Predicting in-hospital rupture of type A aortic dissection using Random Forest
Introduction
Type A aortic dissection (TAAD), referring to aortic dissection (AD) involving the ascending thoracic aorta, is a highly lethal event necessitating emergency surgery in the vast majority of cases to ensure survival (1). In China, total arch replacement combined with stented elephant trunk is standard therapy for TAAD (2). The entire procedure, often lasting 5–10 hours, is technically demanding and physically exhausting, resulting in only a small number of specialized thoracic aortic teams capable of performing it. As a result, some patients do not receive timely surgical treatment even when they are admitted in-hospital. Furthermore, existing studies suggest that the incidence of thoracic AD has increased from 2–3.5/100,000/year to more than 6/100,000/year in the general population, and up to 15/100,000/year in older individuals (3-5). Additionally, the occurrence of AD exhibits significant chronological and climatic variations, leading to the abrupt admission of a large number of patients over a short period of time (6,7). These factors further put a strain on meagre medical resources.
In the aforementioned scenarios, arranging operations and informing patients of the management strategy becomes a very critical issue. Some patients die soon after admission, whereas others are relatively “safer”. We hope to identify the patients who are extremely unstable and give them treatment priority, thus making the most efficient use of limited medical resources. There have already been some studies that try to predict postoperative mortality in TAAD patients (8,9). Unfortunately, there is currently no study specifically investigating the risk of in-hospital rupture in TAAD patients. Being the largest cardiovascular center in China, we have accumulated a substantial clinical experience in the diagnosis and treatment of TAAD. We have observed clinically that patients who suffered rupture prior to surgery had various comparable characteristics, especially imaging features. Accordingly, this study is aimed at identifying predictors and establish prediction model for in-hospital rupture in TAAD patients, to assist clinicians for optimal treatment planning using Random Forest, a classic algorithm of machine learning (10).
Methods
Study design and data collection
This retrospective cohort study, as part of our ongoing registered national AD investigation on Chinese Clinical Trial Registry (ChiCTR1800015338), is being reported in line with Strengthening The Reporting Of Cohort Studies in Surgery (STROCSS) guidelines (11). The study was approved by the Chinese ethics committee, with informed consent not required (reference number: ChiECRCT-20180041).
A total of 1,133 consecutive patients with TAAD were enrolled between January 2010 and December 2016. We then divided them randomly into training and testing datasets, in a 70:30 ratio, with 799 patients being assigned to the training cohort and 334 to the testing cohort. Diagnosis of TAAD was confirmed by the presence of an intimal flap on CT scan, and rupture was confirmed based on autopsy and/or CT scan. The data collected included demographic information, medical history, clinical presentation, laboratory tests, imaging findings (CT and echocardiography), and patient outcomes. Information on these patients was collected exclusively from the database of electronic medical records, laboratory test reports, and echocardiography reports. All CT scans were revisited and read, analyzed, and reported by two experienced radiological experts blinded to the study purpose. CT scan features that we hypothesized may predict in-hospital rupture are shown in Figure 1, and include DeBakey classification, hemopericardium, aortic size/AHI, periaortic hematoma, pleural effusion, pulmonary opacification, and branch vessels involvement.
Data conversion was carried out for age, which was divided by 10. In this way, the impact of age could be assessed per 10 units. Aortic height index (AHI) was calculated by aortic size as a function of height (AHI = aortic size/height). Periaortic hematoma indicated the collection of fluid around the aorta at or near the site of the AD.
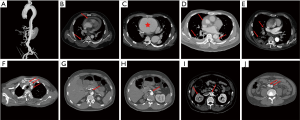
Statistical analysis
Continuous variables were expressed as mean with standard deviation (SD) and were tested for normality distribution with the Kolmogorov-Smirnov test. Independent t-tests were performed for normally distributed variables, or Mann-Whitney U tests otherwise. Categorical variables were presented as frequencies with percentages, and analyzed by Chi-square test or Fisher’s exact test, as appropriate.
As one of the classic algorithms of machine learning, Random Forest has high accuracy in disease risk prediction and diagnosis. Random Forest is an algorithm that does classification or regression by combining the voting results of multiple decision trees. The specific model construction process is as follows: (I) assuming that the Random Forest uses K trees, each tree needs a certain number of sample sets to train. The sample set is randomly generated by the Bootstrapping resampling/Bagging method; (II) N is used to represent the number of original training sets, and M is the number of features. For each tree node, m features are randomly selected, where m should be much smaller than M. The best splitting method is calculated based on m features using Gini coefficient; (III) through Bagging, about 36.8% of the data is not sampled, which is called out-of-bag (OOB) data. These data are not fitted to the training model. So, we can use OOB error to detect generalization ability of the model. The number of decision trees constructed in this study was 500, and three variables were randomly selected on each decision tree node. The Random Forest selected or excluded variables according to the feature importance. The confirmed variables were used to create a simplified model instead of a full model with all variables. The model was then verified in the training dataset and testing dataset, respectively, with following parameters as the assessment tool: area under curve (AUC)/C-static, accuracy, sensitivity, specificity, positive predicative value, and negative predictive value.
In addition, given the outstanding feature selection capabilities of the least absolute shrinkage and selection operator (Lasso), we also performed a Lasso regression and compared the results to Random Forest. Lasso is a regression analysis method for simultaneous feature selection and regularization. It adds a L1 norm as a penalty in the calculation of the minimum residual sum of squares (RSS). When the lambda is large enough, some coefficients can be accurately shrinked to zero. Tuning parameter λ (0.0261) of 1 standard error to the minimum was determined with cross-validation in this study.
R software (version 3.5.1) was used for data analysis. R packages “randomforest”, “Boruta”, and “caret” were used to develop and validate Random Forest model. The package “glmnet” was used for Lasso regression. To facilitate the application of the prediction model, we developed a web page based on the Random Forest using Flask (version 1.0.2). Two tailed P<0.05 indicated statistical significance.
Results
Table 1 summarizes TAAD patients’ characteristics in the total population (n=1,133). The mean age of the cohort was 49.5±11.7 years, with the majority being male (75.3%). Clinical characteristics that showed a significant association with in-hospital rupture on univariate analysis included advanced age (P<0.001), syncope (P<0.001), acute thoracic/back pain (P=0.051), lower limb numbness/pain (P<0.001), smoking (P=0.001), and acute phase of the TAAD (P<0.001). Risk factors such as dyslipidemia (P=0.011) and MFS (P=0.037) were also more frequent in patients who died than in those who survived. The presence of acute liver dysfunction (P<0.001), acute renal dysfunction (P=0.039), WBC >15×109 (P=0.002), DeBakey type I AD (P=0.005), greater aortic size (P=0.002), greater AHI (P=0.002), periaortic hematoma (P<0.001), reduced EF (P=0.023), pleural effusion (P<0.001), brachiocephalic artery involvement (P=0.006), and hemopericardium (P<0.001) were also associated with higher in-hospital rupture rates. Despite a temporal disconnect, baseline characteristics were basically comparable in both the training (Table 2) and testing cohorts (Table 3), which were consistent with the overall population.
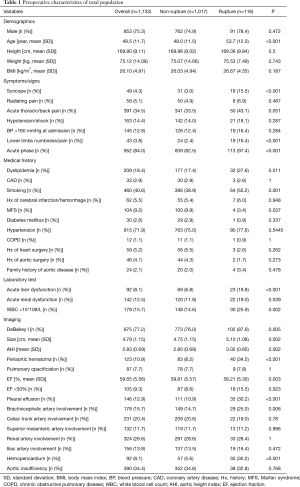
Full table
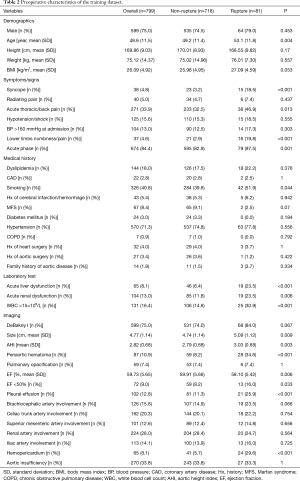
Full table
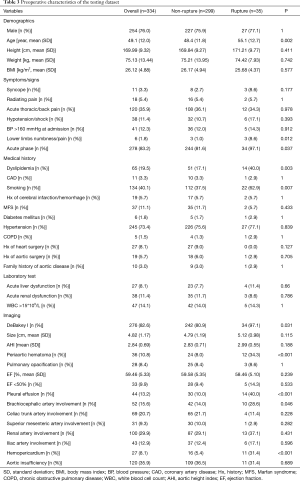
Full table
The process and results of feature selection by Random Forest are shown in Figure 2, which identified 16 important variables predisposing to in-hospital rupture in TAAD patients: periaortic hematoma, hemopericardium, lower limbs numbness/pain, syncope, AHI, size, pleural effusion, age, acute phase, brachiocephalic artery involvement, acute liver dysfunction, gender, WBC >15×109/L, BP >160 mmHg at admission, renal artery involvement, and BMI. To consolidate the results of Random Forest feature selection, we performed a Lasso regression which is depicted in Figure 3. Unsurprisingly, due to the strong shrinkage capability of Lasso regression, only 11 variables were left in the Lasso regression model, much less than in the Random Forest: AHI, syncope, acute thoracic/back pain, lower limbs numbness/pain, acute phase, acute liver dysfunction, WBC >15×109, periaortic hematoma, pleural effusion, and hemopericardium. From among these variables, except for acute thoracic/back pain, the remaining 10 variables were exactly overlapping with the confirmed variables in Random Forest.
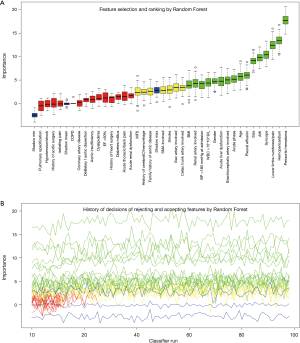
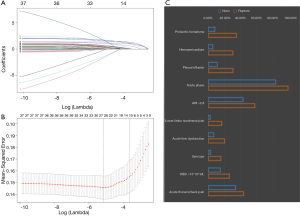
We then developed a prediction model with the 16 confirmed important risk factors selected by the Random Forest. OOB error for the Random Forest model was 7.88%, meaning the generalization error is quite small. In internal validation of training dataset, the ROC showed that the resulting model had a perfect discrimination with AUC of 0.994 (Figure 4A). In the independent testing cohort, the model displayed a decreased but satisfactory discrimination with an AUC of 0.752 (Figure 4B). Good discrimination was also demonstrated by validation, with an accuracy, sensitivity, specificity, positive predictive value and negative predictive value of 0.998, 0.994, 1.000, 0.987, 0.998 and 1.000, respectively, in the training dataset, and 0.940, 0.752, 0.990, 0.514, 0.945 and 0.857, respectively in the testing dataset. The model is further exhibited as a web calculator to facilitate its application (http://47.107.228.109/).
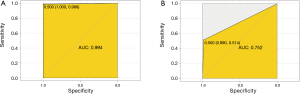
Discussion
Somewhat intriguingly, aortic dissection accounts for the vast majority of aortic diseases for Asians, just the opposite to Caucasians. Up to 1,133 patients were documented in our single-center database over a 7-year period. What’s worse, due to its high demand on surgeon’s technique and specialized teamwork, only a small number of surgeons from experienced centers can perform aortic surgery. Although we doubt that any delaying surgery in acute TAAD could ever be an accepted strategy, we have to face the excruciating question as to who would be operated first frequently, unfortunately and reluctantly. To make such a choice is often arbitrary and could be fatally wrong without an objective scoring system.
This study demonstrated several clinical variables that can significantly predict in-hospital rupture in patients with TAAD: age, BMI, gender, syncope, lower limbs numbness/pain, acute phase of the TAAD, BP >160 mmHg at admission, acute liver dysfunction, WBC >15×109/L,aortic diameter, AHI, periaortic hematoma, pleural effusion, brachiocephalic artery involvement, renal artery involvement, and hemopericardium. We also provide a simple risk prediction tool (http://47.107.228.109/) with powerful a discriminatory ability that can be used to help make a clinical decision regarding management and patient counseling. For patients with a high risk of in-hospital rupture, prioritizing surgical treatment should be considered strongly. A “life-saving first” strategy may also be applied, such as simple ascending aortic replacement, hemi-arch replacement, or hybrid surgery, instead of extensive aortic surgery like total arch replacement with deep hypothermic circulatory arrest.
The strongest predictor identified in this study by Random Forest was periaortic hematoma. Periaortic hematoma is believed to be caused by slow oozing of blood from a dissected and dilated aorta, and portends impending catastrophic rupture. As shown in Figure 1D, it is a completely distinct entity from a pseudoaneurysm or intramural hematoma. Ascending aortic periaortic hematoma is often associated with extension into the pericardial space, causing pericardial effusion and eventually tamponade, which may play a role in the frequent presence of hemopericardium in the cohort of patients who died before surgery. Similarly, periaortic hematoma complicating descending aortic dissection may extend into the pleural space, explaining the significantly greater incidence of pleural effusions in this cohort. Mukherjee and colleagues (12) also demonstrated that patients with periaortic hematomas were more likely to have shock, cardiac tamponade, coma, and/or an altered state of consciousness. Accordingly, we strongly recommend early aggressive intervention in TAAD patients with periaortic hematoma.
Greater aortic size was found to be related to a higher probability of in-hospital rupture in this study. We speculate that greater size denotes greater pressure, and a thinner and more fragile aortic wall. We further explored the impact of AHI on patients’ survival. It has been demonstrated that indexing aortic dimensions to patient stature is a better determining factor than purely size for prophylactic intervention in patients with thoracic aortic aneurysm (TAA) (13). The AHI, recently proposed by Drs. Zafar and Elefteriades (14), was shown to be a more reliable predictor for adverse aortic events than the aortic size index (aortic size indexed to body surface area), given that weight may fluctuate over time and that height is genetically predetermined and may be more closely correlated to aortic size. Although AHI was originally proposed to stratify risk of dissection/rupture for patients with TAA, we also found it to be a stronger predictor than raw aortic size for in-hospital rupture in patients with TAAD.
Apart from radiographic imaging, simple clinical information such as syncope, lower limb numbness/pain, and time from onset can be very useful in stratifying a patient’s risk. This is consistent with other studies focusing on overall postoperative mortality (15). Among these presenting symptoms, syncope is a well-recognized symptom that had a significant association with in-hospital death. It may result from cardiac tamponade and/or great vessel involvement. We found that there was indeed a higher percentage of brachiocephalic artery involvement in the cohort of patients who died. However, the relationship between branch artery involvement and visceral ischemia in this study was not very clear, since we observed a significantly increased occurrence of acute liver dysfunction and renal dysfunction in patients who died, but did not find concomitantly increased rates of visceral artery involvement by aortic dissection. More work is required in this regard in the future with a more detailed and refined classification of branch artery involvement to understand this paradox.
We explored the value of WBC count as a predictor for in-hospital rupture and found that WBC >15×109/L indeed predisposed to a bad prognosis for TAAD patients. It has been well-known that inflammatory factors contribute to medial degeneration and remodeling of the aortic wall (16). Inflammatory cells such as lymphocytes and macrophages have been detected in medial degeneration (17). Nonspecific inflammatory markers such as WBCs and C reactive protein (CRP) have been proposed as diagnostic biomarkers (18). Luo et al. pointed out that patients with serious clinical syndromes and advanced disease had a higher inflammatory cell activity in the aortic wall than asymptomatic and clinically stable patients (19). We demonstrated that inflammation was not only involved in the pathogenesis of TAAD, but also played a role in the outcome. Future work is still needed to determine the best cutoff of the WBC count as a predictor, since we took WBC >15×109/L as a diagnosis cutoff just based on our clinical experience.
This is the first attempt in aortic surgery to use Random Forest as a prediction tool. Traditionally, research regarding risk factors or predictive models typically performed univariate regression followed by multivariate logistic regression. Random Forest was proposed by Breiman in 2001 and gained attention quickly due to its superior performance (20). It is basically a collection of multiple decision trees. It improves prediction accuracy without significantly increasing the amount of computation. In contrast to traditional logistic regression, Random Forest is not sensitive to multicollinearity, and the results are relatively robust to missing data. It can predict well the effects of up to several thousand explanatory variables. Dr. Fernández-Delgado evaluated 179 classifiers arising from 17 families (Random Forests, neural networks, logistic regression etc.) which included all the relevant classifiers available today using 121 datasets. The Random Forest was clearly the best family of classifiers, achieving 94.1% of the maximum accuracy in the 84.3% of the datasets (21). Large-scale multi-center clinical research has become a trend, and logistic regression may not be suitable in the setting of big data. Therefore, we recommend new classifiers of machine learning represented by Random Forest in the upcoming research with a similar study purpose.
Limitations
As discussed before, some variables were put forward based on our own clinical experience such WBC >15×109/L, pleural effusion, brachiocephalic artery involvement, etc. They have been proven to have good predictive value. However, these indicators can be further divided into different levels according to severity to better explore their impacts on the outcomes. Also, this study was also subject to the inherent shortcomings of retrospective studies.
Conclusions
- An easy-to-use tool to predict the risk of in-hospital rupture in patients with TAAD was developed and validated using Random Forest, which can assist surgeons in better ascertaining the urgency and extent of surgical correction of emergently presenting TAAD (http://47.107.228.109/);
- Periaortic hematoma is the strongest predictor for in-hospital rupture in patients with TAAD;
- Simple clinical information such as syncope, lower limbs numbness/pain can be very useful in stratifying a TAAD patient’s risk of in-hospital rupture;
- Inflammation was not only involved in the pathogenesis of TAAD, but also played a role in the outcome.
Acknowledgments
We thank Da Xu sincerely for the development and maintenance of the Random Forest based website calculator.
Funding: This study was supported by CAMS Initiative for Innovative Medicine (CAMS-I2M). Identification number: 2016-I2M-1-016.
Footnote
Conflicts of Interest: The authors have no conflicts of interest to declare.
Ethical Statement: The authors are accountable for all aspects of the work in ensuring that questions related to the accuracy or integrity of any part of the work are appropriately investigated and resolved. The study was approved by the Chinese ethics committee, with informed consent not required (reference number: ChiECRCT-20180041).
References
- Coady MA, Rizzo JA, Goldstein LJ, et al. Natural history, pathogenesis, and etiology of thoracic aortic aneurysms and dissections. Cardiol Clin 1999;17:615-35. vii. [Crossref] [PubMed]
- Sun L, Qi R, Zhu J, et al. Total arch replacement combined with stented elephant trunk implantation: a new "standard" therapy for type a dissection involving repair of the aortic arch? Circulation 2011;123:971-8. [Crossref] [PubMed]
- Mészáros I, Mórocz J, Szlávi J, et al. Epidemiology and clinicopathology of aortic dissection. Chest 2000;117:1271-8. [Crossref] [PubMed]
- Olsson C, Thelin S, Stahle E, et al. Thoracic Aortic Aneurysm and Dissection: Increasing Prevalence and Improved Outcomes Reported in a Nationwide Population-Based Study of More Than 14 000 Cases From 1987 to 2002. Circulation 2006;114:2611-8. [Crossref] [PubMed]
- Melvinsdottir IH, Lund SH, Agnarsson BA, et al. The incidence and mortality of acute thoracic aortic dissection: results from a whole nation study. Eur J Cardiothorac Surg 2016;50:1111-7. [Crossref] [PubMed]
- Benouaich V, Soler P, Gourraud PA, et al. Impact of meteorological conditions on the occurrence of acute type A aortic dissections. Interact Cardiovasc Thorac Surg 2010;10:403-6. [Crossref] [PubMed]
- Takagi H, Ando T, Umemoto T, et al. Meta-Analysis of Seasonal Incidence of Aortic Dissection. Am J Cardiol 2017;120:700-7. [Crossref] [PubMed]
- Tolenaar JL, Froehlich W, Jonker FH, et al. Predicting In-Hospital Mortality in Acute Type B Aortic Dissection: Evidence From International Registry of Acute Aortic Dissection. Circulation 2014;130:S45-50. [Crossref] [PubMed]
- Leontyev S, Légaré JF, Borger MA, et al. Creation of a Scorecard to Predict In-Hospital Death in Patients Undergoing Operations for Acute Type A Aortic Dissection. Ann Thorac Surg 2016;101:1700-6. [Crossref] [PubMed]
- Genuer R, Poggi JM, Tuleau-Malot C. Variable selection using random forests. Pattern Recogn Lett 2010;31:2225-36. [Crossref]
- Agha RA, Borrelli MR, Vella-Baldacchino M, et al. The STROCSS statement: Strengthening the Reporting of Cohort Studies in Surgery. Int J Surg 2017;46:198-202. [Crossref] [PubMed]
- Mukherjee D, Evangelista A, Nienaber CA, et al. Implications of Periaortic Hematoma in Patients With Acute Aortic Dissection (from the International Registry of Acute Aortic Dissection). Am J Cardiol 2005;96:1734-8. [Crossref] [PubMed]
- Evangelista A, Hutchison S, Montgomery D, et al. Aortic size indices: a more comprehensive evaluation of aortic risk. J Am Coll Cardiol 2014;63:A1209. [Crossref]
- Zafar MA, Li Y, Rizzo JA, et al. Height alone, rather than body surface area, suffices for risk estimation in ascending aortic aneurysm. J Thorac Cardiovasc Surg 2018;155:1938-50. [Crossref] [PubMed]
- Rampoldi V, Trimarchi S, Eagle KA, et al. Simple Risk Models to Predict Surgical Mortality in Acute Type A Aortic Dissection: The International Registry of Acute Aortic Dissection Score. Ann Thorac Surg 2007;83:55-61. [Crossref] [PubMed]
- del Porto F, Proietta M, Tritapepe L, et al. Inflammation and immune response in acute aortic dissection. Ann Med 2010;42:622-9. [Crossref] [PubMed]
- Kuehl H, Eggebrecht H, Boes T, et al. Detection of inflammation in patients with acute aortic syndrome: comparison of FDG-PET/CT imaging and serological markers of inflammation. Heart 2008;94:1472-7. [Crossref] [PubMed]
- Ranasinghe AM, Bonser RS. Biomarkers in acute aortic dissection and other aortic syndromes. J Am Coll Cardiol 2010;56:1535-41. [Crossref] [PubMed]
- Luo F, Zhou XL, Li JJ, et al. Inflammatory response is associated with aortic dissection. Ageing Res Rev 2009;8:31-5. [Crossref] [PubMed]
- Breiman L. Random Forests. Mach Learn 2001;45:5-32. [Crossref]
- Cernadas E, Amorim D. Do we need hundreds of classifiers to solve real world classification problems? J Mach Learn Res 2014;15:3133-81.