Clinicopathological characteristics and prediction of cancer-specific survival in large cell lung cancer: a population-based study
Introduction
Lung cancer is the leading cause of cancer death worldwide (1,2). Large cell lung cancer (LCLC) constitutes a small proportion of lung cancer incidence, accounting for just 9% of all cases of lung cancer (3), and is a sub-type of non-small cell lung carcinoma (NSCLC) without glandular or squamous differentiation. The diagnosis of LCLC is often excluded from adenocarcinoma, squamous, and small cell lung cancer (3). LCLC can be found in any part of the lung, with men being more susceptible (4). The growth and spread of LCLC is quick, which makes it harder to treat. The features and survival outcomes of LCLC are scarcely reported due to its low incidence. As a result, the prognoses of LCLC is still unclear. In general, patients with a solid tumor can be classified by the American Joint Committee on Cancer (AJCC) staging system (5). However, the AJCC classification does not predict the survival of patients with rare or special cancer types (6).
As a kind of decision-making tool for patients with cancer, a nomogram can predict patient survival (7), and nomograms have also been widely used to stratify the treatment and evaluate outcomes (8). To date, however, reports on the use of a nomogram for patients with LCLC are still unavailable.
In this study, we examined the characteristics and prognosis of LCLC by using a United States population-based database. Also, we developed and independently validated a nomogram model based on the selected data to predict the prognosis of LCLC patients visually.
Methods
Ethics statement
We obtained permission to access the Surveillance, Epidemiology, and End Results (SEER) database with the reference number 10782-Nov2016. The informed consent for patients was not required in this study, as the research data are de-identified and publicly available.
Study population
We obtained data from the November 2013 submission of the SEER Research Data.
In this study, we extracted patients’ data from 2005 to 2014 in the SEER database by using SEER*Stat software version 8.3.5. As an authoritative source of cancer information, the SEER database contains U.S. cancer incidence and survival data, and there are 18 population-based registries included in the database, representing about 28% of the American population (9,10). In our study, patients diagnosed with large-cell carcinoma of the lung, according to the International Classification of Diseases for Oncology, Third Edition (ICD-O-3), (ICD-O-3:8012/3) were included. Patients with incomplete survival data were excluded. Since the recent version of the World Health Organization (WHO) classification for lung tumors used in the SEER was updated in 2004, we also excluded patients diagnosed before 2005.
Covariates
We collected patient demographic characteristics including age at diagnosis, race, sex, and marital status, and tumor clinicopathological features such as primary site, laterality, grade, AJCC stage (6th). Surgery information was also included. We used the cancer-specific survival (CSS) as the primary outcome, which was defined as a time interval from the diagnosis to death due to this cancer. Data of individuals who died and whose death was attributed to other causes or who were alive on the cutoff date (December, 2017) were censored.
Statistical analysis
Categorical variables for the demographic and clinical characteristics were reported as numbers and percentages. Continuous variables were expressed as the mean ± standard deviation (11). The random sampling strategy was used to split the primary cohort into the training cohort and validation cohort. In the training cohort, the optimal cutoff points for continuous variables were determined by using the maximally selected rank statistics in advance of the Cox proportional hazards regression (12,13). The CSS curve was plotted by the Kaplan-Meier method, log-rank test was used for the comparisons between CSS distributions, while proportional hazards regression analysis was used to evaluate the factors influencing CSS and to compute the hazard ratios and the 95% confidence intervals (95%CIs). A P value <0.05 (two-sided) was considered statistically significant. A nomogram was built to predict the probability of 1-year, 3-year, and 5-year CSS based on the final model of Cox proportional hazards regression. In the validation cohort, the total scores of the established nomogram for each patient were calculated. The performance of the scores was assessed by calculating the C-index and the average of the time-dependent area under the receiver operating characteristic curve (time-dependent AUC) in 1-year, 3-year, and 5-year, CSS. The calibration curves were also drawn to visualize the performance based on the total scores. All the statistical analyses were performed using R version 3.2.5 software (12).
Results
Summary of characteristics
A total of 4,963 of LCLC patients from the SEER database were identified; the median CSS time was 6 months, during which time all the patients were included in the study.
The demographic and tumor clinicopathological features for the eligible patients are summarized in Table 1, which shows that the mean age of the LCLC patients was 66.9±11.2. The male/female ratio was 1.37, and most of the recorded patients were White (79.27%). The main primary site labeled was up lobe (52.63%), and laterality was recorded more in the right-origin of primary (56.48%). The mean tumor size was 49.96±33.61, most of the diagnosis patients were in stage IV (49.77%), and 20.69% of the patients underwent surgery. Based on the random sampling strategy, all the patients were divided into a training cohort (n=342, 69.05%) and a validation cohort (n=1,536, 30.95%). The training and validation cohort were also listed in Table 1. All the characteristics were similar in the training cohort compared with the validation cohort. There were no observed significant CSS differences between the 2 cohorts (P=0.8).
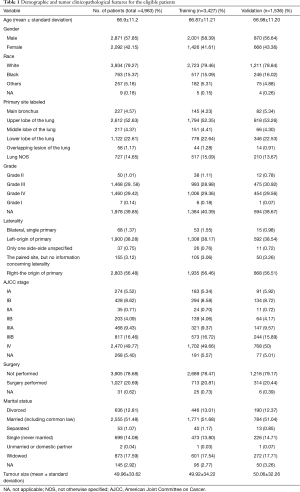
Full table
Development of the nomogram
Based on the maximally selected rank statistics, patients were classified into 2 group in terms of age (≤77 years, >77 years) and tumor size (≤41 mm, >41 mm) in the training cohort. In the univariate analysis, age, gender, race, primary site, laterality, AJCC stage, surgery, and marital status were found to be significantly correlated with the CSS in the training cohort (Table 2). The potential redundancy was removed according to the AIC-base backward selection procedure in the multivariate Cox proportional hazards regression analysis. The finally recruited independent prognostic factors, including age, sex, race, AJCC stage, surgery, and marital status, were used to construct the nomogram model. The hazard ratios (95% confidence interval, 95%CI) of nomogram parameters are shown in Table 3. The detailed scores of each independent prognostic factors are also listed in Table 3, and the nomogram is plotted in Figure 1. Patients were classified into 7 groups according to the nomogram scores. The CSS curve for these groups is shown in Figure 2.
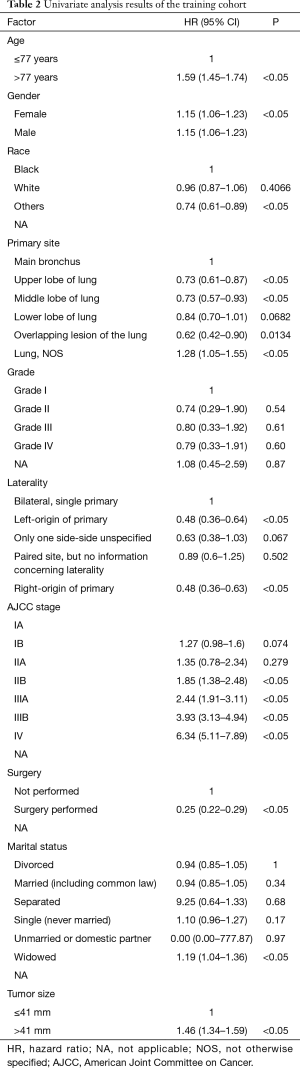
Full table
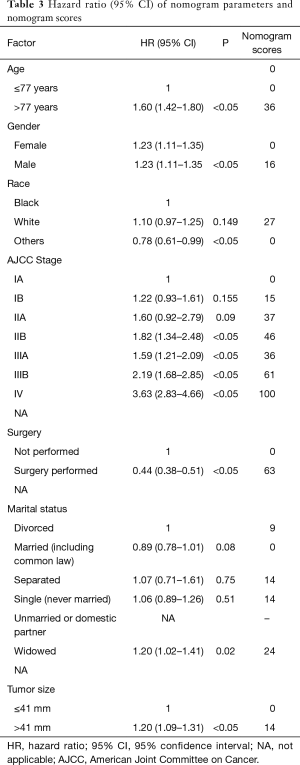
Full table
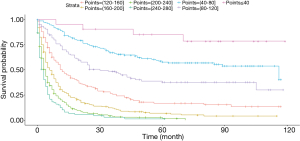
Validation of the nomogram
The C-index of the nomograms for predicting CSS was 0.701±0.01 in the validation cohort. The average of the time-dependent AUC in 1-year, 3-year, and 5-year CSS was 0.88. The calibration curve (Figure 3) for 1-year, 3-year, and 5-year CSS showed little gap between the predictions and actual outcomes in the validation cohort.
Discussion
In the present study, 4,936 LCLC patients were identified from the SEER database.
Nearly half of LCLC patients were diagnosed with stage IV, and only 20% of patients underwent surgery. This means that most of the LCLC cancer patients were diagnosed with advance stage and the optimal treatment opportunity was often missed. Therefore, an efficient diagnosis and treatment method are urgently needed.
In this study, the routinely available characteristics of the patients were extracted from the SEER database. Based on these, a nomogram was developed and confirmed (14). The model performance of the developed nomogram was confirmed by the calculated C-index, time-dependent AUC, and calibrated curves. Seven significant factors (age, sex, tumor size, AJCC stage, surgery, race, and marital status) were included in the nomogram. All the factors are routinely available in daily practice, which allows for the nomogram to be easily used in predicting an individual’s CSS and making treatment decisions for patients and clinicians.
In agreement with other types of NSCLC, the age and sex were all crucial predictors for CSS of LCLC (15). Old age and male patients were associated with a worse prognosis. According to Harrell’s guidelines, in this study, patients’ age was divided into 2 groups (16), and 77 years old was the best cutoff point. At present, a consistent conclusion has not been not reached for the CSS disparities of patients with lung cancer across different races (17). In general, better CSS outcomes would be seen in a race with higher health awareness, and the treatment in these races would be more active. In this study, the significantly different influence of race for LCLC patient’s CSS was seen both in univariable and multivariable analysis. The CSS outcome for other races was better than for the White race. Treatment activity might be a possible explanation for this phenomenon, and another reason might be the sample of the SEER database: small proportions of other races were collected in the database, which might have affected the statistical results for this study. As the most commonly used tumor-associated indices, the AJCC stage still contributed most to the established nomogram model, which is in line with other types of NSCLC (18). Tumor size is an essential indicator for the T stage; in this study, it was also found to be an independent risk factor for LCLC. It was confirmed that patients with a tumor size >41 mm show less CSS time than those with a tumor size ≤41 mm using the maximally selected rank statistics. Surgery is the primary treatment for most types of lung cancer. In this study, surgery was also found to be an essential treatment for LCLC in that patients with surgery had a significant decrease in cancer-specific death. Marital status has been confirmed to be associated with the CSS in a series of cancer (19-23). This phenomenon, in our present study, was consistent with the previous study in which married LCLC patients represented by lower nomogram scores had more CSS benefit compared with other types of marital statuses.
The nomogram validation is of great importance in the prevention of overfitting for the established model, and also crucial for model generalization (24). In the present study, an independent validation cohort was used for the validation of the nomogram. The calculated C-index (0.701±0.01) confirmed the discriminatory capacity of the established nomogram. Optimal consistency between the predicted CSS and actual observed CSS was seen in the calibration curves for 1-year, 3-year, and 5-year CSS. The time-dependent ROC for the validation cohort was acceptable enough to keep the AUC at a comparatively higher level (>0.5) in the prediction of CSS.
Still, some limitations should be considered in the current study. Firstly, since many vital factors identified in previous studies that influence the CSS of lung cancer in SEER database are lacking, many critical factors such as the chemotherapy, radiotherapy, smoking status, and performance status were not obtained in this study, and thus a more detailed understanding of the prognosis factors for LCLC could not be obtained. Additionally, the lack of these factors may affect the accuracy of the nomogram model. Thirdly, as a retrospective study, the selection bias could not be excluded, and thus a validation with a prospective clinical study is still needed.
Conclusions
This study summarized the clinical features and prognosis of LCLC by using a U.S. population-based cohort from the SEER database, and we found that sex, age, race, marital status, AJCC stage, surgery, and tumor size are all the independent prognostic factors of CSS for LCLC. Furthermore, a visual nomogram was developed to predict the CSS of LCLC. The discrimination of the nomogram was confirmed to be acceptable in the validation cohort. This nomogram can provide a more precise evaluation of CSS for LCLC patients and help clinicians to make individual management decisions.
Acknowledgments
Funding: This work was supported by the CAMS Innovation Fund for Medical Sciences (CIFMS) (Grants Nos. 2016-I2M-1-001, 2017-I2M-1-005, and 2017-I2M-1-003).
Footnote
Conflicts of Interest: All authors have completed the ICMJE uniform disclosure form (available at http://dx.doi.org/10.21037/jtd.2020.04.24). Dr. CL reports grants from CAMS Innovation Fund for Medical Sciences (Grants Nos. 2016-I2M-1-001, 2017-I2M-1-005, and 2017-I2M-1-003), during the conduct of the study; Dr. GL reports grants from CAMS Innovation Fund for Medical Sciences (Grants Nos. 2016-I2M-1-001, 2017-I2M-1-005, and 2017-I2M-1-003), during the conduct of the study; Dr. SQ reports grants from CAMS Innovation Fund for Medical Sciences (Grants Nos. 2016-I2M-1-001, 2017-I2M-1-005, and 2017-I2M-1-003), during the conduct of the study; Dr. WC reports grants from CAMS Innovation Fund for Medical Sciences (Grants Nos. 2016-I2M-1-001, 2017-I2M-1-005, and 2017-I2M-1-003), during the conduct of the study; Dr. YS reports grants from CAMS Innovation Fund for Medical Sciences (Grants Nos. 2016-I2M-1-001, 2017-I2M-1-005, and 2017-I2M-1-003), during the conduct of the study; Dr. YL reports grants from CAMS Innovation Fund for Medical Sciences (Grants Nos. 2016-I2M-1-001, 2017-I2M-1-005, and 2017-I2M-1-003), during the conduct of the study; Dr. YZ reports grants from CAMS Innovation Fund for Medical Sciences (Grants Nos. 2016-I2M-1-001, 2017-I2M-1-005, and 2017-I2M-1-003), during the conduct of the study; Dr. XZ reports grants from CAMS Innovation Fund for Medical Sciences (Grants Nos. 2016-I2M-1-001, 2017-I2M-1-005, and 2017-I2M-1-003), during the conduct of the study.
Ethical Statement: The authors are accountable for all aspects of the work in ensuring that questions related to the accuracy or integrity of any part of the work are appropriately investigated and resolved.
Open Access Statement: This is an Open Access article distributed in accordance with the Creative Commons Attribution-NonCommercial-NoDerivs 4.0 International License (CC BY-NC-ND 4.0), which permits the non-commercial replication and distribution of the article with the strict proviso that no changes or edits are made and the original work is properly cited (including links to both the formal publication through the relevant DOI and the license). See: https://creativecommons.org/licenses/by-nc-nd/4.0/.
References
- Wei J, Yan Y, Chen X, et al. The Roles of Plant-Derived Triptolide on Non-Small Cell Lung Cancer. Oncol Res 2019;27:849-58. [Crossref] [PubMed]
- Yan Y, Xu Z, Qian L, et al. Identification of CAV1 and DCN as potential predictive biomarkers for lung adenocarcinoma. Am J Physiol Lung Cell Mol Physiol 2019;316:L630-43. [Crossref] [PubMed]
- Sholl LM. Large-cell carcinoma of the lung: a diagnostic category redefined by immunohistochemistry and genomics. Curr Opin Pulm Med 2014;20:324-31. [Crossref] [PubMed]
- Monica V, Ceppi P, Righi L, et al. Desmocollin-3: a new marker of squamous differentiation in undifferentiated large-cell carcinoma of the lung. Mod Pathol 2009;22:709-17. [Crossref] [PubMed]
- Moitra D, Mandal RK. Automated AJCC (7th edition) staging of non-small cell lung cancer (NSCLC) using deep convolutional neural network (CNN) and recurrent neural network (RNN). Health Inf Sci Syst 2019;7:14.
- Liang W, Zhang L, Jiang G, et al. Development and validation of a nomogram for predicting survival in patients with resected non-small-cell lung cancer. J Clin Oncol 2015;33:861-9. [Crossref] [PubMed]
- Zhou H, Shen J, Zhang Y, et al. Risk of second primary malignancy after non-small cell lung cancer: a competing risk nomogram based on the SEER database. Ann Transl Med 2019;7:439. [Crossref] [PubMed]
- Asare EA, Liu L, Hess KR, et al. Development of a model to predict breast cancer survival using data from the National Cancer Data Base. Surgery 2016;159:495-502. [Crossref] [PubMed]
- Kuo TM, Mobley LR. How generalizable are the SEER registries to the cancer populations of the USA? Cancer Causes Control 2016;27:1117-26. [Crossref] [PubMed]
- Xie JC, Yang S, Liu XY, et al. Effect of marital status on survival in glioblastoma multiforme by demographics, education, economic factors, and insurance status. Cancer Med 2018;7:3722-42. [Crossref] [PubMed]
- Wang X, Xu Z, Chen X, et al. A tropomyosin receptor kinase family protein, NTRK2 is a potential predictive biomarker for lung adenocarcinoma. PeerJ 2019;7:e7125. [Crossref] [PubMed]
- Liu Y, Zhai X, Li J, et al. Is there an optimal time to initiate adjuvant chemotherapy to predict benefit of survival in non-small cell lung cancer? Chin J Cancer Res 2017;29:263-71. [Crossref] [PubMed]
- Wei S, Tian J, Song X, et al. Causes of death and competing risk analysis of the associated factors for non-small cell lung cancer using the Surveillance, Epidemiology, and End Results database. J Cancer Res Clin Oncol 2018;144:145-55. [Crossref] [PubMed]
- Wang Y, Yang Y, Chen Z, et al. Development and validation of a novel nomogram for predicting distant metastasis-free survival among breast cancer patients. Ann Transl Med 2019;7:537. [Crossref] [PubMed]
- Mao Q, Xia W, Dong G, et al. A nomogram to predict the survival of stage IIIA-N2 non-small cell lung cancer after surgery. J Thorac Cardiovasc Surg 2018;155:1784-92.e3. [Crossref] [PubMed]
- Harrell FE Jr, Califf RM, Pryor DB, et al. Evaluating the yield of medical tests. Jama 1982;247:2543-6. [Crossref] [PubMed]
- Varlotto JM, McKie K, Voland RP, et al. The Role of Race and Economic Characteristics in the Presentation and Survival of Patients With Surgically Resected Non-Small Cell Lung Cancer. Front Oncol 2018;8:146. [Crossref] [PubMed]
- Xiao HF, Zhang BH, Liao XZ, et al. Development and validation of two prognostic nomograms for predicting survival in patients with non-small cell and small cell lung cancer. Oncotarget 2017;8:64303-16. [Crossref] [PubMed]
- Wu Y, Ai Z, Xu G. Marital status and survival in patients with non-small cell lung cancer: an analysis of 70006 patients in the SEER database. Oncotarget 2017;8:103518-34. [Crossref] [PubMed]
- Feng Y, Dai W, Li Y, et al. The effect of marital status by age on patients with colorectal cancer over the past decades: a SEER-based analysis. Int J Colorectal Dis 2018;33:1001-10. [Crossref] [PubMed]
- Li Z, Wang K, Zhang X, et al. Marital status and survival in patients with rectal cancer: A population-based STROBE cohort study. Medicine (Baltimore) 2018;97:e0637. [Crossref] [PubMed]
- Huang TB, Zhou GC, Dong CP, et al. Marital status independently predicts prostate cancer survival in men who underwent radical prostatectomy: An analysis of 95,846 individuals. Oncol Lett 2018;15:4737-44. [PubMed]
- Wang H, Wang L, Kabirov I, et al. Impact of marital status on renal cancer patient survival. Oncotarget 2017;8:70204-13. [Crossref] [PubMed]
- Iasonos A, Schrag D, Raj GV, et al. How to build and interpret a nomogram for cancer prognosis. J Clin Oncol 2008;26:1364-70. [Crossref] [PubMed]