Identification of potential microRNAs and their targets in promoting gefitinib resistance by integrative network analysis
Introduction
Lung cancer is one of the most frequent and fatal neoplasms. It is also the leading cause of cancer mortality in all parts of the world (1), with around 80–85% of lung cancers being non-small cell lung cancer (NSCLC). Despite being the most prevalent type of lung cancer, NSCLC remains a global challenge because of its high morbidity and mortality (2). Recently, molecular targeted therapy has dramatically arrived as a milestone clinical improvement for patients with precise genomic variations, especially for those patients with NSCLC-harboring epidermal growth factor receptor (EGFR)-sensitive mutations (3,4). EGFR-TKIs have been developed to resist epithelial-derived solid tumors for advanced NSCLC.
One of these EGFR-tyrosine kinase inhibitors (TKIs), gefitinib, is a selective inhibitor of epidermal growth factor and plays an essential role in controlling cell growth, apoptosis, and angiogenesis (5). It functions as a first-line single therapeutic agent for NSCLC (6). Unfortunately, resistance to therapeutic EGFR-TKIs has developed, thus limiting the usefulness of gefitinib in clinical treatment (7). For example, Yonesaka et al. found that over-expression of HER3 could cause substantial resistance to EGFR-TKIs by stimulating the downstream PI3K/AKT signaling cascades (8). However, apart from the findings of few studies like the one above, little else is understood concerning the mechanism underlying gefitinib resistance or other developed resistances to EGFR-TKI.
MiRNAs generally bind to the 3'-untranslated regions (3'-UTRs) of target messenger RNAs (mRNAs) and cause either degradation of mRNA or inhibit translation of mRNA (9). The recent discovery of miRNAs in TKI resistance has revealed the role of non-coding RNA in gefitinib resistance in NSCLC. Garofalo et al. examined the importance of miR-30b, which was regulated by EGFR as well as MET receptor tyrosine kinases in NSCLC gefitinib resistance (10). Gao et al. explored the involvement of miR-138-5p in reversing the resistance to gefitinib in NSCLC (9). Recently, microarrays have been used to evaluate gene expression, demonstrating promising clinical application in cancer diagnosis and the predictive response of targeted drugs to tumor cells. They represent an innovative research approach to studying the molecular processes of therapeutic resistance in tumors (11-13).
The objective of our study was to identify likely miRNAs and their targets to promote the resistance to gefitinib in NSCLC. First, we obtained and integrated the Gene Expression Omnibus (GEO) datasets and conducted scientific bioinformatics analysis to build a gefitinib-resistance miRNA-target regulatory network. Then, functional enrichment was employed to identify the GO terms and pathways of this network. The hsa-miR-342-3p and its target CPA4 were selected. Finally, we found that enforced CPA4 expression partially reversed miR-342-3p effects in A549/GR cells. Thus, this study reveals the impact of hsa-miR-342-3p in gefitinib-resistant NSCLC and implicates hsa-miR-342-3p as an impending treatment option for improving the effectiveness of gefitinib in NSCLC patients.
Methods
Microarray data
NCBI-GEO is a free database for next-generation sequencing. In this study, to construct a gefitinib resistance-related network, we searched miRNA and mRNA datasets for gefitinib resistance in the GEO database (https://www.ncbi.nlm.nih.gov/geo). To ensure that the same samples were used in miRNA and mRNA datasets, three datasets, GSE74253, GSE117610, and GSE110815—all focusing on the PC9 cells—were finally selected. The sequencing data of GSE74253 and GSE117610 were based on the GPL11154 platform [Illumina HiSeq 2000 (Homo sapiens)] (11,12). The GSE74253 dataset was designed to compare the whole genome transcriptome of the gefitinib-resistant NSCLC cell line (PC9R) with its gefitinib-sensitive counterpart (PC9). The GSE117610 dataset was mainly used so that the NSCLC cell line PC9 could be made tolerant to gefitinib over 6 days. Finally, the GSE110815 dataset investigated the genome-wide miRNA expression analysis, which was performed in gefitinib-resistant sub-cell lines and gefitinib-sensitive parental cell lines, based on the GPL18402 platform [Agilent-046064 Unrestricted_Human_miRNA_V19.0_Microarray (miRNA ID version)] (13).
Identification of differentially expressed genes (DEGs)
The raw microarray data files of high throughput functional genomics expression were integrated for the analysis. The TXT format data were processed in the algorithm, and DEGs were identified. For the GSE74253 dataset, statistically significant DEGs were defined with a GFOLD value of above 1 and 6% of total detected genes. Additionally, a GFOLD value less than −1 and 5% of the total detected genes was used as a cut-off criterion. For the GSE117610 dataset, statistically significant DEGs were defined with P values <0.05, and |log2FC| >1 was set as the statistically significant threshold.
Functional and pathway enrichment analyses
Gene Ontology analysis (GO), an extremely valuable technique, is generally used for interpreting genes and gene products. It is also used to identify specific biological attributes for high-throughput genome or transcriptome data. Kyoto Encyclopedia of Genes and Genomes (KEGG) is a resource base for the methodical examination of gene functions, and it links genomic evidence with higher-order functional evidence. To investigate function annotation and pathway enrichment of the DEGs, the Database for Annotation, Visualization and Integrated Discovery (DAVID) was used to analyze the GO and KEGG enrichment analyses. Statistical significance was achieved when P<0.01.
The miRNA-target gene interactions
Seven databases including Targetscan (14), RNAhybrid (15), Rna22 (16), PicTar5 (17), mirBase (18), Miranda (19), and DIANA_microRNA (20) were searched to collect the miRNA-target gene interactions. Once the appropriate processing was completed to avoid any redundancy, 289,469 miRNA-target interactions amongst 15,185 genes and 1,089 miRNAs were acquired.
Cell culture
Human non-small cell adenocarcinoma cell line A549 and PC9 were purchased from the American Type Culture Collection (Manassas, VA, USA). The culture medium was treated with different doses of gefitinib to establish gefitinib-resistant A549/GR and PC9/GR cells. The range of dosages of gefitinib ranged from 0 to 80 nM, and the dosage gradient was 25–50% of the prior dose. The subsequent bout was provided up until the cells became steady in proliferation without any substantial death (5,21).
All cells were cultured by using either RPMI 1640 medium or DMEM (Life Technologies, Carlsbad, CA, USA). The medium contained 10% (v/v) fetal bovine serum (FBS; Gibco, Grand Island, NY, USA). Cells were incubated in an incubator with humidified air of 5% (v/v) CO2 at 37 °C.
Plasmid construction
As mentioned in previous studies, both wild-type and mutated CPA4 3'-UTRs were cloned into a dual-luciferase reporter vector (Promega, Madison, WI, USA) (22). Briefly, the 3'-UTR of the human CPA4 gene was acquired by performing genomic PCR. The primers used were as follows: 5'-CGGAATTCATCCAGATGGCAAATGACCA-3' (forward) and 5'-CCGCTCGAGTTAGCCCCCGTGACCTCTT-3' (reverse). Moreover, these two primers, along with the other two following primers, were used to conduct recombinant PCR to introduce alterations into the seed sequences of the projected miR-342-3p target sites within CPA4 3'-UTR: 5'-TTATTTTATGAAAGTGGGCACTTGTCAAAGTGATG-3' (forward) and 5'-CATCACTTTGACAAGTGCCCACTTTCATAAAATAA-3' (reverse). The miRNA-342-3p mimics or the antisense of miR-342-3p-5p (anti-miR-342-3p) and their analogous miRNA controls were constructed from RuiBo (Guangzhou, China). Scrambled and nontargeted irrelevant RNA sequences were used as negative controls. Anti- CPA4 (1:1,000; Cat. ab85206, ABCOM, Boston, MA, USA) and anti-GAPDH (sc-25778) antibodies were bought from Santa Cruz Biotechnology (Dallas, TX, USA). Gefitinib was bought from Sigma-Aldrich (St. Louis, MO, USA).
Luciferase reporter assay
A549 and PC9 cells were cultured in 24-well plates at a density of 1×103 cells/well. Next, the cells were co-transfected with luciferase reporters. Co-transfection was performed either with wild-type (WT) CPA4 (CPA4-WT), mutant CPA4 (CPA4-Mut), a combination with miR-342-3p mimic, or a negative control (NC) group using Lipofectamine 3000. Cells were collected, and a luciferase assay kit was used to measure luciferase activity (Promega, Madison, WI, USA) as per the supplier’s protocol after 48 h.
Reverse transcription-quantitative polymerase chain reaction (RT-qPCR)
Total RNA was isolated from A549 and PC9 cells by using Trizol reagent (Thermo Fisher Scientific, USA). Either the TaqMan MicroRNA Reverse Transcription Kit or TaqMan High-Capacity cDNA Reverse Transcription Kit (Thermo Fisher Scientific) was used to perform qRT-PCR. Power SYBR Green PCR master mix (Thermo Fisher Scientific, Inc.) was used to conduct qPCR for 35 cycles at 95 °C for 30 sec, 60 °C for 30 sec, and 72 °C for 35 sec. Gene expression levels were standardized with β-actin and evaluated using the 2−ΔΔCq method.
CCK-8 cell viability assay
A549 and PC9 cells were cultured into 96-well plates with a density of 2×104 cells/well. After 24 h, cells transfected with either miR-342-3p mimic or miR-342-3p inhibitor were treated with different concentrations of gefitinib (0, 2.5, 5, 10, 20, 40, and 80 nM) for another 24 hours. Cell counting kit-8 (CCK-8) (Dojindo, Kumamoto, Japan) was used to evaluate cell viability after 48-h treatment.
Acridine orange/ethidium bromide (AO/EB) staining
miR-342-3p mimic and miR-342-3p + CPA4 or control cells (1×105 cells) were seeded in 6-cm culture plates and treated with gefitinib (60 nM) for 24 h. The next day, the cells were treated for 5 mins with AO and EB mixing solution (Solarbio of Biotechnology, Shang Hai, China). Fluorescence microscopy was used to visualize morphological changes in cells at 200× magnification. Apoptotic cell percentage was determined with the following formula:
Apoptotic rate (%) = number of apoptotic cells/number of all cells counted ×100%.
Statistical data analysis
Data were obtained from 3 to 6 independent experiments. Results are represented as the mean ± standard deviation. Data were analyzed by unpaired Student’s t-test. Statistical comparisons among multiple groups were performed by analysis of variance (ANOVA) followed by Tukey’s multiple comparison test. Spearman’s rank correlation tests were used to analyze miR-342-3p and the IC50 of gefitinib. Statistical significance was achieved when P<0.05.
Results
Identification and functional characterization of upregulated DEGs in gefitinib-resistant NSCLC
In order to identify the candidate genes that confer gefitinib resistance in NSCLC, the GSE117610 dataset containing the gefitinib-resistant cells and their parental cells was obtained. Then, 1,690 DEGs were identified based on 2-fold change (Figure 1A). Out of these 1,690 DEGs, 595 were upregulated, and 1,095 were downregulated (Figure 1B). To get insights into the functions of these significant DEGs, we uploaded all DEGs on DAVID to recognize the overrepresented GO categories and KEGG pathways. This process identified the top 5 GO terms and enrichment pathways, and also indicated that DEGs were highly related to viral gene expression, translational initiation, and ribosomal subunits (Figure 1C). In addition, as shown in Figure 1D, the significant DEGs were also augmented in ribosome and oxidative phosphorylation (Figure 1D).
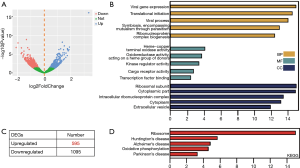
For the GSE74253 dataset, there were 1,487 significantly upregulated and 1,112 significantly downregulated genes (Figure 2A). After comparing the significant DEGs in GSE117610, the intersecting genes were obtained (Figure 2B). GO and KEGG results also indicated the significant DEGs of GSE74253 dataset were highly related to cell-cell adhesion and ECM-receptor interaction, along with cell adhesion molecules (CAMs) (Figure 2C,D).
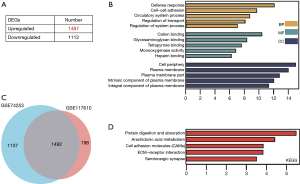
Construction of the miRNA-target regulatory network
To construct the miRNA-target differential regulatory network, differentially expressed miRNAs were first identified. From the GSE110815 dataset, 32 significantly upregulated and 44 downregulated miRNAs were recognized (Figure 3A,B).
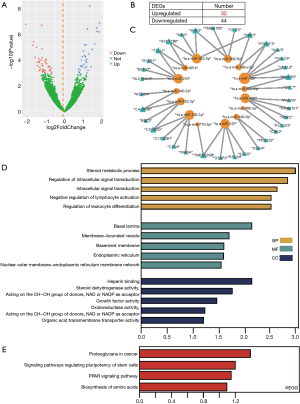
We then constructed all the terms of miRNA-target interactions included across the 7 databases. Only the miRNA-target associations recorded in more than 2 databases were retained. Then, we mapped the significant differentially expressed miRNAs and intersecting DEGs into the above miRNA-target interactions. As a result, 43 unique miRNA-target associations were finally obtained from 289469 primary terms. The 43 miRNA-target associations were composed of 15 miRNAs and 34 targets (Figure 3C). Finally, for the miRNA-target regulation network, the degrees of all miRNAs ranged from 1 to 5, while the degrees of all target genes ranged from 1 to 2. This suggests that miRNAs as regulators tend to deregulate more, and some miRNAs may act as important regulators for multiple target genes, including hsa-miR-128’s 5 regulation genes (POU2F3, HMGB3, FNDC4, YPEL3, NAV3). Meanwhile, some miRNAs may act as specific regulators to single genes, whereas some target genes may be regulated by multiple miRNAs. GO, and KEGG results showed these targets in the network were enriched into intracellular signal transduction and pathway of PPAR signaling along with proteoglycans in cancer (Figure 3D).
miR-342-3p enhances gefitinib resistance in A549 and PC9 cells
To examine the involvement of miR-342-3p in gefitinib resistance in NSCLC A549 and PC9 cells, we first established an NSCLC gefitinib resistance cell line (A549/GR and PC9/GR) (5,21) (Figure S1). We evaluated the effect of miR-342-3p in A549/GR and PC9/GR cells. Our data showed that increased expression of miR-342-3p significantly augmented the resistance to gefitinib of A549/GR and PC9/GR cells (Figure 4A,B). However, decreased expression of the miR-342-3p inhibited the viability of the A549/GR and PC9/GR cells that were treated with various dosages of gefitinib for 24 h compared to the NC group (Figure 4C,D). These data reveal that miR-342-3p might be an agent in gefitinib resistance in lung cancer cells.
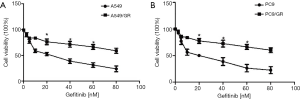
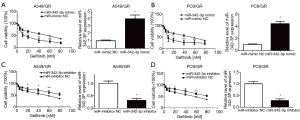
Identification of CPA4 as a target of hsa-miR-342-3p
To further confirm the association between CPA4 and miR-342-3p, we first inspected the manifestation of miR-342-3p after transfection with miR-342-3p mimic or inhibitor (Figure 5A). We then measured the mRNA level of CPA4 after miR-342-3p knockdown or overexpression in A549 and PC9 cells (Figure 5B,C). When compared to the NC group, the mRNA, along with the protein level of CPA4, was significantly up-regulated in the miR-342-3p inhibitor group and significantly down-regulated in the miR-342-3p mimic group (P<0.01). These data suggest that CPA4 is regulated by miR-342-3p (Figure 5D,E).
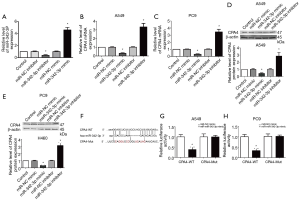
The bioinformatics analysis results showed that miR-342-3p contained the specific binding sequence of the 3'-UTR regions of the CPA4 gene (Figure 5F). A luciferase reporter assay showed that the expression of miR-342-3p diminished the luciferase activity of the 3'-UTR of CPA4. Nevertheless, the miR-342-3p-Mut did not inhibit the manifestation of CP4 on luciferase activity (Figure 5G,H).
Enforced CPA4 expression partially reversed miR-342-3p effects in A549/GR cells
To investigate if the involvement of miR-342-3p in A549/GR cells was through CPA4, a CPA4 expression vector was co-transfected with miR-342-3p. First, we found that the viability of A549/GR cells was reversed by AO/EB (Figure 6A,B). Meanwhile, we confirmed that the expression of miR-342-3p expression was restored by CPA4 expression vector co-transfection after treatment with or without gefitinib (Figure 6C).
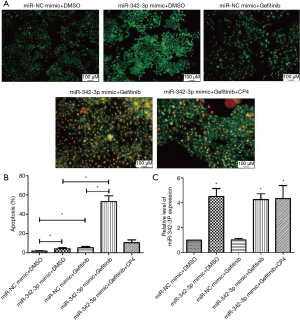
Discussion
Even though many effective types of medication are available to treat tumors via the targeting of various molecules, many patients still acquire permanent resistance towards certain treatments (22). NSCLC prognosis remains unfavorable since it is a systemic disease rendered uncontrollable by such drug resistance. This study identified miR-342-3p as a prospective molecule for conferring gefitinib resistance in NSCLC.
We firstly identified 1,690 DEGs from the GSE117610 dataset, and GO and KEGG pathway enrichment were implemented. Of these DEGS, those that were significant were found to be highly related to GO BP terms like viral gene expression, translational initiation, and ribosomal subunits (Figure 1C), along with pathways including ribosome and oxidative phosphorylation (Figure 1D). Animals use ATP generated from oxidative phosphorylation to maintain day-to-day activities; thus, it is accountable for preserving metabolic homeostasis (23). Abnormal metabolism is a hallmark of cancer, and aberrant phosphorylation in A549/GR was found to be related to the ineffectiveness of gefitinib (24-26). For the GSE74253 dataset, there were 1,487 significantly upregulated and 1,112 significantly downregulated genes (Figure 2A). The results obtained from GO and KEGG indicated that the significant DEGs were highly related to cell-cell adhesion, ECM-receptor interaction, and CAMs (Figure 2B,C). ECM-receptor interaction pathway was identified in crizotinib-resistant NSCLC cells and trastuzumab-resistant gastric cancer cells (27,28). Several cell-adhesion molecules are also recognized to be effective clinical biomarkers and are thus promising treatment options for lung, colorectal, and pancreatic cancer (29).
To construct the miRNA-target differential regulatory network, the differentially expressed miRNAs were first identified. From the dataset GSE110815, 32 significantly upregulated and 44 downregulated miRNAs were found (Figure 3A,B). We then integrated the 7 databases to obtain miRNA-target interactions. Subsequently, 43 unique miRNA-target associations, composed of 15 miRNAs and 34 targets, were obtained from 289,469 primary terms (Figure 3C). Finally, for the miRNA-target regulation network, the degrees of all miRNA ranged from 1 to 5, of which hsa-miR-128 regulated 5 genes (POU2F3, HMGB3, FNDC4, YPEL3, NAV3). Chen et al. reported that miR-128a confers chemoradiotherapy sensitivity of laryngeal cancer cells, and targeting the BMI1 gene is a possible mechanism of the effect (30). Furthermore, Jiang et al. reported that compared to the non-CSCs, there was substantial inhibition miR-128 in the PC9-CSCs and suggested that the miR-128/c-met pathway augments the sensitivity of gefitinib towards the lung cancer stem cells through inhibiting the PI3K/AKT pathway (31). There were 5 miRNAs with a degree of 4. Of these miRNAs, miR-342-3p has been found to be involved in the pathogenesis and drug resistance of NSCLC. Han et al. reported that miR-342-3p targeted ATF3, which promotes osteogenic differentiation (32). In addition, colleagues from Zhang et al.’s labs also found that miR-342-3p, as a ceRNA agent in lncRNA SCARNA2, intermediates colorectal cancer chemoresistance (33). In a previous study, LINC00460 regulated KDM2A to stimulate cell proliferation and migration by pursuing miR-342-3p in gastric cancer (34). However, the involvement of miR-342-3p in gefitinib resistance for NSCLC is not well understood.
miR-342-3p has been found to be involved in the pathogenesis and drug resistance of NSCLC. We reported that the significant DEGs were enriched in the intracellular signal transduction and pathway of PPAR signaling, along with the proteoglycans in cancer (Figure 3D). Peroxisome proliferator-activated receptor gamma (PPARγ) is known to regulate the metabolism of glucose and lipid, inflammation, in addition to endothelial function (35). Efatutazone and gefitinib are agonists of PPARγ, and both together could prevent the propagation of the cells which are resistant to EGFR-TKI through the PPARγ/PTEN/Akt pathway (36). These results indicate that our miRNA-target network provides insights into the miRNA-related mechanisms underlying gefitinib resistance in NSCLC. Here, we are first to reveal that the overexpression of miR-342-3p can significantly increase the gefitinib resistance of A549/GR and PC9/GR cells (Figure 4).
We then selected CPA4, a member of the carboxypeptidase A family (37), as a potential target of miR-342-3p. Because the upregulation of the expression of CPA4 in NSCLC is related to a negative prediction, serum CPA4 level along with serum CYFRA21-1 level may be additionally used for the timely discovery of NSCLC (38). In our data, we found that CPA4 was regulated by miR-342-3p (Figure 5). These data reveal that CPA4 is a straight target gene of miR-342-3p. In previous years, the prevention of cell-death stimulation by gefitinib was the main concern of cancer drug resistance research (39). We also observed the cell apoptosis of NSCLC after the upregulation of CPA4 in gefitinib-resistant lung cancer cells compared to the treatment with miR-342-3p knockdown or up-regulation (Figure 6).
In conclusion, we identified gefitinib resistance-related miRNA and genes and then constructed a miRNA-target network. We demonstrated that miR-342-3p may confer gefitinib resistance in NSCLC via targeting CPA4. Nevertheless, additional studies, such as in vivo experiments aimed to explain the mechanism of drug resistance in up/down streams of miR-342-3p in gefitinib-resistant NSCLC animal models, are still needed.
Acknowledgments
None.
Footnote
Conflicts of Interest: The authors have no conflicts of interest to declare.
Ethical Statement: The authors are accountable for all aspects of the work in ensuring that questions related to the accuracy or integrity of any part of the work are appropriately investigated and resolved.
References
- Agustoni F, Suda K, Yu H, et al. EGFR-directed monoclonal antibodies in combination with chemotherapy for treatment of non-small-cell lung cancer: an updated review of clinical trials and new perspectives in biomarkers analysis. Cancer Treat Rev 2019;72:15-27. [Crossref] [PubMed]
- Torre LA, Bray F, Siegel RL, et al. Global cancer statistics, 2012. CA Cancer J Clin 2015;65:87-108. [Crossref] [PubMed]
- Zito Marino F, Ronchi A, Accardo M, et al. Concomitant ALK/KRAS and ALK/EGFR mutations in non small cell lung cancer: different profile of response to target therapies. Transl Cancer Res 2017;6:S457-60. [Crossref]
- Yang RF, Yu B, Zhang RQ, et al. Bevacizumab and gefitinib enhanced whole-brain radiation therapy for brain metastases due to non-small-cell lung cancer. Braz J Med Biol Res 2017;51:e6073. [Crossref] [PubMed]
- Ma G, Zhu J, Liu F, et al. Long Noncoding RNA LINC00460 Promotes the Gefitinib Resistance of Nonsmall Cell Lung Cancer Through Epidermal Growth Factor Receptor by Sponging miR-769-5p. DNA Cell Biol 2019;38:176-83. [Crossref] [PubMed]
- Peng K, Tian X, Qian Y, et al. Novel EGFR inhibitors attenuate cardiac hypertrophy induced by angiotensin II. J Cell Mol Med 2016;20:482-94. [Crossref] [PubMed]
- Lu H, Liu S, Zhang G, et al. PAK signalling drives acquired drug resistance to MAPK inhibitors in BRAF-mutant melanomas. Nature 2017;550:133-6. [Crossref] [PubMed]
- Yonesaka K, Hirotani K, Kawakami H, et al. Anti-HER3 monoclonal antibody patritumab sensitizes refractory non-small cell lung cancer to the epidermal growth factor receptor inhibitor erlotinib. Oncogene 2016;35:878-86. [Crossref] [PubMed]
- Gao Y, Fan X, Li W, et al. miR-138-5p reverses gefitinib resistance in non-small cell lung cancer cells via negatively regulating G protein-coupled receptor 124. Biochem Biophys Res Commun 2014;446:179-86. [Crossref] [PubMed]
- Garofalo M, Romano G, Di Leva G, et al. EGFR and MET receptor tyrosine kinase-altered microRNA expression induces tumorigenesis and gefitinib resistance in lung cancers. Nat Med 2011;18:74-82. [Crossref] [PubMed]
- Song KA, Hosono Y, Turner C, et al. Increased Synthesis of MCL-1 Protein Underlies Initial Survival of EGFR-Mutant Lung Cancer to EGFR Inhibitors and Provides a Novel Drug Target. Clin Cancer Res 2018;24:5658-72. [Crossref] [PubMed]
- Qi M, Tian Y, Li W, et al. ERK inhibition represses gefitinib resistance in non-small cell lung cancer cells. Oncotarget 2018;9:12020-34. [Crossref] [PubMed]
- Zhi H, Li X, Wang P, et al. Lnc2Meth: a manually curated database of regulatory relationships between long non-coding RNAs and DNA methylation associated with human disease. Nucleic Acids Res 2018;46:D133-8. [Crossref] [PubMed]
- Lewis BP, Shih IH, Jones-Rhoades MW, et al. Prediction of mammalian microRNA targets. Cell 2003;115:787-98. [Crossref] [PubMed]
- Rehmsmeier M, Steffen P, Hochsmann M, et al. Fast and effective prediction of microRNA/target duplexes. RNA 2004;10:1507-17. [Crossref] [PubMed]
- Miranda KC, Huynh T, Tay Y, et al. A pattern-based method for the identification of MicroRNA binding sites and their corresponding heteroduplexes. Cell 2006;126:1203-17. [Crossref] [PubMed]
- Krek A, Grun D, Poy MN, et al. Combinatorial microRNA target predictions. Nat Genet 2005;37:495-500. [Crossref] [PubMed]
- Kozomara A, Griffiths-Jones S. miRBase: integrating microRNA annotation and deep-sequencing data. Nucleic Acids Res 2011;39:D152-7. [Crossref] [PubMed]
- Betel D, Wilson M, Gabow A, et al. The microRNA.org resource: targets and expression. Nucleic Acids Res 2008;36:D149-53. [Crossref] [PubMed]
- Maragkakis M, Vergoulis T, Alexiou P, et al. DIANA-microT Web server upgrade supports Fly and Worm miRNA target prediction and bibliographic miRNA to disease association. Nucleic Acids Res 2011;39:W145-8. [Crossref] [PubMed]
- Chandimali N, Huynh DL, Zhang JJ, et al. MicroRNA-122 negatively associates with peroxiredoxin-II expression in human gefitinib-resistant lung cancer stem cells. Cancer Gene Ther 2019;26:292-304. [Crossref] [PubMed]
- Nakatani K, Yamaoka T, Ohba M, et al. KRAS and EGFR Amplifications Mediate Resistance to Rociletinib and Osimertinib in Acquired Afatinib-Resistant NSCLC Harboring Exon 19 Deletion/T790M in EGFR. Mol Cancer Ther 2019;18:112-26. [Crossref] [PubMed]
- Wilson DF. Oxidative phosphorylation: regulation and role in cellular and tissue metabolism. J Physiol 2017;595:7023-38. [Crossref] [PubMed]
- Giordano CR, Mueller KL, Terlecky LJ, et al. A targeted enzyme approach to sensitization of tyrosine kinase inhibitor-resistant breast cancer cells. Exp Cell Res 2012;318:2014-21. [Crossref] [PubMed]
- Zhang L, Li J, Hu J, et al. Cigarette smoke extract induces EGFR-TKI resistance via promoting EGFR signaling pathway and ROS generation in NSCLC cell lines. Lung Cancer 2017;109:109-16. [Crossref] [PubMed]
- Yu L, Lu M, Jia D, et al. Modeling the Genetic Regulation of Cancer Metabolism: Interplay between Glycolysis and Oxidative Phosphorylation. Cancer Res 2017;77:1564-74. [Crossref] [PubMed]
- Yu C, Xue P, Zhang L, et al. Prediction of key genes and pathways involved in trastuzumab-resistant gastric cancer. World J Surg Oncol 2018;16:174. [Crossref] [PubMed]
- Wei J, van der Wekken AJ, Saber A, et al. Mutations in EMT-Related Genes in ALK Positive Crizotinib Resistant Non-Small Cell Lung Cancers. Cancers (Basel) 2018. [Crossref] [PubMed]
- Beauchemin N, Arabzadeh A. Carcinoembryonic antigen-related cell adhesion molecules (CEACAMs) in cancer progression and metastasis. Cancer Metastasis Rev 2013;32:643-71. [Crossref] [PubMed]
- Chen H, Jin L, Zhou L, et al. Overexpressed miR-128a enhances chemoradiotherapy to laryngeal cancer cells and its correlation with BMI1. Future Oncol 2018;14:611-20. [Crossref] [PubMed]
- Jiang J, Feng X, Zhou W, et al. MiR-128 reverses the gefitinib resistance of the lung cancer stem cells by inhibiting the c-met/PI3K/AKT pathway. Oncotarget 2016;7:73188-99. [Crossref] [PubMed]
- Han Y, Zhang K, Hong Y, et al. miR-342-3p promotes osteogenic differentiation via targeting ATF3. FEBS Lett 2018;592:4051-65. [Crossref] [PubMed]
- Zhang PF, Wu J, Wu Y, et al. The lncRNA SCARNA2 mediates colorectal cancer chemoresistance through a conserved microRNA-342-3p target sequence. J Cell Physiol 2019;234:10157-65. [Crossref] [PubMed]
- Wang F, Liang S, Liu X, et al. LINC00460 modulates KDM2A to promote cell proliferation and migration by targeting miR-342-3p in gastric cancer. Onco Targets Ther 2018;11:6383-94. [Crossref] [PubMed]
- Wang S, Dougherty EJ, Danner RL. PPARgamma signaling and emerging opportunities for improved therapeutics. Pharmacol Res 2016;111:76-85. [Crossref] [PubMed]
- Ni J, Zhou LL, Ding L, et al. PPARgamma agonist efatutazone and gefitinib synergistically inhibit the proliferation of EGFR-TKI-resistant lung adenocarcinoma cells via the PPARgamma/PTEN/Akt pathway. Exp Cell Res 2017;361:246-56. [Crossref] [PubMed]
- Tanco S, Zhang X, Morano C, et al. Characterization of the substrate specificity of human carboxypeptidase A4 and implications for a role in extracellular peptide processing. J Biol Chem 2010;285:18385-96. [Crossref] [PubMed]
- Sun L, Wang Y, Yuan H, et al. CPA4 is a Novel Diagnostic and Prognostic Marker for Human Non-Small-Cell Lung Cancer. J Cancer 2016;7:1197-204. [Crossref] [PubMed]
- Ajabnoor GM, Crook T, Coley HM. Paclitaxel resistance is associated with switch from apoptotic to autophagic cell death in MCF-7 breast cancer cells. Cell Death Dis 2012;3:e260. [Crossref] [PubMed]