The developing role of FDG PET imaging for prognostication and radiotherapy target volume delineation in non-small cell lung cancer
Introduction
Lung cancer is the most common cause of death from cancer worldwide (1). For patients with non-small cell lung cancer (NSCLC) who are being considered for curative intent treatment, 18F-fluorodeoxyglucose positron emission tomography (FDG PET)/computed tomography (CT) imaging has become the standard of care in baseline staging, and has also shown benefit for radiotherapy planning (RTP) (2-4).
The implementation and applications of FDG PET and CT for NSCLC have changed over time. FDG PET was initially acquired as a standalone modality, and was demonstrated to be superior to CT alone in the staging of lung cancer (5,6). When PET was acquired in conjunction with a CT using an integrated scanner (PET/CT), the combined information has shown to have higher staging accuracy than PET imaging alone (7-12). Evaluation of combined PET/CT images has traditionally been based on visual interpretation, and was predominantly applied for tumor detection, staging and treatment selection. Subsequently, the value of FDG PET/CT in manual target volume delineation (TVD) for radiotherapy was also demonstrated (13). In the more recent years, PET tracers other than FDG have come available for evaluation of different biological tumor characteristics. Advancements in multimodal technology now create possibilities for PET/CT imaging in new applications, including (semi-)automatic definition of target volumes, quantitative response assessment, determining patient prognosis, and predicting treatment outcome in NSCLC (14-20).
Radiomics has been introduced as a sophisticated way to extract and mine a large number of quantitative image features believed to provide a comprehensive picture of tumor phenotypes, for example related to necrosis, angiogenesis and radioresistant cells (21). The assessment of biological processes with PET radiomics features may further improve tumor characterization (22). The relation between CT image texture patterns and tumor biology has provided further insights into tumor phenotype and genotype (19,23,24); such a relationship for PET still warrants extensive investigation (25-27). Meanwhile, studies already indicated that radiomics features contain prognostic information regarding response to therapy or treatment outcome in lung cancer studies (19,28-31). In addition, radiomics features may also contribute to visual or (semi-)automatic definition of gross tumor volume (GTV) (32). Ultimately, proper use of PET/CT imaging could contribute in all steps of the treatment procedure and optimal use may lead to better tumor characterization, treatment decision making, treatment guidance, tumor response assessment, and local tumor control.
Based on these factors, the use and role of PET imaging has expanded from a primarily diagnostic tool to a more central role in the context of personalized medicine. This review discusses the current state of art in applications of FDG PET/CT for prognostication and TVD in NSCLC, and describes the challenges related to the clinical implementation of these new developments.
A literature research was conducted to assess recent advancements in the use of PET/CT imaging for prognostication and radiotherapy TVD in NSCLC. A search query was undertaken at the PubMed database, using a combination of the following keywords: (“PET”) and (“non-small cell lung cancer” or “NSCLC”) and (“target volume delineation” or “segmentation” or “prognostic” or “prognostication” or “radiomics” or “textural features” or “precision medicine”). The search query yielded a total of 410 papers. Only studies written in English, related to PET/CT for prognostication and TVD in the treatment of NSCLC with radiotherapy and of relevance to this overview were included. To illustrate this, papers regarding PET guided patient examination, PET and particle therapy, surgery, PET/CT adapted RT, PET and dosimetric planning, PET and lung toxicity, PET and lung ventilation studies, drug assessment, or economic related were excluded. No limitations were set on the year of publication. However, in the case of review papers, solely the most recent ones for each topic were included. Other reasons for exclusion were inaccessibility, case reports, editorials or conference abstracts, resulting in 77 papers. In addition, references within retrieved articles were analyzed to expand the search. In the end, this led to 124 papers covering the relevant topics, which were studied and incorporated in the descriptive evaluations below.
Prognostic factors in NSCLC
In the last decades, the overall survival (OS) of lung cancer patients has not improved tremendously (33). The selection of treatment strategies for NSCLC patients is mainly based on empirical models. The most important prognostic indicator is the disease stage, which is determined by the extent of the primary tumor, nodal involvement, and the presence of distant metastasis according to the TNM classification (34). Disease staging also plays an important part in guiding therapy (6). In locally advanced NSCLC, however, treatment selection based on TNM staging and other clinical variables may not be accurate enough for survival probability prediction (35,36). As technology improves and more treatment options become available, the search for more accurate prognostic factors is warranted in the context of personalized medicine.
Conventional evaluations
The value of many well-established prognostic factors, such as the distinction between stage IIIA, IIIB, and IIIC, performance status (PS), histology, and other clinical and therapeutic variables, have been confirmed in locally advanced NSCLC (37). Other studies have shown that basic imaging-derived features in pre-treatment and post-treatment scans provide clinically relevant prognostic information for patients with NSCLC of various stages. Examples include tumor size and volume on CT, and standardized uptake value (SUV) based metrics like SUVmax, SUVpeak, and SUVmean, or metabolic active tumor volume (MTV) on FDG PET (38-44). As a combined parameter, total lesion glycolysis (TLG) was shown to be more promising than MTV, and in combination with other parameters such as shape based features complementary prognostic information could be extracted from PET images (19,45,46). These metrics are all related to tumor burden and metabolic characterization. An overview of studies about image-based prognostication using PET in NSCLC together with their findings is given in Table 1.
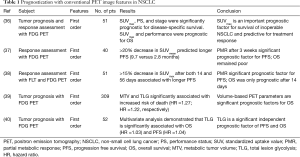
Full table
Even though basic PET metrics have been proclaimed to be of prognostic or predictive value, there are still contradictions found in the literature where certain SUV metrics do not show any prognostic value, when used with other prognostic factors (47). Paesmans et al. conducted a meta-analysis about the prognostic value of SUVmax in NSCLC and concluded that these contradictory findings could be due to influence of disease stage or tumor histology, or due to differences in assessment methods (16). These dependencies may not hold for all PET features, hence PET features still may play a role in prognostication.
Radiomics textural features for improved prognostication
PET radiomics represents the high-throughput mining of quantitative image features from PET imaging to characterize tumor phenotypes. Radiomics features include first order features, that are based on the gray level intensity and its distribution in the image, but do not consider the three dimensional (3D) distribution of gray levels. Examples are the max, mean, standard deviation, skewness, and kurtosis of SUV within a volume of interest. The prognostic value of these ‘simple’ features may be weaker in large well differentiated tumors that are known to exhibit higher hypoxia, necrosis, or anatomic and physiologic complexity, which translates to higher complexity in the spatial distribution of PET tracer uptake (16).
Radiomics features also include second and higher order features, called textural features, which may cover this higher complexity by describing the relationship between the voxel intensity and their position within an image. This relationship can be calculated with various mathematical methods, such as the gray level co-occurrence matrix (GLCM) for pairwise arrangement of voxels (48), the gray level run-length matrix (GLRLM) for alignment of voxels with the same intensity (49), the gray level size-zone matrix (GLSZM) for characteristics of zones with identical voxel values (50), and the neighborhood grey tone difference matrix (NGTDM) for determining changes in neighboring voxel intensities (51). As an example, GLCM entropy measures the variability in neighborhood intensity values and may be useful to characterize necrotic cores, a factor that has been associated with worse prognosis. Higher entropy represents a more heterogeneous FDG PET activity within the tumor, as is depicted in Figure 1. Another example includes GLSZM features that mostly relate to the size of subregions within a tumor with similar intensities, which hypothetically characterizes cell subpopulations with distinct clonogenic growth. Some of these textural features have shown prognostic value for clinical outcome and tumor response (17,29,52-54). An overview of studies about the prognostic value of textural features in NSCLC together with their findings is given in Table 2.
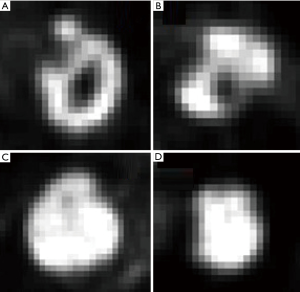
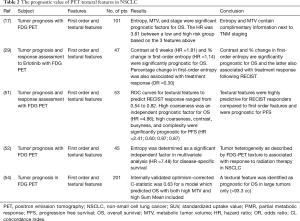
Full table
PET textural features are calculated on a group of voxels within a region-of-interest (ROI). A common ROI that is used for PET radiomics analysis is MTV. The relationship between MTV and radiomics textural features has been investigated and studies demonstrated that specific PET textural features are closely correlated to MTV (17,29,52,55). Therefore, in these cases prognostic textural features would rather act as a surrogate than as an independent variable. On the other hand, several studies demonstrated that this dependency is decreasing with increasing MTV (17,55), suggesting that for larger tumors these specific PET features may become of relevance. This is probably caused by the partial volume effect for small tumors, making it difficult to obtain reliable PET radiomics features for tumors smaller than 10 cc (56). For instance, to determine the GLCM entropy, tumors were required to be at least more than 10 cc to reduce correlations with MTV (17,55). Unfortunately, these findings were based on only four PET textural features and are not representative for all radiomics features, since this volume dependency differs amongst textural features. A high GLCM entropy (see Figure 1 for an example) in combination with a large MTV (>35 cc) led to a worse prognosis in 101 patients with stage I-III NSCLC receiving surgery, chemotherapy, chemoradiotherapy, or a combination (17). Ohri et al. performed PET radiomics analysis in 201 patients with locally advanced NSCLC and concluded that a feature called GLCM Sum Mean had prognostic value for tumors with an MTV larger than 93 cc (54). On the contrary, Pyka et al. concluded that GLCM entropy was predictive for disease-specific survival in 45 early stage NSCLC patients with mainly small tumors (mean MTV 34 cc, ranging from 1.74 to 178 cc) receiving primary stereotactic radiation therapy (53). However, only 12 patients were used to assess this specific endpoint and divided in two unbalanced groups (2 versus 10) for comparison, which is a serious limitation. These results point in the direction that for larger tumors, PET textural features contain complimentary information above a specific tumor volume. The volume threshold differs per textural feature. We suggest assessing volume dependencies in detail for each textural feature in large patient cohorts, when building prognostic models containing PET textural features.
The literature also reports on correlations between first order SUV metrics and higher order features (texture) (29,52). Additionally, PET textural features also depend on image segmentation and image reconstruction settings (57), SUV binning (58,59), and feature calculation method (17). And although it is clear how these choices influence relationships between PET textural features or their reproducibility, it is not always clear how these factors affect the prognostic value. In the search for independent prognostic PET textural features, some investigators find optimal cutoff values that result in prognostic variables, but do not validate their results (52). Fave et al. (60) argued that testing multiple cutoffs to find the best one without an independent validation dataset for testing, could yield overly optimistic results. In combination with the inclusion of multiple variables that have not been corrected for multiple hypothesis testing, the models will probably not perform well in other patient cohorts. From the studies shown in Table 1, the issue of multiple-hypothesis testing was solely addressed by Ohri et al. (54), but none of them had validated their results externally. Internal or external validation is lacking in most studies, but is required to strengthen results regarding model performance. In absence of an external validation cohort, it is advised to split the initial cohort into a training and a test set. We would like to emphasize that prognostic models should always be corrected for multiple hypothesis testing and should be validated, preferably with an external patient cohort.
Even though many studies reported on the prognostic value of PET radiomics features, results are difficult to compare amongst studies, and convincing evidence remains poor (61). Many studies about PET radiomics features make the remark that a standardized approach is lacking, which influences generalizability and makes meta-analyses difficult. For radiomics studies, guidelines can be found in the literature that promotes standardization for designated terms, extraction and calculation of textural features (62), and statistical analysis (63). We agree that standardization is necessary and that studies should comply with best-practice procedures to move forward in the field of radiomics. In light of these demands, a Radiomics Quality Score was proposed to evaluate radiomics studies in literature following standardized criteria (64). These criteria can also aid in setting up future radiomics studies and check whether they comply with the written guidelines. The Radiomics Quality Score will hopefully lead to comparable high quality radiomics studies, and would facilitate future meta-analyses.
Other PET tracers
The majority of evidence on PET/CT for prognostication concerns the use of FDG. Although very sensitive for staging most tumor types, a disadvantage of FDG is that it is not tumor specific. FDG uptake can be seen in inflammatory lesions, increasing the chance of false-positive findings.
The tracer 3’-deoxy-3’-18F-fluorothymidine for PET imaging (FLT PET), which allows assessment of tumor proliferation, does not seem to accumulate in inflammatory processes. Therefore, FLT is considered a more specific oncological tracer than FDG, but sensitivity is lower (65). Its main role is currently envisioned in evaluating early treatment response (66-68). However, the combination of dual-tracer PET/CT may improve diagnostic accuracy (69) and may also contribute in early response assessment and prediction of clinical outcomes (70). One study on NSCLC compared the prognostic value of FLT PET to FDG PET first order features and showed that both FDG and FLT PET baseline maximum SUV were associated with OS. However, clinical studies proving the prognostic value of textural features in FLT PET imaging are scarce. Therefore, the additional value of FLT PET textural features next to well-established prognostic factors for evaluation of treatment response and predicting clinical outcomes in NSCLC has to be further studied (71).
Other tracers which are currently under investigation cover different aspects of lung cancer biology that may enable better phenotypic characterization for improved prognostication. 11C-methionine or l-3-18F-α-methyl tyrosine (18F-FAMT) characterizes amino acid transport and protein metabolism. These processes are upregulated in malignant cells as a consequence of increased cellular proliferation activity. In addition, 18F-fluoromisonidazole (18F-FMISO), 18F-fluoroazomycin arabinoside (18F-FAZA), and 18F-flortanidazole (18F-HX4) are hypoxic tracers. Hypoxia is an important factor in oncology, because this increases radioresistance and chemoresistance, and is related to poor clinical outcome (72). Lastly, there are PET tracers targeting integrins expressed on tumor vasculature, which measure levels of angiogenesis growth factors, to characterize angiogenesis. The clinical usage of these PET tracers in lung cancer has been limited so far, and pre-clinical research is still dominating (67). These upcoming tracers have potential for tumor detection, characterization, prognostication, and response assessment, but efforts need to be made to ensure safety, optimal signal-to-noise, and reproducibility of measurements in order to move forward. Therefore, there is insufficient data on the use of these tracers for prognostication for NSCLC, either as a separate parameter or in combination with FDG PET.
TVD in locally advanced NSCLC
The definition of target volumes for radiotherapy of NSCLC was traditionally based on anatomical imaging with CT, in combination with findings from e.g., physical examination, endoscopy and biopsies. Multiple advances in CT imaging have further improved its spatial and contrast resolution with benefit for tumor delineation, for example using multi-slice detectors and respiratory motion correction techniques (73-76). In addition, it has been shown that CT images can be enhanced for discrimination of tumor and normal tissues, using e.g., intravenous contrast or dual-energy CT (77-79). However, despite these improvements, CT alone does not provide sufficiently clear information to reliably and consistently discriminate tumor and normal tissues in all situations (80-82).
After the introduction of integrated PET/CT, its additional value for target definition was rapidly acknowledged. The relevance of good and reproducible quality of the images and accurate anatomical registration with planning CT were acknowledged. This resulted in the standardization of PET/CT for radiotherapy TVD (2). Several studies confirmed the value of adding PET imaging to planning CT to reduce inter-observer variation in TVD in RTP in NSCLC patients, and is specifically helpful in TVD when the tumor boundaries are not easily distinguished from surrounding healthy tissue (81-84). Even with the use of PET imaging there is still variability amongst observers (85,86). The remaining question is how to derive the most optimal target volumes using the information gleaned from combinations of PET and CT.
Visual interpretation
Most early clinical studies have used a visual interpretation technique for target definition from combined FDG PET and CT scans (83,84). As in any observer-dependent procedure, it is essential to standardize this interpretation where possible. In one study, the benefit of using strict protocols was shown (85). Another study demonstrated that interdisciplinary cooperation between the radiation oncologist and nuclear medicine specialist is beneficial for consistent contouring (87).
Automatic TVD
Many groups have investigated the use of automated segmentation techniques to either guide or generate the relevant target volume (14,15,88-91). A previous International Atomic Energy Agency (IAEA) publication provided guidance on the use and role of PET/CT imaging for RTP in a range of tumor sites (92) and an update was given in 2015 with additional practical guidelines for the use of FDG PET/CT for the purposes of radiotherapy TVD in NSCLC (3). These guidelines state that target volumes generated following any automatic segmentation algorithm should always be verified, and edited where needed, by a trained observer. The skepticism towards automatic contouring in general, demonstrates that there are still hurdles to overcome in automatic contouring before it is fully accepted in the clinic.
The most common application of automatic segmentation on PET imaging (PET-AS) is definition of the MTV, however studies tried to go even further and proposed methods for GTV definition. Many different PET-AS algorithms have been proposed to define the edge of the GTV for this purpose (14,93,94). It was thought that these algorithms would provide contours that are more consistent and better represent true tumor borders, when compared to visual interpretation by radiation oncologists. Unfortunately, none of these methods have been tested on large patient datasets and in the presence of a ground truth, probably because pathological correlation has proven difficult (95,96). On top of that, the different PET-AS algorithms all failed under specific circumstances, which prevented recommending a single algorithm (15). A possible solution for this variable performance of PET-AS methods was suggested in the form of a consensus algorithm that combines different PET-AS methods (97). Another difficulty with PET-AS is the variability of SUV values due to factors other than tumor activity alone, such as patient factors and technical factors (98). More recently, the AAPM task group has said to undertake steps to establish a standardized procedure for PET-AS algorithms, which could help with the acceptance and implementation of these delineation methods in the clinic (15). Perhaps the question is whether the focus should be solely on PET imaging for TVD or should be more on the combination of multiple imaging modalities, if there are already many studies that show its shortcomings.
The most important factor is that PET-AS neglects all other available information, like anatomical information from CT and in some cases MRI, the locations of tumor-positive biopsies, and findings at endoscopic examinations. Therefore, the information obtained from PET should be considered complementary. Combining the information contained in the PET and CT scans may lead to more successful auto-contouring (95). Still, resulting contours should be checked visually and edited to other sources of information where needed.
Radiomics for target definition
The application of textural features for use in TVD has yet to garner attention, leading to a minimal number of published studies. Early attempts to automate tumor contouring using textural features were promising. For example Wang et al. (99) designed a radiomics based automatic contouring method by training a ROI-based decision-tree–based K-nearest neighbor (K-nn) classifier using 14 PET and 13 CT textural features. The K-nn classifier is a classical machine learning method, and here its purpose was to detect and classify image voxels in NSCLC. It was demonstrated that a combination of these textural features from PET and CT images could distinguish between abnormal and normal tissue in the head and neck and that their classifier method was able to generate accurate and consistent delineations compared to other automatic and to manual delineations. Further studies using a larger sample size and with pathologic validation are necessary to determine its clinical value in thoracic diseases.
Interestingly, it is said that the more advanced deep learning method (100), also able to classify objects, outperforms these classical machine learning methods. A recent comparison, however, of lymph node metastasis classifications in NSCLC patients between classical machine learning methods and a deep learning approach showed comparable results (99). Nevertheless, a big advantage of deep learning is its ability to generate data driven features instead of relying on hand crafted features and is thus potentially more powerful (100). This comes with a higher risk of over-fitting if not appropriately trained and validated, and typically needs more data. Its use in medical image analysis is increasing as algorithms become more sophisticated and more data becomes available (101,102), which might lead to new insights in tissue classification and delineation.
Discussion
The literature presented in this review indicates that FDG PET/CT has a substantial role in prognostication and RTP for NSCLC. First order metrics such as SUVmax and certain PET textural features were prognostic for survival and treatment response assessment. It is noted, however, that many publications about PET radiomics are based on relatively small datasets without robust internal and/or external validation which challenges clinical translation. Both prognostication and GTV definition should not rely on PET alone, but rather on its addition to and integration with other independently validated sources of information. The optimal implementation of such strategies is expected to continue to evolve over the coming years.
Current challenges
Challenges in prognostication with PET imaging cover a broad range of topics including image acquisition and reconstruction settings, tumor segmentation, image feature calculation, and statistical methods. Standardization of these topics for PET radiomics studies would promote reproducibility of study results, which is typically lacking (103). This lack of standardization challenges prognostication as variations in all these topics may introduce changes that are not due to underlying biology. It is already shown that this may lead to false positive results (61), and therefore standardization of these topics is warranted to move further in the field.
A challenge to overcome in image acquisition is in finding optimal image quality, hence preserving heterogeneity information. Respiratory motion during PET imaging causes lesion smearing (104,105), which reduces contrast in the image and therefore affects the quantification of heterogeneity. Use of partial volume and respiratory motion correction techniques may improve quantification accuracy (56,106). Also differences in matrix grid size can confound results, as larger voxel sizes tend to be more affected by the partial volume effect. This leads to a more uniform intensity distribution, which subsequently has an impact on most radiomics PET features (107), and study designs need to take this into account. Hence, it is known that differences in image quality or matrix grid size affect textural feature outcome, and further studies should focus on its impact on the prognostic value.
The effect of tumor segmentation on the prognostic and predictive value of imaging features is also not yet clear. PET-AS algorithms or manual delineation by radiation oncologists determine which voxels within an image will be analyzed, thus, the variability in segmentation affects reproducibility when extracting imaging parameters from PET and CT scans (108-110). The accuracy and reproducibility of TVD is important as it has an impact on imaging parameters that are used to determine patient prognosis and to predict and monitor response to therapy. Interestingly, in NSCLC it is not yet known whether the GTV used for treatment planning or the MTV results in higher prognostication. The inclusion of low metabolically active regions within a tumor could contain valuable information and also contribute to the heterogeneity in a tumor, whereas on the other side it also holds the risk of including non-pathological tissue. Further studies should assess the impact of these different volumes on prognostication in NSCLC patients.
Interchangeable SUV measurements across centers are very important in PET radiomics, but the methodology used to determine textural features also demonstrates lack of consistency. There are multiple methods to calculate textural features, which may have impact on the prognostic value of these quantitative PET imaging features. In addition, there are textural features that rely on image intensity resampling or SUV discretization. Discretization reduces the large number of intensity values [typically 16-bit in PET imaging (111)] to a smaller number, e.g., 32 or 64 bins. Each SUV discretization method results in a new set of features (58). Also, textural features can be calculated in 2D or 3D, in one direction or in multiple directions and so on. Hence, the number of textural features can easily reach to hundreds. Therefore sufficiently large patient cohorts need to be included combined with sophisticated statistical methods to prevent overfitting. With multiple possibilities to calculate features, it is often not clear how researchers achieved the final results. Hence, there is the urge to create guidelines for standardized PET radiomics analysis, and for reporting study results about prognostication with PET radiomics features in NSCLC.
As more complex PET radiomics features are designed, and hundreds of these complex imaging features are included in PET radiomics studies, it is understandable that studies choose a hypothesis generating strategy. This is a legit strategy, as long as results are validated and tested for any kind of confounding as described before. It should be avoided that studies suffer from a poor design and result in the publication of overly optimistic results. Although quantitative studies about image heterogeneity in cancer have shown associations with aspects of tumor behavior, it is not fully understood how underlying biology is affecting the PET signal, and it is foreseen that answers to this will facilitate implementation in the clinic (112). Therefore, more studies should investigate the relationship of tumor biology and proven robust independent textural features.
Future perspectives for PET imaging in prognostication and TVD
Innovation in PET technology opens up more possibilities and holds perspective for the future, although not necessarily the solution for all above described issues. Examples include opportunities for PET/MR (113-116) and 4D PET/CT image reconstruction and partial volume correction methods for increased quantitative accuracy (106,117,118). New PET tracers might become available to improve tumor characterization, and potentially lead to new and improved prognostic biomarkers (67,119-122). Newer PET/CT scanners with improved sensitivity and spatial resolution could lead to better tumor detection and target volume definition (123). In addition, new digital PET technology will be used within hybrid PET/MR systems and could facilitate target volume definition by improved motion correction (124). Since almost every patient with lung cancer is scanned with PET/CT, management of this valuable data for quantitative image analysis on a large scale is strongly desired. This allows for studies with larger sample sizes in the future, benefiting radiomics analysis in specific, but also demands proper data management.
Conclusions
PET/CT has an increasing role in prognostication and target volume definition of NSCLC. The implementation of new PET based applications will facilitate the shift from visual interpretation and manual delineation to (semi)automated target volume definition. In order to establish common ground in the clinic, studies about the prognostic value of quantitative PET imaging features will require external validation cohorts and pathological validation. The implementation of quantitative PET imaging features in a clinical setting would require substantial effort to standardize both imaging and methods for radiomics analysis. Current efforts to create larger databases will hopefully lead to strong evidence in prognostication with PET/CT imaging in NSCLC.
Acknowledgements
None.
Footnote
Conflicts of Interest: The authors have no conflicts of interest to declare.
References
- Ferlay J, Soerjomataram I, Dikshit R, et al. Cancer incidence and mortality worldwide: sources, methods and major patterns in GLOBOCAN 2012. Int J Cancer 2015;136:E359-86. [Crossref] [PubMed]
- Ung JC, Bezjak A, Coakley N, et al. Positron Emission Tomography with 18Fluorodeoxyglucose in Radiation Treatment Planning for Non-small Cell Lung Cancer. J Thorac Oncol 2011;6:86-97. [Crossref]
- Konert T, Vogel W, MacManus MP, et al. PET/CT imaging for target volume delineation in curative intent radiotherapy of non-small cell lung cancer: IAEA consensus report 2014. Radiother Oncol 2015;116:27-34. [Crossref] [PubMed]
- Vogel WV, Lam MG, Pameijer FA, et al. Functional Imaging in Radiotherapy in the Netherlands Availability and Impact on Clinical Practice. Clin Oncol (R Coll Radiol)l 2016;28:e206-15.
- Gould MK, Kuschner WG, Rydzak CE, et al. Test performance of positron emission tomography and computed tomography for mediastinal staging in patients with non-small-cell lung cancer: A meta-analysis. Ann Intern Med 2003;139:879-92. [Crossref] [PubMed]
- MacManus MP, Hicks RJ, Matthews JP, et al. High rate of detection of unsuspected distant metastases by pet in apparent stage III non-small-cell lung cancer: Implications for radical radiation therapy. Int J Radiat Oncol Biol Phys 2001;50:287-93. [Crossref] [PubMed]
- Cerfolio RJ, Ojha B, Bryant AS, et al. The accuracy of integrated PET-CT compared with dedicated PET alone for the staging of patients with nonsmall cell lung cancer. Ann Thorac Surg 2004;78:1017-23. [Crossref] [PubMed]
- De Wever W, Ceyssens S, Mortelmans L, et al. Additional value of PET-CT in the staging of lung cancer: comparison with CT alone, PET alone and visual correlation of PET and CT. Eur Radiol 2007;17:23-32. [Crossref] [PubMed]
- Antoch G, Stattaus J, Nemat AT, et al. Non-small cell lung cancer: Dual-modality PET-CT in preoperative staging. Radiology 2003;229:526-33. [Crossref] [PubMed]
- Lardinois D, Weder W, Hany TF, et al. Staging of non-small-cell lung cancer with integrated positron-emission tomography and computed tomography. N Engl J Med 2003;348:2500-7. [Crossref] [PubMed]
- Gregory DL, Hicks RJ, Hogg A, et al. Effect of PET/CT on management of patients with non-small cell lung cancer: results of a prospective study with 5-year survival data. J Nucl Med 2012;53:1007-15. [Crossref] [PubMed]
- Shim SS, Lee KS, Kim BT, et al. Non-small cell lung cancer: prospective comparison of integrated FDG PET/CT and CT alone for preoperative staging. Radiology 2005;236:1011-9. [Crossref] [PubMed]
- Steenbakkers RJ, Duppen JC, Fitton I, et al. Reduction of observer variation using matched CT-PET for lung cancer delineation: a three-dimensional analysis. Int J Radiat Oncol Biol Phys 2006;64:435-48. [Crossref] [PubMed]
- Zaidi H, El Naqa I. PET-guided delineation of radiation therapy treatment volumes: a survey of image segmentation techniques. Eur J Nucl Med Mol Imaging 2010;37:2165-87. [Crossref] [PubMed]
- Hatt M, Lee JA, Schmidtlein CR, et al. Classification and evaluation strategies of auto-segmentation approaches for PET: Report of AAPM task group No. 211. Med Phys 2017;44:e1-e42. [Crossref] [PubMed]
- Paesmans M, Berghmans T, Dusart M, et al. Primary tumor standardized uptake value measured on fluorodeoxyglucose positron emission tomography is of prognostic value for survival in non-small cell lung cancer: update of a systematic review and meta-analysis by the European Lung Cancer Working Party for the International Association for the Study of Lung Cancer Staging Project. J Thorac Oncol 2010;5:612-9. [Crossref] [PubMed]
- Hatt M, Majdoub M, Vallieres M, et al. 18F-FDG PET uptake characterization through texture analysis: investigating the complementary nature of heterogeneity and functional tumor volume in a multi-cancer site patient cohort. J Nucl Med 2015;56:38-44. [Crossref] [PubMed]
- Shang J, Ling X, Zhang L, et al. Comparison of RECIST, EORTC criteria and PERCIST for evaluation of early response to chemotherapy in patients with non-small-cell lung cancer. Eur J Nucl Med Mol Imaging 2016;43:1945-53. [Crossref] [PubMed]
- Aerts HJ, Velazquez ER, Leijenaar RT, et al. Decoding tumour phenotype by noninvasive imaging using a quantitative radiomics approach. Nat Commun 2014;5:4006. [Crossref] [PubMed]
- Carvalho S, Leijenaar RT, Troost EGC, et al. Early variation of FDG-PET radiomics features in NSCLC is related to overall survival — the “delta radiomics” concept. Radiother Oncol 2016;118:S20-1. [Crossref]
- Lambin P, Rios-Velazquez E, Leijenaar R, et al. Radiomics: extracting more information from medical images using advanced feature analysis. Eur J Cancer 2012;48:441-6. [Crossref] [PubMed]
- Gatenby RA, Grove O, Gillies RJ. Quantitative imaging in cancer evolution and ecology. Radiology 2013;269:8-15. [Crossref] [PubMed]
- Weiss GJ, Ganeshan B, Miles KA, et al. Noninvasive image texture analysis differentiates K-ras mutation from pan-wildtype NSCLC and is prognostic. PLoS One 2014;9. [Crossref] [PubMed]
- Yamamoto S, Korn RL, Oklu R, et al. ALK molecular phenotype in non-small cell lung cancer: CT radiogenomic characterization. Radiology 2014;272:568-76. [Crossref] [PubMed]
- Yip SS, Kim J, Coroller TP, Parmar C, et al. Associations Between Somatic Mutations and Metabolic Imaging Phenotypes in Non-Small Cell Lung Cancer. J Nucl Med 2017;58:569-76. [Crossref] [PubMed]
- Del Gobbo A, Pellegrinelli A, Gaudioso G, et al. Analysis of NSCLC tumour heterogeneity, proliferative and 18F-FDG PET indices reveals Ki67 prognostic role in adenocarcinomas. Histopathology 2016;68:746-51. [Crossref] [PubMed]
- van Baardwijk A, Bosmans G, van Suylen RJ, et al. Correlation of intra-tumour heterogeneity on 18F-FDG PET with pathologic features in non-small cell lung cancer: a feasibility study. Radiother Oncol 2008;87:55-8. [Crossref] [PubMed]
- Gevaert O, Xu J, Hoang CD, et al. Non-small cell lung cancer: identifying prognostic imaging biomarkers by leveraging public gene expression microarray data—methods and preliminary results. Radiology 2012;264:387-96. [Crossref] [PubMed]
- Cook GJR, O’Brien ME, Siddique M, et al. Non-small cell lung cancer treated with erlotinib: heterogeneity of 18F-FDG uptake at PET—association with treatment response and prognosis. Radiology 2015;276:883-93. [Crossref] [PubMed]
- Depeursinge A, Yanagawa M, Leung AN, et al. Predicting adenocarcinoma recurrence using computational texture models of nodule components in lung CT. Med Phys 2015;42:2054-63. [Crossref] [PubMed]
- Fried DV, Tucker SL, Zhou S, et al. Prognostic value and reproducibility of pretreatment CT texture features in stage III non-small cell lung cancer. Int J Radiat Oncol Biol Phys 2014;90:834-42. [Crossref] [PubMed]
- Yu H, Caldwell C, Mah K, et al. Coregistered FDG PET/CT-Based Textural Characterization of Head and Neck Cancer for Radiation Treatment Planning. IEEE Trans Med Imaging 2009;28:374-83. [Crossref] [PubMed]
- Aupérin A, Le Péchoux C, Rolland E, et al. Meta-analysis of concomitant versus sequential radiochemotherapy in locally advanced non-small cell lung cancer. J Clin Oncol 2010;28:2181-90. [Crossref] [PubMed]
- Detterbeck FC, Boffa DJ, Kim AW, et al. The Eighth Edition Lung Cancer Stage Classification. Chest 2017;151:193-203.
- Strøm HH, Bremnes RM, Sundstrom SH, et al. Poor prognosis patients with inoperable locally advanced NSCLC and large tumors benefit from palliative chemoradiotherapy: a subset analysis from a randomized clinical phase III trial. J Thorac Oncol 2014;9:825-33. [Crossref] [PubMed]
- Mahar AL, Compton C, McShane LM, et al. Molecular Modellers Working Group of the American Joint Committee on Cancer. Refining prognosis in lung cancer: A report on the quality and relevance of clinical prognostic tools. J Thorac Oncol 2015;10:1576-89. [Crossref] [PubMed]
- Berghmans T, Paesmans M, Sculier JP. Prognostic factors in stage III non-small cell lung cancer: a review of conventional, metabolic and new biological variables. Ther Adv Med Oncol 2011;3:127-38. [Crossref] [PubMed]
- Borst GR, Belderbos JS, Boellaard R, et al. Standardised FDG uptake: a prognostic factor for inoperable non-small cell lung cancer. Eur J Cancer 2005;41:1533-41. [Crossref] [PubMed]
- Dingemans AM, de Langen AJ, van den Boogaart V, et al. First-line erlotinib and bevacizumab in patients with locally advanced and/or metastatic non-small-cell lung cancer: a phase II study including molecular imaging. Ann Oncol 2011;22:559-66. [Crossref] [PubMed]
- Mileshkin L, Hicks RJ, Hughes BG, et al. Changes in 18F-fluorodeoxyglucose and 18F-fluorodeoxythymidine positron emission tomography imaging in patients with non-small cell lung cancer treated with erlotinib. Clin Cancer Res 2011;17:3304-15. [Crossref] [PubMed]
- Hyun SH, Ahn HK, Kim H, et al. Volume-based assessment by (18)F-FDG PET/CT predicts survival in patients with stage III non-small-cell lung cancer. Eur J Nucl Med Mol Imaging 2014;41:50-8. [Crossref] [PubMed]
- Moon SH, Cho SH, Park LC, et al. Metabolic response evaluated by 18F-FDG PET/CT as a potential screening tool in identifying a subgroup of patients with advanced non-small cell lung cancer for immediate maintenance therapy after first-line chemotherapy. Eur J Nucl Med Mol Imaging 2013;40:1005-13. [Crossref] [PubMed]
- Naqa IE. The role of quantitative PET in predicting cancer treatment outcomes. Clin Transl Imaging 2014;2:305-20. [Crossref]
- Tixier F, Hatt M, Valla C, et al. Visual versus quantitative assessment of intratumor 18F-FDG PET uptake heterogeneity: prognostic value in non-small cell lung cancer. J Nucl Med 2014;55:1235-41. [Crossref] [PubMed]
- Grootjans W, Tixier F, van der Vos CS, et al. The impact of optimal respiratory gating and image noise on evaluation of intra-tumor heterogeneity in 18F-FDG positron emission tomography imaging of lung cancer. J Nucl Med 2016;57:1692-8. [Crossref] [PubMed]
- Salavati A, Duan F, Snyder BS, et al. Optimal FDG PET/CT volumetric parameters for risk stratification in patients with locally advanced non-small cell lung cancer: results from the ACRIN 6668/RTOG 0235 trial. Eur J Nucl Med Mol Imaging 2017;44:1969-83. [Crossref] [PubMed]
- Downey RJ, Akhurst T, Gonen M, et al. Fluorine-18 fluorodeoxyglucose positron emission tomographic maximal standardized uptake value predicts survival independent of clinical but not pathologic TNM staging of resected non-small cell lung cancer. J Thorac Cardiovasc Surg 2007;133:1419-27. [Crossref] [PubMed]
- Haralick RM, Shanmugam K, Dinstein I. Textural features for image classification. IEEE Trans Syst Man Cybern 1973;3:610-21. [Crossref]
- Galloway MM. Texture analysis using gray level run lengths. Comput Graph Image Process 1975;4:172-9. [Crossref]
- Thibault G, Angulo J, Meyer F. Advanced statistical matrices for texture characterization: application to cell classification. IEEE Trans Biomed Eng. 2014;61:630-7. [Crossref] [PubMed]
- Amadasun M, King R. Textural features corresponding to textural properties. IEEE Trans Syst Man Cybern 1989;19:1264-74. [Crossref]
- Cook GJR, Yip C, Siddique M, et al. Are pre-treatment 18F-FDG PET tumor textural features in non-small cell lung cancer associated with response and survival after chemoradiotherapy? J Nucl Med 2013;54:19-26. [Crossref] [PubMed]
- Pyka T, Bundschuh RA, Andratschke N, et al. Textural features in pre-treatment (F18)-FDG-PET/CT are correlated with risk of local recurrence and disease-specific survival in early stage NSCLC patients receiving primary stereotactic radiation therapy. Radiat Oncol 2015;10:100. [Crossref] [PubMed]
- Ohri N, Duan F, Snyder BS, et al. Pre-treatment FDG PET Textural Features in Locally Advanced NSCLC Secondary Analysis of ACRIN 6668/RTOG 0235. J Nucl Med 2016;57:842-8. [Crossref] [PubMed]
- Brooks FJ, Grigsby PW. The effect of small tumor volumes on studies of intratumoral heterogeneity of tracer uptake. J Nucl Med 2014;55:37-42. [Crossref] [PubMed]
- Soret M, Bacharach SL, Buvat I. Partial-volume effect in PET tumor imaging. J Nucl Med 2007;48:932-45. [Crossref] [PubMed]
- van Velden FHP, Kramer GM, Frings V, et al. Repeatability of Radiomic Features in Non-Small-Cell Lung Cancer (18F)FDG-PET/CT Studies: Impact of Reconstruction and Delineation. Mol Imaging Biol 2016;18:788-95. [Crossref] [PubMed]
- Leijenaar RT, Nalbantov G, Carvalho S, et al. The effect of SUV discretization in quantitative FDG-PET radiomics: the need for standardized methodology in tumor texture analysis. Sci Rep 2015;5:11075. [Crossref] [PubMed]
- Desseroit MC, Tixier F, Weber WA, et al. Reliability of PET/CT Shape and Heterogeneity Features in Functional and Morphologic Components of Non-Small Cell Lung Cancer Tumors: A Repeatability Analysis in a Prospective Multicenter Cohort. J Nucl Med 2017;58:406-11. [Crossref] [PubMed]
- Fave X, Mackin D, Yang J, et al. Can radiomics features be reproducibly measured from CBCT images for patients with non-small cell lung cancer? Med Phys 2015;42:6784-97. [Crossref] [PubMed]
- Chalkidou A, O'Doherty MJ, Marsden PK. False Discovery Rates in PET and CT Studies with Texture Features: A Systematic Review. PLoS One 2015;10. [Crossref] [PubMed]
- Zwanenburg A, Leger S, Vallières M, et al. Image biomarker standardisation initiative. arXiv:1612.07003.
- Collins GS, Reitsma JB, Altman DG, et al. Transparent reporting of a multivariable prediction model for individual prognosis or diagnosis (TRIPOD): the TRIPOD Statement. Ann Intern Med 2015;162:55-63. [Crossref] [PubMed]
- Lambin P, Leijenaar RTH, Deist TM, et al. Radiomics: the bridge between medical imaging and personalized medicine. Nat Rev Clin Oncol 2017;14:749-62. [Crossref] [PubMed]
- Yang W, Zhang Y, Fu Z, et al. Imaging of proliferation with 18F FLT PET/CT versus 18F-FDG PET/CT in non-small-cell lung cancer. Eur J Nucl Med Mol Imaging 2010;37:1291-9. [Crossref] [PubMed]
- Bollineni VR, Kramer GM, Jansma EP, et al. A systematic review on (18F)FLT-PET uptake as a measure of treatment response in cancer patients. Eur J Cancer 2016;55:81-97. [Crossref] [PubMed]
- Szyszko TA, Yip C, Szlosarek P, et al. The role of new PET tracers for lung cancer. Lung Cancer 2016;94:7-14. [Crossref] [PubMed]
- Everitt SJ, Ball DL, Hicks RJ, et al. Differential (18)F-FDG and (18)F-FLT Uptake on Serial PET/CT Imaging Before and During Definitive Chemoradiation for Non-Small Cell Lung Cancer. J Nucl Med 2014;55:1069-74. [Crossref] [PubMed]
- Tian J, Yang X, Yu L, et al. A multicenter clinical trial on the diagnostic value of dual-tracer PET/CT in pulmonary lesions using 3'-deoxy-3'-18F-fluorothymidine and 18F-FDG. J Nucl Med 2008;49:186-94. [Crossref] [PubMed]
- Crandall JP, Tahari AK, Juergens RA, et al. A comparison of FLT to FDG PET/CT in the early assessment of chemotherapy response in stages IB-IIIA resectable NSCLC. EJNMMI Res 2017;7:8. [Crossref] [PubMed]
- Scheffler M, Zander T, Nogova L, et al. Prognostic Impact of (18F)fluorothymidine and (18F)fluoro-D-glucose baseline uptakes in patients with lung cancer treated first-line with erlotinib. PLoS One 2013;8. [Crossref] [PubMed]
- Vaupel P, Mayer A. Hypoxia in cancer: significance and impact on clinical outcome. Cancer Metastasis Rev 2007;26:225-39. [Crossref] [PubMed]
- Yi CA, Lee KS, Kim EA, et al. Solitary pulmonary nodules: dynamic enhanced multi-detector row CT study and comparison with vascular endothelial growth factor and microvessel density. Radiology 2004;233:191-9. [Crossref] [PubMed]
- Hanna GG, van Sörnsen de Koste JR, Dahele MR, et al. Defining target volumes for stereotactic ablative radiotherapy of early-stage lung tumours: a comparison of three-dimensional 18F-fluorodeoxyglucose positron emission tomography and four-dimensional computed tomography. Clin Oncol (R Coll Radiol) 2012;24:e71-80. [Crossref] [PubMed]
- Wolthaus JW, Schneider C, Sonke JJ, et al. Mid-ventilation CT scan construction from four-dimensional respiration-correlated CT scans for radiotherapy planning of lung cancer patients. Int J Radiat Oncol Biol Phys 2006;65:1560-71. [Crossref] [PubMed]
- Wolthaus JW, Sonke JJ, van Herk M, et al. Reconstruction of a time-averaged midposition CT scan for radiotherapy planning of lung cancer patients using deformable registration. Med Phys 2008;35:3998-4011. [Crossref] [PubMed]
- Nanni C, Zompatori M, Ambrosini V, et al. The additional diagnostic value of contemporary evaluation of FDG PET/CT scan and contrast enhanced CT imaging both acquired by a last generation PET/CT system in oncologic patients. Biomed Pharmacother 2013;67:172-8. [Crossref] [PubMed]
- Ito R, Iwano S, Shimamoto H, et al. A comparative analysis of dual-phase dual-energy CT and FDG-PET/CT for the prediction of histopathological invasiveness of non-small cell lung cancer. Eur J Radiol 2017;95:186-91. [Crossref] [PubMed]
- Iwano S, Ito R, Umakoshi H, et al. Evaluation of lung cancer by enhanced dual-energy CT: association between three-dimensional iodine concentration and tumour differentiation. Br J Radiol 2015;88. [Crossref] [PubMed]
- Mansoor A, Bagci U, Foster B, et al. Segmentation and Image Analysis of Abnormal Lungs at CT: Current Approaches, Challenges, and Future Trends. Radiographics 2015;35:1056-76. [Crossref] [PubMed]
- Caldwell CB, Mah K, Ung YC, et al. Observer variation in contouring gross tumor volume in patients with poorly defined nonsmall-cell lung tumors on CT: The impact of 18FDG-hybrid PET fusion. Int J Radiat Oncol Biol Phys 2001;51:923-31. [Crossref] [PubMed]
- Greco C, Rosenzweig K, Cascini GL, et al. Current status of PET/CT for tumour volume definition in radiotherapy treatment planning for non-small cell lung cancer (NSCLC). Lung Cancer 2007;57:125-34. [Crossref] [PubMed]
- Steenbakkers RJ, Duppen JC, Fitton I, et al. Observer variation in target volume delineation of lung cancer related to radiation oncologist-computer interaction: a ‘Big Brother’ evaluation. Radiother Oncol 2005;77:182-90. [Crossref] [PubMed]
- Fox JL, Rengan R, O'Meara W, et al. Does registration of PET and planning CT images decrease interobserver and intraobserver variation in delineating tumor volumes for non-small-cell lung cancer? Int J Radiat Oncol Biol Phys 2005;62:70-5. [Crossref] [PubMed]
- Konert T, Vogel WV, Everitt S, et al. Multiple training interventions significantly improve reproducibility of PET/CT-based lung cancer radiotherapy target volume delineation using an IAEA study protocol. Radiother Oncol 2016;121:39-45. [Crossref] [PubMed]
- Rasch C, Belderbos J, van Giersbergen A, et al. The Influence of A Multi-disciplinary Meeting for Quality Assurance on Target Delineation in Radiotherapy Treatment Preparation. Int J Radiat Oncol Biol Phys 2009;75:S452-3. [Crossref]
- Doll C, Duncker-Rohr V, Rücker G, et al. Influence of experience and qualification on PET-based target volume delineation. When there is no expert--ask your colleague. Strahlenther Onkol 2014;190:555-62. [Crossref] [PubMed]
- Werner-Wasik M, Nelson AD, Choi W, et al. What is the best way to contour lung tumors on PET scans? Multiobserver validation of a gradient-based method using a NSCLC digital PET phantom. Int J Radiat Oncol Biol Phys 2012;82:1164-71. [Crossref] [PubMed]
- Cui H, Wang X, Feng D. Automated localization and segmentation of lung tumor from PET-CT thorax volumes based on image feature analysis. Conf Proc IEEE Eng Med Biol Soc 2012;2012:5384-7. [PubMed]
- Bayne M, Hicks RJ, Everitt S, et al. Reproducibility of "intelligent" contouring of gross tumor volume in non-small-cell lung cancer on PET/CT images using a standardized visual method. Int J Radiat Oncol Biol Phys 2010;77:1151-7. [Crossref] [PubMed]
- Foster B, Bagci U, Mansoor A, et al. A review on segmentation of positron emission tomography images. Comput Biol Med 2014;50:76-96. [Crossref] [PubMed]
- MacManus M, Nestle U, Rosenzweig KE, et al. Use of PET and PET/CT for radiation therapy planning: IAEA expert report 2006-2007. Radiother Oncol 2009;91:85-94. [Crossref] [PubMed]
- Schinagl DA, Vogel WV, Hoffmann AL, et al. Comparison of five segmentation tools for 18F-fluoro-deoxy-glucose-positron emission tomography-based target volume definition in head and neck cancer. Int J Radiat Oncol Biol Phys 2007;69:1282-9. [Crossref] [PubMed]
- Shepherd T, Teras M, Beichel R, et al. Comparative study with new accuracy metrics for target volume contouring in PET image guided radiation therapy. IEEE Trans Med Imaging 2012;31:2006-24. [Crossref] [PubMed]
- Wu K, Ung YC, Hornby J, et al. PET CT thresholds for radiotherapy target definition in non-small-cell lung cancer: how close are we to the pathologic findings? Int J Radiat Oncol Biol Phys 2010;77:699-706. [Crossref] [PubMed]
- van Loon J, Siedschlag C, Stroom J, et al. Microscopic disease extension in three dimensions for non-small-cell lung cancer: development of a prediction model using pathology-validated positron emission tomography and computed tomography features. Int J Radiat Oncol Biol Phys 2012;82:448-56. [Crossref] [PubMed]
- Schaefer A, Vermandel M, Baillet C, et al. Impact of consensus contours from multiple PET segmentation methods on the accuracy of functional volume delineation. Eur J Nucl Med Mol Imaging 2016;43:911-24. [Crossref] [PubMed]
- Weiss GJ, Korn RL. Interpretation of PET scans: do not take SUVs at face value. J Thorac Oncol 2012;7:1744-6. [Crossref] [PubMed]
- Wang H, Zhou Z, Li Y, et al. Comparison of machine learning methods for classifying mediastinal lymph node metastasis of non-small cell lung cancer from 18F-FDG PET/CT images. EJNMMI Res 2017;7:11. [Crossref] [PubMed]
- LeCun Y, Bengio Y, Hinton G. Deep learning. Nature 2015;521:436-44. [Crossref] [PubMed]
- Lee JG, Jun S, Cho YW, et al. Deep learning in medical imaging: general overview. Korean J Radiol 2017;18:570-84. [Crossref] [PubMed]
- Kalpathy-Cramer J, Freymann JB, Kirby JS, et al. Quantitative Imaging Network: data sharing and competitive algorithm validation leveraging the Cancer Imaging Archive. Transl Oncol 2014;7:147-52. [Crossref] [PubMed]
- Aide N, Lasnon C, Veit-Haibach P, et al. EANM/EARL harmonization strategies in PET quantification: from daily practice to multicentre oncological studies. Eur J Nucl Med Mol Imaging 2017;44:17-31. [Crossref] [PubMed]
- Kruis MF, van de Kamer JB, Houweling AC, et al. PET motion compensation for radiation therapy using a CT-based mid-position motion model: methodology and clinical evaluation. Int J Radiat Oncol Biol Phys 2013;87:394-400. [Crossref] [PubMed]
- Kruis MF, van de Kamer JB, Sonke JJ, et al. Registration accuracy and image quality of time averaged mid-position CT scans for liver SBRT. Radiother Oncol 2013;109:404-8. [Crossref] [PubMed]
- Lamare F, Fayad H, Fernandez P, et al. Local respiratory motion correction for PET/CT imaging: Application to lung cancer. Med Phys 2015;42:5903-12. [Crossref] [PubMed]
- Yan J, Chu-Shern JL, Loi HY, et al. Impact of image reconstruction settings on texture features in 18FFDG PET. J Nucl Med 2015;56:1667-73. [Crossref] [PubMed]
- Cheebsumon P, van Velden FH, Yaqub M, et al. Effects of image characteristics on performance of tumor delineation methods: a test-retest assessment. J Nucl Med 2011;52:1550-8. [Crossref] [PubMed]
- Leijenaar RT, Carvalho S, Velazquez ER, et al. Stability of FDG-PET Radiomics feature: an integrated analysis of test-retest and inter-observer variability. Acta Oncol 2013;52:1391-7. [Crossref] [PubMed]
- Parmar C, Rios Velazquez E, Leijenaar R, et al. Robust Radiomics feature quantification using semiautomatic volumetric segmentation. PLoS One 2014;9. [Crossref] [PubMed]
- Larobina M, Murino L. Medical image file formats. J Digit Imaging 2014;27:200-6. [Crossref] [PubMed]
- Wu W, Parmar C, Grossmann P, et al. Exploratory Study to Identify Radiomics Classifiers for Lung Cancer Histology. Front Oncol 2016;6:71. [Crossref] [PubMed]
- Antoch G, Bockisch A. Combined PET/MRI: a new dimension in whole-body oncology imaging? Eur J Nucl Med Mol Imaging 2009;36 Suppl 1:S113-20. [Crossref] [PubMed]
- Heusch P, Buchbender C, Köhler J, et al. Thoracic staging in lung cancer: prospective comparison of 18F-FDG PET/MR imaging and 18F-FDG PET/CT. J Nucl Med 2014;55:373-8. [Crossref] [PubMed]
- Schwenzer NF, Schraml C, Müller M, et al. Pulmonary lesion assessment: comparison of whole-body hybrid MR/PET and PET/CT imaging—pilot study. Radiology 2012;264:551-8. [Crossref] [PubMed]
- Ohno Y, Koyama H, Yoshikawa T, et al. Three-way Comparison of Whole-Body MR, Coregistered Whole-Body FDG PET/MR, and Integrated Whole-Body FDG PET/CT Imaging: TNM and Stage Assessment Capability for Non-Small Cell Lung Cancer Patients. Radiology 2015;275:849-61. [Crossref] [PubMed]
- Aristophanous M, Berbeco RI, Killoran JH, et al. Clinical utility of 4D FDG-PET/CT scans in radiation treatment planning. Int J Radiat Oncol Biol Phys 2012;82:e99-105. [Crossref] [PubMed]
- Bettinardi V, Castiglioni I, De Bernardi E, et al. PET quantification: strategies for partial volume correction. Clin Transl Imaging 2014;2:199-218. [Crossref]
- Siva S, Hardcastle N, Kron T, et al. Ventilation/Perfusion Positron Emission Tomography--Based Assessment of Radiation Injury to Lung. Int J Radiat Oncol Biol Phys 2015;93:408-17. [Crossref] [PubMed]
- Everitt S, Ball D, Hicks RJ, et al. Prospective Study of Serial Imaging Comparing Fluorodeoxyglucose Positron Emission Tomography (PET) and Fluorothymidine PET During Radical Chemoradiation for Non-Small Cell Lung Cancer: Reduction of Detectable Proliferation Associated With Worse Survival. Int J Radiat Oncol Biol Phys 2017;99:947-55. [Crossref] [PubMed]
- Kerner GS, Bollineni VR, Hiltermann TJ, et al. An exploratory study of volumetric analysis for assessing tumor response with (18)F-FAZA PET/CT in patients with advanced non-small-cell lung cancer (NSCLC). EJNMMI Res 2016;6:33. [Crossref] [PubMed]
- Bahce I, Yaqub M, Smit EF, et al. Personalizing NSCLC therapy by characterizing tumors using TKI-PET and immuno-PET. Lung Cancer 2017;107:1-13. [Crossref] [PubMed]
- Wright CL, Binzel K, Zhang J, et al. Advanced Functional Tumor Imaging and Precision Nuclear Medicine Enabled by Digital PET Technologies. Contrast Media Mol Imaging 2017;2017. [Crossref] [PubMed]
- Kolbitsch C, Neji R, Fenchel M, et al. Respiratory-resolved MR-based attenuation correction for motion-compensated cardiac PET-MR. Phys Med Biol 2018;63. [Crossref] [PubMed]