Simple pre-procedure risk stratification tool for contrast-induced nephropathy
Introduction
Contrast-induced nephropathy (CIN) has been previously shown to be associated with increased cardiovascular events and mortality following exposure of contrast medium during coronary angiography (CAG) (1,2). Current guidelines recommend intravenous hydration, use of low- or iso-osmolar contrast media, and reduced volume of contrast agents, as prevention strategies for CIN (3,4). Meanwhile, pre-procedural identification of patients at risk for CIN would be of immense value in targeting prophylactic therapy to those at high risk (5).
Studies have reported several models of prediction for CIN following CAG (6,7). However, simple pre-procedural risk models validated by the downstream effects of decision making and patient outcomes have not been explored for clinical guidance (7). Therefore, in the present study, we intended to develop a simple pre-procedure risk model of CIN.
Methods
Study population
According to our institution’s protocol, we enrolled consecutive patients undergoing CAG or PCI between January 2010 and October 2012. The details of the inclusion and exclusion criteria were described previously (8,9). The Guangdong General Hospital Ethics Committee approved the study, and written informed consent was obtained from all patients. Finally, 3,469 patients were included in the retrospectively analysis.
Definitions and follow-up
CIN0.5 was defined as an elevated serum creatinine (SCr) level >0.5 mg/dL of baseline SCr level within 72 hours after CAG (10). The definitions of anemia, hypotension, and congestive heart failure (CHF) were the same as those in Mehran’s study (11).
Follow-up major adverse clinical events (MACEs) were carefully monitored and recorded by trained nurses through office visits and telephone interviews at 1, 6, 12, 24, 36, and 48 months after CAG. MACEs included death, re-nonfatal acute myocardial infarction (re-AMI), target vessel revascularization (TVR), CI-AKI requiring renal replacement therapy (RRT), stroke, and re-hospitalization after index hospitalization.
Model development and model validation
A total of 3,469 eligible patients from the entire database were randomly established into a development dataset (n=2,313) and a validation dataset (n=1,156) in a 2:1 manner. After identifying the associations of clinical baseline and key procedural characteristics with CIN, independent predictors of CIN were analyzed in the development dataset. Risk factors that were significant in the univariate analysis were available for selection in the final mode. Age >75 years, hypotension, acute myocardial infarction (AMI), SCr ≥1.5 mg/dL, CHF were identified as independent pre-procedural predictors of CIN. The risk score was established subsequently and tested in the validation dataset. Model discrimination and its predictive performance for the occurrence of CIN and its short- and long-term outcomes were assessed via comparisons with Mehran’s score and ACEF score (12).
Statistical analysis
The association between CIN and variables in the development group was evaluated using univariable logistic regression analysis. A stepwise multivariable logistic regression analysis was then performed to identify independent predictors of CIN. For all logistic regression analysis models, odds ratios (OR) with 95% confidence intervals (95% CI) were calculated. The variables that were independently and significantly associated with CIN in the final multivariable model were assigned a weighted integer coefficient value based upon its beta value. Therefore, a risk score model was constructed where the final risk score for each patient represented the sum of integer coefficients. The risk score was tested in the validation dataset. Model discrimination was assessed by the goodness-of-fit Hosmer-Lemeshow statistic, and its predictive performance was assessed with the c-statistic. Continuous variables were presented as mean ± standard deviation (SD), and as percentages for categorical data. The differences between groups for continuous variables were analyzed by using independent Student’s t-test. Comparisons between categorical variables were performed with the Pearson χ2 test or Fisher’s exact test. The tendency test of the risk score was analyzed by the Cochran-Armitage trend test. Survival curves were generated with the Kaplan-Meier method, and the differences between groups were assessed by log-rank test. A P<0.05 (2-sided) was considered to indicate statistical significance. The IBM PASW-SPSS Statistics 22.0 statistical software package (SPSS Inc., Chicago, IL, USA) was used for all calculations with an exception of area under the curve (AUC) comparison for which MedCalc 11.4 Statistical Software (MedCalc Software, Mariakerke, Belgium) was used.
Results
Baseline characteristics
The cumulative incidence of CIN was 115 (3.32%) in the whole study population (n=3,469), with 74 (3.20%) occurring in the development dataset. Overall (Table 1), the mean age was 62.9±11.1 years old, and there were 807 (23.3%) females. The mean baseline SCr level was 1.04±0.48 mg/dL, whereas 291 (8.4%) of patients presented creatinine levels≥1.5 mg/dL. Suffering hypotension (20.0% vs. 2.0%, P<0.001), anemia, AMI, and CHF also showed significant difference between the CIN group and non-CIN group. Laboratory measurements such as B-type natriuretic peptide (BNP), serum urea nitrogen, and uric acid were remarkably higher in the CIN group when compared with the non-CIN group, along with procedural characteristics contrast volume and hydration volume. There were no intergroup differences in terms of gender and a medical history of previous myocardial infarction (MI), diabetes mellitus (DM), or hyperlipidemia. In addition, the comparison of the baseline clinical and procedural characteristics in the development dataset defined by CIN0.5 is listed in Table 2.
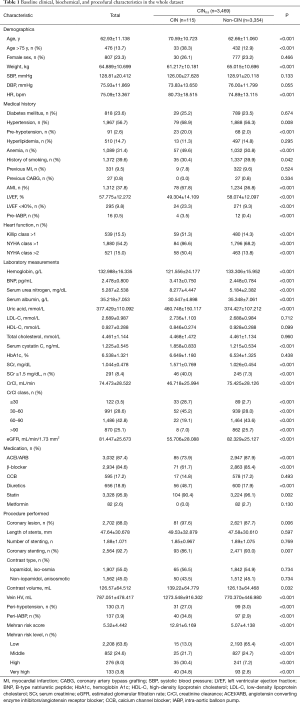
Full table
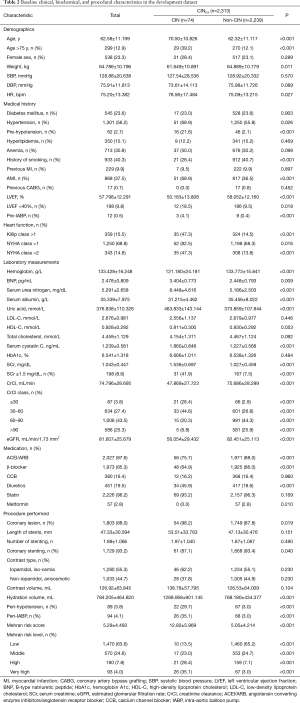
Full table
Univariable logistic regression models and multivariate model
Univariable logistic regression models associated with CIN are shown in Table 3. A total of 12 pre-procedural variables were analyzed in the development of CIN. The significant correlates included demographics (age >75 years, weight, and heart rate) and medical history such as hypertension, hypotension, AMI, anemia, CHF, use of IABP as well as history of smoking, and laboratory findings for SCr.
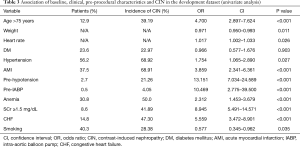
Full table
The multivariate model of CIN predictors was obtained from all 2,313 patients in the development dataset with no missing co-variate values. Age >75 years, hypotension, AMI, SCr ≥1.5 mg/dL, and CHF were identified as independent predictors and demonstrated to be markedly associated with CIN (Table 4). The Hosmer-Lemeshow statistic for the multivariable model did not suggest a lack of fit (χ2=4.913, P=0.178).
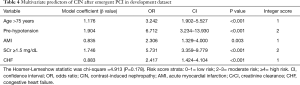
Full table
Development of risk score
The incidence of CIN by risk score assignment is depicted in Figure 1, with significant trends across increasing score values for predicting CIN (Cochran Armitage chi-square, P<0.001). Based on the obtained frequencies of CIN in relation to different risk scores, 2,313 patients in the development dataset were further categorized into 3 groups: low risk (n=17, 0.9%), moderate risk (n=31, 8.1%), and high risk (n=26, 27.1%), while corresponding to risk scores of <2, 2 to 3, and ≥4, respectively (Figure 2).
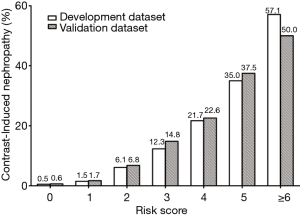
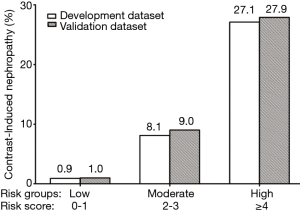
Validation and comparison of risk score
CIN occurred in 41 (3.55%) of the 1,156 patients in the validation dataset. The rates of CIN in the validation set presented in parallel to those in the development set inside each of the 3 risk groups (Figure 2). The developed CIN model demonstrated similar discriminative power (Figure 3) with respect to the incidence of CIN in the validation population (c-statistic =0.829) when compared with Mehran and ACEF score.
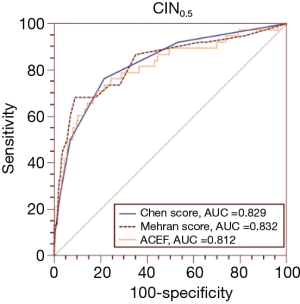
The mean follow-up time was 2.31±0.93 years (median, 2.18 years; interquartile range, 1.64–3.04 years). The impacts of short- and long-term outcomes in the development and validation datasets according to risk strata are shown in Figure 4, and the comparison of the risk scores on outcomes was also conducted (Table 5). The present risk score model as assessed in the validation population by the c-statistic demonstrated an even higher predictive accuracy on outcomes compared to Mehran, ACEF risk scores (Figure 5), and the calibration (Figure 6). The predictive accuracy for short- and long-term outcomes in the 3 risk scores shows no significant P value, except for in-hospital MACEs (Chen score vs. Mehran score, P<0.05). All risk scores performed with excellent discriminative power and with no significant difference for predicting mortality (c statistics: 0.757 to 0.937), especially in-hospital mortality (c statistics: 0.866 to 0.937). In addition, all 3 risk scores also had good predictive accuracy for long-term MACEs with c-statistics ranging from 0.696 (Mehran) to 0.759 (ACEF).
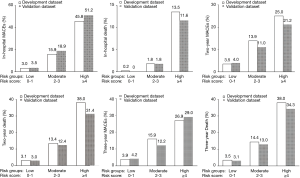
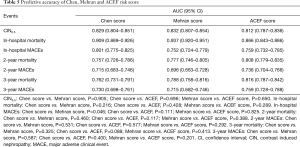
Full table
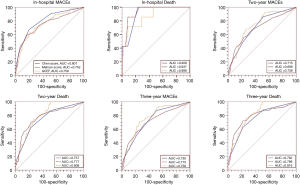
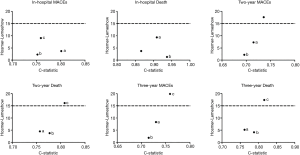
As shown in Figure 7, patients with high risk score (≥4) presented with a higher rate of all-cause death than patients with a moderate [2–3] and low risk score (<2) according to log-rank analysis. Significant increases in follow-up mortality rate were observed with increment of risk score (χ2=89.23, P<0.001).
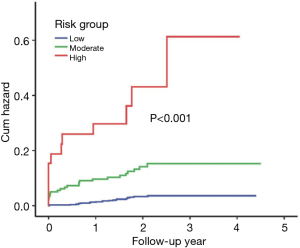
Discussion
According to retrospective analysis of the single center data among patients undergoing CAG, we established one simple and precise CIN risk assessment tool with pre-procedural key variables including old age, high SCr, hypotension, CHF, and AMI. Compared to classical Mehran and ACEF scores, the new, simple, and pre-procedural risk score across the validation dataset exhibited similar discrimination and predictive ability for the risk of CIN and mortality.
With the growing trend towards minimally invasive diagnostic and interventional procedures with contrast, there has been a concomitant rise in the incidence of CIN (10). CINs have been associated with increased risk of adverse clinical outcomes, including more complex complications and death (1). Because we lack effective therapies for CIN, the establishment of useful prediction models for CIN would be important and instrumental in prevention. Many studies searching for a prediction model of CIN were performed worldwide in an attempt to help identify those patients at high risk who might benefit from peri-procedural strategies that protect the kidney or improve pre-intervention counseling (11,13-21). In a recent systematic review, models using preprocedural variables performed decently with similar results to those of models that incorporated post-procedural variables (22). Our pre-procedure also showed good performance despite lacking post-procedural variables. Chen et al. established 1 preprocedural score for risk of CIN after PCI with good predictive value (c-statistic: 0.82), but the score system included 9 variables, which was more complex than ours. Risk factors lacking the validation and improvement of downstream effects make it challenging for a clinician to select the ideal model in practice. Further research is needed to evaluate the effect of their implementation in clinical care.
Most CIN risk models were developed with definitions of CIN0.5 (increase ≥0.5 mg/dL in SCr) and CIN25 (increase ≥25% and/or >0.5 mg/dL in SCr) (11,13-21,23). A large collaborative registry that included 58 957 patients undergoing PCI suggested that the definition of CIN (increase ≥0.5 mg/dL) is superior to ≥25% increase in SCr for identifying patients at a greater risk of adverse renal and cardiac events among patients undergoing PCI (20). In addition, the combined CIN (increase of ≥25% or absolute 0.5 mg/dL creatinine levels) was not significantly correlated with long-term mortality in patients without CKD (21). Therefore, we chose CIN0.5 (increase ≥0.5 mg/dL in SCr) as the endpoint for a risk model with more prognosis value
Advanced age and worse renal function (CKD) were common risk factors for CIN following CAG (11). Recent studies showed that adequate hydration appeared to have a very low risk of CIN following angiography among patients with CKD and CHF. The HYDRA Study showed that evaluation of pre-procedural hydration status allowed higher volume expansion with reduced risk of CIN; thus, a personalized hydration volume could be a more reasonable strategy for the prevention of CIN in the future (24,25). Among patients with CHF, renal vasoconstriction and medullary hypoxia play an important role in the development of CIN. In addition, the effective circulatory volumes are lower in patients who have diminished left ventricular function. The patients were likely to have kidney hypoperfusion with hemodynamic instability, high degree of inflammatory status in the body, along with an inflammation status, endothelial dysfunction, and oxidative stress, that were contributing to the development of CIN. Our new score also included AMI as an independent predictor of CIN following CAG (19,26).
The patients with diabetic nephropathy undergoing CAG had a very high risk of developing CIN (27). Assareh’s study also found that CIN is a common problem in patients with diabetic nephropathy undergoing CAG (28). Peripheral arterial disease (PAD) was also considered as a risk factor (6,15,16), but it did not appear to be associated with CIN in our data, which is more consistent with Mehran and Gurm’s studies (11,18).
Limitations
Several limitations exist in the present study. First, since this prospective, observational study was conducted in a single center, further research is needed to evaluate the effect of their implementation in clinical care. Secondly, the CrCl was calculated with the Cockcroft-Gault formula, rather than measured directly. Thirdly, variations in our measurement times might have given rise to missing post-procedure peak SCr levels. Furthermore, half of the patients were discharged 3 days after the CAG, so Scr concentrations were not measured on day 3 in these patients. Variation in the measurement times may have led to overlooked peak levels of the SCr post procedure, which may have also led to an underestimation of the true incidence of CIN in the current study population. At last, poor patient compliance led to a high rate of follow-up loss, which may have affected the results about clinical adverse outcomes during follow-up, and may have influenced the significance of the analysis.
Conclusions
The present study established a simple risk score, which included only 5 key pre-procedural variables with excellent predictive and high discriminative ability, even when compared with classical Mehran and ACEF score, for predicting CIN and short- and long-term outcome in patients before CAG. This could benefit timely administration of pre-procedural preventions. However, the value of the pre-procedural risk model needs to be evaluated in large scale multicenter trials in the future.
Acknowledgements
Funding: The study was supported by The Science and Technology Planning Project of Guangdong Province (grant No. 2014B070706010), The Technology Planning Project of Dongguan Province (grant No. 2015108101022), The National Science Foundation for Young Scientist of China (grant No. 81500520), The Progress in Science and Technology Project of Guangdong Province (grant No. 2015A030302037), Guangdong Provincial Medical Research Fund Project (GSIC20140526), and Guangdong Provincial People’s Hospital Clinical Transformation Research Project (2015zh01), National Clinical Key Specialty Construction Project of China (2012-649, 2013-544).
Footnote
Conflicts of Interest: The authors have no conflicts of interest to declare.
Ethical Statement: The study was approved by The Guangdong General Hospital Ethics Committee and written informed consent was obtained from all patients.
References
- Wi J, Ko YG, Shin DH, et al. Prediction of Contrast-Induced Nephropathy With Persistent Renal Dysfunction and Adverse Long-term Outcomes in Patients With Acute Myocardial Infarction Using the Mehran Risk Score. Clin Cardiol 2013;36:46-53. [Crossref] [PubMed]
- Luo Y, Wang X, Ye Z, et al. Remedial hydration reduces the incidence of contrast-induced nephropathy and short-term adverse events in patients with ST-segment elevation myocardial infarction: a single-center, randomized trial. Intern Med 2014;53:2265-72. [Crossref] [PubMed]
- Ballı M, Taşolar H, Çetin M, et al. Is atrial fibrillation a risk factor for contrast-induced nephropathy in patients with ST-elevation myocardial infarction? J Cardiol 2016;67:327-30. [Crossref] [PubMed]
- Wang N, Qian P, Yan TD, et al. Periprocedural effects of statins on the incidence of contrast-induced acute kidney injury: A systematic review and trial sequential analysis. Int J Cardiol 2016;206:143-52. [Crossref] [PubMed]
- Maioli M, Toso A, Gallopin M, et al. Preprocedural score for risk of contrast-induced nephropathy in elective coronary angiography and intervention. J Cardiovasc Med (Hagerstown) 2010;11:444-9. [Crossref] [PubMed]
- Ji L, Su X, Qin W, et al. Novel risk score of contrast-induced nephropathy after percutaneous coronary intervention. Nephrology (Carlton) 2015;20:544-51. [Crossref] [PubMed]
- Silver SA, Shah PM, Chertow GM, et al. Risk prediction models for contrast induced nephropathy: systematic review. BMJ 2015;351:h4395. [Crossref] [PubMed]
- Liu Y, Chen JY, Tan N, et al. Safe limits of contrast vary with hydration volume for prevention of contrast-induced nephropathy after coronary angiography among patients with a relatively low risk of contrast-induced nephropathy. Circ Cardiovasc Interv 2015. [Crossref] [PubMed]
- Liu Y, Lin L, Li Y, et al. Relationship Between the Urine Flow Rate and Risk of Contrast-Induced Nephropathy After Emergent Percutaneous Coronary Intervention. Medicine (Baltimore) 2015;94:e2258. [Crossref] [PubMed]
- Slocum NK, Grossman PM, Moscucci M, et al. The changing definition of contrast-induced nephropathy and its clinical implications: insights from the Blue Cross Blue Shield of Michigan Cardiovascular Consortium (BMC2). Am Heart J 2012;163:829-34. [Crossref] [PubMed]
- Mehran R, Aymong ED, Nikolsky E, et al. A simple risk score for prediction of contrast-induced nephropathy after percutaneous coronary intervention: development and initial validation. J Am Coll Cardiol 2004;44:1393-9. [PubMed]
- Andò G, Morabito G, de Gregorio C, et al. The ACEF score as predictor of acute kidney injury in patients undergoing primary percutaneous coronary intervention. Int J Cardiol 2013;168:4386-7. [Crossref] [PubMed]
- Liu Y, Liu YH, Tan N, et al. Novel risk scoring for pre-procedural prediction of contrast-induced nephropathy and poor long-term outcomes among patients with chronic total occlusion undergoing percutaneous coronary intervention. Eur Heart J Suppl 2015;17:C34-C41. [Crossref]
- Marenzi G, Lauri G, Assanelli E, et al. Contrast-induced nephropathy in patients undergoing primary angioplasty for acute myocardial infarction. J Am Coll Cardiol 2004;44:1780-5. [Crossref] [PubMed]
- Bartholomew BA, Harjai KJ, Dukkipati S, et al. Impact of nephropathy after percutaneous coronary intervention and a method for risk stratification. Am J Cardiol 2004;93:1515-9. [Crossref] [PubMed]
- Tziakas D, Chalikias G, Stakos D, et al. Development of an easily applicable risk score model for contrast-induced nephropathy prediction after percutaneous coronary intervention: a novel approach tailored to current practice. Int J Cardiol 2013;163:46-55. [Crossref] [PubMed]
- Tsai TT, Patel UD, Chang TI, et al. Validated contemporary risk model of acute kidney injury in patients undergoing percutaneous coronary interventions: insights from the National Cardiovascular Data Registry Cath-PCI Registry. J Am Heart Assoc 2014;3:e001380. [Crossref] [PubMed]
- Gurm HS, Seth M, Kooiman J, et al. A novel tool for reliable and accurate prediction of renal complications in patients undergoing percutaneous coronary intervention. J Am Coll Cardiol 2013;61:2242-8. [Crossref] [PubMed]
- Bouzas-Mosquera A, Vázquez-Rodríguez JM, Calviño-Santos R, et al. Contrast-induced nephropathy and acute renal failure following emergent cardiac catheterization: incidence, risk factors and prognosis. Rev Esp Cardiol 2007;60:1026-34. [Crossref] [PubMed]
- Andò G, Morabito G, de Gregorio C, et al. Age, glomerular filtration rate, ejection fraction, and the AGEF score predict contrast-induced nephropathy in patients with acute myocardial infarction undergoing primary percutaneous coronary intervention. Catheter Cardiovasc Interv 2013;82:878-85. [Crossref] [PubMed]
- Fu N, Li X, Yang S, et al. Risk score for the prediction of contrast-induced nephropathy in elderly patients undergoing percutaneous coronary intervention. Angiology 2013;64:188-94. [Crossref] [PubMed]
- Allen DW, Ma B, Leung KC, et al. Risk Prediction Models for Contrast-Induced Acute Kidney Injury Accompanying Cardiac Catheterization: Systematic Review and Meta-analysis. Can J Cardiol 2017;33:724-36. [Crossref] [PubMed]
- Chen YL, Fu NK, Xu J, et al. A simple preprocedural score for risk of contrast-induced acute kidney injury after percutaneous coronary intervention. Catheter Cardiovasc Interv 2014;83:E8-16. [Crossref] [PubMed]
- Qian G, Fu Z, Guo J, et al. Prevention of Contrast-Induced Nephropathy by Central Venous Pressure-Guided Fluid Administration in Chronic Kidney Disease and Congestive Heart Failure Patients. JACC Cardiovasc Interv 2016;9:89-96. [Crossref] [PubMed]
- Maioli M, Toso A, Leoncini M, et al. Bioimpedance-Guided Hydration for the Prevention of Contrast-Induced Kidney Injury: The HYDRA Study. J Am Coll Cardiol 2018;71:2880-9. [Crossref] [PubMed]
- Rihal CS, Textor SC, Grill DE, et al. Incidence and prognostic importance of acute renal failure after percutaneous coronary intervention. Circulation 2002;105:2259-64. [Crossref] [PubMed]
- Chong E, Poh KK, Liang S, et al. Risk factors and clinical outcomes for contrast-induced nephropathy after percutaneous coronary intervention in patients with normal serum creatinine. Ann Acad Med Singapore 2010;39:374-80. [PubMed]
- Assareh A, Yazdankhah S, Majidi S, et al. Contrast induced nephropathy among patients with normal renal function undergoing coronary angiography. J Renal Inj Prev 2016;5:21-4. [Crossref] [PubMed]
(English Language Editor: John Ayric Gray, AME Publishing Company)