A prognostic 11-DNA methylation signature for lung squamous cell carcinoma
Introduction
Lung cancer is the most frequent malignancy and the leading cause of cancer death worldwide (1) Lung squamous cell carcinoma (LUSC) is the second frequent subtype of lung cancer, accounts for approximately 30% (2,3). Although the progress has been made in early diagnosis and therapy, the 5-year survival of LUSC patients remains dissatisfactory. There is still a lack of effective biomarkers for identifying patients with high risk of recurrence and poor prognosis. Hence, there is an urgent need to find effective biomarkers to improve the ability of clinical prognosis prediction and make individualized therapy decisions.
Emerging studies have indicated that epigenetics plays a vital role in the occurrence, development, therapy response, and outcome of human tumors (4,5). The occurrence and development of cancer have been accompanied by abnormal DNA methylation, which has great potential as a biomarker of prognosis (6). For instance, p16 methylation induced paclitaxel resistance in non-small cell lung cancer (NSCLC), so it can predict paclitaxel chemosensitivity (7). KLF2 region 4 hypermethylation led to the downregulation of KLF2 and promoted the proliferation and metastasis in NSCLC cells (8). Downregulation of miR-1247 by DNA methylation promoted invasion and migration of NSCLC by targeting STMNI (9). Moreover, predictive models based on DNA methylation sites have been constructed in some tumors, such as ovarian serous cystadenocarcinoma, cutaneous melanoma, gastric adenocarcinoma and choroid plexus tumor (10-13). However, the prediction model based on DNA methylation sites remains to be constructed in LUSC.
In our present study, we aimed to construct a novel prognostic DNA methylation signature related to patient’s overall survival. The DNA methylation and follow-up data were downloaded from The Cancer Genome Atlas (TCGA) dataset. We performed several statistical methods, including univariate Cox regression, the least absolute shrinkage and selection operator (LASSO), and multivariate Cox regression methods to reduce dimensionality. As a result, an independent prognostic model based on 11-DNA methylation sites was successfully constructed. Besides, to improve the clinical practicability of the risk prognosis model, the metastasis stage and tobacco smoking history were integrated into the 11-DNA methylation signature and the nomogram was constructed.
Methods
Collection of DNA methylation and clinical data from TCGA and differential DNA methylation sites selection
Genome-wide DNA methylation data (level 3) and corresponding follow-up data of LUSC were downloaded from the TCGA dataset (http://cancergenome.nih.gov/). The DNA methylation data were detected by Infinium HumanMethylation450 BeadChip. Differential DNA methylation sites were identified between LUSC tissues and paracancerous tissues using the limma package (version 3.34.7; https://bioconductor.org/packages/release/bioc/html/limma.html). The selection criteria were fold change >2 or <0.5 and false discovery rate (FDR) <0.01. After removing tissues without survival records and follow-up time, filtered tissues were analyzed in the following study.
Development of DNA methylation signature in survival prediction
LUSC patients were classified into training set and testing set by random grouping method. All initial analyses were performed in the training set to construct a signature based on the DNA methylation site and validated the signature in the testing set. The DNA methylation sites associated with the overall survival of LUSC patients were screened using univariate Cox proportional hazard analysis with P<0.05 as statistical significance. LASSO analysis is a high-dimensional indicator regression method, which obtains a more refined model by compressing some regression coefficients. LASSO analysis was used to screen the critical DNA methylation sites from the significant DNA methylation sites in univariate Cox regression analysis using R with glmnet package (Version 3.0-2, https://CRAN.R-project.org/package=glmnet). Thus, we performed multivariate Cox regression, stepwise regression, to reduce dimensionality and establish a risk score formula weighted by the corresponding coefficients. The univariate and multivariate cox regression analysis used survival package (Version 2.41-1, http://bioconductor.org/packages/survivalr/) in R language. The risk score of each patient in training set was calculated according to the above formula. According to the median value, patients were classified into low- and high-risk groups. Survival difference between the low- and high-risk group was assessed by the Kaplan-Meier (K-M) survival analysis using R with survival package (Version 2.41-1, http://bioconductor.org/packages/survivalr/). To evaluate the predictive performance at 5 years of the DNA methylation signature, the time-dependent receiver operating characteristic (ROC) curve was performed using R with survivalROC package (Version 1.0.3, https://CRAN.R-project.org/package=survivalROC). Subsequently, we perform K-M survival analysis and ROC analysis to evaluate predictive accuracy of this signature in the testing set based on the same cutoff value. The area under the curve (AUC) is used as the evaluation criterion of the signature.
Construction and evaluation of nomogram
We performed multivariate Cox regression analysis to examine whether constructed DNA methylation signature is independent of other clinical data, consisting of age, gender, tumor stage, TNM stage, and tobacco smoking history. According to the results of multivariate Cox regression analysis, we constructed a nomogram for individualized prediction of overall survival to predict 1-, 3-, and 5-year overall survival using R with rms package (Version 5.1-4, https://CRAN.R-project.org/package=rms). Then, we performed ROC curve to appraise the predictive performance of this nomogram and only the DNA methylation signature (5-year survival).
Results
Data gathering and differential methylation analysis
A total of 412 samples with 485,577 DNA methylation sites were acquired from the TCGA dataset, including 370 LUSC tissues and 42 paracancerous tissues. Among them, 363 LUSC samples with clinical follow-up information were further randomly divided into two groups, 183 patients as a training set and 180 patients as a testing set. The clinical data of age, gender, race, tumor stage, TNM stage, and tobacco smoking history was summarized (Table S1). Compared with the paracancerous tissues, 15,343 differential DNA methylation sites were selected in LUSC tissues using fold change >2 or <0.5 and FDR <0.01 as the criteria.
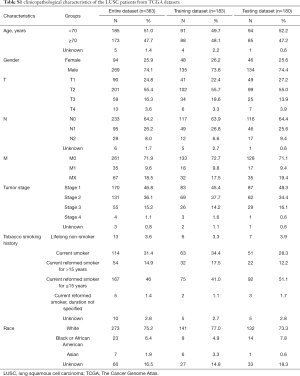
Full table
DNA methylation signature establishment and validation
As showed in the workflow diagram (Figure 1), we used the training set to construct DNA methylation signature and validated the predictive ability of signature in the testing set. First, we carried out the univariate Cox regression to filter DNA methylation sites associated with overall survival of LUSC patients in training set. Then, 392 DNA methylation sites were significantly associated with overall survival of patients (P<0.01). Next, these selected DNA methylation sites were put into LASSO analysis. Therefore, 44 DNA methylation sites were selected as critical sites that were of significance in univariate analysis (Figure 2). Multivariate Cox regression was performed on these 44 DNA methylation sites, stepwise regression and screening, and a risk prognosis model including 11-DNA methylation sites was determined as the optimal risk prognosis formula to predict overall survival (Table 1). The genes corresponding with these 11-DNA methylation sites were RASSF6 (Ras association domain family member 6), LHX5 (LIM homeobox 5), ZNF773 (zinc finger protein 773), HES7 (hes family bHLH transcription factor 7), APOBEC3C (apolipoprotein B mRNA editing enzyme catalytic subunit 3C), INSM2 (INSM transcriptional repressor 2), RPS18 (ribosomal protein S18), SPC25 (SPC25 component of NDC80 kinetochore complex), TRIM71 (tripartite motif containing 71), and ISL2 (ISL LIM homeobox 2), except for cg20565374. The correlation between the methylation degree of the DNA methylated sites screened and their corresponding gene expression was also analyzed (Figure S1).
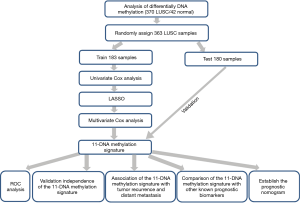
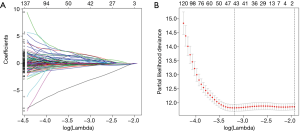
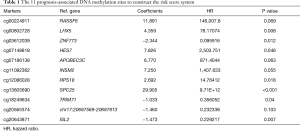
Full table
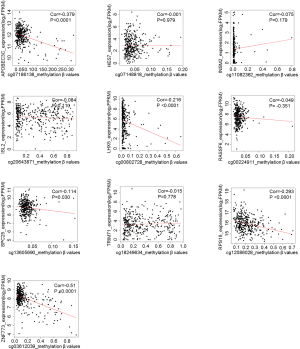
Based on the corresponding coefficients of the prognostic methylation β-values, a risk score formula was generated for predicting prognosis. Risk score =11.891× β-value of cg00224911 + 4.359 × β-value of cg00802728 − 2.344 × β-value of cg03612039 + 7.826 × β-value of cg07148818 + 6.770 × β-value of cg07186138 + 7.250 × β-value of cg11082362 + 2.692 × β-value of cg12086028 + 29.905 × β-value of cg13605690 − 1.033 × β-value of cg18249634 − 1.460 × β-value of cg20565374 − 1.473 × β-value of cg20643871. Cg00224911, cg00802728, cg07148818, cg07186138, cg11082362, cg12086028 and cg13605690 were negative related to overall survival in LUSC patients while cg03612039, cg18249634, cg20565374 and cg20643871 were positive factors. To evaluate the predicted performance of 11-DNA methylation signature, patients were classified into high-risk (N=91) and low-risk (N=92) groups using the median score as the threshold. First, the distribution of risk score, survival status, and β-value of methylation sites was analyzed in the training set (Figure 3A), and then confirmed in the testing set (Figure 3B). We analyzed the β-value of each methylation site in the signature of the high- and low-risk groups in the training set (Figure S2). K-M survival curves confirmed that the risk score was significantly related to overall survival and AUC is 0.787 (Figure 3C). Subsequently, the 11-DNA methylation signature was evaluated in testing set. Using the same risk score formula and threshold value, patients in testing set were divided into two groups: high-risk group (N=103) and low-risk group (N=77). The high-risk group also had a shorter survival time, and AUC was 0.750 (Figure 3D). The results demonstrated that our 11-DNA methylation signature performed significant sensitivity and specificity in assessing LUSC patients’ overall survival.
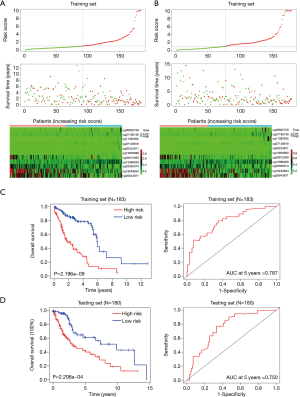
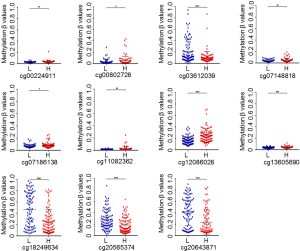
Detection of predicted power of 11-DNA methylation signature in different clinical characteristics
A crucial characteristic of a great prognostic signature should be independent or added to the clinical pathology prognostic factors currently in use. Clinicopathologic characteristics, including patients’ age, gender, tumor stage, TNM stage, and tobacco smoking history, have been considered as chief prognostic factors for patients with LUSC. In order to evaluate the independence and reliability of the 11-DNA methylation signature, patients were regrouped based on different clinical pathology features. Several factors were related to prognostic survival, consisting of age, gender, tumor stage, TNM stage, and tobacco smoking history. Age and gender were related to prognosis in NSCLC patients (14,15). All LUSC patients were classified into two groups according to their initial diagnosis age: <70 (N=185) and ≥70 (N=173), to analyze the prognostic predictive effect of this 11-DNA methylation signature in patients of different age groups. K-M curves suggested that overall survival time of high-risk group was worse in both age cohorts, with AUC values of 0.789 and 0.743, respectively (Figure S3A), indicating that the 11-DNA methylation signature was independent of age. Based on patients’ gender, patients were classified into 269 males and 94 females. The overall survival was significantly different between high- and low-risk groups, and AUC in male and female cohorts was 0.774 and 0.736, respectively (Figure S3B). The prognosis of patients in T1 and T2 was significantly better than patients in T3 and T4 (16). Compared with low-risk patients, the overall survival time of high-risk patients was significantly shortened, and the AUC in T1 and T2 (N=291) was 0.771. Nevertheless, in T3 and T4 (N=72), there was no significant difference in overall survival between the high- and low-risk groups (Figure S4A). Given that distant metastasis or lymph node metastasis can seriously affect the prognosis of patients, we regrouped patients according to whether the tumor has lymph node metastasis or distant metastasis. K-M and ROC analyses indicated that the prognosis of high-risk groups was significantly worse than low-risk groups (Figure 4A,B). The above results suggested that this 11-DNA methylation signature provides a superior reference for different distant metastasis or lymph node metastasis cohorts due to the effectiveness of risk stratification. Compared with early lung cancer, advanced lung cancer is more prone to recurrence and shorter survival time (17). As for tumor stage, we evaluated the predictive power of this 11-DNA methylation signature in stage 1 (N=170), stage 2 (N=131), stages 3 and 4 (N=59). In stages 1 and 2, the high-risk patients had obviously shorter overall survival, and AUC values in stages 1 and 2 cohorts were 0.774 and 0.762, respectively (Figure S4B). However, there was no significant difference in the overall survival of the high- and low-risk groups in stages 3 and 4, probably due to small numbers (Figure S4B). Tobacco serves as an important risk factor for NSCLC, approximately 80% of which is associated with smoking that closely related to DNA methylation (18-20). Based on the patient’s tobacco smoking history, patients were classified into three groups: current smoker (N=114), current reformed smoker for >15 years (N=54) and current reformed smoker for ≤15 years (N=167), and then to analyze the prognostic predictive efficiency of the 11-DNA methylation signature in patients of different tobacco smoking history. As shown, the difference in the overall survival between low- and high-risk groups was also significant, and AUC values of different smoking history groups were greater than 0.75 (Figure 4C). Results of K-M and ROC analyses according to various regrouping methods were also summarized in Table S2. The above results suggested that this 11-DNA methylation signature showed satisfactory availability when patients were regrouped according to different clinical pathology features, indicating that the 11-DNA methylation signature was an independent and applicative prognostic predictor of patients’ survival.
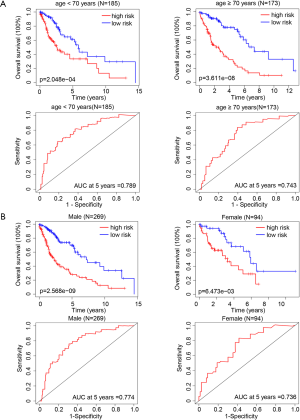
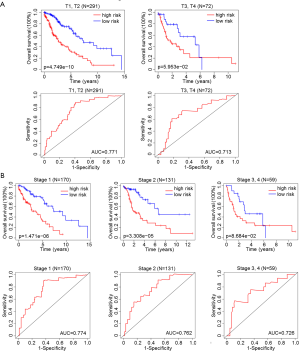
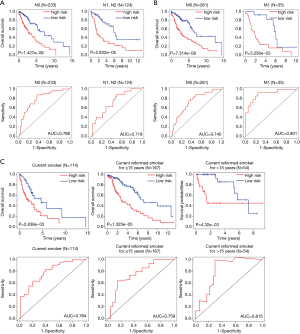
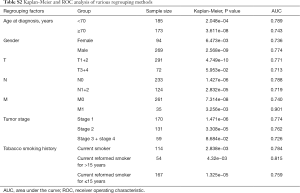
Full table
Establishment of the nomogram
According to the results from univariate analysis, histologic grade, tumor stage, lymph node stage, metastasis stage, and tobacco smoking history were significantly related to overall survival of patients with LUSC (Table 2). Through multivariate analysis of the above factors, metastasis stage and tobacco smoking history and the risk score, independent and stable prognostic factor (Table 2), were used to construct a nomogram (Figure 5A). Compared with the 11-DNA methylation signature, the nomogram shows higher accuracy of 5-year survival prediction (AUC =0.811, Figure 5B).
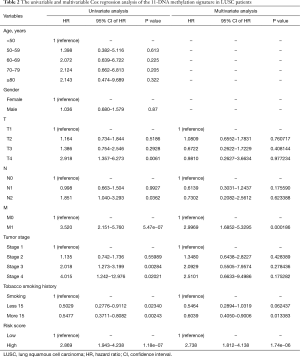
Full table
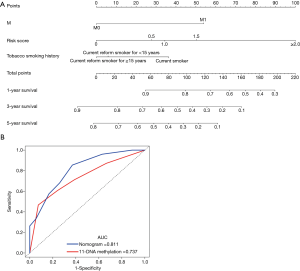
Association of the 11-DNA methylation signature with tumor recurrence and distant metastasis
We next studied the utility of the risk score in assessing tumor recurrence and distant metastasis of LUSC. Clinical and demographic features, including age, gender, race, tumor stage, TNM stage and tobacco smoking history were included in the analysis. The risk score of patients with metastasis (N=32) was significantly higher than those without metastasis (N=253) (Figure 6A). Similarly, the risk scores of patients with tumor recurrence (N=89) were significantly higher than those with no tumor recurrence (N=206) (Figure 6B). Collectively, these results indicate that risk scores can be used to predict tumor recurrence, metastasis, and surveillance.
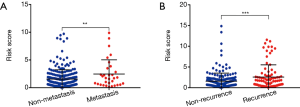
Comparison of the 11-DNA methylation signature with other known prognostic biomarkers
Previous studies have focused on building predictive signatures using protein-coding genes or miRNAs or lncRNAs. For instance, cathepsin B (CTSB) is a predictor of poor prognosis and promotes tumor metastasis and might have the potential to be a therapeutic target for LUSC (21). Zhang et al. constructed a prognostic signature using 17 mRNAs and a miRNA in LUSC (22). Based on lncRNA expression, Wang et al. identified eight lncRNAs as a prognostic signature (23) and Tang et al. constructed a predictive 5-lncRNA model (24). PD-L1 can severe as a poor prognostic signature in LUSC patients (25). CD271 promoted cell proliferation and was related to the poor prognosis of LUSC (26). RBMS3, as a tumor suppressor gene, inhibited the occurrence and development of LUSC (27). The expression of RRM1 and ERCC1 was related to the better prognosis of patients with LUSC (28). Li et al. identified methylation-driven genes and used four methylation driving genes GCSAM, GPR75, NHLRC1 and TRIM58 as prognostic indicators of LUSC. They used the average methylation level of the methylation-driven gene to build a prognostic model, instead of methylation sites (29). To evaluate whether our DNA methylation signature has a robust and reliable performance advantage, we compared the sensitivity and specificity of our DNA methylation signature with other known prognostic signatures in the same 363 patients with LUSC (Figure 7). According to the results of the ROC analysis, the predicted performance at 5 years of our 11-DNA methylation signature was better than other known prognostic biomarkers, including mRNAs, miRNAs, and lncRNAs. All the above results showed that the 11-DNA methylation signature had better stability and reliability and was currently the best predictor of overall survival in predicting LUSC patients.
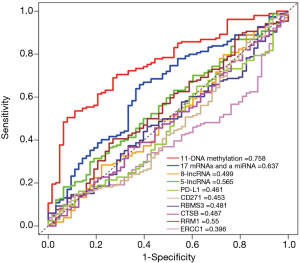
Discussion
Despite advances in the prevention, diagnosis, and therapy of LUSC over the past few decades, the 5-year survival rate remains low, less than 15 percent (17). Therefore, the prognostic prediction of LUSC patients is critical to the selection and improvement of appropriate treatment options. To distinguish between high- and low-risk patients for more effective management, previous studies developing a series of molecular biomarkers related to the prognosis of LUSC patients have focused on protein-coding genes or miRNAs or lncRNAs while ignoring the impact of methylation on patient’s survival. With the deepening of epigenetic research, increasing evidence has shown that DNA methylation is critical to gene regulation and is early events of some tumors. DNA methylation is one of the earliest detectable neoplastic changes that give it a unique advantage as cancer diagnosis and prognosis biomarkers (30-32). In addition, a prognostic signature formed by combining multiple DNA methylation sites has higher sensitivity and specificity than a single DNA methylation site (33). Our study emphasized the potential role for a combination of epigenetic biomarkers in improving prognosis prediction and providing tailored therapeutic decisions, as well as providing alternative biomarkers and therapeutic targets for LUSC patients.
Our study first identified differential methylation sites according to genome-wide DNA methylation analysis. We performed COX regression and ROC analysis to identify an 11-DNA methylation signature that was significantly related to overall survival of LUSC patients. To detect the predictive performance and independence of the 11-methylation signature, patients were regrouped based on different clinicopathological features (age, gender, tumor stage, TNM stage, and tobacco smoking history). We used K-M and ROC analysis to estimate the prognostic ability of the 11-DNA methylation signature in different subgroups. Based on the risk scores of the 11-DNA methylation signature, we performed risk stratification and survival prediction for LUSC patients. In addition, comparison of our 11-DNA methylation signature with other known prognostic biomarkers indicates that it has significantly higher sensitivity and specificity in the prognosis prediction of LUSC. Among these 11 methylation sites, 10 sites have corresponding reference genes. RASSF6 is a tumor suppressor with methylation of its promoter region leading to decreased expression, thereby promoting melanoma development and brain metastasis (34,35). ZNF773 has a higher level of DNA methylation in human papillomavirus-related oropharyngeal squamous cell carcinoma compared to normal samples (36). HES7 is a biomarker gene for early epithelial-mesenchymal transition in lung adenocarcinoma (37). SPC25 increases tumor stem cell characteristics in NSCLC and pancreatic cancer, and enhances cell proliferation and poor prognosis of breast cancer (38-40). TRIM71 promotes cell proliferation in NSCLC and hepatocellular carcinoma (41,42). Nevertheless, the relationship between five of these ten corresponding reference genes (LHX5, APOBEC3C, RPS18, ISL2, and INSM2) and tumor biology and the related molecular mechanisms have not been studied.
Gene expression is affected by epigenetic changes, and inactivation of tumor suppressor genes caused by DNA methylation is related to occurrence and development in multiple tumors, consisting of LUSC (43,44). Although DNA methylation can affect gene regulation, there are a few exceptions (45,46). In our signature, the expression of APOBEC3C, LHX5, SPC25, RPS18, and ZNF773 were negatively related to the methylation levels (P<0.05), but no association between the expression and the methylation level of other five genes (HES7, INSM2, ISL2, RASSF6, and TRIM71). Further, we will focus on verifying the biological functions of these 11-DNA methylation sites and their corresponding genes through more experiments, which may provide more targets and therapeutic decisions.
To improve a more sensitive and specific prognostic signature for LUSC, we constructed a prognostic nomogram that combines the 11-DNA methylation signature with distant metastasis of the patient’s tumor and smoking history and demonstrates more satisfied predictive performance. To apply the model to the clinic in the future, more clinical investigations are needed to assess the robustness of this 11-DNA methylation signature. It is undeniable that there may be some deviations in the process of constructing a model by selecting prognostic-related DNA methylation sites. The correlation analysis suggests that subsequent research should focus on the combination of mRNA and DNA methylation signature to construct better prognostic biomarkers.
Conclusions
In conclusion, we constructed the first risk prognosis model based on DNA methylation site in LUSC, which had better stability and reliability and was currently the best predictor of overall survival in predicting LUSC patients. In addition, in order to better apply the risk prognosis model to clinical decision-making, a nomogram integrating the DNA methylation signature, metastasis stage, and tobacco smoking history was developed.
Acknowledgments
Funding: This study was supported by grants from the National Natural Science Foundation of China (No. 81872197 and No. 81672616); supported by grants from Guangdong Natural Science Funds for Distinguished Young Scholars (No. 2016A030306003); supported by Guangdong Special Support Program (No. 2017TQ04R809); supported by Guangzhou key medical discipline construction project fund; supported by grants from Science and Technology Program of Guangzhou, China (No. 201710010100).
Footnote
Conflicts of Interest: All authors have completed the ICMJE uniform disclosure form (available at http://dx.doi.org/10.21037/jtd.2020.03.31). The authors have no conflicts of interest to declare.
Ethical Statement: The authors are accountable for all aspects of the work in ensuring that questions related to the accuracy or integrity of any part of the work are appropriately investigated and resolved.
Open Access Statement: This is an Open Access article distributed in accordance with the Creative Commons Attribution-NonCommercial-NoDerivs 4.0 International License (CC BY-NC-ND 4.0), which permits the non-commercial replication and distribution of the article with the strict proviso that no changes or edits are made and the original work is properly cited (including links to both the formal publication through the relevant DOI and the license). See: https://creativecommons.org/licenses/by-nc-nd/4.0/.
References
- Bray F, Ferlay J, Soerjomataram I, et al. Global cancer statistics 2018: GLOBOCAN estimates of incidence and mortality worldwide for 36 cancers in 185 countries. CA Cancer J Clin 2018;68:394-424. [Crossref] [PubMed]
- Network TCGAR. Comprehensive genomic characterization of squamous cell lung cancers. Nature 2012;489:519-25. [Crossref] [PubMed]
- Siegel R, Naishadham D, Jemal A. Cancer statistics, 2013. CA Cancer J Clin 2013;63:11-30. [Crossref] [PubMed]
- Cai L, Bai H, Duan J, et al. Epigenetic alterations are associated with tumor mutation burden in non-small cell lung cancer. J Immunother Cancer 2019;7:198. [Crossref] [PubMed]
- Azmi AS, Li Y, Aboukameel A, et al. DNA-Methylation-Caused Downregulation of miR-30 Contributes to the High Expression of XPO1 and the Aggressive Growth of Tumors in Pancreatic Ductal Adenocarcinoma. Cancers (Basel) 2019. [Crossref] [PubMed]
- Zhou F, Tao G, Chen X, et al. Methylation of OPCML promoter in ovarian cancer tissues predicts poor patient survival. Clin Chem Lab Med 2014;52:735-42. [Crossref] [PubMed]
- Liu Z, Lin H, Gan Y, et al. P16 Methylation Leads to Paclitaxel Resistance of Advanced Non-Small Cell Lung Cancer. J Cancer 2019;10:1726-33. [Crossref] [PubMed]
- Jiang W, Xu X, Deng S, et al. Methylation of kruppel-like factor 2 (KLF2) associates with its expression and non-small cell lung cancer progression. Am J Transl Res 2017;9:2024-37. [PubMed]
- Zhang J, Fu J, Pan Y, et al. Silencing of miR-1247 by DNA methylation promoted non-small-cell lung cancer cell invasion and migration by effects of STMN1. Onco Targets Ther 2016;9:7297-307. [Crossref] [PubMed]
- Guo W, Zhu L, Zhu R, et al. A four-DNA methylation biomarker is a superior predictor of survival of patients with cutaneous melanoma. Elife 2019. [Crossref] [PubMed]
- Guo W, Zhu L, Yu M, et al. A five-DNA methylation signature act as a novel prognostic biomarker in patients with ovarian serous cystadenocarcinoma. Clin Epigenetics 2018;10:142. [Crossref] [PubMed]
- Pienkowska M, Choufani S, Turinsky AL, et al. DNA methylation signature is prognostic of choroid plexus tumor aggressiveness. Clin Epigenetics 2019;11:117. [Crossref] [PubMed]
- Hu S, Yin X, Zhang G, et al. Identification of DNA methylation signature to predict prognosis in gastric adenocarcinoma. J Cell Biochem 2019. [Epub ahead of print]. [PubMed]
- de Groot P, Munden RF. Lung cancer epidemiology, risk factors, and prevention. Radiol Clin North Am 2012;50:863-76. [Crossref] [PubMed]
- Torre LA, Siegel RL, Jemal A. Lung Cancer Statistics. Adv Exp Med Biol 2016;893:1-19. [Crossref] [PubMed]
- Yoon JY, Sigel K, Martin J, et al. Evaluation of the Prognostic Significance of TNM Staging Guidelines in Lung Carcinoid Tumors. J Thorac Oncol 2019;14:184-92. [Crossref] [PubMed]
- Chansky K, Detterbeck FC, Nicholson AG, et al. The IASLC Lung Cancer Staging Project: External Validation of the Revision of the TNM Stage Groupings in the Eighth Edition of the TNM Classification of Lung Cancer. J Thorac Oncol 2017;12:1109-21.
- Hopkins JM, Evans HJ. Cigarette smoke-induced DNA damage and lung cancer risks. Nature 1980;283:388-90. [Crossref] [PubMed]
- D'Addario G, Felip E. Non-small-cell lung cancer: ESMO clinical recommendations for diagnosis, treatment and follow-up. Ann Oncol 2009;20 Suppl 4:68-70. [Crossref] [PubMed]
- Herceg Z, Ambatipudi S. Smoking-associated DNA methylation changes: no smoke without fire. Epigenomics 2019;11:1117-9. [Crossref] [PubMed]
- Gong F, Peng X, Luo C, et al. Cathepsin B as a potential prognostic and therapeutic marker for human lung squamous cell carcinoma. Mol Cancer 2013;12:125. [Crossref] [PubMed]
- Zhang J, Bing Z, Yan P, et al. Identification of 17 mRNAs and a miRNA as an integrated prognostic signature for lung squamous cell carcinoma. J Gene Med 2019;21:e3105. [Crossref] [PubMed]
- Wang Y, Yang F, Zhuang Y. Identification of a progression-associated long non-coding RNA signature for predicting the prognosis of lung squamous cell carcinoma. Exp Ther Med 2018;15:1185-92. [PubMed]
- Tang RX, Chen WJ, He RQ, et al. Identification of a RNA-Seq based prognostic signature with five lncRNAs for lung squamous cell carcinoma. Oncotarget 2017;8:50761-73. [Crossref] [PubMed]
- Takada K, Okamoto T, Toyokawa G, et al. The expression of PD-L1 protein as a prognostic factor in lung squamous cell carcinoma. Lung Cancer 2017;104:7-15. [Crossref] [PubMed]
- Mochizuki M, Nakamura M, Sibuya R, et al. CD271 is a negative prognostic factor and essential for cell proliferation in lung squamous cell carcinoma. Lab Invest 2019;99:1349-62. [Crossref] [PubMed]
- Liang YN, Liu Y, Meng Q, et al. RBMS3 is a tumor suppressor gene that acts as a favorable prognostic marker in lung squamous cell carcinoma. Med Oncol 2015;32:459. [Crossref] [PubMed]
- Zheng Z, Chen T, Li X, et al. DNA synthesis and repair genes RRM1 and ERCC1 in lung cancer. N Engl J Med 2007;356:800-8. [Crossref] [PubMed]
- Li Y, Gu J, Xu F, et al. Novel methylation-driven genes identified as prognostic indicators for lung squamous cell carcinoma. Am J Transl Res 2019;11:1997-2012. [PubMed]
- Baylin SB, Jones PA. Epigenetic Determinants of Cancer. Cold Spring Harb Perspect Biol 2016. [Crossref] [PubMed]
- Irizarry RA, Ladd-Acosta C, Wen B, et al. The human colon cancer methylome shows similar hypo- and hypermethylation at conserved tissue-specific CpG island shores. Nat Genet 2009;41:178-86. [Crossref] [PubMed]
- Baylin SB, Jones PA. A decade of exploring the cancer epigenome - biological and translational implications. Nat Rev Cancer 2011;11:726-34. [Crossref] [PubMed]
- Dai W, Teodoridis JM, Zeller C, et al. Systematic CpG islands methylation profiling of genes in the wnt pathway in epithelial ovarian cancer identifies biomarkers of progression-free survival. Clin Cancer Res 2011;17:4052-62. [Crossref] [PubMed]
- Allen NP, Donninger H, Vos MD, et al. RASSF6 is a novel member of the RASSF family of tumor suppressors. Oncogene 2007;26:6203-11. [Crossref] [PubMed]
- Mezzanotte JJ, Hill V, Schmidt ML, et al. RASSF6 exhibits promoter hypermethylation in metastatic melanoma and inhibits invasion in melanoma cells. Epigenetics 2014;9:1496-503. [Crossref] [PubMed]
- Ren S, Gaykalova D, Wang J, et al. Discovery and development of differentially methylated regions in human papillomavirus-related oropharyngeal squamous cell carcinoma. Int J Cancer 2018;143:2425-36. [Crossref] [PubMed]
- Song J, Wang W, Wang Y, et al. Epithelial-mesenchymal transition markers screened in a cell-based model and validated in lung adenocarcinoma. BMC Cancer 2019;19:680. [Crossref] [PubMed]
- Wang Q, Zhu Y, Li Z, et al. Up-regulation of SPC25 promotes breast cancer. Aging (Albany NY) 2019;11:5689-704. [PubMed]
- Chen J, Chen H, Yang H, et al. SPC25 upregulation increases cancer stem cell properties in non-small cell lung adenocarcinoma cells and independently predicts poor survival. Biomed Pharmacother 2018;100:233-9. [Crossref] [PubMed]
- Cui F, Tang H, Tan J, et al. Spindle pole body component 25 regulates stemness of prostate cancer cells. Aging (Albany NY) 2018;10:3273-82. [Crossref] [PubMed]
- Ren H, Xu Y, Wang Q, et al. E3 ubiquitin ligase tripartite motif-containing 71 promotes the proliferation of non-small cell lung cancer through the inhibitor of kappaB-alpha/nuclear factor kappaB pathway. Oncotarget 2017;9:10880-90. [Crossref] [PubMed]
- Chen YL, Yuan RH, Yang WC, et al. The stem cell E3-ligase Lin-41 promotes liver cancer progression through inhibition of microRNA-mediated gene silencing. J Pathol 2013;229:486-96. [Crossref] [PubMed]
- Herman JG, Baylin SB. Gene silencing in cancer in association with promoter hypermethylation. N Engl J Med 2003;349:2042-54. [Crossref] [PubMed]
- Wu CY, Tseng RC, Hsu HS, et al. Frequent down-regulation of hRAB37 in metastatic tumor by genetic and epigenetic mechanisms in lung cancer. Lung Cancer 2009;63:360-7. [Crossref] [PubMed]
- Croes L, Beyens M, Fransen E, et al. Large-scale analysis of DFNA5 methylation reveals its potential as biomarker for breast cancer. Clin Epigenetics 2018;10:51. [Crossref] [PubMed]
- Phelps DL, Borley JV, Flower KJ, et al. Methylation of MYLK3 gene promoter region: a biomarker to stratify surgical care in ovarian cancer in a multicentre study. Br J Cancer 2017;116:1287-93. [Crossref] [PubMed]