Identification of key genes for esophageal squamous cell carcinoma via integrated bioinformatics analysis and experimental confirmation
Introduction
As one of the most common malignant cancer types, esophageal cancer (EC) ranks the sixth leading cause of cancer-related mortality all over the world, with an estimated 400,000 deaths annually (1,2). EC contains two major histologic subtypes: esophageal squamous cell carcinoma (ESCC) and esophageal adenocarcinoma, which are classified based on geographic location and genetic alterations (3). ESCC is the predominant histological classification worldwide, accounting for about 80% of EC cancer (4). To be specific, ESCC accounts for about 90% of EC cancer and is the fourth leading cause of cancer-related death in China (5). Current treatments for ESCC include chemotherapy, radiation therapy and surgery. Despite advances in early diagnosis and clinical management, the overall 5-year survival rate of ESCC patients remains less than 25% due to delayed diagnosis at an advanced stage and lack of effective targeted therapy (6). Development of ESCC is a multistep process containing a series of genetic and epigenetic alterations associated with life and environment factors (7). Thus, it is important to fully understand the molecular mechanisms of carcinogenesis process to identify critical targets and develop novel and effective treatments for ESCC.
Various genes, such as mRNAs and non-coding RNAs including miRNAs and lncRNAs have been reported to form complex networks regulating the tumorigenesis and progression of human cancers. Recently, in order to screen differentially expressed genes (DEGs) associated with carcinogenesis and progression of human cancer, and to identify biomarkers and potential therapeutic targets, more and more microarray and high throughput sequencing technologies combined with bioinformatics analysis have been widely used (8,9). However, despite numerous of studies have been performed using high throughput technologies, only very few biomarkers and drug targets have been translated into clinical practice, mainly due to false-positive rates in independent microarray analysis, different technological platforms or small sample size (10,11). In the present study, two original datasets were downloaded from Gene Expression Omnibus (GEO) database and analyzed to obtain DEGs in ESCC. A total of 746 DEGs commonly shared by both data sets were selected for further bioinformatics analysis, including gene ontology (GO)/Kyoto Encyclopedia of Genes and Genomes (KEGG) analysis, Gene Set Enrichment Analysis (GSEA) pathway analysis and construction of protein-protein interaction (PPI) networks. Additionally, the correlation between the hub genes and ESCC dataset from the Cancer Genome Atlas (TCGA) was analyzed using weighted gene co-expression network analysis (WGCNA). DLGAP5 was selected for function confirmation in ESCC cells. DLAGP1 positively regulated ESCC cells proliferation.
Methods
Microarray data collection
The original microarray data were downloaded from the GEO database (12). The GSE20347 based on GPL571 platform (Affymetrix Human Genome U133A 2.0 Array) contains 17 paired ESCC samples and normal adjacent esophageal tissues. The GSE26886 based on GPL570 platform (Affymetrix Human Genome U133 Plus 2.0 Array) contains 9 ESCC samples and 19 normal esophageal tissues. We used R, affy package and gcrma package (GC Robust Multi-array Average method) for data processing (13).
GO and pathway enrichment analysis of DEGs
The online tool database for annotation, visualization, integrated discovery (DAVID; https://david.ncifcrf.gov/) containing GO and KEGG pathway analysis was used to analyze the biological characteristics and function annotation of candidate DEGs (14). GO is a useful tool for analyzing characteristic biological information including biological process in the present study (15). Here, KEGG pathway analysis was also performed to analyze the signaling pathways mediated by the DEGs (16). Additionally, GSEA was selected to determine whether DEGs was involved in one phenotype or signaling pathway using GSEA software (http://software.broadinstitute.org/gsea/index.jsp) (17).
PPI network construction and hub genes screening
The Search Tool for Retrieval of Interacting Genes database (STRINGdb: https://string-db.org/) was used to get the PPI network information (18). Here, the DEGs were mapped into PPIs using Cytoscape software 3.4.0 (http://www.cytoscape.org) and a combined score of >0.4 was used as the cut-off value that was considered statistically significant. Then the 40 nodes with edge of >20 were selected as hub genes for further analysis.
WGCNA network construction and key modules identification
To further confirm the DEGs with a critical role in ESCC, ESCC data from TCGA database was downloaded. The “WGCNA” R package was used to put the DEGs of ESCC samples from TCGA database into modules by average linkage clustering (19,20). Here, the power of β=5 (scale free R2=0.8) was set as the soft thresholding to ensure a scale-free network. The average linkage clustering tree based on the topological overlapping distance of the gene expression spectrum is constructed. The hierarchical clustering tree displays the gene classification module, and the final module group is obtained after fusion. The gene tree is visually inspected by different module colors. Then, the relevance between each module and the 40 hub genes selected from GEO data was analyzed. The KEGG and GSEA analysis for selecting module was performed.
Cell culture and transfection
ESCC cell lines including TE-1, KYSE30 and KYSE410, KYSE180 and KYSE520 were cultured in RPMI-1640 or DMEM medium (Gibco, Carlsbad, CA, USA) supplemented with 10% fetal bovine serum. To establish transfectants with DLGAP5 knockdown, TE-1 and KYSE410 were transfected with psi-LVRU6GP vectors with DLGAP5 shRNAs (target sequence for sh-1#: 5'-GGATATAAGTACTGAAATGAT-3', sh-2#: 5'- GGTATTTCTTGTAAAGTCGAT-3', sh-3#: 5'- CCATATTTCAGAAATATCCTC-3'). The transfection was performed using Lipofectamine 3000 (Invitrogen, Carlsbad, CA, USA) referring to recommendations.
Western blot
Cells were harvested and washed with PBS. Total protein was collected with RIPA Lysis Buffer with Protease and Phosphatase inhibitor. The protein concentration was measured by BCA Protein Assay Kit. 30 µg of protein was used for separation by 10% SDS-PAGE gels and transferred onto 0.2 µm PVDF membranes. The membrane was blocked with 5% non-fat milk in TBS-Tween (TBS-T, 0.1% Tween) at 37 °C for 2 h and incubated overnight at 4 °C with the primary antibodies. The membrane was incubated with horseradish peroxidase (HRP)-conjugated secondary antibodies (Sigma-Aldrich) at room temperature for 2 h. Finally, the membranes were washed and the immunoreactive bands were visualized using an ECL western blotting system (Beyotime, Shanghai, China). The monoclonal mouse anti-β-actin antibody was from Sigma and the polyclonal rabbit anti-DLGAP5 antibody was from abcam.
Proliferation assay
Cells were seeded into 24-well plates at 10,000 cells/well. The cell number was counted every 2 days and the time-cell number curves were plotted. Alternatively, cells were seeded into 6-well plates at 1,000 cells/well in triplicates and incubated to allow colony formation for 7 days. The colonies were stained with crystal violet and then counted.
Statistical analysis
All data were presented as the mean ± SD. The student’s t test was used to compare the differences among different groups. Statistical analyses were performed using GraphPad Prism 6. P value <0.05 was considered statistically significant.
Results
Identification of DEGs in ESCC
To identify the DEGs those maybe play critical roles in the development and progression of ESCC, two microarray datasets were collected from the GEO database. In the GSE20347 dataset concluding ESCC samples and paired normal adjacent esophageal tissues from 17 patients, 465 upregulated and 559 downregulated DEGs were identified using fold change >2 and P<0.05 (Figure 1A). In the other dataset GSE26886 concluding 9 ESCC samples and 19 normal esophageal tissues, 838 upregulated and 947 downregulated DEGs were identified using fold change >2 and P<0.05 (Figure 1A). Among these candidate DEGs, a total of 746 DEGs were commonly shared in the two datasets, including 286 commonly upregulated DEGs and 460 commonly downregulated DEGs shown in the Venn diagrams (Figure 1A). Meanwhile, the volcano plots (Figure 1B) and heatmaps (Figure 1C) were used to represent the differentially expressed profiles of DEGs within the datasets.
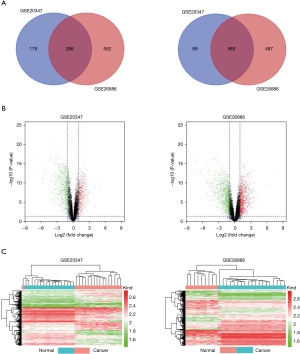
Enrichment analysis of the candidate DEGs in ESCC
To further gain insights into the function of DEGs, the candidate DEGs between ESCC and normal esophageal tissues were analyzed using DAVID software for enrichment. The GO analysis showed that the biological process enrichment terms of upregulated DEGs were mainly associated with extracellular matrix organization, collagen biosynthetic and catabolic processes, cell cycle and proliferation (Figure 2A) and the downregulated DEGs were mainly associated with epidermis development, keratinocyte differentiation, oxidation-reduction process and Hippo signaling (Figure 2B). Then, KEGG analysis of the DEGs was performed and the biological pathway analysis indicated that the upregulated DEGs were mainly enriched in ECM-receptor interaction, focal adhesion, PI3K-Akt signaling pathway and cell cycle (Figure 3A) and the downregulated DEGs were mainly enriched in metabolic pathways and chemical carcinogenesis (Figure 3B). Furthermore, stratified GSEA analysis also indicated the enrichment of upregulated DEGs in the cell cycle (Figure 3C).
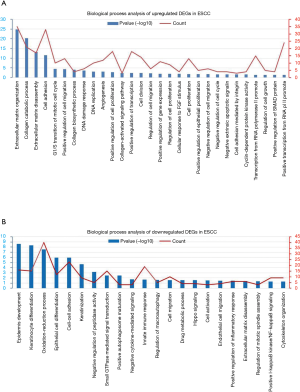
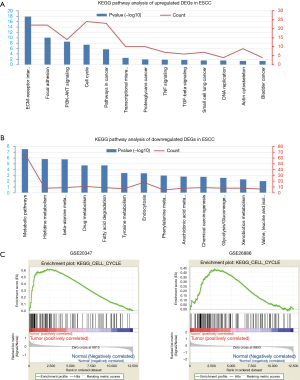
PPI network construction and hub genes screening
The PPI network of the DEGs was constructed with Cytoscape software based on the STRING database. The interaction with a combined score of >0.4 were selected to construct the PPI network. The PPI network contains 465 nodes and 3,384 edges (Figure 4A). To identify the critical genes involved in ESCC development and progression, 40 nodes with edge of >20 were selected as hub genes for further analysis, among which ESPL1, VAMP8, TIMP2 and RHOA were downregulated genes and others were upregulated genes (Figure 4B).
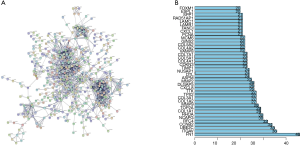
Weighted co-expression network construction and key modules identification
To further investigate the precise expression of DEGs in ESCC, we analyzed the expression pattern of the selected 40 hub genes in 80 ESCC samples from the TCGA database. After excluding the abnormal samples, under the screening conditions of FDR <0.05 and ∣log2FC∣>2, the 40 DEGs were well clustered and were selected for follow-up analysis (Figure S1). Then, we used “WGCNA” R package to put the DEGs of ESCC samples from TCGA database into modules by average linkage clustering. In the present study, the power of β=5 (scale free R2=0.8) was set as the soft thresholding to ensure a scale-free network (Figure S2A). The average linkage clustering tree based on the topological overlapping distance of the gene expression spectrum is constructed. The hierarchical clustering tree displays the gene classification module, and the final module group is obtained after fusion. The gene tree is visually inspected by different module colors (Figure S2B). Then, the relevance between each module and the 40 hub genes selected from GEO data was analyzed (Figure 5A). After filtering of modules with low quality and relevance lower than 0.5, the MEyellowgreen and MEblack modules met the requirement (Figure 5A). The MEblack module was selected for further analysis. The biological pathway analysis indicated that the MEblack module was mainly enriched in cell cycle, Spliceosome, DNA replication and Oocyte meiosis (Figure 5B). Among the hub genes correlated with MEblack module, GSEA analysis indicated that DEGs of TCGA samples with DLGAP5 upregulation was enriched in cell cycle (Figure 5C).
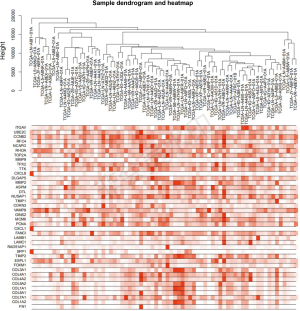
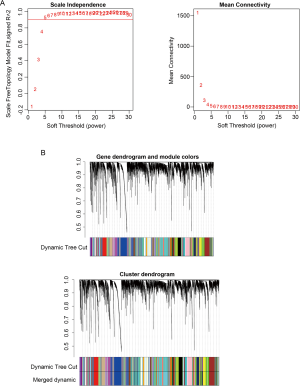
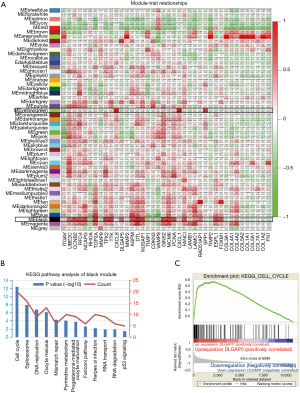
DLGAP5 promotes ESCC cells proliferation
To investigate the biological function of DLGAP5 in ESCC cells, firstly DLGAP5 expression was analyzed between ESCC samples from different clinical stages, but DLGAP5 was not significantly correlated with cancer stage (data not shown). Then, the DLGPA5 protein level was detected in a series of ESCC cell lines including TE-1, KYSE30, KYSE180, KYSE410 and KYS E520. As indicated DLGAP5 protein was endogenously highly expressed in ESCC cells lines (Figure 6A). Then, DLGAP5 expression was knocked down using specific shRNAs and sh-1# and sh-2# were selected for further studies because of their high efficiency in DLGAP5 knockdown (Figure 6B). MTS assay was performed to examine ESCC cells proliferation, which indicated that DLGAP5 knockdown significantly inhibited the proliferation of TE-1 and KYSE410 cells (Figure 6C). The colony formation assay also indicated that DLGAP5 knockdown markedly inhibited colony formation capacity of TE-1 and KYSE410 cells (Figure 6D). These results suggested that DLGAP5 expression was correlated with ESCC cells proliferation.
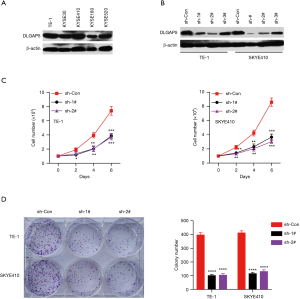
Discussion
ESCC as the predominant histological subtype of EC, is a highly aggressive malignancy. Although great attention has been paid to ESCC, the response to treatments is poor and the clinical outcomes of ESCC are still seriously unfavorable (4,6). It has been reported that ESCC is associated with multiple environmental factors including smoking, alcohol consumption, diet with pickled vegetables, and exposure to chemical factors such as N-nitroso compounds (21). Recent genomic studies have suggested the mutational genes in ESCCs, including genes involved in tumorigenesis, cell cycle, apoptosis and epigenetic modification. Some of those mutational genes are well-known cancer-associated genes such as TP53, RB1, CDKN2A, PIK3CA and NOTCH1, and histone regulator genes such as MLL2, SETD1B and EP300, suggesting the important roles of gene mutation and amplification in ESCCs development and progression (5,22). Despite intensive studies in molecular mechanisms and advances in early diagnosis and clinical management, the outcomes of patients with ESCCs are still very unsatisfactory. Thus, it is important and urgent to fully understand the molecular mechanisms for ESCCs development and progress, and then to identify effective targets and develop novel therapeutic strategies for ESCCs.
Recently, high-throughput technologies such as microarray and RNA sequencing, and bioinformatics analysis have been used to identify the DEGs and signaling pathways involved in the development and progression of ESCCs. However, only very few functional DEGs have been confirmed and translated into clinical practice, mainly due to false-positive or negative rates in independent analysis (10,11). In the present study, to gain reliable results, we first used to two independent datasets to screen DEGs for ESCCs. After series bioinformatics analysis including GO, KEGG, GSEA and PPI network construction, 40 DEGs were selected as hub genes for WGCNA with ESCCs data from TCGA. Then, MEyellowgreen and MEblack modules were identified. Among the hub genes correlated with MEblack module, GSEA analysis indicated that DEGs of TCGA samples with DLGAP5 upregulation was enriched in cell cycle.
DLGAP5 (also known as HURP) belongs to the DLGAP (discs large-associated protein) family that includes five members, termed as DLGAP1-5 (23), which share three key domains: a dynein light chain domain, a 14-amino-acid repeat domain and a guanylate kinase-associated protein homology domain (24). DLGAP5 as a microtubule-associated protein has a critical role in spindle assembly, kinetochore fibers stabilization and chromosomal segregation during mitosis (25,26). The activity of DLGAP5 (HURP) is regulated by Aurora A via phosphorylating the C-terminal domain and then releasing the inhibition on its N-terminal domain binding with microtubule, thus regulating centrosome formation, chromosome segregation and spindle apparatus formation (27). Previous study reported that DLGAP5 is highly expressed in the bone marrow precursor cells but not expressed in the peripheral blood monocytes, and that DLGAP5 express decreased during the stem cell differentiation process (28). These data implied that DLGAP5 might be involved in some cancer types originating from multipotent cancer stem cells. Factually, DLGAP5 overexpression has been reported in variety of cancer types such as hepatocellular carcinoma (29), urinary bladder transitional cell carcinoma (30), meningioma (31), adrenocortical carcinoma (32,33) and prostate cancer (34). Recently, Wang et al. showed that DLGAP5 was highly expressed in aggressive non-small cell lung cancer (NSCLC) and negatively correlated with survival. DLGAP5 silence resulted into inhibition of NSCLC cell proliferation and invasion (35). Here, we confirmed the endogenously high expression of DLGAP5 protein in ESCC cells. Although based on DLGAP5 expression we did not get information for prognosis mainly due to small sample size, our experimental results indicated that DLGAP5 knockdown significantly suppressed ESCC cell proliferation. Of course, we will analyze the role of DLGAP5 in prognosis using a larger ESCC patients cohort, investigate its function in ESCC using in vitro and in vivo models, and explore the detailed mechanisms in our further studies. In regard to the therapeutic point of view, recent study reported a strong synergistic effect between DLGAP5 knockdown and docetaxel in the androgen-sensitive prostate cancer cells (36). Here, our findings also provided strong support for rational design of novel treatment strategies based on DLGAP5 function inhibition using gene therapy mediated known or inhibitor, combined with chemotherapy or radiation. The potent implication also needs further studies.
In summary, here, we screened DEGs that may be involved in the development or progression of ESCC using two independent GEO database. After bioinformatics analysis, potential hub genes from DEGs were correlated with ESCCs from TCGA database using weighted co-expression analysis and DLGAP5 was identified. Preliminary experiments suggested DLGAP5 promoted ESCC cells proliferation. However, further studies are required to elucidate the roles and mechanisms of DLGAP5 in ESCCs.
Acknowledgments
We would also like to thank all investigators helped in data collection and analysis. We are also grateful to all who reviewed and commented on an early draft of the paper. And finally, we would like to thank those who suggested on ways to enhance the paper and the reviewers.
Funding: None.
Footnote
Conflicts of Interest: All authors have completed the ICMJE uniform disclosure form (available at http://dx.doi.org/10.21037/jtd.2020.01.33). The authors have no conflicts of interest to declare.
Ethical Statement: The authors are accountable for all aspects of the work in ensuring that questions related to the accuracy or integrity of any part of the work are appropriately investigated and resolved. This study was approved by the Ethics Committee of Sun Yat-sen University Cancer Center (approval number: GZR 2018-120).
Open Access Statement: This is an Open Access article distributed in accordance with the Creative Commons Attribution-NonCommercial-NoDerivs 4.0 International License (CC BY-NC-ND 4.0), which permits the non-commercial replication and distribution of the article with the strict proviso that no changes or edits are made and the original work is properly cited (including links to both the formal publication through the relevant DOI and the license). See: https://creativecommons.org/licenses/by-nc-nd/4.0/.
References
- Schweigert M, Dubecz A, Stein HJ. Oesophageal cancer--an overview. Nat Rev Gastroenterol Hepatol 2013;10:230-44. [Crossref] [PubMed]
- Bray F, Ferlay J, Soerjomataram I, et al. Global cancer statistics 2018: GLOBOCAN estimates of incidence and mortality worldwide for 36 cancers in 185 countries. CA Cancer J Clin 2018;68:394-424. [Crossref] [PubMed]
- Smyth EC, Lagergren J, Fitzgerald RC, et al. Oesophageal cancer. Nat Rev Dis Primers 2017;3:17048. [Crossref] [PubMed]
- Arnold M, Soerjomataram I, Ferlay J, et al. Global incidence of oesophageal cancer by histological subtype in 2012. Gut 2015;64:381-7. [Crossref] [PubMed]
- Song Y, Li L, Ou Y, et al. Identification of genomic alterations in oesophageal squamous cell cancer. Nature 2014;509:91-5. [Crossref] [PubMed]
- Pennathur A, Gibson MK, Jobe BA, et al. Oesophageal carcinoma. Lancet 2013;381:400-12. [Crossref] [PubMed]
- Hao JJ, Lin DC, Dinh HQ, et al. Spatial intratumoral heterogeneity and temporal clonal evolution in esophageal squamous cell carcinoma. Nat Genet 2016;48:1500-7. [Crossref] [PubMed]
- Kulasingam V, Diamandis EP. Strategies for discovering novel cancer biomarkers through utilization of emerging technologies. Nat Clin Pract Oncol 2008;5:588-99. [Crossref] [PubMed]
- Zhang L, Yang Y, Cheng L, et al. Identification of Common Genes Refers to Colorectal Carcinogenesis with Paired Cancer and Noncancer Samples. Dis Markers 2018;2018:3452739. [Crossref] [PubMed]
- Ni M, Liu X, Wu J, et al. Identification of Candidate Biomarkers Correlated With the Pathogenesis and Prognosis of Non-small Cell Lung Cancer via Integrated Bioinformatics Analysis. Front Genet 2018;9:469. [Crossref] [PubMed]
- Li L, Lei Q, Zhang S, et al. Screening and identification of key biomarkers in hepatocellular carcinoma: Evidence from bioinformatic analysis. Oncol Rep 2017;38:2607-18. [Crossref] [PubMed]
- Edgar R, Domrachev M, Lash AE. Gene Expression Omnibus: NCBI gene expression and hybridization array data repository. Nucleic Acids Res 2002;30:207-10. [Crossref] [PubMed]
- Yang K, Gao J, Luo M. Identification of key pathways and hub genes in basal-like breast cancer using bioinformatics analysis. Onco Targets Ther 2019;12:1319-31. [Crossref] [PubMed]
- Dennis G Jr, Sherman BT, Hosack DA, et al. DAVID: Database for Annotation, Visualization, and Integrated Discovery. Genome Biol 2003;4:3. [Crossref] [PubMed]
- Torto-Alalibo T, Purwantini E, Lomax J, et al. Genetic resources for advanced biofuel production described with the Gene Ontology. Front Microbiol 2014;5:528. [Crossref] [PubMed]
- Du J, Yuan Z, Ma Z, et al. KEGG-PATH: Kyoto encyclopedia of genes and genomes-based pathway analysis using a path analysis model. Mol Biosyst 2014;10:2441-7. [Crossref] [PubMed]
- Subramanian A, Kuehn H, Gould J, et al. GSEA-P: a desktop application for Gene Set Enrichment Analysis. Bioinformatics 2007;23:3251-3. [Crossref] [PubMed]
- Szklarczyk D, Franceschini A, Wyder S, et al. STRING v10: protein-protein interaction networks, integrated over the tree of life. Nucleic Acids Res 2015;43:D447-52. [Crossref] [PubMed]
- Horvath S, Dong J. Geometric interpretation of gene coexpression network analysis. PLoS Comput Biol 2008;4:e1000117. [Crossref] [PubMed]
- Mason MJ, Fan G, Plath K, et al. Signed weighted gene co-expression network analysis of transcriptional regulation in murine embryonic stem cells. BMC Genomics 2009;10:327. [Crossref] [PubMed]
- Liu K, Zhao T, Wang J, et al. Etiology, cancer stem cells and potential diagnostic biomarkers for esophageal cancer. Cancer Lett 2019;458:21-8. [Crossref] [PubMed]
- Gao YB, Chen ZL, Li JG, et al. Genetic landscape of esophageal squamous cell carcinoma. Nat Genet 2014;46:1097-102. [Crossref] [PubMed]
- Rasmussen AH, Rasmussen HB, Silahtaroglu A. The DLGAP family: neuronal expression, function and role in brain disorders. Mol Brain 2017;10:43. [Crossref] [PubMed]
- Liu J, Liu Z, Zhang X, et al. Examination of the expression and prognostic significance of DLGAPs in gastric cancer using the TCGA database and bioinformatic analysis. Mol Med Rep 2018;18:5621-9. [PubMed]
- Wong J, Fang G. HURP controls spindle dynamics to promote proper interkinetochore tension and efficient kinetochore capture. J Cell Biol 2006;173:879-91. [Crossref] [PubMed]
- Ye F, Tan L, Yang Q, et al. HURP regulates chromosome congression by modulating kinesin Kif18A function. Curr Biol 2011;21:1584-91. [Crossref] [PubMed]
- Wong J, Lerrigo R, Jang CY, et al. Aurora A regulates the activity of HURP by controlling the accessibility of its microtubule-binding domain. Mol Biol Cell 2008;19:2083-91. [Crossref] [PubMed]
- Gudmundsson KO, Thorsteinsson L, Sigurjonsson OE, et al. Gene expression analysis of hematopoietic progenitor cells identifies Dlg7 as a potential stem cell gene. Stem Cells 2007;25:1498-506. [Crossref] [PubMed]
- Tsou AP, Yang CW, Huang CY, et al. Identification of a novel cell cycle regulated gene, HURP, overexpressed in human hepatocellular carcinoma. Oncogene 2003;22:298-307. [Crossref] [PubMed]
- Huang YL, Chiu AW, Huan SK, et al. Prognostic significance of hepatoma-up-regulated protein expression in patients with urinary bladder transitional cell carcinoma. Anticancer Res 2003;23:2729-33. [PubMed]
- Stuart JE, Lusis EA, Scheck AC, et al. Identification of gene markers associated with aggressive meningioma by filtering across multiple sets of gene expression arrays. J Neuropathol Exp Neurol 2011;70:1-12. [Crossref] [PubMed]
- de Reyniès A, Assie G, Rickman DS, et al. Gene expression profiling reveals a new classification of adrenocortical tumors and identifies molecular predictors of malignancy and survival. J Clin Oncol 2009;27:1108-15. [Crossref] [PubMed]
- Fragoso MC, Almeida MQ, Mazzuco TL, et al. Combined expression of BUB1B, DLGAP5, and PINK1 as predictors of poor outcome in adrenocortical tumors: validation in a Brazilian cohort of adult and pediatric patients. Eur J Endocrinol 2012;166:61-7. [Crossref] [PubMed]
- Gomez CR, Kosari F, Munz JM, et al. Prognostic value of discs large homolog 7 transcript levels in prostate cancer. PLoS One 2013;8:e82833. [Crossref] [PubMed]
- Wang Q, Chen Y, Feng H, et al. Prognostic and predictive value of HURP in nonsmall cell lung cancer. Oncol Rep 2018;39:1682-92. [PubMed]
- Hewit K, Sandilands E, Martinez RS, et al. A functional genomics screen reveals a strong synergistic effect between docetaxel and the mitotic gene DLGAP5 that is mediated by the androgen receptor. Cell Death Dis 2018;9:1069. [Crossref] [PubMed]