Forecasting pulmonary air leak duration following lung surgery using transpleural airflow data from a digital pleural drainage device
Introduction
Prolonged air leak (PAL) can afflict 5–25% of patients after pulmonary resections (1-3). PAL is defined as an air leak lasting longer than 5 days postoperatively and has been associated with increasing length of hospital stay and other post-operative morbidity such as pneumonia, empyema, and atelectasis (4-6). Many patients with PAL may be discharged home with a portable chest drain valve device. Extended length of stay and associated complications from PAL can impair the patient’s quality of life and increase health care costs (7). Various algorithms, nomograms, and scoring systems have also been proposed to predict patients likely to develop PAL (1-3,8-12). However, there has yet to be a widespread adoption of a single predictive model because of complexity associated with implementation.
The introduction of digital drainage devices permit continuous measurement and recording of parameters suggest as airflow and intrapleural pressure in the pleural space. The use of these digital devices has been shown to decrease inter-observer variability in the management of patients with chest drains (13). Research focusing on transforming digital drainage data into relevant information to guide patient care holds promise and is in its infancy (14). Our goal was to develop a statistical model that reliably predicts pulmonary air leak resolution by applying statistical time series modeling and forecasting techniques to digital drainage data. From an air leak management standpoint, we also postulated that the forecasted data could be used to predict whether or not it would be safe for a patient to have their last chest tube removed prior to discharge.
Methods
Autoregressive Integrated Moving Average (ARIMA) time series modeling
We used an ARIMA time series modeling approach to forecast air leak flow from transpleural airflow data (15). ARIMA is a widely used statistical analysis model, similar to a regression analysis, that predicts future trends. ARIMA examines differences in past values to predict current value and its main application is in short term forecasting. It is particularly useful when there is little mathematical background knowledge about the underlying data and trends (16). For our model, the minimum historical time window to provide enough observations to train the model was set at 16 hours (THistorical). The time into the future for which values were predicted was defined as (THorizon). THorizon was set at 24 hours to represent the best compromise between accurate forecasting and sufficient lead time to guide clinical practice. Details pertaining to the model can be found in the appendix (Supplementary I).
Predicting pulmonary air leak resolution
ARIMA was used to generate a 95% confidence interval (95% CI) for the forecasted airflow in the 24-hour forecasting horizon. As a safety margin, we extended this horizon to include a 3-hour post-leak-resolution period to ensure that air leak resolution criteria were still met. The time at the end of the 3-hour safety period was used to define the predicted chest tube duration in the air leak forecasting model (TPred).
Data collection
Once we established our ARIMA statistical model, we internally validated our model by retrospectively reviewing 100 patients who underwent pulmonary resection between December 2012 and March 2017. Ethics approval was obtained from our institutional research ethics board. We included any patient that had both digital drainage device data available for analysis and for whom persistent air leak was the limiting factor in chest tube removal. Cases included both benign and oncologic disease process as well as elective and emergency cases. Airflow and fluid output were recorded prospectively using a digital drainage device (Thopaz-Plus™, Medela, Switzerland).
Clinical chest tube protocol
Our institutional clinical chest tube removal protocol is based on resolution of parenchymal air leak, volume of chest tube drainage, status of subcutaneous emphysema and results of chest X-ray. Staff surgeons as well as residents under the guidance of staff, assessed the patients twice a day. Removal of the last chest tube was considered safe when the following criteria were met:
- Resolution of air leak: initially we had a more conservative criteria of a air flow threshold of 20 mL/min with a pleural pressure −8 mmHg or 40 mL/min with set pleural pressure <−8 mmHg both over 12 hours. However, subsequent to ongoing assessment of our outcomes, a less conservative target of 30 mL/min over 8 hours regardless of the intrapleural pressure.
- Pleural fluid output: volume less than five times body weight in kilograms in a 24 hour period (i.e., ≤20% of whole-body lymphatic flow) (2,4,8).
- Status of subcutaneous emphysema: absent, mild, or stable over 24-hour period.
- Results of chest X-ray (CXR): absent or small pneumothorax, absent or small amount of pleural effusions.
Assessing chest tube duration
We reviewed patient’s chart to determine optimal duration of chest tube placement (TOpt). Chest tubes are inserted at the end of the case; therefore we used the end surgical time as a surrogate for chest tube insertion. TOpt was defined as the length of time between chest tube insertion to the time when the last chest tube fulfilled the chest tube protocol criteria (resolution of air leak, appropriate pleural drainage, stable subcutaneous emphysema, benign and/or stable chest X-ray).
Specificity and sensitivity of the model was determined by comparing the calculated TPred to the clinical TOpt. The predicted chest tube removal time was considered clinically appropriate when it was within 12 hours in advance of the optimal removal time. Twelve hours was arbitrarily selected as a conservative number for patient safety.
Refining the model to account for delay
In clinical practice, a potential reason for delay is the availability of qualified personnel to immediately assess and remove a chest tube. Also, at our institution, chest tubes are not removed in the evening or overnight. Our model was adjusted to take these factors into account. The details are described in the appendix (Supplementary II).
Statistical analysis
Numerical continuous data were expressed as a median value with a 25th–75th percentile interquartile range (IQR). Statistical analysis of ordinal variables was conducted. Confidence intervals (CI) and hypothesis testing were performed with statistical significance level α=0.05. All tests of significance were two-sided. Categorical variables were analyzed for statistical significance using Fisher’s exact test. Numerical variables were compared using the Student’s t-test or the Wilcoxon rank sum test. Statistical analyses were carried out using SPSS24 (IBM, Armonk, NY) and Excel 2010 (Microsoft, Redmond, WA).
Results
Demographics
The baseline characteristics of the 100 patients are summarized in Table 1. Ninety percent (90/100) of patients had a diagnosis of lung cancer, 60% (60/100) were female, and 64% (64/100) had a history of smoking. The majority of patients underwent lobectomies, and video-assisted thoracoscopic surgery (VATS) was the most common approach.
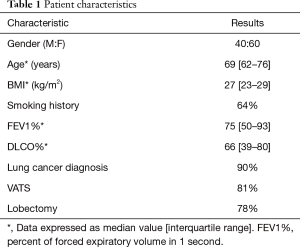
Full table
Accuracy of our model
In total, the model correctly identified 82% (82/100) of patients as to whether or not removal of the last chest tube was indicated (n=60 chest tube remain; n=22 remove chest tube) (Table 2).
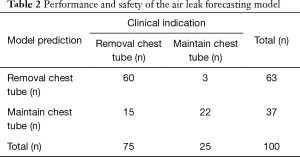
Full table
Chest tube removal was deemed clinically appropriate in 75% (75/100) of the patients in this study. Of these 75 patients, 80% (60/75) were correctly identified using the air leak forecasting model.
For the group of 25 patients for whom chest tube removal was not clinically indicated, 88% (22/25) were appropriately classified as having a non-resolving air leak. The performance characteristics of the model in properly identifying patients whose air leak would resolve and who would therefore be candidates for safe chest tube removal were: sensitivity 80% (95% CI, 69–88%), specificity 88% (95% CI, 68–97%), positive predictive value 95% (95% CI, 86–99%), and negative predictive value 59% (95% CI, 42–79%). The false positive and false negative rate was 12% (95% CI, 12–31%) and 20% (95% CI, 12–31%), respectively. The median absolute error on forecasted air leak flow was 5.2 mL/min (IQR, 2.4–14.4 mL/min).
As shown in Table 2, the model forecasted resolution of the air leak and thus predicted that all chest tubes could be safely removed in 63/100 of the patients. Tpred was clinically appropriate (i.e., within the 12 hours preceding TOpt) in 84% of these patients (53/63; 95% CI, 73–91%). In the remaining 16% (10/63) of patients for whom Tpred was deemed not clinically appropriate, three were misclassified by the model because the air leak initially resolved and subsequently recurred. The latter three patients were discharged with an indwelling chest tube attached to a portable device because of a PAL. Figure 1 illustrates one of these three cases. In this example, the chest tube removal criteria were satisfied three times between periods of air leak recurrence.
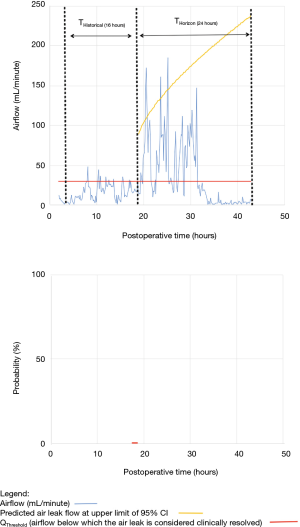
For the other seven patients, air leak resolution predictions were accurate. In 6 of these 7 cases, TPred appropriately coincided with a period when the air leak resolution criteria were met. However, there were clinically insignificant brief airflow spikes above QThreshold [maximum airflow threshold value over a period of length ≥ TClinical criteria (length of minimum time period over which airflow ≤ QThreshold)] which delayed chest tube removal until they eventually subsided over a median period of time of 28 hours (IQR, 23–33.5 hours).
Discussion
As was the case for cardiac monitors over 50 years ago, digital drainage devices have introduced a continuous stream of clinical data from patients who have a chest tube. In-depth analysis of pleural space monitoring data to generate clinically actionable information is an emerging field of study (17-19). Many factors are involved in successfully managing patients with chest drains. Clinical decisions revolving around parenchymal air leaks may be challenging for novices and experts alike. We were able to develop a statistical model that reliably predicted resolution of PAL forecasting over a 24-hour period. The model performance was within clinically acceptable safety boundaries when differentiating patients whose air leak would soon resolve from those whose air leak would be prolonged. The 24-hour prediction window provided by the current model would provide sufficient time for the surgical team to plan ahead for chest tube removal or discharge with an indwelling tube.
Clinical application of the model is illustrated using a single patient’s data in Figures 2-5. At any point in time, air leak flow can be forecasted based on the preceding 16 hours. The time to air leak resolution can be predicted by evaluating whether or not the predicted air leak flow remains below a predetermined threshold of 30 mL/min for a sufficient amount of time. If the predicted air leak remained below threshold, the air leak is considered resolved and the chest tube can be removed.
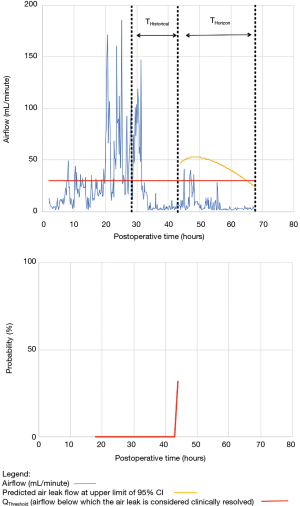
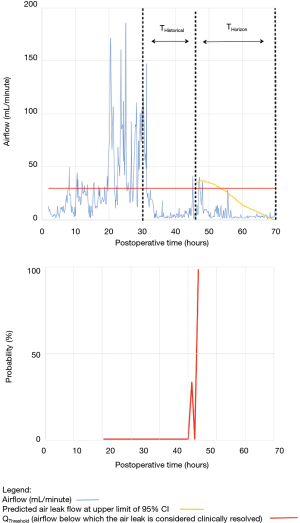
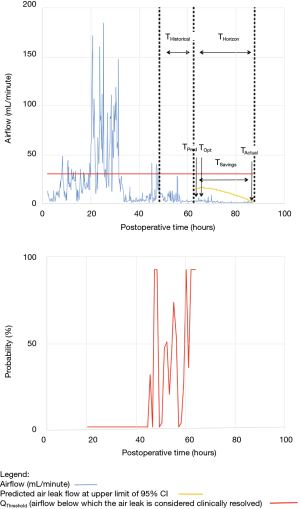
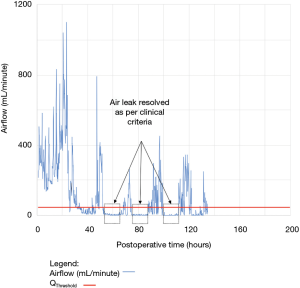
We know from previous institutional experience that up to 6% of patients may require chest tube re-insertion for a recurrent or undetected persistent air leak. As a safety measure, we required that the upper limit of the 95% CI of the predicted airflow satisfy the set of criteria for discontinuing pleural drainage. Since the purpose of this model is to assist rather than replace the clinical team, it seems unlikely that the three patients whose air leak was incorrectly predicted to resolve would have experienced adverse consequences.
We recognized that limitations associated with a retrospective study design introduce bias in the results. The focus on safety and reducing false-positives led to a low negative predictive value (59%). Also, the proposed model has limited capabilities to forecast recurrence of an air leak after a transient period of complete resolution (non-stationarity airflow variations). Prediction errors due to fluctuation in transpleural airflow data may be minimized by exploring alternative forecasting strategies. For instance, artificial neural network, a type of adaptive model, has been shown to perform well in the presence of significant non-stationarity, when combined with ARIMA (16). We think that successful transformation of pleural space monitoring data into clinically relevant information will require a combination of various dynamic and adaptive modeling approaches.
The literature has had mixed results on whether digital devices provides additional benefit over a traditional device (13,20,21). We believe that using digital devices to forecast PAL resolution demonstrates that digital devices have great potential to be integrated into an effective clinical decision system. For instance, if an air leak is anticipated to quickly resolve, the patient may remain admitted to hospital with the goal of removing chest tube prior to discharge for a better quality of life. However, if the digital device indicates a non-resolving air leak, we may discharge the patient with a portable chest valve drain or consider use of pleurodesis. The ability to predict the nature of the PAL could shorten length of stay. More research efforts are needed to develop such clinical decision support systems and evaluate their impact on clinical outcomes.
Supplementary
I ARIMA time series modeling
An ARIMA time series modeling approach was used to forecast air leak flow from transpleural airflow data (9). The minimum historical time window to provide enough observations to effectively train the model was 16 hours (THistorical). If the current time is TNow, the historical data window extends from TNow–16 hours until TNow (TNow–16, TNow). For each patient, numerous ARIMA(p, d, q) models were tested by varying parameters p, d, and q, over the set of values [0, 1, 2, 3, 4, 5]. The optimal ARIMA(p, d, q) model was selected based on two performance criteria: the Bayesian Information Criterion (BIC) and the Real Mean Square Root (RMSE) (10). The BIC model selection criterion trains the model on (TNow–16, TNow) and selects the ARIMA(p1, d1, q1) model which minimizes the BIC over this interval (2). The RMSE model selection criterion trains the model on the time window (TNow–16, TNow–8) and selects the ARIMA(p2, d2, q2) model which minimizes the forecasting RMSE over the subsequent interval (TNow–8, TNow). The optimal ARIMA(p, d, q) model was the average of ARIMA(p1, d1, q1) and ARIMA(p2, d2, q2) fitted models. The optimal ARIMA(p, d, q) model was selected based on historical transpleural airflow measurements observed up to TNow on a patient-by-patient basis. Future values of these measurements were forecasted with the model over a specified time horizon (THorizon), (TNow, TNow+THorizon). Airflow readings were analyzed retrospectively for each patient. Within the dataset, forecasting was calculated progressively as time elapsed. The optimal forecasting model for each patient was regenerated every hour. An analysis of various durations of THorizon was performed. THorizon =24 hours represented the best compromise between generating an accurate forecast and providing sufficient lead time to be clinically relevant to the healthcare team.
II Adjusting for availability of qualified personnel
This specific constraint was taken into account by creating a constant (R), arbitrarily set to 6 hours, and subtracting it from any delay period. Chest tubes are not typically removed in the evening or at night. If TPred or TOpt ended between 18:00 and 06:00 then the chest tube removal time was put on hold for the number of hours until the next 06:00 time slot (THold).
Our formula:
For example, if a patient was deemed to have met all clinical criteria for chest tube removal by 21:00 on Wednesday and the last chest tube was removed the next day at 16:00.
TOpt = 21:00
TAct = 16:00 the following day
=Number of hour delay between optimal time of removal and actual time of removal =19 hours
THold = time between 21:00 and 06:00 =9 hours
R =6 hours
Therefore:
TDelay=19–(6+9)= 4 hours.
Acknowledgements
We would also like to thank Dr. Jennifer Dawson for her assistance manuscript preparation.
Funding: This study is supported by the Innovation Grant from the Ministry of Health and Long-term Care Innovation and Ontario Medical Association (grant No. TOH 15-001).
Footnote
Conflicts of Interest: The authors have no conflicts of interest to declare.
Ethical Statement: Ethics approval was obtained from our institutional research ethics board (20120228-01H).
References
- Rivera C, Bernard A, Falcoz P, et al. Characterization and prediction of prolonged air leak after pulmonary resection: a nationwide study setting up the index of prolonged air leak. Ann Thorac Surg 2011;92:1062-8. [Crossref] [PubMed]
- Brunelli A, Varela G, Refai M, et al. A scoring system to predict the risk of prolonged air leak after lobectomy. Ann Thorac Surg 2010;90:204-9. [Crossref] [PubMed]
- Gilbert S, Maghera S, Seely AJ, et al. Identifying patients at higher risk of prolonged air leak after lung resection. Ann Thorac Surg 2016;102:1674-9. [Crossref] [PubMed]
- Brunelli A, Cassivi S, Halgren L. Risk factors for prolonged air leak after pulmonary resection. Thorac Surg Clin 2010;20:359-64. [Crossref] [PubMed]
- Pompili C, Miserocchi G. Air leak after lung resection: pathophysiology and patients’ implications. J Thorac Dis 2016;8:S46-54. [PubMed]
- Drewbrook C, Das S, Mousadoust D, et al. Prolonged air leak in 269 consecutive pulmonary resection patients over nine months: a single-center retrospective cohort study. Open Journal of Thoracic Surgery 2016;6:33-46. [Crossref]
- Varela G, Jiménez MF, Novoa N, et al. Estimating hospital costs attributable to prolonged air leak in pulmonary lobectomy. Eur J Cardiothorac Surg 2005;27:329-33. [Crossref] [PubMed]
- Attaar A, Winger DG, Luketich JD, et al. A clinical prediction model for prolonged air leak after pulmonary resection. J Thorac Cardiovasc Surg 2017;153:690-9. [Crossref] [PubMed]
- Cerfolio RJ, Bass CS, Pask AH, et al. Predictors and treatment of persistent air leaks. Ann Thorac Surg 2002;73:1727-30; discussion 1730-1.
- Orsini B, Baste JM, Gossot D, et al. Index of prolonged air leak score validation in case of video-assisted thoracoscopic surgery anatomical lung resection: results of a nationwide study based on the French national thoracic database, EPITHOR. Eur J Cardiothorac Surg 2015;48:608-11. [Crossref] [PubMed]
- Lee L, Hanley SC, Robineau C. Estimating the risk of prolonged air leak after pulmonary resection using a simple scoring system. J AM Coll Surg 2011;212:1027-32. [Crossref] [PubMed]
- Pompili C, Falcoz P, Salati M. A risk score to predict the incidence of prolonged air leak after video-assisted thoracoscopic lobectomy: an analysis from the European Society of Thoracic Surgeons database. J Thorac Cardiovasc Surg 2017;153:957-65. [Crossref] [PubMed]
- Gilbert S, McGuire A, Maghera S, et al. Randomized trial of digital versus analog pleural drainage in patients with or without pulmonary air leak after lung resection. J Thorac Cardiovasc Surg 2015;150:1243-9. [Crossref] [PubMed]
- Brunelli A, Cassivi S, Michele S, et al. Digital measurements of air leak flow and intrapleural pressures in the immediate postoperative period predict risk of prolonged air leak after pulmonary lobectomy. European Journal of Cardio-thoracic Surgery 2011;39:584-8. [Crossref] [PubMed]
- Box G. Box and Jenkins: Time Series Analysis, Forecasting and Control. In: A Very British Affair. Palgrave Advanced Texts in Econometrics. London: Palgrave Macmillan, 2013.
- Zhang GP. Time series forecasting using a hybrid ARIMA and neural network model. Neurocomputing 2003;50:159-75. [Crossref]
- George RS, Papagiannopoulos K. Advances in chest drain management in thoracic disease. J Thorac Dis 2016;8:S55-64. [PubMed]
- Anegg U, Lindenmann J, Matzi V, et al. AIRFIX: the first digital postoperative chest tube airflowmetry--a novel method to quantify air leakage after lung resection. Eur J Cardiothorac Surg 2006;29:867-72. [Crossref] [PubMed]
- Cerfolio RJ, Bryant AS. The benefits of continuous and digital air leak assessment after elective pulmonary resection: a prospective study. Ann Thorac Surg 2008;86:396-401. [Crossref] [PubMed]
- Pompili C, Detterbeck F, Papagiannopoulos K, et al. Multicenter international randomized comparison of objective and subjective outcomes between electronic and traditional chest drainage systems. Ann Thorac Surg 2014;98:490-6; discussion 496-7. [Crossref]
- Takamochi K, Nojiri S, Oh S, et al. Comparison of digital and traditional thoracic drainage systems for postoperative chest tube management after pulmonary resection: A prospective randomized trial. J Thorac Cardiovasc Surg 2018;155:1834-40. [Crossref] [PubMed]