Evaluation of the performance of serum miRNAs as normalizers in microRNA studies focused on cardiovascular disease
Introduction
MicroRNAs (miRNAs) are a class of endogenous 20–27 nt long RNAs involved in post-transcriptional gene silencing (1,2). In general, their overall function is to modulate or fine-tune cellular phenotypes through controlling the expression of complementary target mRNAs (3). Functional studies have shown that miRNAs play a role in physiological and pathological processes, such as cell proliferation (4,5), fat metabolism (6,7), inflammation (8), apoptosis (9,10) and carcinogenesis (11). Therefore, miRNAs participate in the process of various diseases, including cardiovascular disease (12,13), cancer (14,15) and so on (16,17). In addition, considering the fact that miRNAs remain stable in serum and other body fluids, miRNAs can be used as the biomarker for human disease (18). These findings suggest broad opportunities for developing miRNAs as potential therapeutic targets or biomarkers for human disease.
Circulating miRNAs have been a hot topic as a biomarker for human disease for several years (18-21), but there’s still no consensus on the reference genes in miRNA studies, which makes it difficult to compare between different samples and studies. There’re several major suggested normalization strategies for circulating miRNA studies, small nucleolar RNA (SNOU6, 5S, et al.), miRNAs with stable expression (miRNA-16, let-7i, miR-19b, miR-15b, miR-24, et al.) and exogenous controls (synthetic miRNAs). Among the above normalization strategies, synthetic miRNA molecules, used as spike-in control, cannot correct sample-to-sample variation. And thus, the normalization against a stable reference gene (or better a set of multiple stable reference genes) is currently the most accurate and suitable method for evaluation of circulating miRNAs.
However, despite the fact that those small RNAs were commonly used as reference genes in previous studies, the levels of those reference miRNAs differ in different disease (22-25), indicating that it’s necessary to evaluate the stability and superiority of those previously used reference genes in different diseases. Specially, few previous study has been done to evaluate the stability and superiority of serum in cardiovascular disease. Therefore, the aim of this study is to assess the suitability of SNOU6, 5S, miR-16 as normalizers in cardiovascular disease.
Methods
Patients and control subjects
Serums samples were collected from patients hospitalized for heart failure (HF) or hypertension in Zhongshan Hospital. The blood samples were collected before any therapeutic procedures. Control subjects were recruited from healthy participants during physical examinations performed at Zhongshan Hospital. All participants signed an informed written consent to participate in the study that was approved by Ethical Committee of Zhongshan Hospital, Fudan University, China.
Serum preparation and RNA isolation
Blood samples (~5 mL) were collected from each donor and placed in a serum separator tube. Samples were processed within one hour. Separation of the serum was accomplished by centrifugation at 800 g for 10 min at room temperature, followed by a 15-min high-speed centrifugation at 10,000 g at room temperature to completely remove the cell debris. The supernatant serum was recovered and stored at −80 °C until analysis.
For RNA isolation, miRNA was extracted from 200 µL of each serum sample and eluted in 30 µL of RNase-free water using a miRcute serum/plasma miRNA isolation kit (Tiangen, Beijing, China) according to the manufacture’s instruction.
Selection criteria for the candidate normalizers
We based on our selection of candidate miRNAs from literature review on reference genes with several additional criteria. First, the candidate miRNAs must be highly expressed in human heart tissue, and can be easily detected in serum (26). Second, candidate miRNAs must be unambiguously annotated in miRBase (version 16) (27). Third, candidate miRNAs must not be clustered in the genome with any other candidate miRNA so as to reduce the possibility of co-regulation (28). In addition, two commonly used small RNA controls (SNOU6 and 5S) were also analyzed in this study.
cDNA synthesis and RT-qPCR
The miRNA isolated from blood sample of the same volume was polyadenylated and reverse transcribed to cDNA in a final volume of 20 µL using miRcute miRNA First-Strand cDNA Synthesis Kit (Tiangen, Beijing, China). Real-time PCR was performed in duplicate measurements using miRcute Plus miRNA qPCR Detection Kit (SYBR Green) (Tiangen, Beijing, China). The miRNA-specific primer sequences were designed by a biologics company (Tiangen, Beijing, China). Each amplification reaction was performed in a final volume of 20 µL containing 1 µL of the cDNA, 0.2 mM of each primer and 1× miRcute plus miRNA premix (with SYBR & ROX). At the end of the PCR cycles, melting curve analyses as well as electrophoresis of the products on 3.0% agarose gels were performed in order to validate the specific generation of the expected PCR product. Each sample was run in duplicates for analysis.
Data analysis
Data are expressed in terms of mean and SD for numeric variables and as number (percent) for categorical variables. Comparisons of continuous variables among groups were performed by the Student t-test. Statistic analysis was performed with SPSS 22.0 (SPSS Inc., Chicago, Illinois, USA).
Gene expression stability was evaluated according to four gold-standard statistical approaches: BestKeeper (29), NormFinder (30), and the comparative delta Cq method (31).
Results
Baseline characteristics of total patients
A total of 62 subjects were enrolled, among which there were 25 patients with HF, 10 patients with hypertension, and 27 healthy people. Male patients were more likely to have cardiovascular disease (HF patients vs. hypertensive patients vs. healthy patients: 80% vs. 70% vs. 63%). Patients with cardiovascular disease were older, and have a lower level of hemoglobin (HF patients vs. hypertensive patients vs. healthy patients: 130.81±22.17 vs. 133.90±11.60 vs. 148.74±14.18) and high-density lipoprotein (HF group vs. hypertensive patients vs. healthy patients: 1.13±0.34 vs. 1.30±0.48 vs. 1.33±0.48) (Table 1).
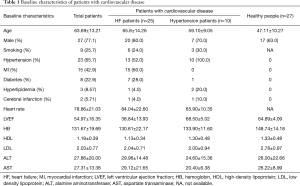
Full table
Reference gene stability calculated by BestKeeper, NormFinder and comparative delta Cq method
The distributions of the qPCR quantification cycle (Cq) values of seven reference genes over the whole sample set were shown in Figure 1. Among the seven candidates, the interquartile range for SNOU6 (2.42) and 5S (2.38) were larger than others, and miR-16 (1.01) and let-7i-5p (1.05) showed a lower interquartile range.
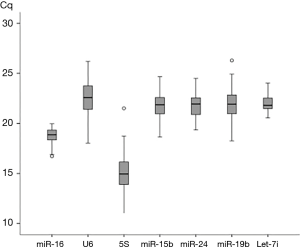
BestKeeper
The descriptive statistics given by BestKeeper are reported in Table 2. The stability of the candidate reference gene is mainly evaluated by standard deviation (SD), correlation coefficient (R), and coefficient of variation (CV) by this software. A high R value, low SD and CV value indicated a stably expressed gene, and SD >1 means that this reference gene is unacceptable. In terms of variability, let-7i displayed the lowest SD values of 0.60, followed by miR-16 (SD: 0.72), while the SD of SNOU6 and 5S showed the highest variability (SD >1). Correlation analysis was performed between each reference gene and BestKeeper index (BKI). This analysis showed that, except for miR-24, all of the rest six miRNAs showed a significant correlation.
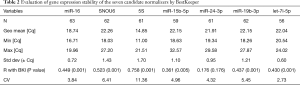
Full table
NormFinder
The stability analysis performed by NormFinder is displayed on Table 3 in terms of stability value (ρ) and inter-group and intra-group variations in total patients. Best overall performance was observed for miR-15b (ρ=0.029) and the smallest intra-group variability (0.002). The worst performance was shown by miR-24 (ρ=0.075), which displayed large values of both intra-group (0.023) and inter-group (0.066). Overall performance was low also for miR-16 (ρ=0.074).

Full table
Comparative delta Cq method
The comparative delta Cq analysis indicated miR-16 and let-7i as the most stable gene, with a lowest average SD of Cq differences for pairwise comparisons (1.78 and 1.82, respectively), followed by miR-15b, miR-19b. The least stable genes were 5S and SNOU6 with average SD of 2.28 and 2.61, respectively (Table 4).
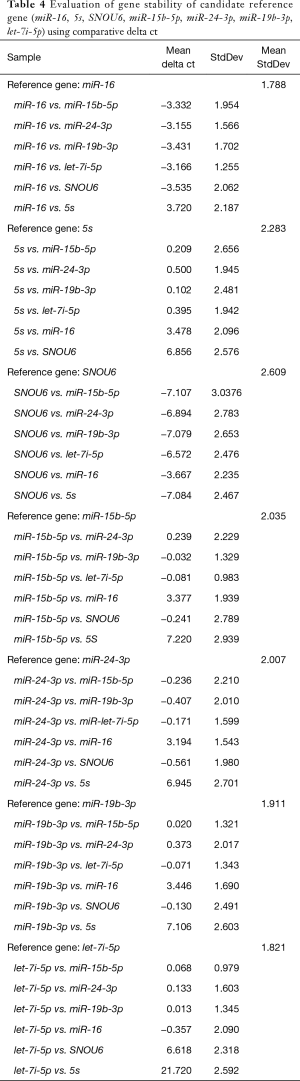
Full table
Overall ranking
The comprehensive ranking of gene stability obtained by combining the three analyses. Let-7i and miR-16 were the two best reference miRNA, followed by miR-15b, miR-19b, miR-24, while SNOU6 and 5S were the least stable gene, displaying the worst performance according to all analyses (Table 5, Figure 2).
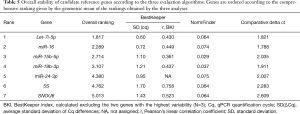
Full table
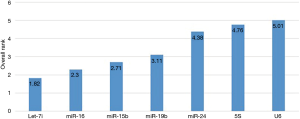
Discussion
This study assessed for the first time the stability properties of the reference genes in serum from patients with cardiovascular disease. In our analysis using a multi-technique quantitative approach, let-7i-5p and miR-16 were the most recommended candidate reference miRNAs in cardiovascular disease (hypertension and HF), followed by miR-15b, miR-19b and miR-24. SNOU6 and 5S were not suitable as normalizers for circulating miRNA research in cardiovascular disease. Our results are of important value for researches focused on serum miRNAs in cardiovascular disease.
qRT-PCR is the golden method for the accurate and sensitive detection in gene expression assay (32-34), it is a powerful technique due to its high sensitivity, specificity, accuracy, and broad quantification range. The accuracy of qRT-PCR results is influenced by numerous factors including the quality of RNA, method of cDNA preparation, PCR efficiency, and very importantly, the selection of reference gene (35). Thus, the selection of an optimal reference gene is critical (30,33), considering that a valid control gene will ensure the real-time PCR data to be reliable for target genes. However, previous studies have shown that the performance of the common recommended reference genes (such as SNOU6, or 5S) varied under different disease or different samples (22,24). And thus, it’s necessary to select an optimal reference gene in the studies of different disease or samples, which can ensure the accuracy of the analysis to some degree. A workflow strategy has been proposed for the normalization of miRNA expression data in an attempt to provide a basis for the establishment of a global standard procedure that will allow comparison across studies (36). Besides, several algorithms, including NormFinder (30), BestKeeper (29), and comparative delta Ct (cycle thresholds) method (31) have been developed for the selection of suitable reference gene.
Some studies have been done to evaluate the suitability of the reference genes in vitro test (37) or animal models (38,39), however, cell or animal models cannot represent the status of human disease precisely. Studies focused on human disease also have been done to identify a valid control gene, but most of the samples were tissues from cancer disease or neurological disease (22,24,40). In terms of HF, studies have shown that HF can affect gene expression levels which may have effects on the disease research (41). Gene expression changes in whole heart tissue have been assessed by previous approaches (42). However, studies for the selection of optimal reference gene of miRNA study focused on cardiovascular disease is few (43). Masè et al. evaluated the stability of the reference genes in the heart tissue focused on atrial fibrillation (43), but there’re some limitations in this study: (I) the samples in this study is the heart tissue from atrial fibrillation patients, and these results can not be applied to serum studies focused on cardiovascular disease; (II) the results can only be used in AF studies, considering that the expression levels of miRNAs differs in different disease; (III) this study failed to compare some other well-known reference genes as control group.
For normalizer miRNA studies in cardiovascular disease, Masè et al. showed that the performance of 5S is the best, while SNOU6 displayed the worst stability. But in our serum study of their stability, miR-16 showed the best stability, while 5S is the worst stable reference gene. The reason for this inconformity may be that the stability of reference genes in tissue is different from serum, indicating that it’s necessary to identify the best housekeeping gene in tissue and serum, respectively. What’s more, previous selection studies of reference gene in serum showed that 5S and SNOU6 maybe are not suitable as a valid control (24,25), which is in consistent with our study.
Some studies have also compared the performance of the selected candidate miRs in other kinds of human disease. For let-7i, Chen et al. evaluated the performance of let-7i/d/g and some other miRNAs (miR-30d, miR-140-3p and so on) as stable reference for normalization of serum miRNAs, and the results showed that let-7i was better that others as the optimal reference gene for normalization, which is in consistent with our results (44). Controversies existed for the suitability of miR-16 as normalizers, several studies identified miR-16 as the most stable reference gene (25,45,46), while the study by Kok et al. showed that a proposed normalization panels is better than miR-16 (47). But we should notice that there’re some limitations in the study by Kok et al. (47): firstly, all of the 3 proposed normalization panels by Kok et al. contains 2 or more miRNAs, and such normalization methods containing several miRNAs are seldom used in the analysis of qPCR; secondly, the miRNAs included in the panels are not very common used; thirdly, they only compared miR-16 with the 3 normalization panels, so we cannot conclude the conclusion that miR-16 cannot be used as normalizer. In consistent with previous studies, miR-15b (21), miR-19b (21) and miR-24 (25,48) showed good performance in other studies, while serum 5s and SNOU6 were unsuitable to be used as reference miRNAs in cardiovascular disease (22,24).
Considering that few studies have identified the optimal reference miRNAs in circulating miRNA research in cardiovascular disease, our study provide important information for further qPCR analysis in miRNA study. The limitation of our study is that we only included patients of HF, hypertension, but considering that both of the expression level and stability of those reference genes varied with the disease status, it’s necessary to assess the suitable of reference genes in more kinds of cardiovascular disease.
Conclusions
This study pointed out that in the serum studies focused on cardiovascular disease, let-7i and miR-16 had the best performance, while SNOU6 and 5S were not suitable as reference gene. This study indicate that the selection of an optimal reference genes is important to get an accurate result in serum miRNA studies, the findings are of clinical significance to guide the further miRNA studies or tests.
Acknowledgements
Funding: This work was supported by grants from the National Natural Science Foundation of China (No. 31430039, 81521001, 81730009) and Shanghai Natural Science Foundation (No. 18ZR1406900).
Footnote
Conflicts of Interest: The authors have no conflicts of interest to declare.
Ethical Statement: This study was approved by the Institutional Ethics Review Board [Zhongshan Hospital, Fudan University Review Board B2012-140(2)]. All participants provided written informed consent prior to the start of the study.
References
- Bartel DP. MicroRNAs: genomics, biogenesis, mechanism, and function. Cell 2004;116:281-97. [Crossref] [PubMed]
- Mallory AC, Vaucheret H. Functions of microRNAs and related small RNAs in plants. Nat Genet 2006;38 Suppl:S31-6. [Crossref] [PubMed]
- Ambros V. The functions of animal microRNAs. Nature 2004;431:350-5. [Crossref] [PubMed]
- Chen JF, Mandel EM, Thomson JM, et al. The role of microRNA-1 and microRNA-133 in skeletal muscle proliferation and differentiation. Nat Genet 2006;38:228-33. [Crossref] [PubMed]
- Luo P, He T, Jiang R, et al. MicroRNA-423-5p targets O-GlcNAc transferase to induce apoptosis in cardiomyocytes. Mol Med Rep 2015;12:1163-8. [Crossref] [PubMed]
- Chai C, Rivkin M, Berkovits L, et al. Metabolic Circuit Involving Free Fatty Acids, microRNA 122, and Triglyceride Synthesis in Liver and Muscle Tissues. Gastroenterology 2017;153:1404-15. [Crossref] [PubMed]
- Cheng L, Zhu Y, Han H, et al. MicroRNA-148a deficiency promotes hepatic lipid metabolism and hepatocarcinogenesis in mice. Cell Death Dis 2017;8:e2916. [Crossref] [PubMed]
- Yin H, Zhang S, Sun Y, et al. MicroRNA-34/449 targets IGFBP-3 and attenuates airway remodeling by suppressing Nur77-mediated autophagy. Cell Death Dis 2017;8:e2998. [Crossref] [PubMed]
- Yang N, Chen J, Zhang H, et al. LncRNA OIP5-AS1 loss-induced microRNA-410 accumulation regulates cell proliferation and apoptosis by targeting KLF10 via activating PTEN/PI3K/AKT pathway in multiple myeloma. Cell Death Dis 2017;8:e2975. [Crossref] [PubMed]
- Ghibaudi M, Boido M, Vercelli A. Functional integration of complex miRNA networks in central and peripheral lesion and axonal regeneration. Prog Neurobiol 2017;158:69-93. [Crossref] [PubMed]
- Hatziapostolou M, Polytarchou C, Aggelidou E, et al. An HNF4alpha-miRNA inflammatory feedback circuit regulates hepatocellular oncogenesis. Cell 2011;147:1233-47. [Crossref] [PubMed]
- Wahlquist C, Jeong D, Rojas-Munoz A, et al. Inhibition of miR-25 improves cardiac contractility in the failing heart. Nature 2014;508:531-5. [Crossref] [PubMed]
- van Rooij E, Olson EN. MicroRNA therapeutics for cardiovascular disease: opportunities and obstacles. Nat Rev Drug Discov 2012;11:860-72. [Crossref] [PubMed]
- Yu T, Guo F, Yu Y, et al. Fusobacterium nucleatum Promotes Chemoresistance to Colorectal Cancer by Modulating Autophagy. Cell 2017;170:548-63 e16.
- Rupaimoole R, Slack FJ. MicroRNA therapeutics: towards a new era for the management of cancer and other diseases. Nat Rev Drug Discov 2017;16:203-22. [Crossref] [PubMed]
- Rottiers V, Naar AM. MicroRNAs in metabolism and metabolic disorders. Nat Rev Mol Cell Biol 2012;13:239-50. [Crossref] [PubMed]
- Mendell JT, Olson EN. MicroRNAs in stress signaling and human disease. Cell 2012;148:1172-87. [Crossref] [PubMed]
- Verma AM, Patel M, Aslam MI, et al. Circulating plasma microRNAs as a screening method for detection of colorectal adenomas. Lancet 2015;385 Suppl 1:S100. [Crossref] [PubMed]
- Gupta SK, Bang C, Thum T. Circulating microRNAs as biomarkers and potential paracrine mediators of cardiovascular disease. Circ Cardiovasc Genet 2010;3:484-8. [Crossref] [PubMed]
- Jin P, Gu W, Lai Y, et al. The Circulating MicroRNA-206 Level Predicts the Severity of Pulmonary Hypertension in Patients with Left Heart Diseases. Cellular Physiology and Biochemistry 2017;41:2150-60. [Crossref] [PubMed]
- Mitchell PS, Parkin RK, Kroh EM, et al. Circulating microRNAs as stable blood-based markers for cancer detection. Proc Natl Acad Sci U S A 2008;105:10513-8. [Crossref] [PubMed]
- Lim QE, Zhou L, Ho YK, et al. snoU6 and 5S RNAs are not reliable miRNA reference genes in neuronal differentiation. Neuroscience 2011;199:32-43. [Crossref] [PubMed]
- Wang Y, Tang N, Hui T, et al. Identification of endogenous reference genes for RT-qPCR analysis of plasma microRNAs levels in rats with acetaminophen-induced hepatotoxicity. J Appl Toxicol 2013;33:1330-6. [PubMed]
- Benz F, Roderburg C, Vargas Cardenas D, et al. U6 is unsuitable for normalization of serum miRNA levels in patients with sepsis or liver fibrosis. Exp Mol Med 2013;45:e42. [Crossref] [PubMed]
- Xiang M, Zeng Y, Yang R, et al. U6 is not a suitable endogenous control for the quantification of circulating microRNAs. Biochem Biophys Res Commun 2014;454:210-4. [Crossref] [PubMed]
- Liang Y, Ridzon D, Wong L, et al. Characterization of microRNA expression profiles in normal human tissues. BMC Genomics 2007;8:166. [Crossref] [PubMed]
- Kozomara A, Griffiths-Jones S. miRBase: annotating high confidence microRNAs using deep sequencing data. Nucleic Acids Res 2014;42:D68-73. [Crossref] [PubMed]
- Bonauer A, Dimmeler S. The microRNA-17-92 cluster: still a miRacle? Cell Cycle 2009;8:3866-73. [Crossref] [PubMed]
- Pfaffl MW, Tichopad A, Prgomet C, et al. Determination of stable housekeeping genes, differentially regulated target genes and sample integrity- BestKeeper – Excel-based tool using pair-wise correlations. Biotechnol Lett 2004;26:509-15. [Crossref] [PubMed]
- Andersen CL, Jensen JL, Orntoft TF. Normalization of real-time quantitative reverse transcription-PCR data: a model-based variance estimation approach to identify genes suited for normalization, applied to bladder and colon cancer data sets. Cancer Res 2004;64:5245-50. [Crossref] [PubMed]
- Silver N, Best S, Jiang J, et al. Selection of housekeeping genes for gene expression studies in human reticulocytes using real-time PCR. BMC Mol Biol 2006;7:33. [Crossref] [PubMed]
- Kubista M, Andrade JM, Bengtsson M, et al. The real-time polymerase chain reaction. Mol Aspects Med 2006;27:95-125. [Crossref] [PubMed]
- Bustin SA. Quantification of mRNA using real-time reverse transcription PCR (RT-PCR): trends and problems. J Mol Endocrinol 2002;29:23-39. [Crossref] [PubMed]
- Citri A, Pang ZP, Sudhof TC, et al. Comprehensive qPCR profiling of gene expression in single neuronal cells. Nat Protoc 2011;7:118-27. [Crossref] [PubMed]
- Bustin SA, Benes V, Garson JA, et al. The MIQE guidelines: minimum information for publication of quantitative real-time PCR experiments. Clin Chem 2009;55:611-22. [Crossref] [PubMed]
- Schwarzenbach H, da Silva AM, Calin G, et al. Data Normalization Strategies for MicroRNA Quantification. Clin Chem 2015;61:1333-42. [Crossref] [PubMed]
- Li T, Diao H, Zhao L, et al. Identification of suitable reference genes for real-time quantitative PCR analysis of hydrogen peroxide-treated human umbilical vein endothelial cells. BMC Mol Biol 2017;18:10. [Crossref] [PubMed]
- Li B, Matter EK, Hoppert HT, et al. Identification of optimal reference genes for RT-qPCR in the rat hypothalamus and intestine for the study of obesity. Int J Obes (Lond) 2014;38:192-7. [Crossref] [PubMed]
- Lardizabal MN, Nocito AL, Daniele SM, et al. Reference genes for real-time PCR quantification of microRNAs and messenger RNAs in rat models of hepatotoxicity. PLoS One 2012;7:e36323. [Crossref] [PubMed]
- Davoren PA, McNeill RE, Lowery AJ, et al. Identification of suitable endogenous control genes for microRNA gene expression analysis in human breast cancer. BMC Mol Biol 2008;9:76. [Crossref] [PubMed]
- El Azzouzi H, Doevendans PA, Sluijter JP. Long non-coding RNAs in heart failure: an obvious lnc. Ann Transl Med 2016;4:182. [Crossref] [PubMed]
- Fauconnier J, Meli AC, Thireau J, et al. Ryanodine receptor leak mediated by caspase-8 activation leads to left ventricular injury after myocardial ischemia-reperfusion. Proc Natl Acad Sci U S A 2011;108:13258-63. [Crossref] [PubMed]
- Masè M, Grasso M, Avogaro L, et al. Selection of reference genes is critical for miRNA expression analysis in human cardiac tissue. A focus on atrial fibrillation. Sci Rep 2017;7:41127. [Crossref] [PubMed]
- Chen X, Liang H, Guan D, et al. A combination of Let-7d, Let-7g and Let-7i serves as a stable reference for normalization of serum microRNAs. PLoS One 2013;8:e79652. [Crossref] [PubMed]
- Lange T, Stracke S, Rettig R, et al. Identification of miR-16 as an endogenous reference gene for the normalization of urinary exosomal miRNA expression data from CKD patients. PLoS One 2017;12:e0183435. [Crossref] [PubMed]
- Chang KH, Mestdagh P, Vandesompele J, et al. MicroRNA expression profiling to identify and validate reference genes for relative quantification in colorectal cancer. BMC Cancer 2010;10:173. [PubMed]
- Kok MG, Halliani A, Moerland PD, et al. Normalization panels for the reliable quantification of circulating microRNAs by RT-qPCR. FASEB J 2015;29:3853-62. [Crossref] [PubMed]
- Lawrie CH, Gal S, Dunlop HM, et al. Detection of elevated levels of tumour-associated microRNAs in serum of patients with diffuse large B-cell lymphoma. Br J Haematol 2008;141:672-5. [Crossref] [PubMed]