Nomogram to predict postoperative PR in patients undergoing CT-guided transthoracic lung biopsy
Introduction
Pleural reaction (PR) refers to a series of presentations, including a continuous cough, dizziness, dyspnea, chest pain, sweating, transient hypotension, and even syncope (1). PR is a common complication of pleural puncture, transthoracic needle biopsy, or thoracotomy tube (1,2). However, when compared to objective complications, such as pneumothorax and hemoptysis, PR is a more subjective complication that is often underestimated in computed tomography-guided transthoracic needle biopsy (CT-TNB) (1).
With the widespread use of low-dose CT for lung cancer screening and diagnosis (3,4), the incidence of pulmonary lesions, including asymptomatic pulmonary nodules and masses has significantly increased (5). In general, for pulmonary lesions larger than 10 mm or smaller lesions with a high growth rate, clinicians will recommend further diagnostic procedures, such as transbronchoscopic lung biopsy, CT-TNB, or surgery (3,6). Among these, CT-TNB is the preferred biopsy method because of its minimal invasiveness, high diagnostic accuracy, and relatively low costs (6-8). CT-TNB-induced complications, including pneumothorax, hemoptysis, and intrapulmonary have been highlighted in several of studies (6,7,9). However, although the incidence is not low, PR following CT-TNB has received insufficient attention (1).
Nowadays, CT-TNB is commonly performed in an outpatient setting. The most important issue concerning outpatient management is not the occurrence of PR per se, but some PR requires immediate treatment. Therefore, it is essential to predict the occurrence of post-CT-TNB PR. To the best of our knowledge, no reported model for the prediction of post-CT-TNB PR is available to date. We hypothesized that variables of patient demographics, lesion features, and biopsy parameters may potentially predict PR. The purpose of this study was to establish a predictive model based on valuable predictors of post-CT-TNB PR.
Methods
Study population
In this study, we performed a retrospective cohort study, including a total of 436 consecutive patients who underwent CT-TNB at a tertiary hospital between June 2016 and December 2017. The study was approved by institutional ethics committee of Jinhua Hospital of Zhejiang University (No. 2018001008). Patient information was handled anonymously and therefore signed informed consent was waived. In patients, PR was defined as the occurrence of one or more presentations following CT-TNB, including puncture site pain [based on a scale of 0 to 10 (0= none, 10= severe)] (1), profuse sweating, dyspnea, continuous cough, dizziness, and hypotension [systolic blood pressure (SBP) decreases ≥20 mmHg, or diastolic blood pressure (DBP) decreases ≥10 mmHg].
Clinical characteristics
Patient clinical information and laboratory tests were retrospectively reviewed using our electronic medical record system. From each patient, the following variables were collected: age, gender, SBP, DBP, heart rate, pulse oximetry saturation (on room air), and coexisting chronic obstructive pulmonary disease (COPD) (yes or no), diagnosis of the lung lesion, lesion location classification I (left or right lung), lesion location classification II (hilum, upper lung, middle lung or lower lung), lesion diameter, lesion characteristics (ground-glass, solid, or cavitary), lesion differentiation (low, moderate, or high), lesion burr (yes or no), the closest distance between the lesion and a vessel (<10 mm, or ≥10 mm), CT value of lesion, puncture position (supine, prone or lateral), puncture distance (defined as the length between the center of the lung lesion and the pleura), procedure duration, biopsy times, and PR following procedure (yes or no). Laboratory tests were performed for blood glucose, C-reactive protein, prothrombin time, squamous cell carcinoma antigen, carcinoembryonic antigen, cytokerantin-19-fragment (CYFRA21-1), D-dimer, platelet counts, and triglyceride levels.
CT-TNB procedure
A clinician experienced in radiology and pneumology performed all biopsies. A coaxial 18-gauge needle (Lot Number, REXK0682; Bard Peripheral Vascular, Inc., Tempe, AZ) was used for all biopsies, and 2% lidocaine was used for local infiltration anesthesia prior to surgery. In general, two biopsies were taken and occasionally additional biopsies were obtained (8,10).
Patients were requested to maintain the supine position for at least 6 hours following CT-TNB, in which patient's symptoms were recorded. Generally, patients with mild PR spontaneously regained normally, and drug treatment (epinephrine or/and rehydration) was only administered when PR was severe.
Statistical analysis
We used descriptive statistics to summarize baseline characteristics. For continuous variables, the median (25–75% interquartile) was calculated, and categorical variables were displayed as numbers with percentages. Between-group comparisons, unpaired t-tests (normal distribution) or Mann-Whitney U test (non-normal distribution), Pearson Chi-squared tests or the Fisher’s exact test were used, as appropriate. Variables with a P value of less than 0.05 in the univariate analysis were included in the multiple logistic regression. To account for missing data, multiple imputation was used (11). The least absolute shrinkage and selection operator (LASSO) regression method was employed to determine value predictors from collected variables. The logistic regression analysis was used to establish the predictive model for post-CT-TNB PR, and a nomogram was constructed based on the model. The area under the curve (AUC) was calculated to assess the discrimination capacity of the model, and bootstrapping with 1000 iterations was performed for internal validation. Calibration was assessed by the unreliability test and calibration curve. Decision curve analysis (DCA) was performed to assess the clinical usefulness of the model by quantifying the net benefits at different threshold probabilities (12). R version 3.5.1 (https://www.r-project.org) with R packages (glmnet, rms) was used for statistical analyses. P<0.05 was considered statistically significant.
Results
Patient’s clinical characteristics, lesion features, and biopsy parameters are shown in Table 1. Of the 436 patients, 34 (7.8%, 95% CI, 5.3–10.3%) patients experienced PR following CT-TNB, and 2 (5.9% of the PR) cases required treatment. With regard to the time window between CT-TNB and occurrence of the PR, 79.4% (27/34) patients experienced PR within half an hour, 11.8% (4/34) cases at 0.5–2 hours, and the remaining 3 cases at 2–6 hours post-CT-TNB. Univariable analysis showed that post-CT-TNB PR more frequently occurred in female patients, younger patients, patients with no coexisting COPD, higher preoperative pulse oxygen saturation, lower preoperative blood glucose levels, smaller lesion diameter, more puncture times, and longer procedure duration; while less frequent in the supine position, and in lesions in the hilus and the middle lung. However, only gender and age were independently associated with post-CT-TNB PR as assessed by multiple logistic regression (Table 2).
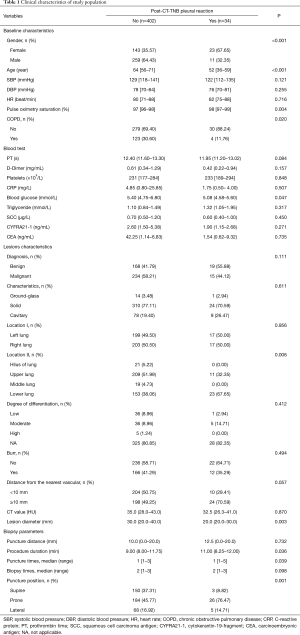
Full table
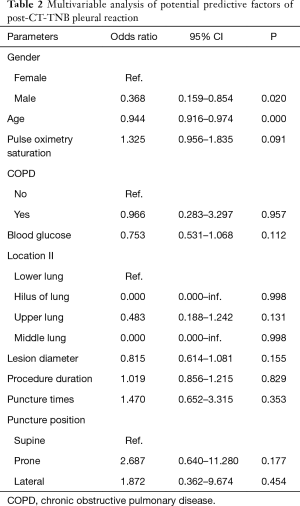
Full table
Based on non-zero coefficients in the LASSO regression analysis (Figure 1), 30 variables were reduced to the 4 most potential predictors (gender, age, lesion location, and puncture position). We constructed a risk prediction model for CT-TNB-induced PR based on the aforementioned 4 predictors. As shown in Figure 2, the AUC for the predictive model was 0.840 (95% CI, 0.767–0.913), whereas the AUC for the internal validation was 0.841 (95% CI, 0.769–0.912). The optimal cut-off value of the predictive model was −2.41, which was based on the value of the maximum Youden index. The accuracy, sensitivity, specificity, positive predictive value, and negative predictive value were 76.8%, 79.4%, 76.6%, 22.3%, and 97.8%, respectively.
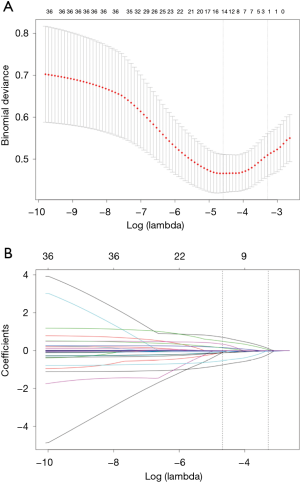
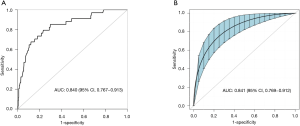
In this study, we established a nomogram that was based on the predictive model to provide clinicians with a quantitative tool to predict the individual probability of post-CT-TNB PR (Figure 3). The calibration curve of the model for the probability of post-CT-TNB PR showed good agreement between prediction and observation of the model (Figure 4). The unreliability test yielded non-significant statistics (Emax =0.083, Eavg =0.013, P=0.966), which suggested that the model was a perfect fit.
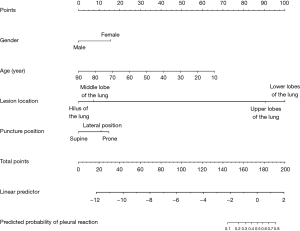
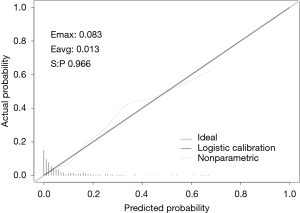
The decision curve for the model is presented in Figure 5. The DCA showed that when the threshold probability of an individual was between 2–48%, application of this model to predict post-CT-TNB PR proved to be more beneficial than either the treat-all or treat-none strategies.
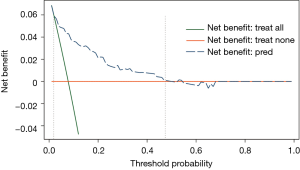
Discussion
Being able to anticipate post-CT-TNB PR may improve postoperative patient management. The reported predictive model is the first initiative of its kind to predict the occurrence of post-CT-TNB PR by combining patient’s gender, age, lesion location, and puncture position, which were selected by LASSO regression and readily available. Furthermore, we developed a nomogram based on the aforementioned predictors for convenient prediction post-CT-TNB PR. The model showed good discrimination with an AUC of 0.840 (95% CI, 0.767–0.913), satisfactory calibration, and clinical usefulness.
Currently, CT-TNB is widely used for the diagnosis of pulmonary diseases (7,13). A number of studies focused on post-CT-TNB complications, including pneumothorax and hemoptysis (6,9). However, only few studies focused on PR in CT-TNB, thereby underestimating PR occurrence following CT-TNB (1). Reportedly, the incidence of PR in thoracocentesis is 5–46% (1,14-16). Mild PR causes patient discomfort whereas severe PR can lead to shock, and in some cases, may be life-threatening (17).
The underlying mechanism of PR is still unclear. Factors that may be associated with PR, include enhanced vagus reflex, patient’s constitutional weakness or low pain threshold, preoperative anxiety, inexperienced operator, and inadequate pleural anesthesia (1). In the present study, we used an 18-gauge coaxial needle for transthoracic puncture, leading to an incidence of post-CT-TNB of 7.8%. Our study indicated a trend that PR occurred more frequently in female and younger patients, whose vagus reflex may be more enhanced following CT-TNB. No statistically significant difference was observed in the number of biopsies between the post-CT-TNB PR group and the non-PR group as assessed by univariable analysis. In this study cohort, 94.7% (413/436) patients received two biopsies. In several studies, it has been demonstrated that for most lesions, one or two biopsies can obtain sufficient specimen for pathological examination, yielding a stable and satisfactory diagnostic accuracy (10,18). When additional laboratory testing was needed (such as immunohistochemistry and analysis of tumor-markers) or when the first two biopsies failed, additional biopsies were considered. However, each biopsy can induce complications (such as pneumothorax and hemoptysis) and therefore unnecessary additional biopsies should be avoided (8,19,20).
Not just inpatients, but also many outpatients commonly receive CT-TNB. The most important issue is not the occurrence of PR per se, but the management of these outpatients. Therefore, it is necessary to accurately predict the probability of PR post CT-TNB. In the present study, we established a nomogram based on four predictors (gender, age, lesion location, and puncture position) filtered by LASSO regression analysis. Regression shrinkage and variable selection based on the LASSO approach was first reported by Robert in 1996 (21), and this approach was considered to surpass the method of choosing predictors based on the strength of their univariable association with outcome, especially when many variables were present (21-23). In addition, all four predictors were readily accessible clinically.
Our prediction model showed both good discrimination ability and calibration. To evaluate its clinical usefulness, we performed DCA to assess whether the decisions made based on this predictive model would improve patient outcomes. This novel method was based on threshold probabilities to gain insight into clinical outcomes of a certain decision, and to weigh the net benefit (12,24,25). The decision curve based on this model was satisfactory and revealed that when the threshold probability of an individual was between 2–48%, applying our model to predict post-CT-TNB PR was more advantageous when compared to either treat-all or treat-none strategies.
There are several limitations to our study. First, it is a single-center and retrospective study therefore additional external verification is needed to confirm the usefulness of the proposed nomogram. Second, we did not further subdivide PR, and therefore this nomogram can only be used to predict the occurrence of post-CT-TNB PR, but not to distinguish PR severity.
Conclusions
Here, we established a nomogram for the prediction of post-CT-TNB PR based on 4 readily available clinical predictors, including gender, age, lesion location, and puncture position. The proposed predictive model showed sufficient discrimination ability and calibration, and demonstrated potential for clinical applications. Therefore, it may be of great value to facilitate the individualized prediction of post-CT-TNB PR to reduce the risk of PR-induced harm to patients.
Acknowledgements
Funding: This study was supported by the Medical and Health Science and Technology Plan Project of Zhejiang Province (No. 2018RC079), the key project of Jinhua Municipal Science and Technology Research Program (No. 20163011) and the Youth Research Fund of Jinhua Hospital of Zhejiang University (No. JY2017205).
Footnote
Conflicts of Interest: The authors have no conflicts of interest to declare.
Ethical Statement: The study was approved by institutional ethics committee of Jinhua Hospital of Zhejiang University (No. 2018001008). The data were anonymous, and the requirement for informed consent was therefore waived.
References
- Collins TR, Sahn SA. Thoracocentesis. Clinical value, complications, technical problems, and patient experience. Chest 1987;91:817-22. [Crossref] [PubMed]
- Gilsanz V, Cleveland RH. Pleural reaction to thoracotomy tube. Chest 1978;74:167-9. [Crossref] [PubMed]
- Kauczor H-U, Bonomo L, Gaga M, et al. ESR/ERS white paper on lung cancer screening. Eur Respir J 2015;46:28-39. [Crossref] [PubMed]
- Moyer VA. Screening for lung cancer: U.S. Preventive services task force recommendation statement. Ann Intern Med 2014;160:330-8. [PubMed]
- National Lung Screening Trial Research Team, Church TR, Black WC, et al. Results of initial low-dose computed tomographic screening for lung cancer. N Engl J Med 2013;368:1980-91. [Crossref] [PubMed]
- Han Y, Kim HJ, Kong KA, et al. Diagnosis of small pulmonary lesions by transbronchial lung biopsy with radial endobronchial ultrasound and virtual bronchoscopic navigation versus CT-guided transthoracic needle biopsy: A systematic review and meta-analysis. PLoS One 2018;13:e0191590. [Crossref] [PubMed]
- Heerink WJ, de Bock GH, de Jonge GJ, et al. Complication rates of CT-guided transthoracic lung biopsy: meta-analysis. Eur Radiol 2017;27:138-48. [Crossref] [PubMed]
- Boskovic T, Stanic J, Pena-Karan S, et al. Pneumothorax after transthoracic needle biopsy of lung lesions under CT guidance. J Thorac Dis 2014;6:S99-S107. [PubMed]
- Tomiyama N, Yasuhara Y, Nakajima Y, et al. CT-guided needle biopsy of lung lesions: a survey of severe complication based on 9783 biopsies in Japan. Eur J Radiol 2006;59:60-4. [Crossref] [PubMed]
- Dong K, Wen G, Jiang T, et al. Clinical application of percutaneous puncture biopsy guided by fused and 3D-reconstructed PET/CT images. Curr Med Imaging Rev 2018;14:1006-10. [Crossref]
- Farrar D, Fairley L, Santorelli G, et al. Association between hyperglycaemia and adverse perinatal outcomes in south Asian and white British women: analysis of data from the Born in Bradford cohort. Lancet Diabetes Endocrinol 2015;3:795-804. [Crossref] [PubMed]
- Vickers AJ, Cronin AM, Elkin EB, et al. Extensions to decision curve analysis, a novel method for evaluating diagnostic tests, prediction models and molecular markers. BMC Med Inform Decis Mak 2008;8:53. [Crossref] [PubMed]
- Manhire A, Charig M, Clelland C, et al. BTS. Guidelines for radiologically guided lung biopsy. Thorax 2003;58:920-36. [Crossref] [PubMed]
- Cao W, Wang Y, Zhou N, et al. Efficacy of ultrasoundguided thoracentesis catheter drainage for pleural effusion. Oncol Lett 2016;12:4445-8. [Crossref] [PubMed]
- Chakrabarti B, Earis JE, Pandey R, et al. Risk assessment of pneumothorax and pulmonary haemorrhage complicating percutaneous co-axial cutting needle lung biopsy. Respir Med 2009;103:449-55. [Crossref] [PubMed]
- Yamagami T, Nakamura T, Iida S, et al. Management of pneumothorax after percutaneous CT-guided lung biopsy. Chest 2002;121:1159-64. [Crossref] [PubMed]
- Cantey EP, Walter JM, Corbridge T, et al. Complications of thoracentesis: incidence, risk factors, and strategies for prevention. Curr Opin Pulm Med 2016;22:378-85. [Crossref] [PubMed]
- Yang W, Sun W, Li Q, et al. Diagnostic accuracy of CT-guided transthoracic needle biopsy for solitary pulmonary nodules. PLoS One 2015;10:e0131373. [Crossref] [PubMed]
- Ko JP, Shepard JO, Drucker EA, et al. Factors influencing pneumothorax rate at lung biopsy: are dwell time and angle of pleural puncture contributing factors? Radiology 2001;218:491-6. [Crossref] [PubMed]
- Yankelevitz DF, Vazquez M, Henschke CI. Special techniques in transthoracic needle biopsy of pulmonary nodules. Radiol Clin North Am 2000;38:267-79. [Crossref] [PubMed]
- Tibshirani R. Regression shrinkage and selection via the LASSO. J R Stat Soc Series B Stat Methodol 1996;58:267-88.
- Huang YQ, Liang CH, He L, et al. Development and validation of a radiomics nomogram for preoperative prediction of lymph node metastasis in colorectal cancer. J Clin Oncol 2016;34:2157-64. [Crossref] [PubMed]
- Tibshirani R. The lasso method for variable selection in the Cox model. Stat Med 1997;16:385-95. [Crossref] [PubMed]
- Vickers AJ, Elkin EB. Decision curve analysis: a novel method for evaluating prediction models. Med Decis Making 2006;26:565-74. [Crossref] [PubMed]
- Balachandran VP, Gonen M, Smith JJ, et al. Smith, R.P. Dematteo, Nomograms in oncology: More than meets the eye. Lancet Oncol 2015;16:e173-80. [Crossref] [PubMed]