Identification key genes, key miRNAs and key transcription factors of lung adenocarcinoma
Introduction
Lung cancer is one of the most common cancers and the leading causes of cancer-related death worldwide (1). Lung adenocarcinoma (LUAD) is a major histological type of lung cancer. Despite recent advances in technology of molecular diagnosis and therapy, the prognosis of LUAD is still not optimistic, and the risk of metastasis and recurrence is still high (2). Therefore, it is necessary to search novel biomarkers and therapy targets for LUAD diagnosis and prognosis.
Transcription factors (TFs), regulate gene expression by binding to specific DNA sequences at the transcriptional level, have an important role in the pathogenesis and development of cancer. The recent study showed that four TFs (ASCL1, NeuroD1, YAP1 and POU2F3) play critical roles in small cell lung cancer (3). The TF family p53/p63/p73 plays a critical role in Squamous Cancer Pathogenesis by regulating homeostasis of squamous epithelium (4).
MicroRNAs (miRNAs) are endogenous small non-coding RNAs (18–22 nucleotide-long) that mainly inhibit gene expression at the post-transcriptional level by directly binding to the 3'UTR of the target mRNAs. MiRNAs play an important role in regulating a range of biological functions, including proliferation, apoptosis, cell survival, tumor growth and metastasis. Several miRNAs have been identified as novel biomarkers and therapy targets of cancer in recent years (5-7). For example, microRNA-193b, act as a tumor suppressor, have antileukemic efficacy and can improve prognostic of acute myeloid leukemia (8).
Both TFs and miRNAs play critical roles in the regulation of mRNAs and participate in the pathogenesis of cancer. It is important to unravel the interaction of TFs, miRNAs, and gene within TF-gene-miRNA regulatory network, which would helpful to discover novel biomarkers and therapy targets of cancer (9,10).
In this study, three gene expression profiles [GSE43458 (11), GSE74706 (12), GSE32863 (13)] of LUAD were analyzed to obtain DEGs between LUAD tissues and normal tissues by GEOR2.The functions, pathways and PPI network analysis of DEGs were performed. Then, the target miRNAs and TFs of DEGs were predicted by bioinformatic methods. Finally, the TF-gene -miRNA co-expression network was established to investigate the interaction between genes, miRNAs and TFs in the pathogenesis of LUAD, and identify key genes, miRNAs and TFs in LUAD.
Methods
Microarray data collection and screen of DEGs
Three gene expression profiles of LUAD (GSE43458, GSE32863, GSE74706) were obtained from Gene Expression Omnibus (https://www.ncbi.nlm.nih.gov/geo/). The GSE43458 [(HuGene-1_0-st) Affymetrix Human Gene 1.0 ST Array (transcript (gene) version)] dataset included 30 normal lung tissues and 80 LUAD tissues. The GSE32863 (Illumina HumanWG-6 v3.0 expression bead chip) dataset included 58 LUAD tissues and 58 adjacent non-tumor lung tissues. The GSE74706 (Agilent-026652 Whole Human Genome Microarray 4x44K v2) dataset contain 18 normal lung tissues and 18 LUAD tissues. GEOR2 (https://www.ncbi.nlm.nih.gov/geo/geo2r/), an online tool of Gene Expression Omnibus, was used to screen DEGs from the gene expression data. DEGs were screened with the criteria of adjust P value <0.05 and |logFC| >1, and overlapped upregulated and downregulated DEGs were obtained respectively.
Functional annotation and pathway enrichment analysis
To explore the function of a large scale of genes, the Gene Ontology (GO) term enrichment analysis and Reactome pathway analysis of upregulated and downregulated DEGs were respectively conducted by using CluoGO APP of Cytoscape software platform (14). P<0.05 as the cut-off criterion of both GO and pathway analysis.
Construct PPI network of the DEGs
The PPI network, which can visualize the interaction and reveal the relationship between each proteins, was constructed by The Search Tool for the Retrieval of Interacting Genes/Proteins (STRING) (http://string-db.org) (15) with the threshold of minimum required interaction score >0.7. The PPI network was visualized and further analyzed using Cytoscape software platform (14). Two kinds of analysis methods were induced to investigate key nodes of the PPI network. One method was to identify hub modules of the network by using a plug-in of Cytoscape software platform, which called MCODE with criteria of maximum depth from Seed =100, k‐core =2, node score Cutoff =0.2 and degree >5. Another method was using cytoHubba, which could rank the nodes according to the properties of nodes, and top 20 nodes were selected.
TF-gene-miRNA co-expression network construction
Target miRNAs of DEGs were predicted by an online tool called miRWalk (http://zmf.umm.uni-heidelberg.de/apps/zmf/mirwalk/) (16) in two miRNA databases (TargetScan and miRDB) with the cutoff value binding score >0.95, the miRNAs identified in both two databases were considered as target miRNAs. The target TFs of DEGs were predicted by TRRUST (https://www.grnpedia.org/trrust/) (17), which is a database of human TF networks, with the criteria of P value <0.05. Then a TF-gene-miRNA co-expression network was constructed, visualized and further analyzed using Cytoscape. In order to explore the hub nodes in TF-gene-miRNA co-expression network, a plug-in of Cytoscape called cytoHubba was used, which can rank the nodes according to the properties of nodes. It provides 11 kinds topological analysis methods including MCC, DMNC, MNC, Degree, EPC and so on. The Degree topological analysis method was used because the degree of a node is directly related to its genetic importance; in other words, a node with a high degree tends to be a key node. According to the nodes’ degree value, top 10 genes, 10 miRNAs and 10 TFs was selected as hub nodes for further investigation.
Validations and Kaplan-Meier survival analysis of the hub nodes
We verified the differential expressions of these hub genes and TFs using GEPIA (http://gepia.cancer-pku.cn/index.html) (18), which is an interactive web that includes 9,736 tumors and 8,587 normal samples from TCGA and the GTEx projects. The criteria of DEGs was adjust P value <0.001 and |logFC| >1. We also verified the differential expressions of these miRNAs using starBase (http://starbase.sysu.edu.cn/index.php), which accompany with 10,546 miRNA-seq data of 32 types cancers. The cutoff criteria was adjust P value <0.05 and |logFC| >1. The relationship between the genes, miRNAs and TFs and the prognosis of LUAD was analyzed by Kaplan-Meier Plotter (http://kmplot.com/analysis/) (19), which contain 720 LUAD patients with detailed survival data. The threshold of significant association was log-rank P value <0.05. Furthermore, Human Protein Atlas (http://www.proteinatlas.org) was used to validate the protein level of hub genes and TFs in LUAD tissues and normal tissues.
Immunohistochemistry
In addition, we used paraffin samples from LUAD patients to verified the protein level of hub gene (FBXO32) by IHC (immunohistochemistry). The resected LUAD tissues were fixed with 10% neutral buffered formalin. The histological evaluation was performed on hematoxylin and eosin stained sections. The LUAD tissue sections were immunostained with primary antibodies against FBXO32 (Proteintech, China). After incubation with primary antibody, the detection of antibodies was accomplished using the streptavidin peroxidase method. Immunohistochemical scoring was based on the staining intensity and the percentage of positively stained cells. The staining intensity was scored as follows: 0, no staining; 1, weakly stained; 2, moderately stained; and 3, strongly stained. The data are presented as the mean ± standard deviation, and P<0.05 was considered significant.
The miRNA-gene co-expression in LUAD patients
The Pan-Cancer Analysis Platform of starBase (http://starbase.sysu.edu.cn/index.php) (20) is designed for decoding networks of noncoding RNAs, RNA-binding proteins and all protein-coding genes by analyzing the expression profiles across 32 cancer types from TCGA project, which provide exploration of co-expression networks of 2 candidate genes, including miRNA-RNA and RNA-RNA in 32 types of cancers. The co-expression between key miRNAs and genes was predicted by starBase.
Results
Identification of DEGs in LUAD
We identified 251, 493, 1,652 upregulated DEGs, and 646, 747, 2,439 downregulated DEGs in the GSE43458, GSE32863 and GSE74706 datasets respectively. A total of 75 upregulated DEGs (Figure 1A) and 262 downregulated DEGs (Figure 1B) overlapped across the three datasets (Tables S1,S2).
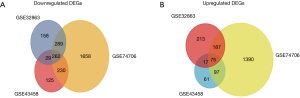
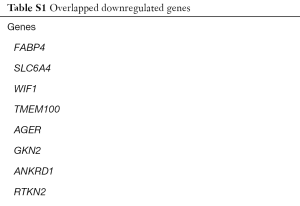
Full table
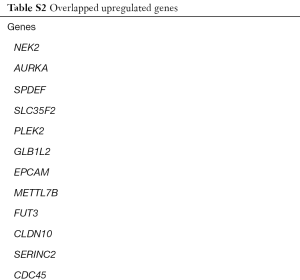
Full table
Functional annotation of overlapped DEGs
To further investigate the function of overlapped DEGs, the GO term and Reactome pathways enrichment analysis were performed. The most significantly enriched Reactome pathway of downregulated DEGs were extracellular matrix organization, semaphoring interactions, surfactant metabolism, complement cascade, regulation of complement cascade and biological oxidations (Figure 2A). The biological process of downregulated DEGs were significantly enriched in blood vessel development, angiogenesis and response to organic substance (Figure 2B). The most significantly enriched Reactome pathway of upregulated DEGs were cell cycle mitotic, collagen degradation and metabolism of amino acids and derivatives (Figure 2C); the biological process of upregulated DEGs were significantly enriched in movement of cell or subcellular component, response to chemical, circulator system development, cell adhesion (Figure 2D).
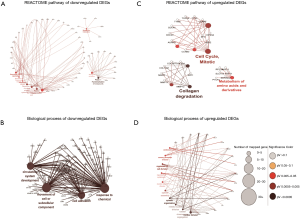
The PPI network and hub genes
262 downregulated overlapped DEGs and 75 upregulated overlapped DEGs were all filtered into the PPI network by using the STRING. A total of 337 nodes and 164 edges were obtained from the PPI network program. The average node degree was 2.14 and PPI enrichment P value was <1.0e-16, with the threshold of minimum required interaction score >0.7 (Figure 3A and Table S3). The PPI network was visualized and further analyzed using Cytoscape software platform. Two kinds of analysis methods were induced to investigate key nodes of the PPI network. The cytoHubba method identified top 20 nodes (Figure 3B and Table S4). The MCODE method identified 3 clusters of genes (Figure 3C and Figure S1). The 18 overlapped genes of these two methods were considered as hub nodes of the PPI network (Figure 3D).
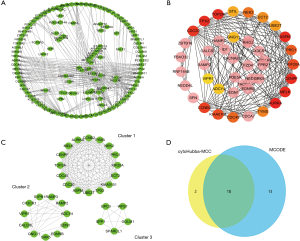
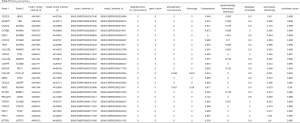
Full table
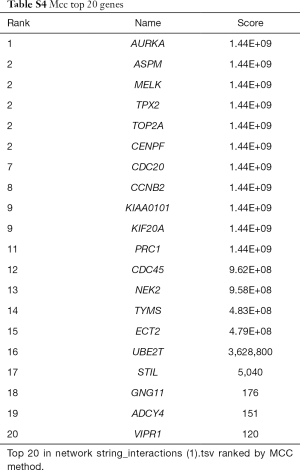
Full table
TF-gene-miRNA co-expression network construction
In two miRNA databases(TargetScan and miRDB), 851 overlapped target miRNAs of all 337 DEGs were obtained with the cutoff value Binding Score >0.95 (Table S5), and gene-miRNA co-expression network was constructed (Figure 4A). 29 TFs of all DEGs were obtained in TRRUST with the criteria of P value <0.05 (Table S6), and TF-gene co-expression network was constructed (Figure 4B). The TF-gene-miRNA co-expression network was constructed and the properties of each node in this co-expression network were calculated by cytoHubba (Figure 5 and Table S7). According to the nodes’ degree value, top 10 key genes (NEDD4L, LDLR, PHACTR2, MSRB3, GHR, PLSCR4, TSPAN18, EPB41L2, NPNT, FBXO32), 10 key miRNAs (hsa-miR-6838-5p, hsa-let-7e-5p, hsa-miR-17-5p, hsa-miR-216a-3p, hsa-miR-25-3p, hsa-let-7d-5p, hsa-miR-93-5p, hsa-miR-92b-3p, hsa-miR-92a-3p, hsa-miR-106a-5p) and 10 key TFs (SP1, ERG, PPARA, STAT6, E2F1, TP53, SIRT1, RELA, ETS1, JUN) was selected as hub nodes for further investigation.
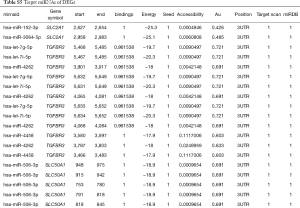
Full table
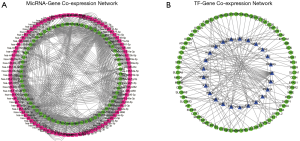
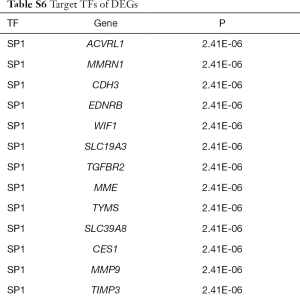
Full table
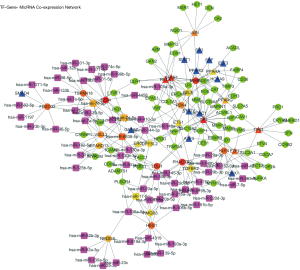
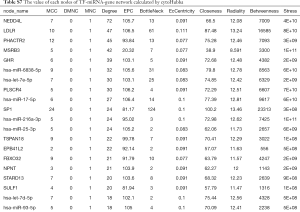
Full table
Validations and Kaplan-Meier survival analysis of these hub nodes
We verified the differential expressions of these hub genes and TFs in 483 LUAD patients and paired 374 normal individuals with the GEPIA datasets. A total of eight genes (LDLR, PHACTR2, MSRB3, GHR, PLSCR4, TSPAN18, EPB41L2 and NPNT) have significant lower expressions in LUAD patients, and FBXO32 had a significant higher expression in LUAD patients (Figure 6). In the Kaplan-Meier Plotter analysis, seven genes [PHACTR2 (P=4.7e-10), MSRB3 (P=6.2e-10), GHR (P=0.0018), PLSCR4 (P=8.4e-10), EPB41L2 (P=1.2e-5), FBXO32 (P=0.037) and NPNT (P=4.2e-7)] were identified association with poor prognosis of LUAD (Figure 7). Additionally, four TFs (ERG, STAT6, ETS1 and JUN) have significant lower expressions in LUAD patients, and E2F1 has a significant higher expression in LUAD patients (Figure 8). In the Kaplan-Meier Plotter analysis, four TFs [STAT6 (P=7.7e-9), ETS1 (P=2e-13), JUN (P=0.0026) and E2F1 (P=5e-9)] show significant association with prognosis of LUAD (Figure 9).
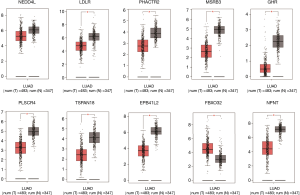
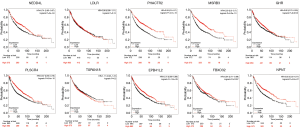
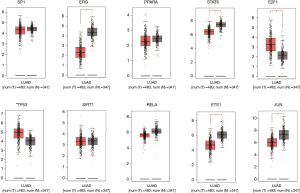
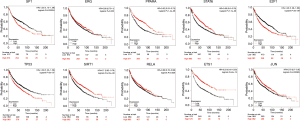
Next, we used starBase database to validate the differential expressions of key miRNAs in 521 LUAD patients and 20 paired normal individuals. Two miRNAs [hsa-let-7e-5p (P=4.2e-5) and hsa-let-7d-5p (P=0.00077)] have significant lower expressions in LUAD patients, and four miRNAs [hsa-miR-17-5p (P=2.4e-19), hsa-let-25-3p (P=0.047), hsa-miR-93-5p (P=2e-11) and hsa-miR-106a-5p (P=1.7e-18)] have significant higher expressions (Figure 10). In the Kaplan-Meier Plotter analysis, two miRNAs [hsa-let-7e (P=0.011) and hsa-let-17 (P=0.035)] show significant association with poor prognosis of LUAD (Figure 11).
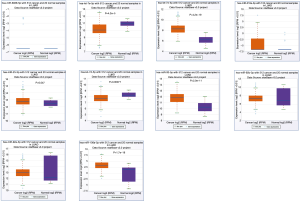
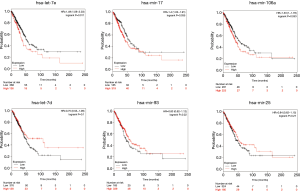
To further demonstrate that the hub genes and key TFs were related to LUAD, we used The Human Protein Atlas database(www.proteinatlas.org) to verify the protein expression of key genes and key TFs. Compared to normal lung tissues, the protein expressions of 6 hub genes (PHACTR2, MSRB3, GHR, PLSCR4, EPB41L2, NPNT) and 3 key TFs (STAT6, ETS1 and JUN) in LUAD tissues were significantly lower, and the protein expression of the key TF (E2F1) in LUAD tissues were significantly higher (Figures S2,S3). Immunohistochemistry staining obtained from The Human Protein Atlas database contain 6 key genes and 4 TFs, while the hub gene (FBXO32) was not included in the website. Therefore, we verified the protein expression level of FBXO32 in LUAD tissues by IHC. The result showed that FBXO32 protein expression level was significantly increased in LUAD tissues compared to that in normal lung tissues (Figure S4). These results showed that the protein levels of these key genes and TFs were consistent with mRNA levels.
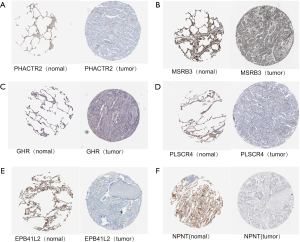
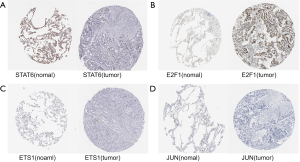
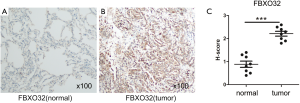
The miRNA-gene co-expression in LUAD patients
The co-expression between key miRNAs and genes in LUAD was predicted by starBase. The results shown that hsa-miR-17-5p and PLSCR4 have a significant negative co-expression (r=−0.33, P=1.67e-14) (Figure 12). Moreover, hsa-miR-17-5p have a binding site with PLSCR4 predicted by starBase (Figure S5).
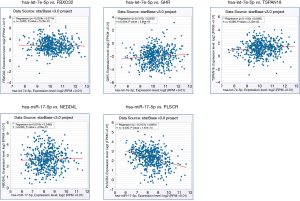
Discussion
LUAD is the major histological type of lung cancers. Although great improvement has been achieved in the treatments of LUAD, the prognosis of LUAD is still not optimistic. The progress of genomic medicine has improved the treatment outcomes and life quality of LUAD patients compared to traditional chemotherapy (21). In recent years, due to the advances in the knowledge of pathways and related genetic mutations, several drugs targeting the major pathways have been identified (22,23). The common pathways are NTRK/ROS1, EGFR, RAS-MAPK and PI3K/AKT/mTOR pathways (24,25). Many drugs targeting the genetic mutations of these pathways have been developed and shown great therapeutic values, such as EGFR inhibitors gefitinib and erlotinib, NTRK/ROS1 inhibitors entrectinib (25,26). Some of these drugs have become first line treatment and replaced chemotherapy (22). Despite the tremendous progress have made in the treatment of LUAD with target drugs, drug resistance remains a prominent and inevitable problem. Therefore, it is necessary to research novel therapy targets of LUAD. Gene chip and bioinformatic technology facilitates the exploration of new therapeutic targets in many diseases.
In present study, three gene express profile datasets of LUAD were obtained from GEO database, including 106 normal lung tissues and 156 LUADs tissues. A total of 75 upregulated overlapped DEGs and 262 downregulated overlapped DEGs were identified. We then performed function annotation, pathways enrichment and PPI network analysis on the DEGs. For the downregulated DEGs, pathway enrichment analysis revealed that the extracellular matrix organization was significantly enriched, which was essential on the development and progression of cancers (27,28). In progression of cancer, the extracellular matrix becomes aligned and providing “highways” for accelerate tumor cell invasion (27,29,30). For the upregulated DEGs, pathway enrichment analysis revealed that Cell cycle mitotic was significantly enriched. DNA replicated and generated two identical copies of each chromosome during the cell cycle, and then two chromosomes segregated evenly to the two daughter cells. Cells would lose genomic stability and become malignant if receive chromosomes not evenly (31,32). Factors regulating the cell cycle mitotic play an important role in the occurrence and progress of tumors (33).
Many studies have revealed that both TFs and miRNAs play critical roles in the regulation of mRNAs and participate in the pathogenesis of cancer. Therefore, TF-gene-miRNA regulate network construction is essential for exploring the molecular mechanism of LUAD. First, the targets TF and miRNA were predicted based on these DEGs. Then, a TF-gene-miRNA co-expression network of LUAD was constructed to identify hub genes, TFs and miRNAs by Cytoscape. In total, 10 hub genes, 10 key miRNAs and 10 key TFs were located in the central hub of the TF-gene-miRNA co-expression network. GEPIA was used to verify the differential expressions of these key nodes in the LUAD patients. We found that 9 genes, 5 TFs and 6 miRNAs were differential expressed in LUAD. In addition, survival analysis of these key genes, TFs and miRNAs were performed by using the Kaplan-Meier Plotter analysis website. As a result, a total of 7 key genes, 4 key TFs and 2 key miRNAs with significance were finally obtained.
Herein, we found seven key genes, including PHACTR2, MSRB3, GHR, PLSCR4, EPB41L2, NPNT and FBXO32, were significantly associated with the prognosis of LUAD. These genes may contribute to the progression of LUAD and could be regarded as biomarkers and therapeutic targets of LUAD. MSRB3 plays an important role in cancer cell apoptosis through ROS production and endoplasmic reticulum stress status (34,35). Morel et al. found that MSRB3 maintain the genome stability of breast cancer (36). Ma et al. also reported that high MSRB3 expression was related with poor prognosis in gastric cancer (37). FBXO32 (also known as atrogin-1) is a member of the F-box protein family and an upstream regulator of epithelial-mesenchymal transition (EMT), which promotes EMT during tumor metastasis (38). FBXO32, as a TGF-β/Smad target gene, had shown biological activities in cell survival, and involved in several kinds of tumors including esophageal squamous cell carcinoma (39), pancreatic cancer (40) and colorectal cancer (41). Nephronectin (NPNT), an extracellular matrix protein, plays a major role in epithelial-mesenchymal interactions and signal transduction. Wang D et al. found that NPNT was a critical regulator of breast cancer metastases, which can promoted bone metastases in the early-stage of breast cancer by regulating the osteogenic niche (42) PHACTR2 (phosphatase and actin regulator 2) was found to be a novel genetic biomarker of cellular DNA repair in lung cancer by a GWAS study (43). PLSCR4 (phospholipid scramblase 4) is a member of transmembrane proteins family localized at the plasma membrane and often stimulated by proinflammatory cytokines. The diverse roles of PLSCRs in multiple cellular processes including immuno-activation, tumorigenesis, cell apoptosis and proliferation have been revealed by recent studies (44,45). Pienkowska et al. reported that PLSCR4 was identified as potential biomarkers for choroid plexus tumor aggressiveness in a DNA methylation profiles study (46). However, to the best of our knowledge, the function of PLSCR4 had not been well studied so far. Our study was the first time to report PLSCR4 as a key gene was associated with LUAD and may be a potential biomarker or therapy target of LUAD.
In addition, four key TFs (TAT6, E2F1, ETS1 and JUN) and two key miRNAs (hsa-let-7e-5p and hsa-miR-17-5p) were also significantly associated with the prognosis of LUAD. According to recent studies, all these 4 TFs have been verified as key regulators and potential therapy targets of numerous cancers including LUAD (47,48). Moreover, Chen et al. found that hsa-let-7e-5p could stimulate colorectal cancer cell migration and was a potential prognosis marker for rectal carcinoma with liver metastases (49). Hsa-let-7e-5p was over-expressed in head and neck squamous cell carcinoma cells (HNSCC), which would inhibit the proliferation and metastasis of HNSCC by inhibiting the expression of chemokine receptor 7 (50). Hsa-miR-17-5p was identified as a dominating oncogenic miRNA by downregulating tumor suppressors in a large pan-cancer research, including 15 epithelial cancer types and 7316 clinical samples (51). Hsa-miR-17-5p was also identified have a significant association with brain metastases breast cancer by bioinformatic analysis of GEO database (52). Shukla et al. reported that hsa-miR-17-5p was upregulated in serum and tissue of 115 cervical cancer patients and may become a potential biomarker as a biosignature of clinical relevance (53). Zhang et al. reported that circulating exosomal miR-17-5p was significantly upregulated in NSCLC patients and was a promising novel clinical diagnostic marker in the diagnosis of NSCLC (54).
In order to explore potential pathways between miRNA-gene-TF, we analyzed the co-expression relationship of miRNA-gene and TF-gene. The result revealed that hsa-miR-17-5p and PLSCR4 had a significant negative co-expression relationship in LUAD by miRNA/gene co-expression analysis. Further, we predicted that hsa-miR-17-5p have a binding site with PLSCR4 by using starBase website tool, which revealed that hsa-miR-17-5p may bind to PLSCR4 and inhibit the expression of PLSCR4. In the present study, PLSCR4 was identified as a downregulated DEG in LUAD. Recent study also suggested that the level of miR-17-5p was significantly higher in LUAD compared with healthy control subjects (54). Taken together, these findings suggested that hsa-miR-17-5p and its target gene PLSCR4 may play an important role in LUAD. The relationship between hsa-miR-17-5p and PLSCR4 has not been reported so far. Our study was the first time to report the co-expression relationship between hsa-miR-17-5p and PLSCR4. But the result was based on bioinformatics, and biological experiment in vivo and vitro would perform to further validate the result.
Conclusions
In our study, we finally identified seven key genes (PHACTR2, MSRB3, GHR, PLSCR4, EPB41L2, NPNT, FBXO32), two key miRNAs (hsa-let-7e-5p, hsa-miR-17-5p) and four key TFs (STAT6, E2F1, ETS1, JUN) as key factors of LUAD by integrating the data of GEO and TCGA database and constructing the TF-gene-miRNA co-expression network. Furthermore, the present study also found that hsa-miR-17-5p and its target gene PLSCR4 may play an important role in LUAD. The findings may provide further understanding of the pathogenesis of LUAD and provide novel insights for the diagnosis and treatment of LUAD. However, there are still the limitations in this study. Our results were based on public database and bioinformatics analysis, a clinical study of large samples and biological experiment would perform in the future to further validate the results.
Acknowledgments
Funding: This work was supported by grants from the National Natural Science Foundation of China (81573234 and 81773445) and “333”Project Of Jiangsu Province (LGY2016006).
Footnote
Conflicts of Interest: All authors have completed the ICMJE uniform disclosure form (available at http://dx.doi.org/10.21037/jtd-19-4168). The authors have no conflicts of interest to declare.
Ethical Statement: The authors are accountable for all aspects of the work in ensuring that questions related to the accuracy or integrity of any part of the work are appropriately investigated and resolved.
Open Access Statement: This is an Open Access article distributed in accordance with the Creative Commons Attribution-NonCommercial-NoDerivs 4.0 International License (CC BY-NC-ND 4.0), which permits the non-commercial replication and distribution of the article with the strict proviso that no changes or edits are made and the original work is properly cited (including links to both the formal publication through the relevant DOI and the license). See: https://creativecommons.org/licenses/by-nc-nd/4.0/.
References
- Torre LA, Bray F, Siegel RL, et al. Global cancer statistics, 2012. CA Cancer J Clin 2015;65:87-108. [Crossref] [PubMed]
- DeSantis CE, Siegel RL, Sauer AG, et al. Cancer statistics for African Americans, 2016: Progress and opportunities in reducing racial disparities. CA Cancer J Clin 2016;66:290-308. [Crossref] [PubMed]
- Rudin CM, Poirier JT, Byers LA, et al. Molecular subtypes of small cell lung cancer: a synthesis of human and mouse model data. Nat Rev Cancer 2019;19:289-97. [Crossref] [PubMed]
- Moses MA, George AL, Sakakibara N, et al. Molecular Mechanisms of p63-Mediated Squamous Cancer Pathogenesis. Int J Mol Sci 2019;20:3590. [Crossref] [PubMed]
- Li Y, Chen Y, Li J, et al. Co-delivery of microRNA-21 antisense oligonucleotides and gemcitabine using nanomedicine for pancreatic cancer therapy. Cancer Sci 2017;108:1493-503. [Crossref] [PubMed]
- Cheng CJ, Bahal R, Babar IA, et al. MicroRNA silencing for cancer therapy targeted to the tumour microenvironment. Nature 2015;518:107-10. [Crossref] [PubMed]
- Rupaimoole R, Slack FJ. MicroRNA therapeutics: towards a new era for the management of cancer and other diseases. Nat Rev Drug Discov 2017;16:203-22. [Crossref] [PubMed]
- Bhayadia R, Krowiorz K, Haetscher N, et al. Endogenous Tumor Suppressor microRNA-193b: Therapeutic and Prognostic Value in Acute Myeloid Leukemia. J Clin Oncol 2018;36:1007-16. [Crossref] [PubMed]
- Zeng JH, Xiong DD, Pang YY, et al. Identification of molecular targets for esophageal carcinoma diagnosis using miRNA-seq and RNA-seq data from The Cancer Genome Atlas: a study of 187 cases. Oncotarget 2017;8:35681-99. [Crossref] [PubMed]
- Chen B, Gao S, Ji C, et al. Integrated analysis reveals candidate genes and transcription factors in lung adenocarcinoma. Mol Med Rep 2017;16:8371-9. [Crossref] [PubMed]
- Kabbout M, Garcia MM, Fujimoto J, et al. ETS2 mediated tumor suppressive function and MET oncogene inhibition in human non-small cell lung cancer. Clin Cancer Res 2013;19:3383-95. [Crossref] [PubMed]
- Marwitz S, Depner S, Dvornikov D, et al. Downregulation of the TGFbeta Pseudoreceptor BAMBI in Non-Small Cell Lung Cancer Enhances TGFbeta Signaling and Invasion. Cancer Res 2016;76:3785-801. [Crossref] [PubMed]
- Selamat SA, Chung BS, Girard L, et al. Genome-scale analysis of DNA methylation in lung adenocarcinoma and integration with mRNA expression. Genome Res 2012;22:1197-211. [Crossref] [PubMed]
- Smoot ME, Ono K, Ruscheinski J, et al. Cytoscape 2.8: new features for data integration and network visualization. Bioinformatics 2011;27:431-2. [Crossref] [PubMed]
- Szklarczyk D, Franceschini A, Wyder S, et al. STRING v10: protein–protein interaction networks, integrated over the tree of life. Nucleic Acids Res 2015;43:D447. [Crossref] [PubMed]
- Dweep H, Gretz N. miRWalk2.0: a comprehensive atlas of microRNA-target interactions. Nat Methods 2015;12:697. [Crossref] [PubMed]
- Han H, Cho JW, Lee S, et al. TRRUST v2: an expanded reference database of human and mouse transcriptional regulatory interactions. Nucleic Acids Res 2018;46:D380-D386. [Crossref] [PubMed]
- Tang Z, Li C, Kang B, et al. GEPIA: a web server for cancer and normal gene expression profiling and interactive analyses. Nucleic Acids Res 2017;45:W98-W102. [Crossref] [PubMed]
- Győrffy B, Surowiak P, Budczies J, et al. Online Survival Analysis Software to Assess the Prognostic Value of Biomarkers Using Transcriptomic Data in Non-Small-Cell Lung Cancer. Plos One 2013;8:e82241. [Crossref] [PubMed]
- Li JH, Liu S, Zhou H, et al. starBase v2.0: decoding miRNA-ceRNA, miRNA-ncRNA and protein-RNA interaction networks from large-scale CLIP-Seq data. Nucleic Acids Res 2014;42:D92-7. [Crossref] [PubMed]
- Politi K, Herbst RS. Lung cancer in the era of precision medicine. Clin Cancer Res 2015;21:2213-20. [Crossref] [PubMed]
- Herbst RS, Morgensztern D, Boshoff C. The biology and management of non-small cell lung cancer. Nature 2018;553:446-54. [Crossref] [PubMed]
- Blons H, Garinet S, Laurent-Puig P, et al. Molecular markers and prediction of response to immunotherapy in non-small cell lung cancer, an update. J Thorac Dis 2019;11:S25-S36. [Crossref] [PubMed]
- Brognard J, Clark AS, Ni Y, et al. Akt/protein kinase B is constitutively active in non-small cell lung cancer cells and promotes cellular survival and resistance to chemotherapy and radiation. Cancer Res 2001;61:3986-97. [PubMed]
- Song Z, Yu X, Zhang Y. Mutation and prognostic analyses of PIK3CA in patients with completely resected lung adenocarcinoma. Cancer Med 2016;5:2694-700. [Crossref] [PubMed]
- Singh SS, Dahal A, Shrestha L, et al. Genotype Driven Therapy for Non-Small Cell Lung Cancer: Resistance, Pan Inhibitors and Immunotherapy. Curr Med Chem 2019. [Epub ahead of print]. [Crossref] [PubMed]
- Pickup MW, Mouw JK, Weaver VM. The extracellular matrix modulates the hallmarks of cancer. EMBO reports 2014;15:1243-53. [Crossref] [PubMed]
- Lee S, Han H, Koo H, et al. Extracellular matrix remodeling in vivo for enhancing tumor-targeting efficiency of nanoparticle drug carriers using the pulsed high intensity focused ultrasound. J Control Release 2017;263:68-78. [Crossref] [PubMed]
- Jones CE, Hammer AM, Cho Y, et al. Stromal PTEN Regulates Extracellular Matrix Organization in the Mammary Gland. Neoplasia 2019;21:132-45. [Crossref] [PubMed]
- Stevens LE, Cheung WKC, Adua SJ, et al. Extracellular Matrix Receptor Expression in Subtypes of Lung Adenocarcinoma Potentiates Outgrowth of Micrometastases. Cancer Res 2017;77:1905-17. [Crossref] [PubMed]
- Schvartzman JM, Sotillo R, Benezra R. Mitotic chromosomal instability and cancer: mouse modelling of the human disease. Nat Rev Cancer 2010;10:102-15. [Crossref] [PubMed]
- Solomon DA, Kim T, Diaz-Martinez LA, et al. Mutational inactivation of STAG2 causes aneuploidy in human cancer. Science 2011;333:1039-43. [Crossref] [PubMed]
- Thole TM, Lodrini M, Fabian J, et al. Neuroblastoma cells depend on HDAC11 for mitotic cell cycle progression and survival. Cell Death Dis 2017;8:e2635. [Crossref] [PubMed]
- Kwak GH, Kim HY. MsrB3 deficiency induces cancer cell apoptosis through p53-independent and ER stress-dependent pathways. Arch Biochem Biophys 2017;621:1-5. [Crossref] [PubMed]
- Kwak GH, Kim TH, Kim HY. Down-regulation of MsrB3 induces cancer cell apoptosis through reactive oxygen species production and intrinsic mitochondrial pathway activation. Biochem Biophys Res Commun 2017;483:468-74. [Crossref] [PubMed]
- Morel AP, Ginestier C, Pommier RM, et al. A stemness-related ZEB1-MSRB3 axis governs cellular pliancy and breast cancer genome stability. Nat Med 2017;23:568-78. [Crossref] [PubMed]
- Ma X, Wang J, Zhao M, et al. Increased expression of methionine sulfoxide reductases B3 is associated with poor prognosis in gastric cancer. Oncol Lett 2019;18:465-71. [PubMed]
- Sahu SK, Tiwari N, Pataskar A, et al. FBXO32 promotes microenvironment underlying epithelial-mesenchymal transition via CtBP1 during tumour metastasis and brain development. Nat Commun 2017;8:1523. [Crossref] [PubMed]
- Guo W, Zhang M, Shen S, et al. Aberrant methylation and decreased expression of the TGF-β/Smad target gene FBXO32 in esophageal squamous cell carcinoma. Cancer 2014;120:2412-23. [Crossref] [PubMed]
- Yang C, Fan P, Zhu S, et al. 3F-Box protein 32 degrades ataxia telangiectasia and Rad3-related and regulates DNA damage response induced by gemcitabine in pancreatic cancer. Oncol Lett 2018;15:8878-84. [PubMed]
- Yuan X, Zhang Z, Jiang K, et al. Preliminary Study of the Role F-Box Protein 32 (FBXO32) in Colorectal Neoplasms Through the Transforming Growth Factor beta (TGF-β)/Smad4 Signalling Pathway. Med Sci Monit 2018;24:1080-8. [Crossref] [PubMed]
- Wang D, Zhao C, Gao L, et al. NPNT promotes early-stage bone metastases in breast cancer by regulation of the osteogenic niche. J Bone Oncol 2018;13:91-6. [Crossref] [PubMed]
- Wang LE, Gorlova OY, Ying J, et al. Genome-wide association study reveals novel genetic determinants of DNA repair capacity in lung cancer. Cancer Res 2013;73:256-64. [Crossref] [PubMed]
- Kodigepalli KM, Bowers K, Sharp A, et al. Roles and regulation of phospholipid scramblases. FEBS Lett 2015;589:3-14. [Crossref] [PubMed]
- Mutch DM, O'Maille G, Wikoff WR, et al. Mobilization of pro-inflammatory lipids in obese Plscr3-deficient mice. Genome Biol 2007;8:R38. [Crossref] [PubMed]
- Pienkowska M, Choufani S, Turinsky AL, et al. DNA methylation signature is prognostic of choroid plexus tumor aggressiveness. Clin Epigenetics 2019;11:117. [Crossref] [PubMed]
- Shanmugam MK, Lee JH, Chai EZ, et al. Cancer prevention and therapy through the modulation of transcription factors by bioactive natural compounds. Semin Cancer Biol 2016;40-41:35-47. [Crossref] [PubMed]
- Rajagopal C, Lankadasari MB, Aranjani JM, et al. Targeting oncogenic transcription factors by polyphenols: A novel approach for cancer therapy. Pharmacol Res 2018;130:273-91. [Crossref] [PubMed]
- Chen W, Lin G, Yao Y, et al. MicroRNA hsa-let-7e-5p as a potential prognosis marker for rectal carcinoma with liver metastases. Oncol Lett 2018;15:6913-24. [PubMed]
- Wang S, Jin S, Liu MD, et al. Hsa-let-7e-5p Inhibits the Proliferation and Metastasis of Head and Neck Squamous Cell Carcinoma Cells by Targeting Chemokine Receptor 7. J Cancer 2019;10:1941-8. [Crossref] [PubMed]
- Dhawan A, Scott JG, Harris AL, et al. Pan-cancer characterisation of microRNA across cancer hallmarks reveals microRNA-mediated downregulation of tumour suppressors. Nat Commun 2018;9:5228. [Crossref] [PubMed]
- Li Z, Peng Z, Gu S, et al. Global Analysis of miRNA-mRNA Interaction Network in Breast Cancer with Brain Metastasis. Anticancer Res 2017;37:4455-68. [PubMed]
- Shukla V, Varghese VK, Kabekkodu SP, et al. Enumeration of deregulated miRNAs in liquid and tissue biopsies of cervical cancer. Gynecol Oncol 2019;155:135-43. [Crossref] [PubMed]
- Zhang Y, Zhang Y, Yin Y, et al. Detection of circulating exosomal miR-17-5p serves as a novel non-invasive diagnostic marker for non-small cell lung cancer patients. Pathol Res Pract 2019;215:152466. [Crossref] [PubMed]