Qualitative coronary artery calcium assessment on CT lung screening exam helps predict first cardiac events
Introduction
Since publication of the National Lung Screening Trial in 2011 over 300,000 Americans have entered CT lung screening (CTLS) programs (1-3). This number is poised to grow dramatically in the coming years as the nearly 10 million Americans that meet the United States Preventive Services Task Force high-risk criteria for CTLS become informed about this new screening recommendation (4). CTLS may confer additional benefit in this population that is also at elevated risk for coronary artery disease (CAD) through identifying and grading coronary artery calcifications (CAC) in the acquired images (5-8). CAC has been shown to be an independent predictor of coronary events beyond the traditional cardiac risk factors provided by the Framingham model (6,7,9-12). Assessing CAC burden from the CTLS exam requires no additional cost or radiation exposure and may provide actionable data for clinicians to better manage these high risk patients. Additionally, knowledge of the presence/degree of CAC from CTLS exams may help improve health care outcomes by motivating patients to incorporate beneficial behavior modifications (13-16).
Methods
All patients who underwent baseline CTLS at our institution between January 1, 2012 and August 26, 2014 and for whom follow-up was available through December 31, 2015 were included in the study. Patients with a prior history of documented CAD were excluded from the study. The study was approved by our institution’s IRB with a waiver of informed consent and a waiver of HIPAA authorization (Study DR13-1609). All individuals enrolled in the screening program fulfilled the National Comprehensive Cancer Network Clinical Practice Guidelines in Oncology: Lung Cancer Screening v1.2012 (NCCN Guidelines®) high risk criteria for lung cancer (Table 1) and had a physician order for CTLS. Patients were not eligible for screening if they had known metastatic disease, had been diagnosed with lung cancer within the previous 5 years, or had symptoms concerning for lung cancer including fever, chest pain, a new or changing cough, new onset shortness of breath, hemoptysis or unexplained weight loss.
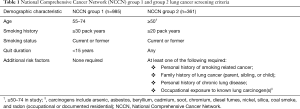
Full table
Qualitative CAC scoring data recorded at time of CTLS exam interpretation was retrospectively reviewed from the CT scan radiology reports for all individuals undergoing CTLS at our institution from January 1, 2012 through August 26, 2014. The reading radiologist qualitatively estimated the CAC burden using a four point scale taking into consideration both the extent and density of CAC present. The absence of CAC was reported as “none”. Estimated Agatston scores less than 100, between 100–400, and greater than 400 were reported as “mild”, “moderate”, and “marked” CAC, respectively. A comparison of three CAC scoring methods in the NLST showed good agreement between similar visual CAC assessments and Agatston scoring (7).
Each CTLS exam was read by one of eight radiologists internally credentialed to interpret CTLS exams per departmental policy (17). Approximately 90% of the exams were read by three of the radiologists. Each of the remaining five radiologists read fewer than 100 exams (min 1; max 80); the results for these five were grouped together for this analysis. The frequency and standard deviation of each CAC category was calculated for each radiologist to survey for consistency among readers.
Participants’ medical records were reviewed through December 31, 2015 for past medical history and cardiac events. Diabetes was defined as glycated hemoglobin (A1c) ≥6.5 mg/dL or prescription of antidiabetic medications. Hyperlipidemia was defined as low density lipid (LDL) ≥130 mmol/L in non-diabetic patients, an LDL ≥100 mmol/L in diabetics, or prescription of statin therapy. Hypertension was defined as a blood pressure ≥140 systolic or ≥90 diastolic on two consecutive occasions or prescription of antihypertensive medications. Diagnosis of chronic obstructive pulmonary disease (COPD) and family history of premature cardiac disease were recorded according to physician documentation closest to the participant’s baseline CTLS exam.
A cardiac event was defined according to the 3rd Universal Definition of Myocardial Infarction Expert Consensus (18). These events included infarctions; ST segment elevation myocardial infarction (STEMI) or non-ST segment elevation myocardial infarction (NSTEMI) and revascularizations by percutaneous intervention (PCI) or coronary artery bypass grafting (CABG) that occurred after the participant’s initial screening CT. If the participant had a STEMI that resulted in an intervention such as a PCI or CABG, the event was recorded only as a PCI or CABG, not as multiple end points. Stroke was not included as an event. Cardiac events were only recorded if they were properly documented in an internist, cardiologist, or cardiothoracic surgery note and occurred after the baseline CT scan but prior to December 31, 2015. Patients were excluded from the statistical analysis if they had a documented myocardial infarction prior to the baseline CTLS exam, did not have any follow up documented after their CT scan at the time of this review, or had missing independent variables. The study did not evaluate if there was any follow-up clinical care based on reporting of CAC from the CTLS exam.
Statistical analysis
Multivariable binary logistic regression models were developed for the sample. A reported initial cardiac event was the dependent variable. CAC was the primary independent variable evaluated during model development. The influences of potential covariates were assessed with univariate and multivariable models. These included age (continuous), pack-year smoking history (continuous), years of smoking cessation (with zero indicating smoking at program entry; continuous), sex (categorical), NCCN group (categorical), smoking at program entry (categorical), diabetes (categorical), hyperlipidemia (categorical), COPD (categorical), family history (categorical), and hypertension (categorical). Smoking status was coded for the status at the baseline scan and did not account for smokers who quit or former smokers who relapsed. CAC was treated as a continuous variable (see appendix for additional details on the rationale for this approach). Our intent was to use the models to understand the strength of the predictive value of qualitative CAC seen on baseline CTLS in initial cardiac events and not for use as predictive tools.
Logistic regression models for the binary outcome of cardiac event were run for each univariate predictor. Multivariable logistic regression models were run for combinations informed by the univariate analysis and by model Akaike information criteria (AIC). For a given sample, AIC provides a relative comparison of the quality of statistical models with lower AIC being indicative of better model performance. The models evaluated included models with predictor variable combinations that did not include CAC. The regression models were limited to four predictor variables to avoid overfit, based on Peduzzi’s rule of thumb of one predictor variable per 10 events (19). The model development effort informed the choice of two models, one including CAC as a predictor variable and one without CAC, for assessment of the discrimination capability of CAC for patients that had an initial cardiac event from those that did not. The chosen models were used to estimate the risk values for the population. Area under the receiver operator curve (AUC) was then used to assess model discrimination. AUC discrimination was considered poor at 0.5 to 0.7, good at 0.7 to 0.8, and excellent at >0.8 (20).
A multivariable regression model was also run including all of the predictor variables and another without CAC. Multiple-comparison analysis of variance (ANOVA) F-test was conducted to compare these models to assess the significance of CAC in this sample when corrected for the other demographic and clinical variables.
A Kaplan-Meier curve was constructed with the time from the baseline CTLS exam to an initial cardiac event or to censoring at the end of follow-up for each of the four CAC categories.
Demographic differences compared to qualitative CAC scores (none, mild, moderate, and marked) were assessed using pairwise Pearson’s Chi-Square test for categorical variables and the Welch two sample t-test (two-sided) for continuous variables. Statistical significance level for differences was set at P≤0.05 for all analyses. The data are reported as mean ± standard deviation, range, or percentage as appropriate. All statistical analysis was performed using the statistical software platforms SPSS version 23 and R version 3.1.2 (21,22).
Results
Of the 1,513 patients who underwent a baseline CTLS exam, 177 were excluded from the statistical analysis because they did not have any follow up documented after their exam at the time of this review, had a prior documented cardiac event, or had missing independent variables (Figure 1). Of the remaining 1,336 patients with full data, 319 (23.9%) had no reported CAC, 386 (28.9%) had mild CAC, 339 (25.4%) had moderate CAC and 292 (21.9%) had marked CAC. Forty-three out of 1,336 (3.2%) patients had an initial cardiac event recorded after the baseline CTLS exam (Figure 1).
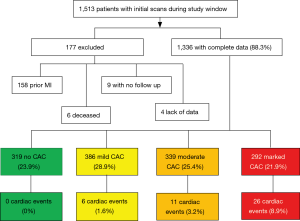
CAC scoring distribution was similar among the reading radiologists. Standard deviation among the radiologists’ average percentage of readings in each CAC category was 0.54–2.06% for none, mild, and moderate and 4.1% for marked (Table 2).
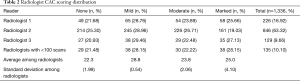
Full table
The sample mean age was 63.0±6.2, 52.5% were male, 67.8% had a history of hypertension, 19.0% had diabetes, 79.8% hyperlipidemia, 37.0% had COPD, 27.3% had a family history of cardiac disease, 45.5% were using aspirin, 62.3% were using statins and 59.9% were using blood pressure medication. Mean pack year smoking history was 47.9±22.8, 48.7% were smoking at program entry, and former smokers were quit for an average of 10.1±9.6 years (Table 3). Ninety-three percent of patients with recorded cardiac events were taking statins (Table 4).
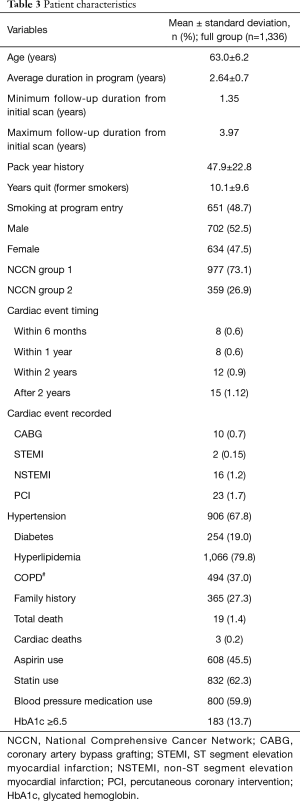
Full table
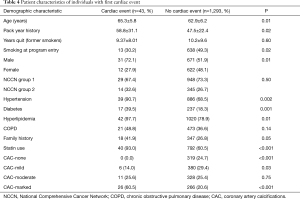
Full table
Coronary artery calcification and cardiac events
Of the 43 recorded cardiac events there were 10 CABG, 2 STEMI, 16 NSTEMI, and 23 PCI. Eight of the 18 patients with myocardial infarction underwent a revascularization procedure.
The proportional distribution of events among CAC levels was 0% (0/319) for no CAC, 1.55% (6/386) for mild CAC, 3.24% (11/339) for moderate CAC, and 8.90% (26/292) for marked CAC (Table 3). Of the patients with an initial cardiac event, 14.0% (6/43) had mild CAC, 25.6% (11/43) had moderate CAC and 60.5% (26/43) had marked CAC (Table 4). Eighteen point six percent (8/43) of patients experienced the event within 6 months of their initial LDCT scan, 18.6% (8/43) within 1 year, 27.9% (12/43) within 2 years, and 34.9% (15/43) more than 2 years after their initial scan (Table 3).
Cardiac event predictors
Table 4 summarizes demographics of the groups of patients with and without cardiac events and Table 5 the univariate analysis. In univariate analysis, sex was predictive of a cardiac event with men more likely to have a cardiac event [odds ratio (OR) =2.39; 95% CI, 1.25–4.88]. Also predictive were hypertension (OR =4.79; 95% CI, 1.91–16.0), diabetes (OR =2.91; 95% CI, 1.53–5.41), hyperlipidemia (OR =11.0; 95% CI, 2.39–196), and family history (OR =1.96; 95% CI, 1.04–3.62). Age and pack year smoking history were both predictive with 1.07 increased odds for each additional year of age (95% CI, 1.01–1.12).
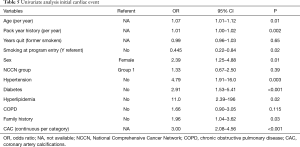
Full table
Those smoking at program entry were less likely to have an event (OR =0.45; 95% CI, 0.22–0.84). Demographic characteristics among former and current smokers were not statistically different except former smokers were more likely to have marked CAC (P=0.046) and former smokers were older (64.3 vs. 61.6; P<0.0001) (Table S1).
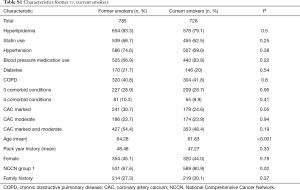
Full table
CAC in univariate analysis had 3.00 increased odds per increase in CAC category (95% CI, 2.08–4.56, P<0.001). Years quit smoking (for former smokers), COPD and NCCN group were not predictive of a cardiac event.
Model results
Using multivariable logistic modeling to control for all of our demographic and clinical variables, the increased odds of an initial cardiac event in our sample was 2.44 (95% CI, 1.64–3.84, P<0.001) for mild CAC, 5.95 (95% CI, 2.88–14.8, P<0.001) for moderate CAC, and 14.1 (95% CI, 4.40–56.7, P<0.001) for marked CAC, as compared to individuals with no CAC seen on baseline CTLS. We assessed the impact of removing CAC from this model. Comparison analysis of variance (ANOVA) F-test of the two models resulted in the removal of CAC being statistically significant (P=0.016). As this model was over-fit to our population characteristics, we also ran logistic regression models limited to four predictor variables to better assess the predictive capability of CAC for other populations.
Thirteen models, limited to four predictor variables to avoid model over-fit, were evaluated for this study (Table 6). Nine of the models included CAC as a predictor variable (CAC models) and four models did not (no-CAC models).
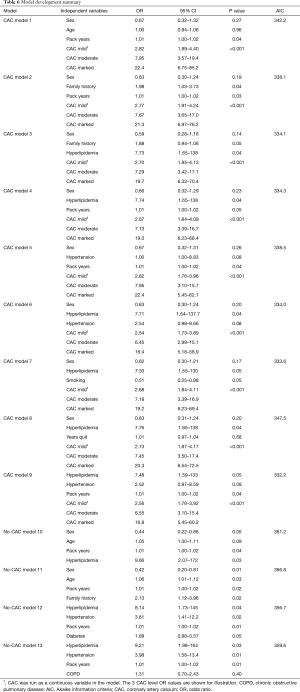
Full table
In all nine of the CAC models, the odds of an initial cardiac event were statistically significantly correlated with CAC. Increased levels of CAC observed on the initial CTLS scan increased the odds of an event as compared to no CAC in a strong “dose response” relationship. The AIC was higher, indicative of poorer model performance, in all of the no-CAC models as compared to any of the CAC models. The lower AIC models for both the CAC and no-CAC models were those with comorbid disease predictor variables, hyperlipidemia, hypertension, diabetes, and pack years smoking history as compared to models with demographic variables, sex, age, and family history.
We selected the best (lowest AIC) models, one from the CAC models (model 9) and one from the no-CAC models (model 12), to assess the model predictive capability for selecting individuals that had an initial cardiac event from those that didn’t (Tables 7,8).
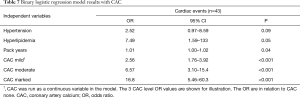
Full table
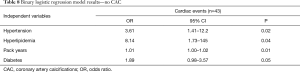
Full table
The best CAC model selected included CAC, hyperlipidemia, hypertension and pack years smoking history as the four predictor variables. Compared to individuals with no CAC the increased odds of an initial cardiac event was 2.56 (95% CI, 1.76–3.92, P<0.001) for mild CAC, 6.57 (95% CI, 3.10–15.4, P<0.0001) for moderate CAC, and 16.8 (95% CI, 5.46–60.3, P<0.001) for marked CAC. Patients with hyperlipidemia had 7.49 increased odds of an initial cardiac event (95% CI, 1.59–133, P=0.048). Patients with hypertension had 2.52 increased odds of an initial cardiac event (95% CI, 0.97–8.59, P=0.09). For each additional pack year of smoking history there was 1.01 increased risk of an initial cardiac event (95% CI, 1.00–1.02, P=0.043). The predictive performance of the model revealed good to excellent discrimination with an AUC of 0.82 (95% CI, 0.76–0.87) (Figure 2A).
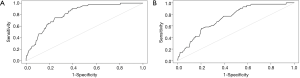
The best no-CAC model selected included hyperlipidemia, hypertension, diabetes and pack year smoking history as predictor variables. Patients with hyperlipidemia had 8.14 increased odds of an initial cardiac event (95% CI, 1.73–145, P=0.040) as compared to patients without hyperlipidemia. Patients with hypertension had 3.61 increased odds of an initial cardiac event (95% CI, 1.41–12.2, P=0.02) and patients with diabetes had 1.89 increased odds (95% CI, 0.98–3.57, P=0.05). For each additional pack year of smoking history there was 1.01 increased risk of an initial cardiac event (95% CI, 1.00–1.02, P=0.01). The predictive performance of the model revealed poor to good discrimination with an AUC of 0.74 (95% CI, 0.68–0.81) (Figure 2B). The no-CAC model had lower AUC or poorer predictive discrimination capability than the CAC model.
We constructed a Kaplan-Meier time to event plot to assess the strength of CAC correlation to the time to an initial cardiac event (Figure 3). There were very distinct differences among the four CAC categories, none, mild, moderate and marked (log rank test highly significant; P<0.001) showing clear separation and trending of increasing probability of having an event with increasing CAC levels.
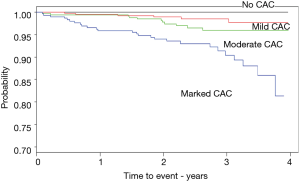
Discussion
We found a strong correlation between qualitative CAC levels seen on CTLS baseline scans and an initial cardiac event. The correlation persisted after adjusting for demographic and clinical variables and was more predictive than the use of clinical and demographic variables by themselves. The modeling indicated a strong “dose-response” relationship between qualitative CAC levels and the odds of an initial cardiac event, with higher CAC ratings associated with increased probability of event. Even with a limited number of events, the time to an initial cardiac event was well differentiated by CAC category, clearly visible in the Kaplan-Meier plot (Figure 3). While we recognize the limitation of time to event analysis in the presence of a limited proportion of the sample experiencing the event, as well as the imbalanced distribution of comorbidities across the CAC categories under comparison, the results are very supportive of clear separation and trending with increasing CAC level.
Our results confirm that the correlation observed in CTLS research studies between CAC and cardiac events is also observed in the clinical setting (23-26). In fact, the odds ratios of an initial cardiac event by CAC category and our time to event survival curves are similar to the Cox hazard ratios and survival curves stratified by Agatston score in the NELSON (Dutch-Belgian randomized lung cancer screening trial) ancillary study (26).
The American Cancer Society National Lung Cancer Roundtable recently discussed setting a goal to screen 80% of the nearly 10 million Americans qualified for CTLS by 2028 (27). Qualitative assessment of the CAC burden from the CTLS screening exam provides an important opportunity to risk stratify this large patient population at elevated risk for CAD with no additional radiation exposure or cost (1-3).
There are limitations to this study. First, currently there is no standard method of qualitatively reporting CAC in CTLS nor is there a requirement to record this data in the CTLS report or in the CTLS registry. Changing clinical practice may require screening sites to change CTLS image reporting. Second, our average follow-up duration from the date of initial scan is 2.64 years with the shortest and longest follow-up durations being 1.35 and 3.97 years, respectively. The heterogeneity in follow-up time may have limited our ability to detect cardiac events that may have occurred in those followed for a shorter amount of time. And finally, this is a single institution observational study not a multi-site randomized controlled clinical trial.
Conclusions
Qualitative coronary artery calcification scoring of CTLS exams may provide a novel method to help select individuals at risk for an initial cardiac event.
Supplemental Methods
Treatment of CAC as a continuous variable
Although CAC is an ordinal measure, attempts to include it in the logistic regression models as a categorical predictor resulted in numerical instabilities in the fitting procedures due to the lack of events in the “none” category. However, we did not want to combine the “none” and “mild” categories for the final model because the absence of CAC may have particular clinical significance. Sample logits for the outcome at each CAC level form a monotone sequence, suggesting the appropriateness of handling the ordered CAC levels as a continuous scored variable. In addition, to check for linearity of the CAC model coefficients we ran a univariate logistic regression model with CAC as a categorical variable with none and mild combined. We also ran a subgroup univariate logistic regression model with the only mild, moderate, and marked CAC patients (none CAC patients were deleted). Since the log odds of CAC in both of these models were approximately linear, we felt comfortable in developing a model with CAC as a continuous variable. Added advantages of this approach were appropriate handling of the ordered nature of the categories and reduction of the number of degrees of freedom for model overfit considerations.
Acknowledgements
None.
Footnote
Conflicts of Interest: SM Regis Received consultancy fees from Covidien (Medtronic), Christoph Wald, Member, Phillips Radiology Medical Advice Network, AB McKee received consultancy and speaking fees from Covidien (Medtronic), BJ McKee, spouse received consultancy and speaking fees from Covidien (Medtronic). Other authors have no conflicts of interest to declare.
References
- Huo J, Shen C, Volk RJ, et al. Use of CT and Chest Radiography for Lung Cancer Screening Before and After Publication of Screening Guidelines: Intended and Unintended Uptake. JAMA Intern Med 2017;177:439-41. [Crossref] [PubMed]
- Jemal A, Fedewa SA. Lung Cancer Screening With Low-Dose Computed Tomography in the United States-2010 to 2015. JAMA Oncol 2017;3:1278-81. [Crossref] [PubMed]
- Lung cancer screening registry. Available online: https://www.acr.org/Practice-Management-Quality-Informatics/Registries/Lung-Cancer-Screening-Registry
- Moyer VA. Screening for lung cancer: U.S. Preventive Services Task Force recommendation statement. Ann intern med 2014;160:330-8. [PubMed]
- 50 Years of Progress A Report of the Surgeon General. Available online: http://www.surgeongeneral.gov/library/reports/50-years-of-progress/50-years-of-progress-by-section.html
- Htwe Y, Cham MD, Henschke CI, et al. Coronary artery calcification on low-dose computed tomography: comparison of Agatston and ordinal scores. Clinical Imaging 2015;39:799-802. [Crossref] [PubMed]
- Chiles C, Duan F, Gladish GW, et al. Association of coronary artery calcification and mortality in the National Lung Screening Trial: A comparison of three scoring methods. Radiology 2015;276:82-90. [Crossref] [PubMed]
- Lu MT, Onuma OK, Massaro JM, et al. Lung cancer screening eligibility in the community. Cardiovascular risk factors, coronary artery calcification, and cardiovascular events. Circulation 2016;134:897-9. [Crossref] [PubMed]
- LaMonte MJ, FitzGerald SJ, Church TS, et al. Coronary artery calcium score and coronary heart disease events in a large cohort of asymptomatic men and women. Am J Epidemiol 2005;162:421-9. [Crossref] [PubMed]
- Ahmed AA, Patel K, Nuaku MA, et al. Risk of heart failure and death after prolonged smoking cessation. Circ Heart Fail 2015;8:694-701. [Crossref] [PubMed]
- Arad Y, Goodman KJ, Roth M, et al. Coronary calcification, coronary disease risk factors, C-Reactive protein, and atherosclerotic cardiovascular disease events. The St. Francis Heart Study. J Am Coll Cardiol 2005;46:158-65. [Crossref] [PubMed]
- McClelland RL, Jorgensen NW, Budoff M, et al. 10-year coronary heart disease risk prediction using coronary artery calcium and traditional risk factors. J Am Coll Cardiol 2015;66:1643-53. [Crossref] [PubMed]
- Mamudu HM, Paul TK, Veeranki SP, et al. The effects of coronary artery calcium screening on behavioral modification, risk perception, and medication adherence among symptomatic adults: A systematic review. Atherosclerosis 2014;236:338-50. [Crossref] [PubMed]
- Schwartz J, Allison M. Health behavior modification after electron beam computed tomography and physician consultation. J Behav Med 2011;34:148-55. [Crossref] [PubMed]
- Orakzai RH, Nasir K, Orakzai SH, et al. Effect of patient visualization of coronary calcium by electron beam computed tomography on changes in beneficial lifestyle behaviors. Am J Cardiol 2008;101:999-1002. [Crossref] [PubMed]
- Johnson JE, Gulanick M, Penckofer S, et al. Does knowledge of coronary artery calcium affect cardiovascular risk perception, likelihood of taking action, and health promoting behavior change? J Cardiovasc Nurs 2015;30:15-25. [Crossref] [PubMed]
- McKee BJ, McKee AB, Borondy Kitts A, et al. Low-dose computed tomography screening for lung cancer in a clinical setting: Essential elements of a screening program. J Thorac Imaging 2015;30:115-29. [Crossref] [PubMed]
- Thygesen K, Alpert JS, Jaffe AS, et al. Third Universal Definition of Myocardial Infarction. Circulation 2012;126:2020-35. [Crossref] [PubMed]
- Peduzzi P, Concato J, Kemper E, et al. A simulation study of the number of events per variable in logistic regression analysis. J Clin Epidemiol 1996;49:1373-9. [Crossref] [PubMed]
- Lloyd-Jones DM. Cardiovascular risk prediction. Basic concepts, current status, and future directions. Circulation 2010;121:1768-77. [Crossref] [PubMed]
- IBM Corp. Released 2015. IBM SPSS Statistics for Windows, Version 23.0. NY: Armonk, IBM Corp.
- R Core Team (2013). R: A language and environment for statistical computing. R Foundation for Statistical Computing, Vienna, Austria. Available online: http://www.R-project.org/
- Willemink MJ, Takx RA, Išgum I, et al. Prognostic value of heart calcifications for cardiovascular events in a lung cancer screening population. Int J Cardiovasc Imaging 2015;31:1243-9. [Crossref] [PubMed]
- Mets OM, Vliegenthart R, Gondrie MJ, et al. Lung cancer screening CT-based prediction of cardiovascular events. JACC Cardiovasc Imaging. 2013;6:899-907. [Crossref] [PubMed]
- Rasmussen T, Køber L, Abdulla J, et al. Coronary artery calcification detected in lung cancer screening predicts cardiovascular death. Scand Cardiovasc J 2015;49:159-67. [Crossref] [PubMed]
- Takx RA, Išgum I, Willemink MJ, et al. Quantification of coronary artery calcium in nongated CT to predict cardiovascular events in male lung cancer screening participants: results of the NELSON study. J Cardiovasc Comput Tomogr 2015;9:50-7. [Crossref] [PubMed]
- Verbal communication at National Lung Cancer Screening Round Table Meeting. December 11, 2018. Available online: https://eurekalert.org/pub_releases/2017-12/acs-nlc121017.php