Big data and clinical research: perspective from a clinician
Introduction
The 21st century is an era of information technology and people faces information explosion with large amount of data. The term big data has been defined in Wikepedia as: big data is the term for a collection of data sets so large and complex that it becomes difficult to process using on-hand database management tools or traditional data processing applications. In all areas of disciplines, such a big data may help to explore underlying mechanisms of varieties of phenomenon, and further facilitate decision-making. For example, in the 2012 USA presidential election, data-driven decision-making played a huge role in helping Obama win (1). In biomedical field, big data also begin to show its important role. Medical decision-making is becoming more and more dependent on data analysis, rather than conventional experience and intuition (2).
The present paper will focus on how to do clinical research by using big data. Firstly, I will review some basic concepts of clinical research and some characteristics of big data. Next, I will take some examples to illustrate how to address clinical uncertainty via data mining. The aim of the article is to provide more insights into clinical research based on big data in the hope that more people will take initiatives to conduct further investigations into clinical big data. I cannot list all detailed technical issues in this article, for which I will provide more references. Interested investigators may take these references as tutorials to guide them through the difficult journal of data exploration.
Bewilderedness in the current clinical research
It is well known that clinical research can be generally categorized into interventional and observational studies. The former is an experimental study that certain interventions will be given to participants. The most commonly performed randomized controlled trial (RCT) belongs to this kind of study. RCT usually has strict inclusion and exclusion criteria for participants. The procedure of randomization is employed to balance potential confounding factors between treatment and control arms. RCTs and/or systematic review involving high quality RCTs are the gold standard for clinical guidelines. However, with more and more RCTs being conducted, its limitations have arisen. In the area of sepsis and critical care medicine, we have compared the results obtained from RCTs and observational studies, and found that interventional effects obtained from these two types of study were quite different (3,4). Biological efficacy, a measurement of effect size under strict experimental condition, can be obtained by RCT. However, this biological efficacy may be attenuated or even not take place at real world setting. In this circumstance the biological efficacy cannot be translated into clinical efficacy (5). Clinical effectiveness is the most relevant to clinicians. The next question goes to why biological efficacy cannot be translated to clinical effectiveness. The plausible explanation is that RCTs are usually conducted with strict experimental design. There is a long list of inclusion and exclusion criteria. The participants are highly selected to exclude potential confounders. The protocol of intervention is also strictly defined with respect to timing, dosing and sequence of procedure. However, in reality, the condition as defined in RCTs cannot be fulfilled. Patients are usually complicated by comorbidities such as renal dysfunction, hypertension, and congestive heart failure. The timing of drug administration may be delayed due to admission at busy hours. As a result, it is probably that we treat our patients based on knowledge derived from a minority of highly selected patients. That is to say, the conclusion from RCTs may not be generalizable to “real world” patients.
Although there is no solution to above-mentioned limitations of current clinical research, big data may provide some insights into future direction of clinical trials (6,7). Wang and colleagues have proposed that big-data clinical trial (BCT) may become a mainstay type of clinical research and complement RCT in an important way (8,9). Big data in clinical study refers to the information collected using electronic database. These data come from daily routine clinical practice without modification or screening with strict inclusion and exclusion criteria, therefore retaining its real-world features (10). The advantage of BCT is that the result directly reflects clinical effectiveness. Big data in medical or epidemiological research generally consists electronic medical record system, administrative registry for chronic and infectious diseases and medical insurance system (11). As clinicians, our research may focused on EMR system which consists information on demographics, laboratory findings, microbiology data, medical order, procedures, surgery and clinical outcomes (12). However, big data is not a panacea without limitations. BCT is a kind of observational study in nature and has inherent limitations of its kind. For example, the observed and un-observed baseline characteristics cannot be well balanced. The conclusion may not be generalizable to other institutions if data were collected from single center. Such limitation can be addressed with advanced statistical method such as random effects model and bootstrap estimation of coefficient.
How to do clinical research with big data: an example from multiparameter intelligent monitoring in intensive care II (MIMIC-II)
This section will take MIMIC-II as an example to illustrate how to incorporate big data into clinical research (13). The flow chart of analysis is shown in Figure 1.
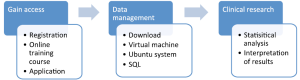
MIMIC-IIis an open access database comprising clinical data of ICU patients from Beth Israel Deaconess Medical Center (http://physionet.org/mimic2/). The database is consistently updating with current version of 2.6 that contains >30,000 ICU patients from 2001 to 2008 (14). MIMIC-II consists clinical data and high resolution waveform.
Gaining access to MIMIC-II
The investigator should register a username via on the website, and then apply for the access to database. An online training course named “protecting human research participants” should be completed and a certification number will be assigned to individual investigator (Figure 2). With this certification number, one is qualified to apply for the access to database. After a couple of days, the whole database is accessible to you and data analysis can be performed according to one’s interests.
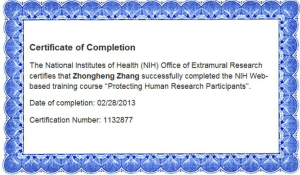
Conceiving clinical research ideas
With such a huge amount of clinical data, the next question is how to conceive clinical research ideas. Firstly, I would like to enumerate several types of clinical research by using big data (Table 1). The first one involves exploration of risk factors, for which high resolution data are usually required for confounding controls. Multivariable models, stratification and propensity score analysis are useful tool for such kind of analysis. The second involves assessment of effectiveness of interventions. Similarly, this type of study requires high-resolution data to control for confounding factors. Bias associated with selective treatment may play an important role and should be considered in study design. The third involves prediction model building, which aims to fit a model for future prediction. The forth type is assessment of cost-effectiveness of health policy.
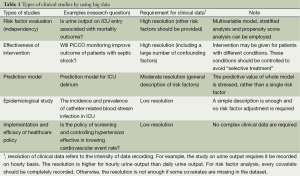
Full table
Another key issue is how to conceive research ideas that can be addressed with database. There are two approaches: one is to perform data mining according to your research question, and the other is to adapt your research question to the database. Sometimes these two approaches may be used simultaneously. In a study investigating the association of lactate and clinical outcome (15), we planed to explore lactate measured on ICU entry at the outset. However, after data mining we found that lactate was usually measured repeatedly and decision was made to explore the trend of lactate (lactate clearance). The study protocol was adapted accordingly.
To conceive research idea based on your data is another way. One can perform statistical description for a dataset, by using traditional central tendency (mean, median) and discrete tendency (full range, 95% confidence interval). Graphical demonstration may be particularly helpful. For example, contour plot may help to explore associations between three variables; histogram can be used to explore distribution of a variable. However, someone may contend that a peek at dataset before drafting a study protocol may introduce bias (e.g., the problem of multiple testing and selective reporting are of this kind). That is to say, twenty times statistical testing for association will result in one with P<0.05 among independently generated random variables. I acknowledge this limitation, but such study can still be used as hypothesis generating and provide rationale for further explorations.
Another type of study is to investigate simple and easily obtainable parameters. By using such parameters, your study and ideas can be addressed by using varieties of database. Our study group has previously performed association study involving urine output and mortality (16). Because urine output is an essential but easily obtainable variable, it should be recorded in all kinds of ICUs. There is no reason to omit this recording, just like all other vital signs. We were confident at the outset that urine output must be carefully recorded in MIMIC-II, and the study was expected to conduct smoothly. Such simple variables included temperature, electrolytes and heart rate. In another study we investigated the association of ionized calcium and mortality (17). However, studies involving simple variables are usually criticized as being lack of novelty, and this may be the most important reason to reject our paper.
Data extraction
There is a small version of the MIMIC-II that can be accessed via query builder (https://mimic2app.csail.mit.edu/querybuilder/). A limited number of rows can be exported from this version, and thus it is primarily used for testing structural query language (SQL). I found that it is useful for preliminary exploration of the data. I was doing research on brain natriuretic peptide (BNP) when I first encounter the MIMIC-II (18,19), the first idea come to my mind is to use this big data to establish a linkage between BNP and clinical outcome. However, when I started to explore the database I found that the information on BNP was scarce. In the end I realized that the payment for medical insurance in USA is closely monitored and not every one was indicated to have BNP measured (e.g., BNP is only covered by insurance when it is used to determine the cause of respiratory distress in emergency department). Query builder can be accessed via windows operating system that is convenient for most Chinese users, whereas the whole database can only be extracted via virtual machine on Ubuntu operating system.
Access to the whole MIMIC-II database requires the users to have some basic knowledge on virtual machine and Ubuntu operating system. The downloaded package compressed file with suffix “.tar”, taking disc space of 30 G. The file can be directly imported into the virtual box (Oracle). After entering username and password, one gains access to the Ubuntu operating system. The username and password are mimic2 and 2CIMIM_2v6, respectively. Pgadmin is the most popular and feature rich open Source administration and development platform for PostgreSQL, it will be opened to extract data under Ubuntu operating system.
Some investigators may want to export data for further analysis under Windows system. Data transferring between different operating systems can be performed via email. The file extracted has suffix of .cvs, which can be imported into Stata statistical package. Other statistical software such as SAS and SPSS also support this format.
Data processing using Stata
The author is more familiar with Stata statistical package in data processing, and the following section will introduce some steps in data processing with Stata.
Data processing using Stata is consisted of data management and statistical analysis. In my experience around 80% time and energy are spent on data management. This step included several aspects: (I) to generate new variables, for example, one wants to transform continuous variable age into binary variable (e.g., old vs. young); (II) variable checking, the sum module can check for some senseless values (e.g., age =200); (III) to transform string variable into numerical variable, or vise versa; (IV) combination of dataset. Different types of variable are stored in different relational tables. They should be combined into one dataset for the purpose of statistical analysis. Stata provided very useful modules such as merge and append for this purpose. Statistical analysis in based on correctly performed data management.
One advantage of Stata is its ability to record complete process of data analysis. Data analysis can be performed in three ways: windows pull-down menu, command input in command window and do-file. I suggest that data analysis be performed by using do-file, because it is able to record the whole process of how data is managed and analyzed. This will facilitate replication of analysis and made revisions. Furthermore, cooperation among researchers also requires do-file. The other two methods (e.g., windows pull-down menu and command input in command window) are mainly used to test a stata syntax or to facilitate draw graphs with complex options.
A complete dual flow chart of data analysis using stata is shown in Figure 3. I suggest split data analysis into two parts: data management and statistical analysis. The left column shows data management that will make changes to the dataset in memory. Dataset generated by using do-file will be stored in memory with suffix of “.dta”. The right column simply performs statistical analysis and will not significantly change the dataset.
Using structural query language (SQL) to extract data
An important step in preparing data for analysis is data extraction by using SQL. Efficient use of SQL will save a lot of time and disc space. Some tasks previously mentioned during data management can be performed at the step of data extraction. For example, one intends to restrict data analysis to adult population. This can be performed by using stata command “if”, or using SQL “where” clause. I prefer to use stata for data management because it tracks the process of data management. However, it is at the discretion of investigator.
A simple SQL syntax can be written as: Select variable name from table name where conditions, where variable name refers to variables contained in relational tables. The asterisk “*” can replace the variable name if all variables are expected to be extracted. The table name refers to the name of the relational table. The conditions are a set of expressions used to select observations fulfilling certain criteria. For example, the expression “age >65” can be used if your target population is old people. For complex SQL syntax, readers can see other textbooks on SQL such as the language of SQL: How to Access Data in Relational Databases edited by Larry Rockoff and SQL Cookbook by Anthony Molinaro.
Conclusions
The exploration of big data is a process of trial and error. Someone may feel that it is a task of distress, but I feel it is a hard way filled with success and surprise. The secret of human disease may lurk under the vast ocean of big data, waiting us to decode and understand them. The article is not intended to serve as a step-by-step guidance on using big data but to inspire people who are interested in doing exploration on it. In China, it is possible to establish our own high quality big data because we have the largest population in the world.
Acknowledgements
Disclosure: The author declares no conflict of interest.
References
- Scherer M. Inside the Secret World of the Data Crunchers Who Helped Obama Win. Available online: http://swampland.time.com/2012/11/07/inside-the-secret-world-of-quants-and-data-crunchers-who-helped-obama-win/
- Margolis R, Derr L, Dunn M, et al. The National Institutes of Health‘s Big Data to Knowledge (BD2K) initiative: capitalizing on biomedical big data. J Am Med Inform Assoc 2014;21:957-8. [PubMed]
- Zhang Z, Ni H, Xu X. Do the observational studies using propensity score analysis agree with randomized controlled trials in the area of sepsis? J Crit Care 2014;29:886.e9-15.
- Zhang Z, Ni H, Xu X. Observational studies using propensity score analysis underestimated the effect sizes in critical care medicine. J Clin Epidemiol 2014;67:932-9. [PubMed]
- Nallamothu BK, Hayward RA, Bates ER. Beyond the randomized clinical trial: the role of effectiveness studies in evaluating cardiovascular therapies. Circulation 2008;118:1294-303. [PubMed]
- Schneeweiss S. Learning from big health care data. N Engl J Med 2014;370:2161-3. [PubMed]
- Psaty BM, Breckenridge AM. Mini-Sentinel and regulatory science--big data rendered fit and functional. N Engl J Med 2014;370:2165-7. [PubMed]
- Wang SD. Opportunities and challenges of clinical research in the big-data era: from RCT to BCT. J Thorac Dis 2013;5:721-3. [PubMed]
- Wang SD, Shen Y. Redefining big-data clinical trial (BCT). Ann Transl Med 2014;2:96. [PubMed]
- Albert RK. "Lies, damned lies .." and observational studies in comparative effectiveness research. Am J Respir Crit Care Med 2013;187:1173-7. [PubMed]
- Cooke CR, Iwashyna TJ. Using existing data to address important clinical questions in critical care. Crit Care Med 2013;41:886-96. [PubMed]
- Peters SG, Buntrock JD. Big data and the electronic health record. J Ambul Care Manage 2014;37:206-10. [PubMed]
- Saeed M, Villarroel M, Reisner AT, et al. Multiparameter Intelligent Monitoring in Intensive Care II: a public-access intensive care unit database. Crit Care Med 2011;39:952-60. [PubMed]
- Scott DJ, Lee J, Silva I, et al. Accessing the public MIMIC-II intensive care relational database for clinical research. BMC Med Inform Decis Mak 2013;13:9. [PubMed]
- Zhang Z, Chen K, Ni H, et al. Predictive value of lactate in unselected critically ill patients: an analysis using fractional polynomials. J Thorac Dis 2014;6:995-1003. [PubMed]
- Zhang Z, Xu X, Ni H, et al. Urine output on ICU entry is associated with hospital mortality in unselected critically ill patients. J Nephrol 2014;27:65-71. [PubMed]
- Zhang Z, Xu X, Ni H, et al. Predictive value of ionized calcium in critically ill patients: an analysis of a large clinical database MIMIC II. PLoS One 2014;9:e95204. [PubMed]
- Zhang Z, Zhang Z, Xue Y, et al. Prognostic value of B-type natriuretic peptide (BNP) and its potential role in guiding fluid therapy in critically ill septic patients. Scand J Trauma Resusc Emerg Med 2012;20:86. [PubMed]
- Zhang Z, Ni H, Lu B, et al. Changes in brain natriuretic peptide are correlated with changes in global end-diastolic volume index. J Thorac Dis 2013;5:156-60. [PubMed]