profiling technologies such as single-cell RNA sequencing can overcome the abovementioned challenges (Figure 1). With single-cell technologies over thousands cells, from one site or multiple sites, of a tumor sample can be spontaneously profiled, with or without cell isolation by flow-cytometry or microfluidics. This technique was used to characterize heterogeneous gene expression within individual tumors and to correlate the loss of essential genes with efficacy of checkpoint blockade immunotherapy (17). The single-cell sequencing of different cancers also demonstrated the promise of this technique to identify novel oncogenes or novel variants of previously known cancer genes (18,19). Furthermore, single-cell sequencing might empower greatly deconvolution of the complex clonal relationships encompassed by a bulk tumor. For example, by tracking sequential mutations or other markers between individual breast cancer cells Navin et al. reconstructed evolutionary model whereby clonal subpopulations arise from punctuated clonal expansions rather than gradual evolution (20). Last but not least, single-cell sequencing can be applied to quantify the extent of heterogeneity which might guide targeted therapy such as EGFR-TKI (21).
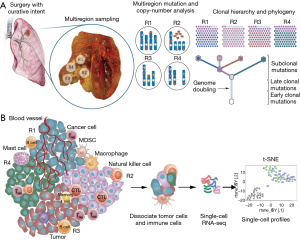
We might even reveal clonality or new immune cell types not evident from population-based studies (22,23). By performing deep single-cell RNA sequencing on T cells isolated from hepatocellular carcinoma (HCC) patients, in total eleven T cell subsets were revealed which showed independencies with each other with respect to phenotype, differentiation status, and functionality that are not obtained using bulk cell-based data (24).
Single-cell technology will also allow us to uncover the interdependency between tumor cells and immune cells in the context of ecosystems: how all these cells differentially modulate each other during cancer development and progression, how these cells convert from one state to another when receiving signals from microenvironment. Ultimately, this added knowledge will help us link tumor molecular signatures with cancer patients’ outcome, and promote a new shift of cancer treatment. Although very few studies have employed single-cell techniques due to mostly high cost and sophisticated bioinformatics requirements, the authors are convinced that it will be clinically implemented in few years with a sharp dropping of sequencing cost and quick development of the corresponding analytic techniques. A recently launched international consortium, The Human Cell Atlas, is aiming to build up a systematic gene expression and its regulation landscape of each type of cells across major organs under healthy as well as disease states, at the extreme level of resolution allowed by single-cell profiling (25). The output of this project will provide a plethora perspective on immune function and dysfunction in cancers, as accomplished by Human Genome Project.
Acknowledgements
None.
Footnote
Conflicts of Interest: The authors have no conflicts of interest to declare.
References
- Jordan EJ, Kim HR, Arcila ME, et al. Prospective Comprehensive Molecular Characterization of Lung Adenocarcinomas for Efficient Patient Matching to Approved and Emerging Therapies. Cancer Discov 2017;7:596-609. [Crossref] [PubMed]
- Wu YL, Cheng Y, Zhou X, et al. Dacomitinib versus gefitinib as first-line treatment for patients with EGFR-mutation-positive non-small-cell lung cancer (ARCHER 1050): a randomised, open-label, phase 3 trial. Lancet Oncol 2017;18:1454-66. [Crossref] [PubMed]
- Sutherland KD, Visvader JE. Cellular Mechanisms Underlying Intertumoral Heterogeneity. Trends Cancer 2015;1:15-23. [Crossref] [PubMed]
- Vogelstein B, Papadopoulos N, Velculescu VE, et al. Cancer genome landscapes. Science 2013;339:1546-58. [Crossref] [PubMed]
- Nowell PC. The clonal evolution of tumor cell populations. Science 1976;194:23-8. [Crossref] [PubMed]
- Gerlinger M, Rowan AJ, Horswell S, et al. Intratumor heterogeneity and branched evolution revealed by multiregion sequencing. N Engl J Med 2012;366:883-92. [Crossref] [PubMed]
- Touat M, Dhermain F, Andre F, et al. Adapting the drivers to the road: a new strategy for cancer evolution? Ann Oncol 2015;26:827-9. [Crossref] [PubMed]
- Amirouchene-Angelozzi N, Swanton C, Bardelli A. Tumor Evolution as a Therapeutic Target. Cancer Discov 2017. [Epub ahead of print]. [Crossref] [PubMed]
- Jamal-Hanjani M, Wilson GA, McGranahan N, et al. Tracking the Evolution of Non-Small-Cell Lung Cancer. N Engl J Med 2017;376:2109-21. [Crossref] [PubMed]
- Chen PL, Roh W, Reuben A, et al. Analysis of Immune Signatures in Longitudinal Tumor Samples Yields Insight into Biomarkers of Response and Mechanisms of Resistance to Immune Checkpoint Blockade. Cancer Discov 2016;6:827-37. [Crossref] [PubMed]
- Wei F, Zhong S, Ma Z, et al. Strength of PD-1 signaling differentially affects T-cell effector functions. Proc Natl Acad Sci U S A 2013;110:E2480-9. [Crossref] [PubMed]
- Cancer Genome Atlas Research Network. Electronic address: wheeler@bcm.edu; Cancer Genome Atlas Research Network. Comprehensive and Integrative Genomic Characterization of Hepatocellular Carcinoma. Cell 2017;169:1327-41.e23. [Crossref] [PubMed]
- Robertson AG, Shih J, Yau C, et al. Integrative Analysis Identifies Four Molecular and Clinical Subsets in Uveal Melanoma. Cancer Cell 2017;32:204-20.e15. [Crossref] [PubMed]
- Gerlinger M, Horswell S, Larkin J, et al. Genomic architecture and evolution of clear cell renal cell carcinomas defined by multiregion sequencing. Nat Genet 2014;46:225-33. [Crossref] [PubMed]
- Harbst K, Lauss M, Cirenajwis H, et al. Multiregion Whole-Exome Sequencing Uncovers the Genetic Evolution and Mutational Heterogeneity of Early-Stage Metastatic Melanoma. Cancer Res 2016;76:4765-74. [Crossref] [PubMed]
- Chevrier S, Levine JH, Zanotelli VRT, et al. An Immune Atlas of Clear Cell Renal Cell Carcinoma. Cell 2017;169:736-49.e18. [Crossref] [PubMed]
- Patel AP, Tirosh I, Trombetta JJ, et al. Single-cell RNA-seq highlights intratumoral heterogeneity in primary glioblastoma. Science 2014;344:1396-401. [Crossref] [PubMed]
- Francis JM, Zhang CZ, Maire CL, et al. EGFR variant heterogeneity in glioblastoma resolved through single-nucleus sequencing. Cancer Discov 2014;4:956-71. [Crossref] [PubMed]
- Yu C, Yu J, Yao X, et al. Discovery of biclonal origin and a novel oncogene SLC12A5 in colon cancer by single-cell sequencing. Cell Res 2014;24:701-12. [Crossref] [PubMed]
- Navin N, Kendall J, Troge J, et al. Tumour evolution inferred by single-cell sequencing. Nature 2011;472:90-4. [Crossref] [PubMed]
- Piotrowska Z, Niederst MJ, Karlovich CA, et al. Heterogeneity Underlies the Emergence of EGFRT790 Wild-Type Clones Following Treatment of T790M-Positive Cancers with a Third-Generation EGFR Inhibitor. Cancer Discov 2015;5:713-22. [Crossref] [PubMed]
- Stubbington MJT, Lonnberg T, Proserpio V, et al. T cell fate and clonality inference from single-cell transcriptomes. Nat Methods 2016;13:329-32. [Crossref] [PubMed]
- Villani AC, Satija R, Reynolds G, et al. Single-cell RNA-seq reveals new types of human blood dendritic cells, monocytes, and progenitors. Science 2017;356:eaah4573. [Crossref] [PubMed]
- Zheng C, Zheng L, Yoo JK, et al. Landscape of Infiltrating T Cells in Liver Cancer Revealed by Single-Cell Sequencing. Cell 2017;169:1342-56.e16. [Crossref] [PubMed]
- Rozenblatt-Rosen O, Stubbington MJT, Regev A, et al. The Human Cell Atlas: from vision to reality. Nature 2017;550:451-3. [Crossref] [PubMed]