A modified acute respiratory distress syndrome prediction score: a multicenter cohort study in China
Introduction
Acute respiratory distress syndrome (ARDS) is associated with significant morbidity and mortality in patients in intensive care units (ICUs) (1). Since the initial description of ARDS in 1967 (2), a great progress has been made in understanding the pathophysiology of ARDS and advanced treatments for ARDS, such as low tidal volume ventilation (3), higher positive end-expiratory pressure (PEEP) (4), prone position ventilation (5), and early neuromuscular blockade in severe ARDS cases (6). However, the mortality of ARDS patients did not show a significant reduction over time, with a mortality of 40% reported in recent studies (1,7-9).
More recently, the focus has shifted from the treatment of ARDS to early identification and prevention of ARDS (10,11). Thus, it is important to recognize the ARDS risk factors early and eventually prevent its development. Some studies have shown that certain interventions could reduce the incidence of ARDS (12-14). However, the patients enrolled in these trials had various risk factors, which made it difficult to predict ARDS in clinical practice. Therefore, a prediction system for ARDS is needed to anticipate which patients are likely to develop ARDS.
A lung injury prediction score (LIPS) was identified by Trillo-Alvarez and his colleagues to recognize ARDS early (15). Later, they validated and refined the LIPS model through a multicenter cohort study (16). LIPS produced a high predicted value of 0.8 in alerting clinicians about the risk of ARDS (16). However, this study was focused on emergency department (ED) patients, and the risk factors for ARDS may vary in different patient populations. In addition, there are more than 20 variables in LIPS, which may make LIPS complicated to use in a clinical setting. Therefore, we performed a study to establish a modified ARDS prediction score (MAPS) to help clinicians in the early recognition of ARDS in patients who need to be admitted to the ICU.
Methods
Study design
This observational multicenter cohort study was performed in 13 tertiary hospitals in China. The protocol of this study was approved by the Institutional Ethics Committee of Zhongda Hospital (Approval Number 2012ZDllKY09.0) and registered on clinicaltrials.gov (Registration No. NCT01666834). All patients, or their next of kin, provided consent for the use of their medical records for this study.
There were retrospective derivation cohort patients and prospective validation cohort patients enrolled in our study. We collected the potential risk factors of ARDS in the retrospective cohort. Patients who developed ARDS in the first three days after ICU admission were placed into the ARDS group. After comparing the ARDS patients with the non-ARDS patients, we observed the risk factors of ARDS and built the MAPS. Finally, we prospectively enrolled patients to verify the accuracy of the MAPS.
Study patients
Critically ill patients who were admitted to a participating ICU from January 1 to January 31, 2012, were included in the retrospective derivation cohort and used to set up the prediction model of ARDS. Exclusion criteria for this cohort of patients included the following: patients with chronic respiratory failure, patients with an age of less than 18 years old, patients who were pregnant, patients who had died within 24 hours from ICU admission, patients who fulfilled the ARDS criteria at ICU admission and patients with a referral from another hospital to a participating ICU. The patients who had a lack of data on ARDS risk factors were also excluded.
Patients who admitted into an ICU from January 1 to January 10, 2013, were prospectively enrolled into the prospective validation cohort. The exclusion criteria of this cohort were the same as the derivation cohort.
Data collection
Trained investigators abstracted data from the electronic medical records of the patients. Baseline characteristics of patients, including sociodemographics, comorbidities and clinical variables, were collected. We collected the data at the time of 24 hours before ICU admission. We also analyzed the data at the time of ICU admission. The worst value during this period was recorded if there was more than one value.
All ARDS risk factors that had been collected in previous studies (15,16) were collected in this study. We also noted other potential ARDS risk factors, such as acute compartment syndrome (17), other immunosuppression conditions besides chemotherapy (18), acute kidney injury (AKI) (19), and blood transfusion (20). Other factors that may affect the ARDS diagnosis, such as cardiac dysfunction and pulmonary embolism, were also recorded.
Definition
ARDS diagnosis was performed according to the Berlin definition established in 2012 (1). The diagnostic criteria included the following: (I) within 1 week of a known clinical insult, or new or worsening respiratory symptoms; (II) chest imaging showing bilateral opacities that were not fully explained by effusions, lobar/lung collapse, or nodules; (III) respiratory failure not fully explained by cardiac failure or fluid overload; and (IV) a PaO2/FiO2 less than or equal to 300 mmHg. All patients diagnosed with ARDS were administered noninvasive or invasive positive pressure ventilation, with PEEP ≥5 cmH2O in our study. Two radiologists, who received the same training for assessment of bilateral infiltrates and diagnosis of ARDS, interpreted the chest radiograph. Any disagreements between the two radiologists were resolved by a discussion with an ICU attending physician, who also received the same training, until a consensus was achieved.
Comparison of MAPS with LIPS
We also recorded all ARDS risk factors and calculated the LIPS score in every included patient. These calculated scores were used to test the accuracy of LIPS in our patients. Afterward, we compared the performance of MAPS with LIPS
Statistical methods
Data were presented as a number (%) for categorical variables and as a mean (standard deviation) or median (interquartile range) for continuous variables. Regarding the basic characteristics of the patients, we assessed the differences in the categorical variables using χ2 test or Fisher’s exact test, and we assessed the differences in continuous variables with Student’s t-test or Mann-Whitney U test, as appropriate.
Univariate and multivariate logistic regression models were used, with the presence or absence of ARDS in patients as the dependent variable and risk factors of ARDS as the independent variables, to calculate the regression coefficients and to build the corresponding score systems. Forward model selection was used with a threshold P value of 0.05 in the multivariate logistic regression model. The model discrimination was then evaluated by the area under the curve (AUC) in the receiver operating characteristic (ROC) analysis. The corresponding 95% confidence interval (CI) and P value based on the Mann-Whitney U test were calculated as well. The optimum cutoff point that gives the best combination of sensitivity and specificity was determined based on the Youden index.
A P value of <0.05 was considered statistically significant. Statistical analyses were carried out using the SPSS 20.0 software (SPSS Inc., Chicago, IL, USA).
Results
There were 732 patients screened in the retrospective cohort. Among them, 253 patients were excluded because of various exclusion criteria and 479 patients were enrolled to build the prediction system (Figure 1A). A total of 222 patients were screened in the prospective cohort. After the exclusion of 24 patients, 198 patients were included to verify the system (Figure 1B). The characteristics of both the retrospective cohort and the prospective cohort are presented in Table 1.
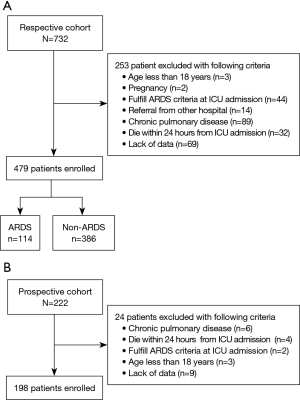
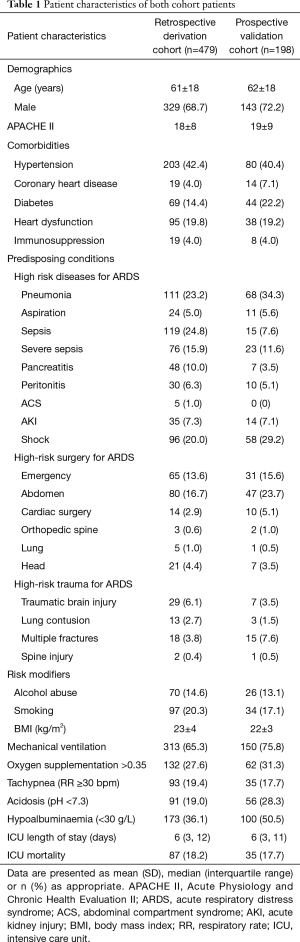
Full table
MAPS establishment of the retrospective derivation cohort
Of the 479 patients finally enrolled in the retrospective derivation cohort, 93 (19.4%) patients developed ARDS in the first three days after ICU admission. The basic characteristics of the ARDS and the non-ARDS patients in the derivation cohort are shown in Table 2. Compared with non-ARDS patients, ARDS patients had higher mortality (15.0% vs. 31.2%, P<0.001). The results of the univariate logistic regression analysis of risk factors of ARDS are given in Table 2.
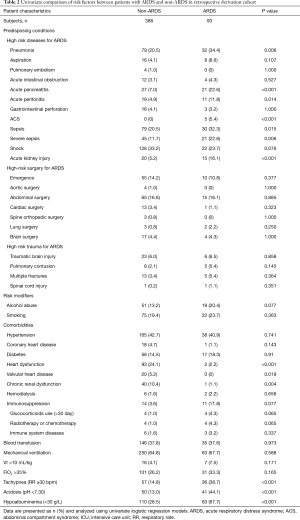
Full table
A multivariate logistic regression, the ARDS risk factors, and the corresponding points are also shown in Table 3, according to the odds ratio (OR) value of the multivariate logistic analysis. The prediction score discriminated well between patients who did and did not develop ARDS, with an AUC of 0.809 (95% CI, 0.758−0.859, P<0.001) (Figure 2A). At the cutoff point of 4.0 determined by ROC analysis, the sensitivity and specificity were 0.66 and 0.83, respectively, with a positive predicted value (PPV) of 0.48 and negative predicted value (NPV) of 0.91.
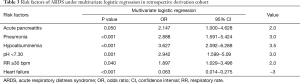
Full table
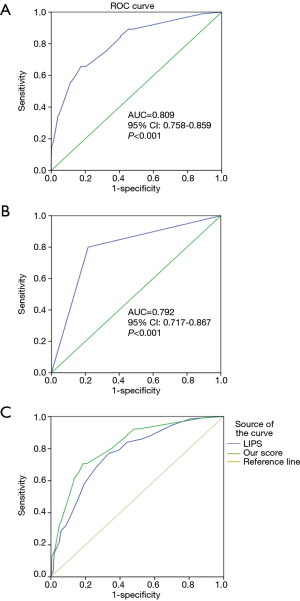
MAPS verification of the prospective validation cohort
One hundred and ninety-eight patients who did not fulfill ARDS criteria at the time of ICU admission were included in the prospective validation cohort. The performance of this prediction score was similar to the retrospective derivation cohort, with an AUC of 0.792 (95% CI, 0.717−0.867, P<0.001) (Figure 2B). At the cutoff point of 4.0, the sensitivity and specificity were 0.66 and 0.83, respectively, with a PPV of 0.48 and an NPV of 0.91.
Comparison of the performance of MAPS with LIPS
We tested the value of LIPS in predicting ARDS in all of the 677 patients enrolled in our study. The performance of LIPS is shown in Figure 2C with an AUC of 0.770 (95% CI, 0.728–0.812, P<0.001). Using the cutoff value of 4 from a previous study (16), the sensitivity and specificity were 0.72 and 0.59, respectively, with a PPV of 0.37 and an NPV of 0.87. In all included patients, MAPS showed a predicted value of an AUC of 0.818 (95% CI, 0.779–0.856, P<0.001). Compared to LIPS, MAPS showed a significantly higher value in predicting the development of ARDS in our patients (P=0.046).
Discussion
There is a limited strategy in improving the outcome of patients with ARDS, and the mortality of patients remains very high, especially after the development of ARDS into a severe condition (3,5,6,21). Thus, it becomes very important to shift from the treatment of ARDS to the prevention of ARDS, in terms of research priorities (10,22,23). The MAPS was set up based on the risk factors of ARDS and had a predictive value, with a sensitivity of 0.66 and a specificity of 0.83. The MAPS was verified by the prospective cohort patients. The present study indicated that MAPS could help us to predict the development of ARDS.
LIPS was identified in a previous study as being able to predict the development of ARDS (15) and was later validated through a multicenter cohort study (16). LIPS had a high value, with an AUC of 0.80, in predicting ARDS development (16). However, these two studies were focused on ED patients. The risk factors of ARDS may be different in various patient populations. We found that the predicted value of LIPS was significantly lower than our prediction score (Figure 2C) for our patients. Moreover, in a recent study, LIPS also showed a low predicted value, with an AUC of 0.70, in a similar patient population to our patient population (24). These results may indicate that LIPS may not be optimal to predict ARDS in patients who need to be admitted into the ICU. More importantly, the LIPS score is very complicated, with more than 20 variables involved in score prediction. Compared to LIPS, the MAPS we proposed, which gave a high predictive value, included only 7 risk factors, which makes it much easier for clinicians to use in daily practice.
We found some ARDS risk factors that were reported in previous studies, such as sepsis, pneumonia, and aspirations (18,25-30). However, some risk factors, such as alcohol abuse and smoking, were not associated with ARDS in our study. Gajic et al. showed that the risk of ARDS development in patients with these risk factors was not high (16). In addition, the risk factors may vary in different populations. In a recent study, which also focused on patients admitted to the ICU, there were no differences in proportions of alcohol abuse, smoking and diabetes between ARDS patients and non-ARDS patients (24).
The incidence of ARDS in our study was much higher than in a previous study (24). However, this high incidence was similar to a survey result in China (31). Because of this, the MAPS produced a higher positive predictive value (PPV) than LIPS (0.48 vs. 0.14). Levitt et al. compared 33 acute lung injury (ALI) patients with 67 non-ALI patients before mechanical ventilation to identify clinical predictors of progression to acute lung injury. The results showed a higher PPV of their prediction system because of a high proportion of ALI in their study population (32).
MAPS had a high predictive value; however, several issues are worthy of attention. First, we only included the patients who were admitted to the ICU. In addition, we included all patients during a short period in the winter season. Therefore, this result may not be suitable for other patients. Second, the criteria for admission to the ICU may vary among different hospitals. Therefore, MAPS may only be suitable for the ICUs in which the types of patients were similar to the types of patients in our study. Whether our score can be used in the ICUs that received different types of patients needs to be confirmed in a further study. Third, some patients may have evidence of lung injury even prior to positive ventilation (32). Therefore, MAPS cannot distinguish patients at high risk for developing ARDS from patients with early lung injury in our study. Fourth, since the risk factors may vary in different patient populations, our results may not extend to other countries. An international prospective validation cohort study is encouraged to validate our results.
We found that heart failure was a ‘protective factor’ for ARDS development in MAPS. This could be related to the difficulty of diagnosing ARDS in patients with heart failure because this was an observational study and most patients with heart failure lacked objective data of left ventricle (LV) filling. In our study, the heart failure definition was according to the clinical assessment and medical records of the patients. This is a limitation of our study. However, in our study, the diagnosis of ARDS was according to the Berlin definition. The chest radiograph was interpreted by two radiologists, who received the same training for assessment of bilateral infiltrates and ARDS independently. We thought this would improve the accuracy of our diagnosis. Indeed, a further study needs to be performed to clarify the role of heart failure in predicting ARDS development. In addition, patients with heart failure are usually combined with increased respiratory rate and hypoalbuminemia. Therefore, our results showed that when we use MAPS, especially for patients with increased respiratory rate and hypoalbuminemia, heart function should also be assessed.
The present study had several other limitations that needed to be expounded. First, even if this was a multicenter study, the sample was relatively small. Second, we excluded all the patients with missing data, even if most of the ARDS risk factors were recorded in our electronic database. There were 69 and 9 patients in the derivation cohort and validation cohort, respectively, who were excluded because of missing data. This exclusion may also induce a biased result, which needs a further study to confirm. Third, the mortality of patients included in our study was approximately 18%. We believe that some patients may have died before they had a chance to fulfill the criteria for ARDS. We did not analyze the competing risk of death in our study and this may also induce a biased result. A further study including a much larger sample size and different types of patients will be needed to improve the predictive value.
Conclusions
In the present study, we developed and validated MAPS to screen for the risk factors of ARDS at ICU admission. Using MAPS may help the clinician to recognize patients who will develop ARDS earlier.
Acknowledgments
Funding: This study was sponsored by a grant from Jiangsu Provincial Key Medical Discipline (grant number: ZDXKA2016025); grants (81571847 and 81601723) from the National Natural Science Foundation of China; a grant (YBJJ1741) from Scientific Research Foundation of Graduate School of Southeast University and a grant (QNRC2016806) from Jiangsu Provincial Medical Youth Talent. We thank all patients for participation in the study, and all the doctors who participated in this study, for their helpful and continuous support.
Footnote
Conflicts of Interest: The authors have no conflicts of interest to declare.
Ethical Statement: The protocol of this study was approved by the Institutional Ethics Committee of Zhongda Hospital (Approval Number 2012ZDllKY09.0)
References
- ARDS Definition Task Force, Ranieri VM, Rubenfeld GD, et al. Acute respiratory distress syndrome: the Berlin Definition. JAMA 2012;307:2526-33. [PubMed]
- Ashbaugh DG, Bigelow DB, Petty TL, et al. Acute respiratory distress in adults. Lancet 1967;2:319-23. [Crossref] [PubMed]
- Acute Respiratory Distress Syndrome Network, Brower RG, Matthay MA, et al. Ventilation with lower tidal volumes as compared with traditional tidal volumes for acute lung injury and the acute respiratory distress syndrome. The Acute Respiratory Distress Syndrome Network. N Engl J Med 2000;342:1301-8. [Crossref] [PubMed]
- Briel M, Meade M, Mercat A, et al. Higher vs lower positive end-expiratory pressure in patients with acute lung injury and acute respiratory distress syndrome: systematic review and meta-analysis. JAMA 2010;303:865-73. [Crossref] [PubMed]
- Guérin C, Reignier J, Richard JC, et al. Prone positioning in severe acute respiratory distress syndrome. N Engl J Med 2013;368:2159-68. [Crossref] [PubMed]
- Papazian L, Forel JM, Gacouin A, et al. Neuromuscular blockers in early acute respiratory distress syndrome. N Engl J Med 2010;363:1107-16. [Crossref] [PubMed]
- Bellani G, Laffey JG, Pham T, et al. Epidemiology, Patterns of Care, and Mortality for Patients With Acute Respiratory Distress Syndrome in Intensive Care Units in 50 Countries. JAMA 2016;315:788-800. [Crossref] [PubMed]
- Tonelli AR, Zein J, Adams J, et al. Effects of interventions on survival in acute respiratory distress syndrome: an umbrella review of 159 published randomized trials and 29 meta-analyses. Intensive Care Med 2014;40:769-87. [Crossref] [PubMed]
- Villar J, Kacmarek RM, Guerin C. Clinical trials in patients with the acute respiratory distress syndrome: burn after reading. Intensive Care Med 2014;40:900-2. [Crossref] [PubMed]
- Gong MN, Thompson BT. Acute respiratory distress syndrome: shifting the emphasis from treatment to prevention. Curr Opin Crit Care 2016;22:21-37. [Crossref] [PubMed]
- Nieman GF, Gatto LA, Bates JH, et al. Mechanical Ventilation as a Therapeutic Tool to Reduce ARDS Incidence. Chest 2015;148:1396-404. [Crossref] [PubMed]
- Yilmaz M, Keegan MT, Iscimen R, et al. Toward the prevention of acute lung injury: protocol-guided limitation of large tidal volume ventilation and inappropriate transfusion. Crit Care Med 2007;35:1660-6; quiz 1667.
- Licker M, Diaper J, Villiger Y, et al. Impact of intraoperative lung-protective interventions in patients undergoing lung cancer surgery. Crit Care 2009;13:R41. [Crossref] [PubMed]
- Futier E, Constantin JM, Paugam-Burtz C, et al. A trial of intraoperative low-tidal-volume ventilation in abdominal surgery. N Engl J Med 2013;369:428-37. [Crossref] [PubMed]
- Trillo-Alvarez C, Cartin-Ceba R, Kor DJ, et al. Acute lung injury prediction score: derivation and validation in a population-based sample. Eur Respir J 2011;37:604-9. [Crossref] [PubMed]
- Gajic O, Dabbagh O, Park PK, et al. Early identification of patients at risk of acute lung injury: evaluation of lung injury prediction score in a multicenter cohort study. Am J Respir Crit Care Med 2011;183:462-70. [Crossref] [PubMed]
- Malbrain ML, Chiumello D, Pelosi P, et al. Incidence and prognosis of intraabdominal hypertension in a mixed population of critically ill patients: a multiple-center epidemiological study. Crit Care Med 2005;33:315-22. [Crossref] [PubMed]
- Iscimen R, Cartin-Ceba R, Yilmaz M, et al. Risk factors for the development of acute lung injury in patients with septic shock: an observational cohort study. Crit Care Med 2008;36:1518-22. [Crossref] [PubMed]
- Darmon M, Clec'h C, Adrie C, et al. Acute respiratory distress syndrome and risk of AKI among critically ill patients. Clin J Am Soc Nephrol 2014;9:1347-53. [Crossref] [PubMed]
- Gajic O, Rana R, Winters JL, et al. Transfusion-related acute lung injury in the critically ill: prospective nested case-control study. Am J Respir Crit Care Med 2007;176:886-91. [Crossref] [PubMed]
- Fanelli V, Vlachou A, Ghannadian S, et al. Acute respiratory distress syndrome: new definition, current and future therapeutic options. J Thorac Dis 2013;5:326-34. [PubMed]
- Brower RG, Antonelli M. What's new in ARDS: can we prevent it?. Intensive Care Med 2016;42:772-4. [Crossref] [PubMed]
- Rittayamai N, Brochard L. What's new in ARDS (clinical studies). Intensive Care Med 2014;40:1731-3. [Crossref] [PubMed]
- Soto GJ, Kor DJ, Park PK, et al. Lung Injury Prediction Score in Hospitalized Patients at Risk of Acute Respiratory Distress Syndrome. Crit Care Med 2016;44:2182-91. [Crossref] [PubMed]
- Ferguson ND, Frutos-Vivar F, Esteban A, et al. Clinical risk conditions for acute lung injury in the intensive care unit and hospital ward: a prospective observational study. Crit Care 2007;11:R96. [Crossref] [PubMed]
- Mangialardi RJ, Martin GS, Bernard GR, et al. Hypoproteinemia predicts acute respiratory distress syndrome development, weight gain, and death in patients with sepsis. Ibuprofen in Sepsis Study Group. Crit Care Med 2000;28:3137-45. [Crossref] [PubMed]
- Fowler AA, Hamman RF, Good JT, et al. Adult respiratory distress syndrome: risk with common predispositions. Ann Intern Med 1983;98:593-7. [Crossref] [PubMed]
- Hudson LD, Milberg JA, Anardi D, et al. Clinical risks for development of the acute respiratory distress syndrome. Am J Respir Crit Care Med 1995;151:293-301. [Crossref] [PubMed]
- Pepe PE, Potkin RT, Reus DH, et al. Clinical predictors of the adult respiratory distress syndrome. Am J Surg 1982;144:124-30. [Crossref] [PubMed]
- Fernández-Pérez ER, Sprung J, Afessa B, et al. Intraoperative ventilator settings and acute lung injury after elective surgery: a nested case control study. Thorax 2009;64:121-7. [Crossref] [PubMed]
- Du B, An Y, Kang Y, et al. Characteristics of critically ill patients in ICUs in mainland China. Crit Care Med 2013;41:84-92. [Crossref] [PubMed]
- Levitt JE, Bedi H, Calfee CS, et al. Identification of early acute lung injury at initial evaluation in an acute care setting prior to the onset of respiratory failure. Chest 2009;135:936-43. [Crossref] [PubMed]