Nomogram predicting cancer-specific mortality in patients with esophageal adenocarcinoma: a competing risk analysis
Introduction
The incidence of esophageal adenocarcinoma (EAC) has dramatically increased in Western countries (1). It is characterized by several epidemiologic features, including male gender, white race, high body mass index, and age (2,3). Most patients with EAC present with symptoms of dysphagia from late-stage tumors, but only a portion of them are identified by screening and surveillance (4). Accurate prognosis estimates based on clinic pathologic factors play a vital role in determining therapeutic strategies in the shortest possible time.
Esophageal squamous cell carcinoma has been widely investigated in a significant amount of research (5-7), while EAC, as the other subtype of esophageal cancers, has been less studied. It has been previously reported that TP53 gene mutations were related to reduced overall survival of patients with EAC (8). Although four genes were demonstrated to be prognostic for EAC (9), none of them are easy to obtain in routine clinical work. Elevated BMI in early adulthood and substantial cumulative smoking history were suggested to be associated with the mortality risk of EAC patients in one study in North America (10). A study using the surveillance, epidemiology, and end results (SEER) database with data from 1973 to 2003 showed that patients who were older and did not undergo esophagectomy had a worse overall survival (11). At the same time, the older and obese population constituted a larger proportion of patients with EAC (1,12), but their mortalities were likely increased due to other causes such as metabolic syndrome and cardiovascular diseases (13). Therefore, competing causes of death should be taken into account when evaluating the prognosis of this disease.
A competing risk is an event whose occurrence precludes the critical event of interest. Competing risk analysis is time-to-event analysis that considers all kinds of fatal or non-fatal events which potentially alter or prevent subjects from experiencing the interest endpoint (14,15). Thus, when predicting the incidence of the outcome of disease, competing risk analysis can provide a more accurate and less biased estimate for clinicians to make individual therapy strategies (16).
The nomogram visualizes the complex regression equation, making the results of the prognostic model more readable and convenient for the evaluation (17). Therefore, the nomogram is intuitive and easy to understand having been gradually applied to medical research and clinical practice.
In this study, we conducted a competing-risk analysis for EAC in the SEER database using cumulative incidence function (CIF) instead of Kaplan-Meier survival function when estimating the crude incidence of endpoint event (16,18). A competing risk nomogram model to predict individual long-term cancer-specific mortality for EAC and a nomogram model to predict overall mortality were constructed and validated.
Methods
Patient selection
The SEER database (https://seer.cancer.gov/) was used in this study. Patients who were clinically or pathologically diagnosed with EAC (based on the histologies stage table of the collaborative stage data set with the ICD-O-3 codes 8050, 8140–8147, 8160–8162, 8170–8175, 8180–8221, 8250–8507, 8514–8551, 8571–8574, 8576, 8940–8941 from SEER database) between 2004 and 2013 were included, among whom subjects without recorded survival time were omitted. The specific process is shown in Figure S1. Tumors were classified according to the 7th edition of the American Joint Committee on Cancer (AJCC) staging manual (19).
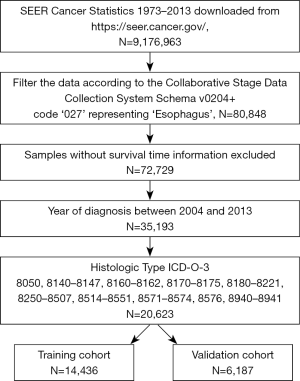
Construction of the nomogram
Variables that achieved a significance value of P<0.05 in univariate Cox analysis were selected for multivariate Cox analysis. On this basis, a total of seven independent clinic pathological prognostic factors were integrated into the nomogram which predicted 1-, 3- and 5-year EAC-specific mortality after diagnosis. In addition to these variables, a factor of age was included to build a nomogram which predicted 1-, 3- and 5-year overall mortality.
The CIFs of mortality were plotted to depict trends over time among the different T, M, and N subgroups of the training cohort and the validation cohort. The calibration plots graphically displayed the relationship between the predicted and observed risk for the outcomes of the nomogram.
Receiver operating characteristic curve and decision curve analysis (DCA)
The time-dependent receiver operating characteristic (td-ROC) curve analysis was adopted to evaluate the predictive capacity of the nomograms for EAC-specific mortality and overall mortality. DCA was used to compare the potential net benefit of the established models.
Statistical analysis
Statistical analysis was performed using SPSS 23 and R version 3.4.4. Univariate and multivariate Cox regression analyses were completed by SPSS. R software was used for building the nomogram. “Rms” and “survival” packages were used for survival analysis, and “Hmics”, “timeROC”, and “rmda” were used for the performance evaluation. All P values resulted from two-sided statistical testing. All calculations of CIFs of EAC-specific survival were carried out using SPSS.
Results
Demographic and clinical characteristics of patients at diagnosis
From 2004 to 2013, we gathered data on 20,623 patients with EAC who met the inclusion criteria from the SEER database. All of the patients were randomly distributed into the training cohort (N=14,436) or the validation cohort (N=6,187). Table 1 shows the demographic and clinical characteristics of patients of the two cohorts at diagnosis, and every cohort was divided into subgroups of death of EAC and other causes.
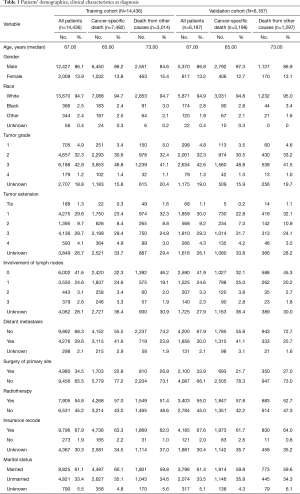
Full table
At diagnosis in the training cohort, the median age of all patients was 67.00 years, whereas patients of cancer-specific death and patients of death from other causes had a significant difference (65.00 vs. 73.00 years). The majority of patients were men (86.2% vs. 84.6%) and of white race (94.7% vs. 94.7%). A considerable proportion of patients had tumors with T1 (23.4% vs. 32.3%) or T3 (29.4% vs. 24.9%) category, no lymph node involvement (32.3% vs. 46.2%), and no distant metastasis (55.5% vs. 74.2%). Most of the cohorts had not received surgical treatment (77.2% vs. 73.1%) at the primary site. The number of patients who were administered by radiotherapy (57.0% vs. 51.4%) slightly outnumber those who were not. Most patients had insurance records (63.3% vs. 62.0%) and were married (60.1% vs. 59.8%).
CIFs of EAC-specific survival
There were 1-, 3- and 5-year CIFs of EAC-specific survival in the training patients estimating by age at diagnosis, gender, tumor grade, tumor extension, the involvement of lymph nodes, distant metastasis, surgery of primary site, radiotherapy, insurance recode, and marital status at diagnosis (Table 2). Table 2 demonstrates that for 1, 3, and 5 years after diagnosis, the CIFs of EAC-specific survival were 60.9%, 37.1%, and 31.3% respectively. Moreover, the CIFs of survival calculated by several characteristics decreased over time. The patients with a high CIF of survival were typically male, had low-grade tumors, were low T, N (no lymph node involvement), and M (no distant metastasis) categories, received surgical treatment-administered radiotherapy, had an insurance record, and were married. The curves are summarized in Figure 1. As can be seen, earlier T stage, N0, and M0 predicted decreased CIFs of cancer-specific death. As for CIFs of other causes of death, there was no significant difference.
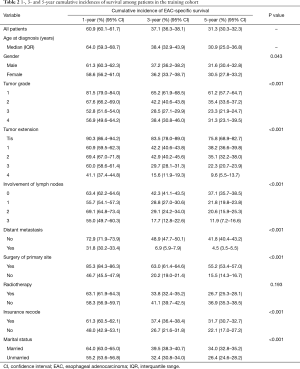
Full table
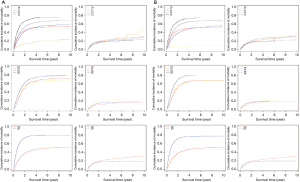
Nomogram and clinical usage
As Table 3 shows, incorporated into the prognostic prediction nomogram, the seven variables of tumor grade, tumor extension, involvement of lymph nodes, distant metastasis, surgery of primary site, insurance record, and marital status at diagnosis (all the significance of P<0.001) were found to be statistically associated with cancer-specific mortality. In addition to the above seven factors, age at diagnosis was also statistically significant (P<0.001) in the nomogram model of overall mortality.
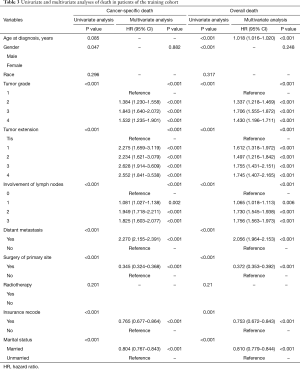
Full table
The nomograms, which calculated the sum of points corresponding to the patient’s characteristics, predicted the probability of 1-, 3- and 5-year cancer-specific mortality via the competing risk model and overall mortality (Figure 2). Regarding the clinical application of this nomogram, we can take a patient who has recently been diagnosed EAC as an example. This patient is a married, 50-year-old Chinese man who has health insurance records. CT showed no distant metastasis. After eliminating relevant contraindication, he received primary site surgery. Combined with intraoperative findings, the stage was evaluated as T2M0N1. Biopsy showed poorly differentiated cell type. As for the EAC mortality prediction, G3 corresponds to 63.7 points vertically at the top bar in nomogram; T2 corresponds to 17 points; and N1 corresponds to 22 points. Moreover, factors of M0, receiving surgery, having health insurance records, and being married correspond to 0. The sum of all the points constitutes the total points and is then used to predict the mortalities according to the corresponding percent at the bottom bar. The result shows that he has 102.7 points and 80.2 points in EAC mortality and overall mortality nomogram respectively. The predictive 1-year EAC mortality is slightly lower than 23%, and the 1-year overall mortality rate is a bit lower than 21%. The predictive 3- and 5-year EAC mortalities are both lower than 50%. Through this practical tool, the physician can stratify this patient and make individualized recommendations for his follow-up administration.
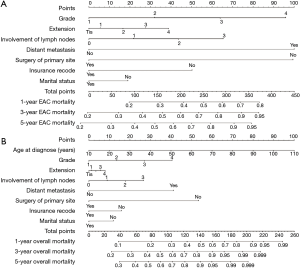
The C-indexes of the 1-, 3- and 5-year EAC-specific mortality nomogram in the training cohort were 0.733, 0.728, and 0.728, respectively, while the values in the validation cohort were 0.721, 0.721, and 0.720 respectively. Moreover, the C-indexes of the 1-, 3-, and 5-year overall mortality nomogram in the training cohort were 0.726, 0.720, and 0.719 respectively, while the values in the validation cohort were 0.715, 0.713, and 0.712 respectively. The td-area under the curve (td-AUC) of the 1-, 3-, and 5-year EAC-specific mortality nomogram in the training cohort were 0.801, 0.852, and 0.863 respectively, while the values in the validation cohort were 0.791, 0.854, and 0.856 respectively. As for 1-, 3- and 5-year overall mortality nomogram, the values of td-AUC were 0.793, 0.843, 0.858 in the training cohort respectively, and 0.782, 0.839, 0.849 in the validation cohort respectively. Figures 3,4 show the curves of the td-ROC. Above all, the nomograms have a good model discriminative capacity. The calibration curves are shown in Figures 5,6. The actual CIFs and the predicted probability of mortalities for 1, 3, and 5 years between training and validation cohorts were in good agreement. Hence, the nomograms were well-calibrated.
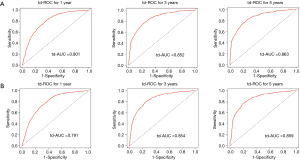
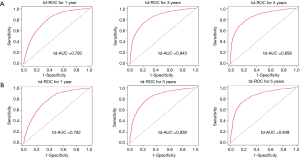
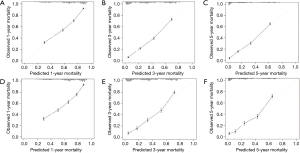
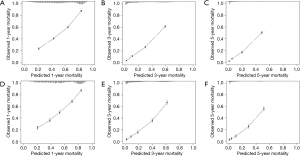
To evaluate the clinical utility, the DCA was introduced. The plots of DCA cancer-specific mortality and overall mortality between the training and validation cohorts are shown in Figure 7, indicating the positive net benefit of the established models.
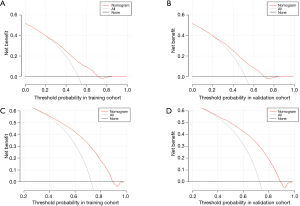
Discussion
In the current study, we estimated the probability of death for patients diagnosed with EAC in the SEER database between 2004 and 2013 and calculated the 1-, 3-, and 5-year CIFs for EAC-specific survival. The brief nomogram based on a competing risks model was built to predict the probability of EAC-specific death and the other nomogram was built to predict the probability of overall death.
We observed that gender made none statistical difference in cancer-specific mortality, which was consistent with a previous study of the relationship between gender and prognosis in esophageal cancer (20,21) but conflicted with several previous studies (22-26), which indicated the higher incidence and poorer prognosis in men. To some extent, the reasons for this could be the vitamin D receptor (22), sex steroid hormones (26), and androgen/estrogen balance (24). In previous studies, age was also indicated to be an independent predictor of survival for patients with EAC (27). Increasing patient age was associated with a statistically increased mortality (28). Similarly, we identified the age at diagnosis as an independent negative prognostic factor for EAC patients when estimating overall mortality.
On the contrary, for EAC-specific mortality, age did not make a statistical difference in our study. It was indicated that the tumor itself has a significant effect on mortality caused by EAC, and age should be taken as a reference instead of an unalterable vital condition to evaluate the prognosis of EAC. After evaluation of the EAC-specific mortality nomogram, more aggressive management might be arranged for more elderly patients who still have a good prognosis prediction.
In summary, introducing EAC-specific mortality via competing for risk analysis when estimating the prognosis of patients with EAC is necessary. We also did not find a significant effect of race on prognosis. By contrast, several studies of mortality disparities by race and ethnicity in EAC showed that adenocarcinoma mainly affected the non-whites or the blacks, and led to a worse prognosis (29-31). This phenomenon might be caused by the higher proportion of the white population having a better income and hence better opportunity for medical treatment.
Furthermore, radiotherapy did not have a significant effect on cancer-specific mortality, which contrasted with other studies that used the SEER cohort (32,33). While these studies indicated that the impact of radiotherapy was reflected among various subgroup analyses, our study investigated the entire population of EAC rather than subgroup analyses. Since most of the population provided with radiotherapy were patients with most late-stage tumors whose condition were too poor to undergo surgery, the benefit of radiotherapy seemed to be limited for the prognosis of EAC patients in this study.
To our knowledge, this is the first study attempting to develop a nomogram for integrating a competing risk model for predicting cancer-specific mortality in EAC. Since EAC was associated with many complications, there was a maximum number of possibilities leading to biased results. Thus, a competing risk analysis was necessary for our study. The nomogram of our study appeared to capture effective discrimination ability and a satisfying clinical net benefit. All the variables listed could be easily obtained from routine clinical work. Therefore, based on combining clinical features and clinical information, this predictive tool can be easily and graphically used by clinicians to make a quick prognosis judgment for patients by drawing a few lines in seconds without any difficulty in calculation.
Additionally, our nomograms can help patients understand the possibility of prognosis graphically. Another virtue of this study is the large cohort size and long-term follow-up provided by the SEER database to refine the model. Also, we set a validation cohort to attain external validation, and the subsequent results were positive.
Our study has several limitations. Firstly, some clinicopathologic factors commonly cited (such as surgical margins, genetic mutation, presence of Barrett’s esophagus, neoadjuvant therapies, the extent of surgery, BMI, and type of surgery) affecting prognosis are not documented in the SEER database and neither are data on cancer recurrence and chemotherapy. Secondly, the AJCC 8th edition has been widely used since being published in 2017, but tumors were still classified according to the AJCC 7th staging manual in this study since the SEER database had not converted the TNM data according to AJCC 8th standard. In addition to this, G4 (undifferentiated cancer) in AJCC 7th was canceled in AJCC 8th. However, the number of G4 cases in the training cohort and validation cohort account for 1.2% of the total, and the application of the nomogram among EAC patients diagnosed by AJCC 8th could cause no more than a 5.5% change of the predicted mortality.
Additionally, the total stage was not included to avoid multicollinearity influences and limitations of the clinical application of the model when considering the addition of clinical staging (cTNM staging) and pathological staging after neoadjuvant therapy (ypTNM staging) in the AJCC 8th staging system. Undoubtedly, further improvement of this model should be conducted in future clinical research according to the AJCC 8th edition. Thirdly, since our data came from the SEER database produced in America, the model needs to be validated in multi-ethnic and multi-regional investigations.
Conclusions
We calculated the CIFs of cancer-specific death and other causes of death for patients with EAC using the SEER database. The brief nomograms based on the competing and overall risk analyses were built with variables that could be obtained with little difficulty. We believe that these nomograms could be easily used by clinicians to predict prognosis and help determine a personalized treatment for EAC patients.
Acknowledgments
None.
Footnote
Conflicts of Interest: The authors have no conflicts of interest to declare.
Ethical Statement: The authors are accountable for all aspects of the work in ensuring that questions related to the accuracy or integrity of any part of the work are appropriately investigated and resolved.
References
- Coleman HG, Xie SH, Lagergren J. The Epidemiology of Esophageal Adenocarcinoma. Gastroenterology 2018;154:390-405. [Crossref] [PubMed]
- Murphy CC, Yang YC, Shaheen NJ, et al. An age-period-cohort analysis of obesity and incident esophageal adenocarcinoma among white males. Dis Esophagus 2017;30:1-8. [PubMed]
- Rubenstein JH, Shaheen NJ. Epidemiology, Diagnosis, and Management of Esophageal Adenocarcinoma. Gastroenterology 2015;149:302-17.e1. [Crossref] [PubMed]
- Spechler SJ, Katzka DA, Fitzgerald RC. New Screening Techniques in Barrett's Esophagus: Great Ideas or Great Practice? Gastroenterology 2018;154:1594-601. [Crossref] [PubMed]
- Domper Arnal MJ, Ferrandez Arenas A, Lanas Arbeloa A. Esophageal cancer: Risk factors, screening and endoscopic treatment in Western and Eastern countries. World J Gastroenterol 2015;21:7933-43. [Crossref] [PubMed]
- Lagergren J, Smyth E, Cunningham D, et al. Oesophageal cancer. Lancet 2017;390:2383-96. [Crossref] [PubMed]
- Penniment MG, De Ieso PB, Harvey JA, et al. Palliative chemoradiotherapy versus radiotherapy alone for dysphagia in advanced oesophageal cancer: a multicentre randomised controlled trial (TROG 03.01). Lancet Gastroenterol Hepatol 2018;3:114-24. [Crossref] [PubMed]
- Fisher OM, Lord SJ, Falkenback D, et al. The prognostic value of TP53 mutations in oesophageal adenocarcinoma: a systematic review and meta-analysis. Gut 2017;66:399-410. [Crossref] [PubMed]
- Peters CJ, Rees JR, Hardwick RH, et al. A 4-gene signature predicts survival of patients with resected adenocarcinoma of the esophagus, junction, and gastric cardia. Gastroenterology 2010;139:1995-2004.e15. [Crossref] [PubMed]
- Spreafico A, Coate L, Zhai R, et al. Early adulthood body mass index, cumulative smoking, and esophageal adenocarcinoma survival. Cancer Epidemiol 2017;47:28-34. [Crossref] [PubMed]
- Cen P, Banki F, Cheng L, et al. Changes in age, stage distribution, and survival of patients with esophageal adenocarcinoma over three decades in the United States. Ann Surg Oncol 2012;19:1685-91. [Crossref] [PubMed]
- Fang X, Wei J, He X, et al. Quantitative association between body mass index and the risk of cancer: A global Meta-analysis of prospective cohort studies. Int J Cancer 2018. [Epub ahead of print]. [Crossref] [PubMed]
- Jokinen E. Obesity and cardiovascular disease. Minerva Pediatr 2015;67:25-32. [PubMed]
- Gooley TA, Leisenring W, Crowley J, et al. Estimation of failure probabilities in the presence of competing risks: new representations of old estimators. Stat Med 1999;18:695-706. [Crossref] [PubMed]
- Arbetter DF, Jain P, Yee MK, et al. Competing risk analysis in a large cardiovascular clinical trial: An APEX substudy. Pharm Stat 2017;16:445-50. [Crossref] [PubMed]
- Austin PC, Lee DS, Fine JP. Introduction to the Analysis of Survival Data in the Presence of Competing Risks. Circulation 2016;133:601-9. [Crossref] [PubMed]
- Lei Z, Li J, Wu D, et al. Nomogram for Preoperative Estimation of Microvascular Invasion Risk in Hepatitis B Virus-Related Hepatocellular Carcinoma Within the Milan Criteria. JAMA Surg 2016;151:356-63. [Crossref] [PubMed]
- van Walraven C, McAlister FA. Competing risk bias was common in Kaplan-Meier risk estimates published in prominent medical journals. J Clin Epidemiol 2016;69:170-3.e8. [Crossref] [PubMed]
- Edge SB, Compton CC. The American Joint Committee on Cancer: the 7th edition of the AJCC cancer staging manual and the future of TNM. Ann Surg Oncol 2010;17:1471-4.
- Kauppila JH, Wahlin K, Lagergren P, et al. Sex differences in the prognosis after surgery for esophageal squamous cell carcinoma and adenocarcinoma. Int J Cancer 2019;144:1284-91. [Crossref] [PubMed]
- Kauppila JH, Mattsson F, Brusselaers N, et al. Prognosis of oesophageal adenocarcinoma and squamous cell carcinoma following surgery and no surgery in a nationwide Swedish cohort study. BMJ Open 2018;8:e021495. [Crossref] [PubMed]
- Zhou Z, Xia Y, Bandla S, et al. Vitamin D receptor is highly expressed in precancerous lesions and esophageal adenocarcinoma with significant sex difference. Hum Pathol 2014;45:1744-51. [Crossref] [PubMed]
- Xie SH, Lagergren J. The Male Predominance in Esophageal Adenocarcinoma. Clin Gastroenterol Hepatol 2016;14:338-47.e1. [Crossref] [PubMed]
- Petrick JL, Falk RT, Hyland PL, et al. Association between circulating levels of sex steroid hormones and esophageal adenocarcinoma in the FINBAR Study. PLoS One 2018;13:e0190325. [Crossref] [PubMed]
- Dong J, Buas MF, Gharahkhani P, et al. Determining Risk of Barrett's Esophagus and Esophageal Adenocarcinoma Based on Epidemiologic Factors and Genetic Variants. Gastroenterology 2018;154:1273-81.e3. [Crossref] [PubMed]
- Mathieu LN, Kanarek NF, Tsai HL, et al. Age and sex differences in the incidence of esophageal adenocarcinoma: results from the Surveillance, Epidemiology, and End Results (SEER) Registry (1973-2008). Dis Esophagus 2014;27:757-63. [Crossref] [PubMed]
- Sepesi B, Schmidt HE, Lada M, et al. Survival in Patients With Esophageal Adenocarcinoma Undergoing Trimodality Therapy Is Independent of Regional Lymph Node Location. Ann Thorac Surg 2016;101:1075-80; Discussion 1080-1. [Crossref] [PubMed]
- Eng OS, Nelson RA, Konstantinidis I, et al. Disparities in survival after trimodality therapy for esophageal adenocarcinoma. Dis Esophagus 2018;31. [Crossref] [PubMed]
- Baquet CR, Commiskey P, Mack K, et al. Esophageal cancer epidemiology in blacks and whites: racial and gender disparities in incidence, mortality, survival rates and histology. J Natl Med Assoc 2005;97:1471-8. [PubMed]
- Revels SL, Morris AM, Reddy RM, et al. Racial disparities in esophageal cancer outcomes. Ann Surg Oncol 2013;20:1136-41. [Crossref] [PubMed]
- Tramontano AC, Nipp R, Mercaldo ND, et al. Survival Disparities by Race and Ethnicity in Early Esophageal Cancer. Dig Dis Sci 2018;63:2880-8. [Crossref] [PubMed]
- Wojcieszynski AP, Berman AT, Wan F, et al. The impact of radiation therapy sequencing on survival and cardiopulmonary mortality in the combined modality treatment of patients with esophageal cancer. Cancer 2013;119:1976-84. [Crossref] [PubMed]
- Wu SG, Xie WH, Zhang ZQ, et al. Surgery Combined with Radiotherapy Improved Survival in Metastatic Esophageal Cancer in a Surveillance Epidemiology and End Results Population-based Study. Sci Rep 2016;6:28280. [Crossref] [PubMed]