Discovery of potential plasma protein biomarkers for acute myocardial infarction via proteomics
Introduction
Cardiovascular disease has become the leading cause of mortality in humans. Nearly 20 million individuals worldwide die from acute cardiovascular events every year. Most individuals do not have precursor symptoms. It is difficult to detect victims prior to cardiovascular events with existing diagnostic techniques. Acute myocardial infarction (AMI) is a myocardial necrosis caused by acute and persistent ischemia and hypoxia of the coronary artery. Approximately 1.5 million individuals in the United States suffer from AMI every year. Because AMI always occurs suddenly and severely, without aura and precursor symptoms, it is difficult to predict and diagnose, as well as prevent and treat, resulting in a financial burden for the government and family and a decline of patient quality of life. Thus, the diagnosis and treatment of AMI are urgent global problems.
Several studies have found changes in the plasma levels of biomarkers in acute coronary syndrome (ACS) patients related to myocyte necrosis, inflammation, plaque rupture, coagulation, and platelet activation (1-3). Many proteins, such as CD40L, several interleukins, and adhesion molecules, have been studied as potential candidates for risk prediction (4). However, none of them have been consistently demonstrated to add predictive value to the clinical variables used in clinical practice (4). Similar to observations in cancer studies, a single biomarker is unlikely to be suitable for all patients with ACS. Accordingly, multiple biomarker approaches may prove more advantageous than a single biomarker (5).
Most studies have focused on atherosclerotic plaques, vascular cells, monocytes and platelets in the blood (4); however, the plasma is few and difficult to consider because of its large quantities of resident proteins. Nevertheless, plasma is the most common and practical test specimen in clinical work. Identification of plasma specific factors expressed in patients with AMI will help identify the disease as early as possible, judge the severity of myocardial infarction, and make an accurate and effective prognosis prediction. Previous studies have identified many new factors (6,7); however, the results are complex and diverse, suggesting that we need to repeat multiple tests to eventually screen out truly useful biomarkers. To screen new useful biomarkers for the diagnosis of AMI, we compared the expression of plasma proteins between patients with myocardial infarction and no myocardial infarction via proteomics.
Methods
Patients
Human plasma samples collected from blood samples of patients with no myocardial infarction (CON, n=5) and patients with acute myocardial infarction (AMI, n=10) were provided by the department of cardiovascular medicine of Taizhou Hospital of Zhejiang Province, China. AMI was diagnosed based on a history of prolonged ischemic chest pain, elevation of troponin-I and creatine kinase more than three times the normal value, with a concomitant rise in troponin-I and MB isoenzyme, and ST-segment elevations. Patients who met at least two of these criteria were included in the study. Patients with noncardiac diseases that may have affected results, such as inflammatory disorders, cancer, or infection, were excluded. The clinical and demographic characteristics of the patients with AMI and the CON are shown in Table 1. The collected blood samples were then centrifuged at 3,000 rpm for 10 min at room temperature to remove cells and debris. The study was approved by the scientific ethics committee (K20130307, Taizhou hospital of Zhejiang Province, China). Written informed consent was provided by all participants and legal guardians prior to the commencement of this study.
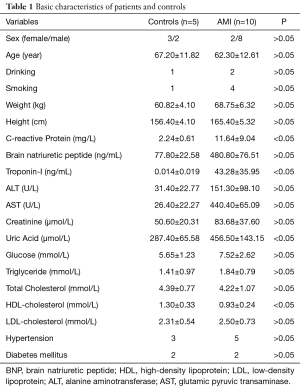
Full table
Removal of high abundance protein
The plasma samples were divided into the following two groups: the control group and the AMI patient group. Two groups of high protein abundant components in human plasma were removed by Multiple Affinity Removal System (MARS 5185–5984) of Agilent Company. The specific operation was performed as follows: add 30 to 120 µL A solution, use 0.22 µm ultrafiltration tube, 4 °C, and centrifuge at 16,000 ×g for 3 minutes. The filtrated filtrate was 100 µL in the sampling bottle. An Agilent 1,100 high performance liquid chromatography (HPLC) system was used to remove the samples with a high abundance. After 5 min of using 1 mL/min A liquid equilibrium MARS column, a 20-min gradient was set, 0.00–9.00 min A, 9.00–12.50 min B and 12.60–20.00 min A. The flow rates were 0.250 mL/min for 0.00–9.00 min and 1 mL/min for 9.01–20.00 min. Low abundance protein was collected in 1.8–5 min, freeze-dried and −80 degree preserved. The absorption wavelength was set at 280 nm.
Fluorescence quantification of protein concentration
After removing 15 plasma samples with high abundance and freeze-drying mix, they were resolved with 150 UL saline (0.9% NaCl) and then quantified. The specific operation was performed as follows: protein quantification was carried out using the BCA Protein Quantitation Kit. A 2 µL protein solution was added to the 200 µL reaction solution. The wavelength was set to 630 nm. The protein standard substance (BSA) was diluted to draw the standard curve and calculate the protein concentration in the sample.
Protein 1D SDS-PAGE
The plasma protein with high abundant protein was removed and detected by 1D SDS-PAGE. The sample size was 15 µg per pore, the concentration of the separating gel was 12%, and Coomassie brilliant blue staining was performed. The protein bands of each sample are consistent, the removal effect of high abundance protein is clear, and there is no significant degradation of protein, which can be used for subsequent enzymatic hydrolysis and labeling experiments.
Enzymatic hydrolysis
According to the quantitative results, 100 µg protein samples were added to 200 µL Urea (6 M Urea + Tris 10% + Milli Q water) buffer, placed on a 10 kDa ultrafiltration tube, followed by 2 µL 1 M DTT, and placed at room temperature for 3 hours. Then, 10 µL 1 M IAA was added and reacted in the dark for 30 min at room temperature. After these operations, the protein is completely denatured, the two sulfur bond is opened, and the thiol group is closed. Thereafter, 200 µL 0.1 M TEAB was added to the system and centrifuged for 30 min at 20 °C and 14,000 g. The filtrate was subsequently discarded, and the membrane was washed three times with 200 µL 0.1 M TEAB buffer. The condition of the ultrafiltration tube was 4 °C, and centrifugation was performed at 14,000 g for 30 min. Finally, 0.1 M TEAB containing trypsin was added to the ultrafiltration tube according to the mass ratio (trypsin: protein =1:50). It was placed in a shaker at 37 °C for 16 hours. Enzyme was subsequently added to the ultrafiltration tube by adding protein =1:50 into the ultrafiltration tube, and 4H was the hydrolysate. After enzymatic hydrolysis, the mixture of peptide segments was centrifuged at 14,000 g to collect filtrate and stored at −80 °C for subsequent experiments.
iTRAQ in vitro isotope labeling
ITRAQ (Isobaric tags for relative and absolute quantification, iTRAQ) has eight channels (113, 114, 115, 116, 117, 118, 119, and 121, respectively); each channel labels a sample, of which 113, 117 channels are used to label mix internal reference samples. ITRAQ labeling steps: using the BCA method to quantify the peptide segment, 30 µg protein was added into the system with 15 µL 0.5 M TEAB and 50 µL isopropanol dissolution labeling reagent; the labeling reagent was subsequently added into the peptide segment and mixed at room temperature for 1.5 h of labeling; the reaction was terminated with 50 µL H2O for 30 min. Eight channel peptide segments in each group were mixed, and the merged samples were added to ddH2O, freeze-dried three times, and stored at −80 degrees.
High pH antiphase peptide grading
Peptide fragments were re-dissolved in 20 µL high pH reversed-phase A solution. The peptide fragments were classified on an Agilent 1290 high performance liquid chromatography system using a Waters XBridge BEH C18 column. The mobile phase A is 10 mM NH4FA, pH10, and the mobile phase B is 10 mM NH4FA, 90% ACN, pH10. The gradient of the gradient chromatography lasts 56 minutes, and one fraction is collected every 2 min. The first fraction is merged with the fifteenth fraction in the order of merging. Finally, 14 fractions are obtained and lyophilized for mass spectrometry analysis.
Multidimensional liquid chromatography tandem mass spectrometry (LC-MS/MS) analysis
All mass spectrometric analyses were carried out by a nanoFlash level high performance liquid chromatography tandem mass spectrometry system. The system consisted of an Easy-nLC 1000 high performance liquid chromatography system, an on-line mass spectrometry Orbitrap Elite system (Thermo Fisher Scientific, San Jose, CA) and a nano electrospray interface. The chromatographic column was a C18 reversed phase capillary column with a diameter of 75 m and a length of 15 cm. The flow rate was 250 nL/min, and the whole analysis time was 120 min/distillate. The spray voltage was 2.3 kV, and the heating capillary temperature was 300 °C. The mass spectrometry scan includes a full scan from 300 to 1,500 m/z, followed by 12 secondary scans (dynamic exclusion is 2 times, repetition time is 30 s) controlled by data mode. The full scan in resolution R=60,000 in the Orbitrap mass analyzer, with a two stage scan in the HCD mode after fragmentation in Orbitrap. To improve the quality accuracy, the real-time internal standard correction (“Lock Mass”) was carried out using m/z 445.120025 ions in the whole process. At the same time, the charge removal function excludes the univalent state and the ions that fail to distinguish valence states. All data collected were carried out based on the platform of Xcalibur (Thermo Fisher Scientific, San Jose, CA).
Database search and quantitative information extraction
The original file was generated using a MaxQuant mass spectrometry (1.3.0.5 Thermo Fisher Scientific, San Jose, CA) database search. The database is the human International Protein Index protein library (IPI Human, version 3.87). The following database search parameters were employed: cysteine residues on the carboxymethylation as a fixed modification; variable modifications, including iTRAQ 8plex marker protein N- terminal and lysine, methionine.
Statistical analysis
One way analysis of variance (ANOVA) and Tukey’s honestly significant difference (HSD) test were performed with language R. A P value <0.05 was defined as statistically significant. The clinical chemistry data are expressed as the mean ± SEM. Hierarchical clustering of proteins was performed on logarithmized data, using Euclidean distances and the Ward clustering method with the Package of “pheatmap” in language R. Correlations between samples were analyzed using the Spearman’s rank correlation coefficient.
Results
Patient characteristics
Table 1 summarizes the clinical characteristics of the 10 AMI patients (aged between 43 and 83 years, mean =62.30±12.61 years) and the 5 controls (aged between 54 and 81 years, mean =67.20±11.82 years) recruited. The baseline data in the two groups are shown in Table 1. There was no significant difference between the two groups with respect to sex, age, drinking, smoking, weight, height, hypertension, and diabetes mellitus.
Identification of significantly changed proteins in AMI group
In this study, the plasma samples from the healthy controls and AMI patients were subjected to LC-MS/MS analysis following the removal of high abundance protein, FASP preparation, tryptic digestion and iTRAQ labeling. With the false discovery rate (FDR) <1%, 468 proteins were identified by the iTRAQ experiments. The quality of the instrumental reproducibility and proteomic dataset was evaluated. As shown in Figure 1A, the box plot analysis showed that the log2 protein intensity medians of all pooled samples were approximately 1.25, nearly at the same levels across all samples, which suggests that there were no biases toward any samples. In addition, a correlation analysis was performed on the intensities between biological replicates inside each cohort or between different cohorts. Figure 1B shows that all correlation coefficients between two samples were greater than 0.95, which demonstrates good reproducibility of the biological replicates. Taken together, these results suggest that the iTRAQ-MS/MS analysis yielded a high-quality reproducible dataset. Filtering the iTRAQ data set using the criterion of a P value <0.05, we identified 33 significantly changed proteins by comparing the two groups. A heatmap analysis of the 33 differential proteins using an unsupervised hierarchical clustering and correlation distance metric was generated to depict the changes in the expression levels in different groups (Figure 1C).
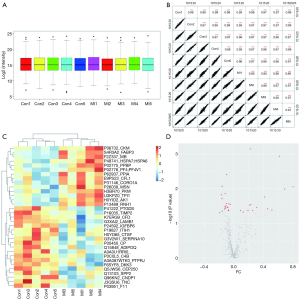
Among the 33 proteins, 12 protein spots showed a ≥1.5-fold change in abundance between the AMI and CON. These proteins included fatty acid binding protein3 (FABP3, ratio =6.36), creatine kinase-MB (CK-MB ratio =4.89), adenylate kinase1 (AK1 ratio =4.16), pro-platelet basic protein (PPBP ratio =3.29), creatine kinase (CK ratio=2.88), platelet factor 4 (PF4 ratio =2.62), peptidyl prolyl isomerase Cyclophilin A (PPIA ratio =2.05), Cofilin-1 (CFL1 ratio =1.81), coronin1A (CORO1A ratio =1.71), protein kinase M (PKM ratio =1.63), ribonuclease inhibitor (RNH1, ratio =1.67), and triose phosphate isomerase (TPI1 ratio =1.56). By contrast, there was a decrease of 19 proteins, such as adiponectin (ADIPOQ ratio =0.70), insulin-like growth factor binding protein6 (IGFBP6 ratio =0.70), Dickkopf-related protein 3 (DKK3 ratio =0.70) and complement 4B (C4B ratio =0.68).
Bioinformatic analysis of differentially expressed proteins
The biological processes of the proteins were explored by Gene Ontology (GO) annotation to understand the biological significance regarding the differentially expressed proteins in the AMI patients. In the cellular component category of GO, the most over-represented term is the regulation of cell proliferation (Figure 2A). The top 3 biological process terms were the regulation of cell proliferation, the response to wounding and wound healing.
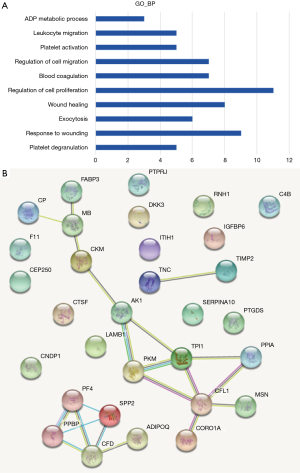
The protein protein interaction (PPI) network based on the STRING action scores was illustrated to understand the functional relationship among the 33 differential proteins between the AMI and CON groups. The annotations of the biological processes based on the GO analysis were also indicated in this view. The PPI analysis showed a complex network with several distinct biological subgroups that contained highly connected proteins. As shown in Figure 2B, proteins involved in immune proteins, blood coagulation, lipid metabolism, cytoskeleton proteins, energy metabolism, gene regulation, myocutaneous activity, and myocardial remodeling were highly connected with each other, which indicates that the functional network of these processes contributes to AMI pathophysiology.
Discussion
Using a plasma profiling proteomic approach, this study has provided insights into the potential mechanisms involved in AMI patients. In particular, the study demonstrates that immune proteins (C4B and PPIA), blood coagulation (PPBP and PF4), lipid metabolism (FABP3 and ADIPOQ), cytoskeleton proteins (coronin 1A), energy metabolism (AK1, PKM, and TPI1), gene regulation (RNH1), myocutaneous activity (CFL1), and myocardial remodeling (DKK3) play a role in the progression of myocardial infarction.
Among these proteins, some of them have been reported clinically, such as Fatty acid binding protein3 (FABP3) (8-10), Pro-platelet basic protein (PPBP) (11), and C4B (12-15). Our study validates these results and provides further clinical evidence for the application of these proteins in clinical practice. Although there are clinical reports for Human peptidyl prolyl isomerase Cyclophilin A (PPIA) and Adiponectin, the results of these trials are inconsistent. Cyclophilin A (CyPA), encoded by the peptidyl-prolyl isomerase A (PPIA) gene, was identified as the intracellular target of the immunosuppressive drug cyclosporine (16). Several studies have reported that CyPA in the plasma of CAD patients and CAD patients with type 2 diabetes mellitus are significantly increased, which indicated that CyPA might be a biomarker of CAD (17-20). But Palacín et al. reported the PPIA variation did not significantly contribute to the risk of suffering from AMI among patients with atherosclerotic diseased vessels (21). Adiponectin has been suggested as a potentially protective factor in CAD (22,23), potentially operating by reducing hepatic triglyceride accumulation and improving insulin sensitivity. A number of animal experiments and other basic studies have proven that adiponectin can protect against myocardial ischemia, reduce myocardial ischemia-reperfusion injury and improve myocardial remodeling (24-27). However, the relation of adiponectin with CAD in clinical research is inconsistent (28). Some reports show positive relations between adiponectin and the risk of CAD reoccurrence (29,30), while a Mendelian randomization study, which includes more sensitivity analyses, showed no relationship between adiponectin and CAD (31). These discrepancies could be driven by confounding factors, such as obesity, which affect both adiponectin and CAD (32), or by selection bias in studies restricted to patients (33), making adjustment difficult and possibly incomplete. In our study, we found PPIA was substantially higher in the AMI patients than in the controls (ratio =2.05), adiponectin was decreased in AMI patients (ratio =0.70), which supports that PPIA was a potation biomarker and adiponectin was a protective factor of coronary artery disease.
The rest of the differential proteins we found have no clinical reports so far, but there are 3 proteins have some basic research support. Cofilin-1 (CFL1), as actin-depolymerizing factors, play an essential role in the dynamics of filaments (34). Other research reported that the gold nanoparticle-based immunoassay localized surface Plasmon-coupled fluorescence biosensor could exploit the potential of urine cofilin-1 as a single biomarker to predict cardiorenal syndrome among coronary care unit patients (35). Dickkopf-related protein 3 (DKK) is a secretory protein involved in vascular smooth muscle cell differentiation and the regulation of myocardial remodeling; however, little is known regarding its role in atherosclerosis. Yu et al. (36) used epidemiological and laboratory methods to verify the hypothesis that DKK3 has an anti-atherosclerotic effect. Further studies using cultured human endothelial cells in vitro showed that DKK3 can induce cell migration, which suggests that DKK3 has substantial therapeutic potential for atherosclerosis. Coronin 1A belongs to a family of evolutionary conserved actin binding proteins that regulate actin cytoskeleton-dependent processes, such as cytokinesis, migration, cell polarization, and phagocytosis (37-40). Moreover, to date, no research has focused on the function of coronin 1A in coronary artery disease. In response to acute cardiac allograft rejection, the actin-binding protein coronin 1A was upregulated more than threefold (41). Another study reported that coronin 1A plays a role in TNFα-induced endothelial cell apoptosis (42). Considering the various functions of coronin 1A in leukocytes, it may be worthwhile pursuing this work further by studying the role of coronin 1A in inflammation (43) and vascular diseases, such as atherosclerosis (44). However, to date, there has been no research regarding the relationship between those three proteins with CAD or AMI. Our research for the first time found the expression of cofilin-1 (ratio =1.81) and coronin 1A (ratio =1.71) in the plasma of patients with myocardial infarction was substantially higher than that of the control group, which suggests that they may be new biomarkers for myocardial infarction. We also found that the expression of DKK3 in the plasma of patients with AMI was down-regulated (ratio =0.70), which supported the anti-atherosclerosis effect of DKK3.
There are no reports on basic experiments and clinical trials for the rest 5 proteins, such as AK1, PKM, RNH1, TPI1 and IGFBP6. Among these proteins, Adenylate kinase (AK), which play an important role in regulating nuclear energy signaling and actin assembly/disassembly during cell movement and chemotaxis (45), was substantially higher in the plasma of patients with myocardial infarction than that of the control group (ratio =4.16). No studies have reported a relationship between AK1 and coronary heart disease to date. In our study, we found for the first time the expression of AK1 may be a new biomarker for myocardial infarction; however, substantially more experimental evidence is required to confirm it.
The many proteins discovered indicates that a multiple biomarker approach may prove more advantageous than a single biomarker. The range of proteins discovered, originate from all aspects of molecular mechanism including immune, blood coagulation, lipid metabolism also indicates areas of future research. These findings direct future molecular mechanistic studies towards investigating the immune response, blood coagulation, lipid metabolism and energy metabolism, as well as in the pathogenesis of the acute deterioration in these patients.
As this experiment is only a systematic screening for AMI biomarkers, one of the limitations is the small number of enrolled patients. Thus, further studies are required to verify the findings. Due to the limitation in case numbers, effective prognostic analysis also couldn’t be carried out. This gap in knowledge can be filled in the future, by collecting samples at different onset time, as well as follow-up work to identify the effective biomarkers of AMI.
Conclusions
In conclusion, we identified a series of differentially expressed proteins in myocardial infarction via proteomics, including immune proteins, blood coagulation proteins, lipid metabolism proteins, cytoskeleton proteins, energy metabolism proteins, gene regulation proteins, myocutaneous proteins, and myocardial remodeling proteins. Some of these proteins have been reported clinically, such as FABP3, PPBP, and C4B. Although there are clinical reports, the results of these trials are inconsistent, such as for PPIA and Adiponectin. For some proteins such as cofilin-1, coronin 1A, and DKK3, there are supporting experiments; however, there are no clinical reports associated with them. Moreover, there are no reports on basic experiments and clinical trials, such as for AK1, PKM, RNH1, TPI1 and IGFBP6. We suggest that these proteins can be considered potential biomarkers for AMI diagnosis. However, additional confirmatory studies are required with the hope that these candidate biomarkers can be applied to routine clinical practice.
Acknowledgments
Funding: Supported by Taizhou Science and Technology Project 131ky13 and Taizhou Science and Technology Project 1901ky03.
Footnote
Conflicts of Interest: The authors have no conflicts of interest to declare.
Ethical Statement: The authors are accountable for all aspects of the work in ensuring that questions related to the accuracy or integrity of any part of the work are appropriately investigated and resolved. The study was approved by the scientific ethics committee (K20130307, Taizhou hospital of Zhejiang Province, China). Written informed consent was provided by all participants and legal guardians prior to the commencement of this study.
References
- Oldgren J, Wallentin L, Grip L, et al. Myocardial damage, inflammation and thrombin inhibition in unstable coronary artery disease. Eur Heart J 2003;24:86-93. [Crossref] [PubMed]
- Newby LK, Goldmann BU, Ohman EM. Troponin: an important prognostic marker and risk-stratification tool in non-ST-segment elevation acute coronary syndromes. J Am Coll Cardiol 2003;41:31S-36S. [Crossref] [PubMed]
- Collet JP, Montalescot G, Vicaut E, et al. Acute release of plasminogen activator inhibitor-1 in ST-segment elevation myocardial infarction predicts mortality. Circulation 2003;108:391-4. [Crossref] [PubMed]
- Vivanco F, Martin-Ventura JL, Duran MC, et al. Quest for novel cardiovascular biomarkers by proteomic analysis. J Proteome Res 2005;4:1181-91. [Crossref] [PubMed]
- Navas-Carrillo D, Marin F, Valdes M, et al. Deciphering acute coronary syndrome biomarkers: High-resolution proteomics in platelets, thrombi and microparticles. Crit Rev Clin Lab Sci 2017;54:49-58. [Crossref] [PubMed]
- Mateos-Cáceres PJ, Garcia-Mendez A, Lopez FA, et al. Proteomic analysis of plasma from patients during an acute coronary syndrome. J Am Coll Cardiol 2004;44:1578-83. [Crossref] [PubMed]
- Kopetz VA, Penno MA, Hoffmann P, et al. Potential mechanisms of the acute coronary syndrome presentation in patients with the coronary slow flow phenomenon - insight from a plasma proteomic approach. Int J Cardiol 2012;156:84-91. [Crossref] [PubMed]
- Bank IE, Dekker MS, Hoes AW, et al. Suspected acute coronary syndrome in the emergency room: Limited added value of heart type fatty acid binding protein point of care or ELISA tests: The FAME-ER (Fatty Acid binding protein in Myocardial infarction Evaluation in the Emergency Room) study. Eur Heart J Acute Cardiovasc Care 2016;5:364-74. [Crossref] [PubMed]
- Başar O, Akbal E, Koklu S, et al. Increased H-FABP concentrations in nonalcoholic fatty liver disease. Possible marker for subclinical myocardial damage and subclinical atherosclerosis. Herz 2013;38:417-22. [Crossref] [PubMed]
- Bivona G, Agnello L, Bellia C, et al. Diagnostic and prognostic value of H-FABP in acute coronary syndrome: Still evidence to bring. Clin Biochem 2018;58:1-4. [Crossref] [PubMed]
- Maneerat Y, Prasongsukarn K, Benjathummarak S, et al. PPBP and DEFA1/DEFA3 genes in hyperlipidaemia as feasible synergistic inflammatory biomarkers for coronary heart disease. Lipids Health Dis 2017;16:80. [Crossref] [PubMed]
- Palikhe A, Lokki ML, Saikku P, et al. Association of Chlamydia pneumoniae infection with HLA-B*35 in patients with coronary artery disease. Clin Vaccine Immunol 2008;15:55-9. [Crossref] [PubMed]
- Palikhe A, Sinisalo J, Seppanen M, et al. Human MHC region harbors both susceptibility and protective haplotypes for coronary artery disease. Tissue Antigens 2007;69:47-55. [Crossref] [PubMed]
- Arason GJ, Kramer J, Blasko B, et al. Smoking and a complement gene polymorphism interact in promoting cardiovascular disease morbidity and mortality. Clin Exp Immunol 2007;149:132-8. [Crossref] [PubMed]
- Kramer J, Rajczy K, Hegyi L, et al. C4B*Q0 allotype as risk factor for myocardial infarction. BMJ 1994;309:313-4. [Crossref] [PubMed]
- Handschumacher RE, Harding MW, Rice J, et al. Cyclophilin: a specific cytosolic binding protein for cyclosporin A. Science 1984;226:544-7. [Crossref] [PubMed]
- Satoh K. Cyclophilin A in cardiovascular homeostasis and diseases. Tohoku J Exp Med 2015;235:1-15. [Crossref] [PubMed]
- Ramachandran S, Venugopal A, Kutty VR, et al. Plasma level of cyclophilin A is increased in patients with type 2 diabetes mellitus and suggests presence of vascular disease. Cardiovasc Diabetol 2014;13:38. [Crossref] [PubMed]
- Satoh K, Fukumoto Y, Sugimura K, et al. Plasma cyclophilin A is a novel biomarker for coronary artery disease. Circ J 2013;77:447-55. [Crossref] [PubMed]
- Ohtsuki T, Satoh K, Omura J, et al. Prognostic Impacts of Plasma Levels of Cyclophilin A in Patients With Coronary Artery Disease. Arterioscler Thromb Vasc Biol 2017;37:685-93. [Crossref] [PubMed]
- Palacín M, Rodriguez I, Garcia-Castro M, et al. A search for cyclophilin-A gene (PPIA) variation and its contribution to the risk of atherosclerosis and myocardial infarction. Int J Immunogenet 2008;35:159-64. [Crossref] [PubMed]
- Hui X, Lam KS, Vanhoutte PM, et al. Adiponectin and cardiovascular health: an update. Br J Pharmacol 2012;165:574-90. [Crossref] [PubMed]
- Pischon T, Girman CJ, Hotamisligil GS, et al. Plasma adiponectin levels and risk of myocardial infarction in men. JAMA 2004;291:1730-7. [Crossref] [PubMed]
- Antonopoulos AS, Tousoulis D, Antoniades C, et al. Genetic variability on adiponectin gene affects myocardial infarction risk: the role of endothelial dysfunction. Int J Cardiol 2013;168:326-30. [Crossref] [PubMed]
- Zhang Y, Wang XL, Zhao J, et al. Adiponectin inhibits oxidative/nitrative stress during myocardial ischemia and reperfusion via PKA signaling. Am J Physiol Endocrinol Metab 2013;305:E1436-43. [Crossref] [PubMed]
- Dębiński M, Buszman PP, Milewski K, et al. Intracoronary adiponectin at reperfusion reduces infarct size in a porcine myocardial infarction model. Int J Mol Med 2011;27:775-81. [Crossref] [PubMed]
- Shibata R, Ouchi N, Murohara T. Adiponectin and cardiovascular disease. Circ J 2009;73:608-14. [Crossref] [PubMed]
- Hao G, Li W, Guo R, et al. Serum total adiponectin level and the risk of cardiovascular disease in general population: a meta-analysis of 17 prospective studies. Atherosclerosis 2013;228:29-35. [Crossref] [PubMed]
- Au Yeung SL, Schooling CM. Adiponectin and coronary artery disease risk: A bi-directional Mendelian randomization study. INT J CARDIOL 2018;268:222-6. [Crossref] [PubMed]
- Sook Lee E, Park SS, Kim E, et al. Association between adiponectin levels and coronary heart disease and mortality: a systematic review and meta-analysis. Int J Epidemiol 2013;42:1029-39. [Crossref] [PubMed]
- Borges MC, Hartwig FP, Oliveira IO, et al. Is there a causal role for homocysteine concentration in blood pressure? A Mendelian randomization study. Am J Clin Nutr 2016;103:39-49. [Crossref] [PubMed]
- Borges MC, Oliveira IO, Freitas DF, et al. Obesity-induced hypoadiponectinaemia: the opposite influences of central and peripheral fat compartments. Int J Epidemiol 2017;46:2044-55. [Crossref] [PubMed]
- Ortega Moreno L, Copetti M, Fontana A, et al. Evidence of a causal relationship between high serum adiponectin levels and increased cardiovascular mortality rate in patients with type 2 diabetes. Cardiovasc Diabetol 2016;15:17. [Crossref] [PubMed]
- Chatzifrangkeskou M, Yadin D, Marais T, et al. Cofilin-1 phosphorylation catalyzed by ERK1/2 alters cardiac actin dynamics in dilated cardiomyopathy caused by lamin A/C gene mutation. Hum Mol Genet 2018;27:3060-78. [Crossref] [PubMed]
- Chen HY, Chou C, Chang CH, et al. Urine Cofilin-1 Detection for Predicting Type 1 Cardiorenal Syndrome in the Coronary Care Unit: A Gold Nanoparticle- and Laser-Based Approach. Cardiorenal Med 2018;8:302-10. [Crossref] [PubMed]
- Yu B, Kiechl S, Qi D, et al. A Cytokine-Like Protein Dickkopf-Related Protein 3 Is Atheroprotective. Circulation 2017;136:1022-36. [Crossref] [PubMed]
- Yan M, Di Ciano-Oliveira C, Grinstein S, et al. Coronin function is required for chemotaxis and phagocytosis in human neutrophils. J Immunol 2007;178:5769-78. [Crossref] [PubMed]
- Gatfield J, Albrecht I, Zanolari B, et al. Association of the leukocyte plasma membrane with the actin cytoskeleton through coiled coil-mediated trimeric coronin 1 molecules. Mol Biol Cell 2005;16:2786-98. [Crossref] [PubMed]
- Yan M, Collins RF, Grinstein S, et al. Coronin-1 function is required for phagosome formation. Mol Biol Cell 2005;16:3077-87. [Crossref] [PubMed]
- Maniak M, Rauchenberger R, Albrecht R, et al. Coronin involved in phagocytosis: dynamics of particle-induced relocalization visualized by a green fluorescent protein Tag. Cell 1995;83:915-24. [Crossref] [PubMed]
- Kienzl K, Sarg B, Golderer G, et al. Proteomic profiling of acute cardiac allograft rejection. Transplantation 2009;88:553-60. [Crossref] [PubMed]
- Kim GY, Kim H, Lim HJ, et al. Coronin 1A depletion protects endothelial cells from TNFalpha-induced apoptosis by modulating p38beta expression and activation. Cell Signal 2015;27:1688-93. [Crossref] [PubMed]
- Moriceau S, Kantari C, Mocek J, et al. Coronin-1 is associated with neutrophil survival and is cleaved during apoptosis: potential implication in neutrophils from cystic fibrosis patients. J Immunol 2009;182:7254-63. [Crossref] [PubMed]
- Hölttä-Vuori M, Vainio S, Kauppi M, et al. Endosomal actin remodeling by coronin-1A controls lipoprotein uptake and degradation in macrophages. Circ Res 2012;110:450-5. [Crossref] [PubMed]
- Kuehnel MP, Reiss M, Anand PK, et al. Sphingosine-1-phosphate receptors stimulate macrophage plasma-membrane actin assembly via ADP release, ATP synthesis and P2X7R activation. J Cell Sci 2009;122:505-12. [Crossref] [PubMed]