Is an activity volume threshold really realistic for lung cancer resection?
Introduction
Measuring the performance of institutions in terms of patient care has become a major challenge which concerns not only surgeons but also health authorities. As Donabedian et al. has shown, quality measurement has three dimensions: structure, process, and results (1).
Among the structural indicators, hospital volume has been shown to be closely linked to the reduction of postoperative mortality (2). Despite convincing literature which indicates that high volume centers have reduced hospital mortality, this indicator is disputed within the surgical community. One of the main critiques concerns the threshold at which the patient death rate can be considered acceptable. Because of the great heterogeneity of publications, there is no consensus regarding a true threshold of hospital volume activity. Many studies have categorized the variable of hospital volume into quintiles or percentiles with very different, and therefore inconclusive, values (2-5).
The other justified criticism indicates that the volume of an institution is an insufficient indicator to measure the performance of a medical team (6,7). For this purpose, the Society of Thoracic Surgeons has developed composite indicators which include result indicators as well as process indicators (6). These remarks are justified, but they deserve to be nuanced if one is interested in the methodology of the studies. In fact, methodologies and databases are very different from one study to another, and have not yet lead to robust conclusions. Factually, it can be said that most published studies demonstrate that volume has a significant influence on postoperative mortality. On the other hand, it is impossible to propose a threshold at which mortality becomes ‘acceptable’ for patients.
The gap in the existing literature led us to suggest a new study whose focus is the estimation of an acceptable threshold of hospital activity necessary to offer a high quality of care to patients. Our work uses a methodology in which volume is analyzed as a continuous variable. This methodology is rarely used for the estimation of a threshold. Another key element of this work is the use of a database that guarantees the completeness of information about centers and patients.
The objective of this work was to assess the hospital volume threshold for lung cancer (LC) surgery which would lead to an acceptable in-hospital mortality (IHM), using data from the French national medico-administrative database.
Methods
Study patients
All data from patients who underwent pulmonary resection for LC in France were collected from January 2005 to December 2016 from the French national medico-administrative database. This database, called PMSI for “Program de Medicalisation des Systèmes d’Informatiques”, was inspired by the US Medicare system. The reliability and validity of PMSI data have already been assessed (8). Routinely collected medical information includes the principal diagnosis, secondary diagnoses and the procedure performed on the patient. Diagnoses identified during the hospital stay are coded according to the International Classification of Diseases, tenth revision (ICD-10) (9-11). We selected patients in whom a diagnosis of primary LC was coded as principal discharge diagnosis (codes C34, C34.0, C34.1, C34.2, C34.3, and C34.9). Procedures are coded according to the CCAM (Classification Commune des Actes Médicaux). For all patients LC was proven by pathologic analyses according to the 2004 World Health Organization classification of LC (12). Surgery-related variables included the surgical approach (thoracotomy, video assisted thoracic surgery), the type of resection (limited resection, lobectomy, bi-lobectomy and pneumonectomy). The limited resection includes wedge resection and segmentectomies.
Patient characteristics
Baseline demographics included age and sex. We included the following comorbidities from the national administrative database: pulmonary disease (chronic bronchitis, emphysema), heart disease (coronary artery disease, cardiac arrhythmia, congestive heart failure, valvular heart disease, pulmonary artery hypertension, pulmonary embolism), peripheral vascular disease, alcoholism, liver disease, cerebrovascular event, neurological diseases (hemiplegia or paraplegia), dementia, diabetes mellitus with or without complications, renal disease, coagulopathy, leukemia, lymphoma, ulcer disease, history of malignant disease, obesity, other therapies (preoperative chemotherapy, steroids) and HIV/AIDS. We also calculated a modified Charlson Comorbidity Index (CCI) as a marker of comorbidity (13). It is a numerically weighted score composed of 17 comorbidities: myocardial infarction, congestive heart failure, peripheral vascular disease, dementia, cerebrovascular disease, chronic pulmonary disease, connective tissue disease, ulcer disease, mild, moderate or severe liver disease, hemiplegia, moderate or severe renal disease, diabetes, leukemia, lymphoma, and HIV/AIDS.
We excluded hospitals with fewer than five lung resections for cancer during this observational period.
Hospital characteristics
In France, establishments that perform LC surgery are classified as non-university public hospitals, private hospitals and university hospitals.
Hospital volume
We determined the volume of patients who were operated for LC using annual condition specific volume averaged over the period of January 1st, 2005 to December 31st, 2016. For the purposes of characterizing the sample, hospital volume was categorized into quintiles: the fifth quintile was defined as hospitals performing more than 70 procedures a year, the fourth was defined as hospitals performing 36 to 70 procedures a year, the third was defined as hospitals performing 16 to 35 procedures a year, the second was defined as hospitals performing 11 to 15 procedures a year and the first quintile as hospitals performing less than 10 procedures a year. For regression analyses, volume was measured as a continuous predictor on the basis of 5-case increments. Hospital volume was represented in 5 different ways in subsequent statistical analyses of the relationship between volume and mortality outcomes. First, volume was represented as a continuous variable, which assumes that the relationship between volume and mortality is linear. Second, the association between volume and mortality was measured using a volume logarithm. Third, the association between volume and mortality was measured using fractional polynomials. Fourth, the association between volume and mortality was measured using restricted cubic spline regression, which allows for more accurate characteristics of nonlinearity in the volume-outcome relationship (14). Fifth, hospital volume was categorized into quintiles.
Death from any cause at 30 days
Our main outcome measure was death from any cause within 30 days of surgery, and those who died later during the same hospitalization.
Statistical analyses
Descriptive data were expressed as count and percentages for qualitative variables, and as means and standard deviations for continuous variables. Comparisons of means were carried out using parametric tests (ANOVA), and Chi-square tests were performed for qualitative variables.
We used a hierarchical logistic regression model to estimate the relationship between hospital volume and death from any cause within 30 days (15). Rates of death were adjusted for age, sex, comorbidity and type of pulmonary resection, including the same variables used for risk-standardization measures developed for a previous publication (16). Comorbidity was measured using modified CCI. The period of surgery was also entered. Interaction effects were sought for all variables included in the model. A two-level hierarchical structure was considered for analysis: patient and hospital. Hospitals were included in the models as random effects, allowing the relationship between volume and death to be different across hospitals (15).
To estimate the volume-threshold, 4 hierarchical logistic regression models were developed using each of the 4 alternative representations of volume, with otherwise identical adjustments for age, sex, comorbidity, type of surgery and period of surgery. For selection the best model we used Akaike’s information criterion (AIC) (15). The discriminative ability of the model was expressed by the area under the receiver operating characteristic curve (AUC ROC) (15).
We calculated the association between the adjusted odds of death within 30 days and hospital volume. We than calculated the volume threshold by examining the relative effect on the adjusted odds of death for increasing the annual volume by 5 patients for a given-size hospital, varying the given-size hospital from an annual volume of 5 procedures to an annual volume of 300 procedures in increment of 5. We defined the volume threshold as the annual hospital volume at which the lower bound of 95% confidence interval (CI) for the odds ratio (OR) reached 1.00. To characterize the uncertainty in the estimated volume threshold, we used bootstrapping techniques based on 1,000 bootstrapped samples to obtain estimates of 95% CI for each volume threshold. For each bootstrap sample, we sampled hospitals with replacement from the original study cohort, fitted the hierarchical logistic regression model to obtain the risk-adjusted OR, and then determined the volume threshold by setting the lower bound of the 95% CI for OR at 1.00. All analyses were performed with STATA 14 statistical software (StataCorp, College Station, Texas, USA).
Results
Patient characteristics
From January 2005 to December 2016, 108,571 patients were operated for LC in France. Patient characteristics according to condition specific hospital volume are reported in Table 1. The observed statistical differences indicate that at least one of the quintiles of hospital volume is different from the others (Table 1). In the high-volume group (>70 procedures per year), there were significantly more male patients, and patients with pulmonary disease, heart disease, peripheral vascular disease, kidney disease, neurological disease, or hematological disease (P<0.0001 for all comparisons) (Table 1). In the high-volume group (>70 procedures per year), there were more patients with a modified CCI score of ≥3 than in the low-volume group (<10 procedures per year) (44% vs. 33%) (P<0.0001) (Table 1).
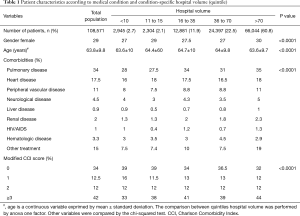
Full table
Management
In the high-volume group, there were significantly more lobectomies than in the low-volume group (70% vs. 64.5%; P<0.0001) (Table 2). The observed statistical differences indicate that at least one of the quintiles of hospital volume is different from the others (Table 2). There was significantly more use of video-assisted thoracic surgery (VATS) in the high volume group than in the low volume group (14% vs. 11%; P<0.0001) (Table 2).
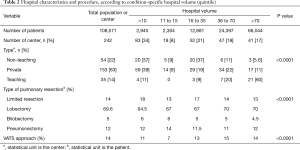
Full table
Hospital characteristics
Over this period, 242 centers performed LC surgery (Table 2), and 83 centers (non-teaching n=20, private n=59 and teaching n=4) performed less than 10 procedures a year (34%) (Table 2). University hospitals were considered high-volume in 60% of cases (21/35) (>70 procedures per year), whereas only 5.6% of non-teaching hospitals (3/54) and 11% of private hospitals had high-volume activity (17/153) (P<0.0001) (Table 2). The percentage of low-volume hospitals decreased over time (Figure 1). The median annual volume for hospitals was of 25 procedures (the 25th percentile: 10 procedures and the 75th percentile: 50 procedures).
Association between volume and mortality
The crude death rate was 5.2% for centers with <10 procedures per year, 4% for center performed between 11 and 15 procedures per year, 4% for center performed between 16 to 35 procedures per year, 3.5% for center performed between 36 to 70 procedures per year and 3.5% in high-volume centers (P<0.0001) (Table 3). Centers performing more than 70 procedures a year reduced the risk of postoperative death by 35% compared to low-volume centers (adjusted OR: 0.65; 95% CI, 0.5–0.84) (Table 3). The association between the hospital volume and risk-adjusted mortality decreased as the hospital’s annual volume increased (Figure 2).
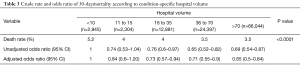
Full table
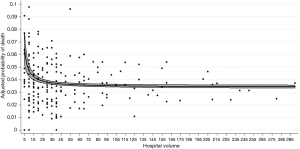
Volume-mortality threshold
Hospital volume was represented in 4 different ways in subsequent statistical analyses of the relationship between volume and mortality outcomes. Among the 4 models, the use of fractional polynomials for volume had the lowest AIC index (Table 4). This model seems the most suitable for showing the relationship between volume and 30-day mortality. The AUC ROC obtained for all models was 0.82 (95% CI, 0.81–0.83), demonstrating that the models have good discrimination.
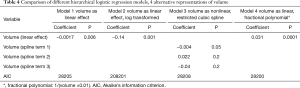
Full table
To identify a volume threshold above which an increase of 5 patients in the annual volume, we used a model with fractional polynomial. The volume threshold was reached once a hospital’s annual volume reached 70 patients (95% CI, 40–85) (Figure 3). In our analyses, the proportion of patients who were admitted to hospitals with an annual volume that was less than identified threshold were 34% of patients operated for LC. For a hospital with an annual volume of only 10 lung resections, an increase to 70 procedures per year would be associated with a 31% reduction in the odds of death within 30 days. However, for a hospital with an initial annual volume of 10 procedures, increasing the annual volume to 40 procedures would be associated with a 27% reduction in the odds of death within 30 days.
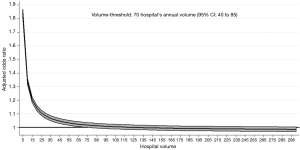
Discussion
We were able to estimate a volume threshold of 70 LC surgeries per year, with an acceptable minimum of 40 surgeries per year. This threshold is above the 30 yearly operations that are required to obtain authorization from the ministry of health (17). The threshold of 30 resections a year includes both therapeutic interventions and diagnostics (17). Our work shows that the official threshold of 30 is inadequate to guarantee quality of care for patients throughout the French territory.
This study reveals that there are too many centers in France performing pulmonary resections for LC as compared to other European countries such as England or Holland (18,19). The dispersion of surgical activity is responsible for an overly high number of centers with low yearly procedure volume in France. Revising the threshold for a number between 40 and 70 pulmonary resections would inevitably lead to a decrease in centers in France, and such a change in the offer of care would send 34% of patients to higher volume structures. From our point of view, it is possible to implement these changes without increasing the wait times for surgery. The reorganization of surgery departments should be part of a regionalization health policy, as has already been done in some countries such as Canada (20). This regionalization adjusts to the real needs of the population within the socio-economic context of the region. The complexity of thoracic surgeries, for example the minimally invasive techniques that are advised to patients, makes the idea of regional technical platforms a change worth considering (21).
Few studies have used volume as a continuous variable for pulmonary resections. A study using volume (22) as a continuous variable failed to show a significant relationship between volume and mortality. The study’s lack of power of could be one reason for the statistical non-significance, especially since postoperative mortality in LC surgery can be considered infrequent. Another French study based on the Epithor database analyzed volume as a continuous variable and did not show a significant relationship between volume and 30-day mortality (23). In this study, 89 French centers actively made up the Epithor (23) database. Over the same period, our study identified and used data from 242 centers in France from the national administrative database. Participating in Epithor was not mandatory, and we can imagine that active centers are motivated and have a volume higher than the national average (23). Volume is unlikely to be a discriminating variable if the selected centers are not representative of French practices in general. We tested several models, and the model that appeared to best describe the change in mortality rate according to volume is the inverse of the variable. To our knowledge this is the first time this model has been used. Some studies have used the log transformation (24), and others have used restricted cubic spline regression (22) which may not be able to accurately describe the relationship between death rate and volume.
Estimating the threshold for volume is implausible in view of current literature data. Systematic review of the literature (25) shows the great diversity of cutoffs used to describe the volume variable. According to the studies selected, the cutoff ranges from 5 to 60 for hospital considered “low-volume” (25) and from 20 to 129 for “high-volume” (25). The heterogeneity of the meta-analysis is 53% for IHM (25). Although the authors show a significant reduction in IHM, we must be cautious because of the heterogeneity of studies, particularly in the choice of the cutoff. The hospital volume is categorized comes from the type of databases and in particular from the number of centers involved. It is easy to understand that when cutting the volume by quintile or percentile, the number of centers and their activity determines the cutoff values. When dealing with few centers with a larger volume, low-volume facilities will have a cutoff of 75 lung resections a year or even 135 as reported in one Asian study (26). In contrast, our study included a large number of centers that perform pulmonary resections, therefore low volume was defined as less than 10 lung resections a year.
We agree that hospital volume is insufficient to assess of quality of care. However, a team will perform LC surgery well if the number of pulmonary resections performed annually is sufficient. Studies have clearly demonstrated the relationship between volume and mortality. The difficulty so far has been to establish a threshold from which the number of interventions per year will result in a high-performance team for the intraoperative and postoperative management of patients. A final consideration is the implementation of new surgical technologies that have a higher learning curve than conventional techniques.
Limitations
There are significant limitations to our study. Given the reliance on ICD-10 codes for the selection of patients and the ascertainment of outcomes, there is a potential for misclassification or under-detection related biases especially for comorbidities. Coding practices vary greatly among institutions and this increases the number of recorded comorbidities. For patients with LC, The French Administrative database does not record information such as stage of the disease, preoperative forced expiratory volume, or the American Society of Anesthesiologist’s score.
Despite these limitations, the strength of our results is our large sample size, stemming from national recruitment. Finally, the results of the present study may be specific to the French healthcare system. Our work may be difficult to extrapolate results to other countries.
Although mortality is an important indicator, the national PMSI database does not provide indicators such as pneumonitis or atelectasis and unplanned readmissions (27). These indicators are essential for quality measurement along with process indicators as demonstrated by STS (28). More and more publications are interested in the volume of activity by surgeon (29,30), but the French database does not currently provide this information.
In conclusion, with data taken from the national PMSI database, we have been able to estimate a minimum volume threshold that could be used to inform the regionalization of thoracic surgery centers. This approach is part of an ongoing process to improve the quality of patient care
Acknowledgements
None.
Footnote
Conflicts of Interest: The authors have no conflicts of interest to declare.
Ethical Statement: This study does not require an ethic approval because it is a study carried out from a database where all data are anonymized. Professor Catherine Quantin has the skill and permission to use the medico-administrative database PMSI.
References
- Donabedian A. the quality of care. How can it be assessed? JAMA 1988;260:1743-8. [Crossref] [PubMed]
- Bach PB, Cramer LD, Schrag D, et al. the influence of hospital volume on survival after resection for lung cancer. N Engl J Med 2001;345:181-8. [Crossref] [PubMed]
- Birkmeyer JD, Siewers AE, Finlayson EVA, et al. Hospital volume and surgical mortality in the united states. N Engl J Med 2002;346:1128-37. [Crossref] [PubMed]
- Christian CK, Gustafson ML, Betensky RA, et al. The Leapfrog Volume Criteria May Fall Short in Identifying High-Quality Surgical Centers. Ann Surg 2003;238:447-55; discussion 455-7. [PubMed]
- Freixinet JL, Julia-Serda G, Rodrıguez PM, et al. Hospital volume: operative morbidity, mortality and survival in thoracotomy for lung cancer. A Spanish multicenter study of 2994 cases. Eur J Cardiothorac Surg 2006;29:20-5. [Crossref] [PubMed]
- Shahian DM, He X, Jacobs JP, et al. The Society of Thoracic Surgeons Isolated Aortic Valve Replacement (AVR) Composite Score: A Report of the STS Quality Measurement Task Force. Ann Thorac Surg 2012;94:2166-71. [Crossref] [PubMed]
- Kozower BD, Stukenborg GJ. Lung cancer resection volume: is procedure volume really an indicator of quality? Semin Thorac Cardiovasc Surg 2012;24:93-8. [Crossref] [PubMed]
- Lorgis L, Cottenet J, Molins G, et al. Outcomes after acute myocardial infarction in HIV-infected patients: analysis of data from a French nationwide hospital medical information database. Circulation 2013;127:1767-74. [Crossref] [PubMed]
- Lainay C, Benzenine E, Durier J, et al. Hospitalization within the first year after stroke: the Dijon stroke registry. Stroke 2015;46:190-6. [Crossref] [PubMed]
- Abdulmalak C, Cottenet J, Beltramo G, et al. Haemoptysis in adults: a 5-year study using the French nationwide hospital administrative database. Eur Respir J 2015;46:503-11. [Crossref] [PubMed]
- Iezzoni LI. Assessing quality using administrative data. Ann Intern Med 1997;127:666-74. [Crossref] [PubMed]
- World Health Organization. International Statistical Classification of Diseases and Related Health Problems 10th Revision. Available online: http://apps.who.int/classifications/icd10/browse/2016/en. Date last accessed: March 1, 2016. Date last updated 2016.
- Charlson M, Szatrowski TP, Peterson J, et al. Validation of a combined comorbidity index. J Clin Epidemiol 1994;47:1245-51. [Crossref] [PubMed]
- Royston P, Altman DG, Sauerbrei W. Dichotomizing conyinuous predictors in multiple regression: a bad idea. Stat Med 2006;25:127-41. [Crossref] [PubMed]
- Steyerberg EW. Clinical Prediction Models: A Practical Approach to Development, Validation, and Updating. New York: Springer-Verlag, 2009.
- Pagès PB, Cottenet J, Mariet AS, et al. In-hospital mortality following lung cancer resection: nationwide administrative database. Eur Respir J 2016;47:1809-17. [Crossref] [PubMed]
- Ministère de l’Enseignement Supérieur et de la Recherche, Ministère de la Santé et des Sports. Plan Cancer 2009–2013. Available online: http://www.e-cancer.fr/Plan-cancer/Les-Plans-cancer-de-2003-a-2013/Le-Plan-cancer-2009-2013. Date last accessed: March 2016. Date last updated: September 30, 2012.
- Møller H, Riaz SP, Holmberg L, et al. High ling cancer surgical procedure volume is associated with shorter length of stay and lower risks of re-admissions and death: national cohort analysis in England. Eur J Cancer 2016;64:32-43. [Crossref] [PubMed]
- Wouters MW, Siesling S, Jansen-Landheer ML, et al. variation in treatment and outcome in patients with non-small cell lung cancer by region, hospital type and volume in the Netherlands. Eur J Surg Oncol 2010;36:S83-92. [Crossref] [PubMed]
- Bendzsak AM, Baxter NN, Darling GE, et al. Regionalization and outcome of lung cancer surgery in Ontario Canada. J Clin Oncol 2017;35:2772-80. [Crossref] [PubMed]
- Louie BE, Wilson JL, Kim S, et al. comparison of video-assisted thoracoscopic surgery and robotic approaches for clinical stage I and stage II non-small cell lung cancer using the society of thoracic surgeons database. Ann Thorac Surg 2016;102:917-24. [Crossref] [PubMed]
- Kozower BD, Stukenborg GJ. The relationship between hospital lung cancer resection volume and patient mortality risk. Ann Surg 2011;254:1032-7. [Crossref] [PubMed]
- Falcoz PE, Puyraveau M, Rivera C, et al. The impact of hospital and surgeon volume on the 30-day mortality of lung cancer surgery: a nation-based reappraisal. J Thorac Cardiovasc Surg 2014;148:841-8. [Crossref] [PubMed]
- Ross JS, Normand SLT, Wang Y, et al. Hospital volume and 30-day mortality for three common medical conditions. N Engl J Med 2010;362:1110-8. [Crossref] [PubMed]
- von Meyenfeldt EM, Gooiker GA, Van Gijn W, et al. the relationship between volume or surgeon speciality and outcome in the surgical treatment of lung cancer. A systematic review and meta-analysis. J Thorac Oncol 2012;7:1170-8. [Crossref] [PubMed]
- Lien YC, Huang MT, Lin HC. Association between surgeon and hospital volume and in-hospital fatalities after lung cancer resections: the experience of an Asian country. Ann Thorac Surg 2007;83:1837-43. [Crossref] [PubMed]
- Morgant MC, Pagès PB, Orsini B, et al. Time trends in surgery for lung cancer in France from 2005 to 2012:a nationwide study. Eur Respir J 2015;46:1131-9. [Crossref] [PubMed]
- Kozower BD, O’Brien SM, Kosinski AS, et al. The society of thoracic surgeons composite score for rating program performance for lobectomy for lung cancer. Ann Thorac Surg 2016;101:1379-86. [Crossref] [PubMed]
- Morche J, Mathes T, Pieper D. Relationship between surgeon and outcomes: a systematic review of systematic reviews. Syst Rev 2016;5:204. [Crossref] [PubMed]
- Hannan EL, Radzyner M, Rubin D, et al. The influence of hospital and surgeon volume on in-hospital mortality for colectomy, gastrectomy, and lung lobectomy in patients with cancer. Surgery 2002;131:6-15. [Crossref] [PubMed]