Sputum and serum autoantibody profiles and their clinical correlation patterns in COPD patients with and without eosinophilic airway inflammation
Introduction
Chronic obstructive pulmonary disease (COPD) is a major cause of morbidity and mortality worldwide, and is characterized by persistent respiratory symptoms and airflow limitation that is not fully reversible due to airway and/or alveolar lesions (1). Heterogenous and complex immunological responses and airway inflammation play critical roles in the pathogenesis and progression of COPD (2). There are various patterns of airway inflammation in COPD. Although it is generally accepted that neutrophilic inflammation is the major inflammation pattern in the airways during COPD, the concept of eosinophilic airway inflammation arose in the 1990s (3,4). Importantly, during stable COPD, sputum eosinophilia may predict the response to inhaled corticosteroids (ICS) used to prevent exacerbation of the disease (5-8).
The role of autoimmunity in chronic airway diseases such as asthma and COPD has obtained attention in recent years (9-12). Airway inflammation in COPD is heterogeneous in nature and pattern. Similar to severe asthma, glucocorticoid treatment of stable COPD is not as effective at reducing airway inflammation (13). However, ICS may reduce adaptive immune responses in stable COPD and may be more effective in patients with increased B-cell responses as indicated by high autoantibody titres (14,15), while long-term withdrawal of ICS in stable COPD cases leads to a significant increase in the number of CD3+, CD4+ and CD8+ T cells in the airways (15). Furthermore, eosinophilic inflammation in COPD reflects the more general presence of type 2 inflammation, which is mediated by both adaptive immune cells and innate immune cells in the lung/airway (16). Eosinophilic inflammation can lead to the release and accumulation of self-antigens, such as collagen, elastin, cytochrome C, and danger-associated molecular (DAMPs), and may consequently foster a microenvironment of breaking immune tolerance (17-20). Therefore, it is reasonable to infer that there would be more autoantibodies involved in eosinophilic COPD than in non-eosinophilic COPD, despite the lack of reports about which autoantibodies are involved in COPD patients with different inflammation patterns.
Antibodies against a set of self-antigens were reported in COPD serum, plasma and tissue, and their associations with clinical parameters were also evaluated. Packard et al. reported increased levels of serum autoantibodies against collagen I, collagen II, collagen IV, elastin, aggrecan and cytochrome C (21). The increased levels of serum anti-collagen II, anti-cytochrome C and anti-aggrecan were associated with emphysema phenotype of COPD. A decreased level of anti-elastin antibody was reported to be associated with emphysema and more severe disease (22). Recently, Mukherjee et al. identified an autoimmune endotype of severe eosinophilic asthma by the presence of sputum autoantibodies against eosinophil peroxidase and autologous cellular components, and also demonstrated that airway autoantibodies were associated with clinical markers of airway eosinophilic degranulation (11), indicating a potential association between autoimmunity and airway eosinophilic inflammation in COPD. However, the airway/circulating autoantibody responses and their clinical correlation patterns in COPD patients with and without airway eosinophilic inflammation are unknown.
Network medicine is an integrative research approach that is suitable for the investigation of complex diseases, such as chronic respiratory diseases (23-26), and it is the human counterpart of the system biology (27). Node is a system component that, by connecting/interacting with other nodes/components, forms a network. An edge (link) represents the interactions between the nodes of a network. In biological networks, nodes can denote genes, RNA molecules, proteins, metabolites or even diseases, and edges can be protein–protein binding interactions, metabolic couplings or correlation coefficients between parameters, among others (23). The degree of a node is the sum of the edges (links) that connect to it. Hubs are the highly connected nodes in the network. A hub node in a network has a high degree of edges, meaning that it interacts with many other nodes in the network, and thus often occupies a central position (28). Grosdidier and colleagues used the network-based approach to investigate the biological relationships between COPD, comorbidities and chemical products contained in tobacco smoke, and found that COPD shared biological pathways, proteins and genes with its comorbidities (29). Divo and colleagues investigated the association between comorbidities in stable COPD patients by integrating 79 comorbidities as well as demographic, clinical and functional parameters in the network analysis (30). They found that the comorbidities were significantly interlinked and formed a scale-free network in which six modules could be identified. Faner and colleagues explored the association between multiple comorbidities in exacerbated COPD patients from a molecular perspective using network analysis (31). However, no known study to date has investigated the interrelationships among airway and circulating autoantibody responses and clinical parameters in different airway inflammatory phenotypes of COPD patients. Hence, we hypothesized that COPD patients of different airway inflammatory phenotypes have distinct autoantibody expression and correlation patterns both in the airways and circulatory system. In the current study, we detected airway and circulating autoantibody profiles in eosinophilic COPD compared with non-eosinophilic COPD and healthy controls, and performed a network-based approach to analyze the integrative data (autoantibody and clinical profiles) in each group. Based on our exploratory purpose and information from published literature (21,22,32-38), we selected the following 13 autoantigens with known or putative links to COPD: (I) extracellular matrix (ECM) proteins, i.e., collagen I, collagen II, collagen IV, aggrecan, and elastin; (II) stress-related molecules, i.e., amyloid-beta (Aβ), heat shock protein (HSP) 47, and HSP70; (III) an alveolar cellular protein, i.e., cytokeratin 18; and (IV) common intracellular antigens, i.e., centromere protein B (CENP-B), cytochrome C, La/Sjögren syndrome type B antigen (La), and vimentin. We aimed to (I) describe the autoantibody profiles in different inflammatory phenotypes of COPD; (II) compare the correlation patterns of the autoantibody and clinical parameters of COPD subgroups with and without eosinophilic airway inflammation; and (III) identify the most important factors (hubs) in each network. Our findings provide new insight into the autoimmune responses involved in the different inflammatory phenotypes of COPD and the need for personalized clinical management of COPD.
Methods
Design and patients
This is a prospective cross-sectional observational study. Sixty-two COPD patients in the stable stage of the disease were enrolled at Guangzhou Institute of Respiratory Health, the First Affiliated Hospital of Guangzhou Medical University (Guangzhou, China), between August 2017 and January 2018. The inclusion criteria were: (I) over 40 years of age; and (II) confirmed diagnosis of COPD according to the Global Initiative for Chronic Obstructive Lung Disease (GOLD) guidelines (post-bronchodilator FEV1/FVC ratio <0.7). The exclusion criteria were: (I) diagnosis of known respiratory disorders other than COPD; (II) history of significant inflammatory disease other than COPD; (III) COPD exacerbation within 4 weeks of enrolment; (IV) history of lung surgery; (V) recent diagnosis of cancer; (VI) received a blood transfusion within 4 weeks of enrolment; (VII) inability to walk; and (VIII) participating in a blinded drug trial. Informed consent was obtained from all patients. A group of 14 non-COPD controls without known medical conditions was also recruited for comparison. Written informed consent was obtained from all subjects. This study was conducted in accordance with the Declaration of Helsinki and approved by the ethics committee of the First Affiliated Hospital of Guangzhou Medical University (approval No.: 2017-22). The current study was registered with www.clinicaltrials.gov (NCT 03240315).
Clinical and functional parameters
Data collected at enrolment included patient demographic characteristics, pulmonary functions, COPD assessment test (CAT) and the modified Medical Research Council dyspnoea scale (mMRC) of subjects before the induction of sputum. Venous blood samples were collected from all subjects and separated at the same visit. Spirometry was performed before sputum induction according to the American Thoracic Society (ATS) guidelines (39).
Blood sample collection and processing
Peripheral venous blood samples were collected in ethylene diamine tetra-acetic acid (EDTA) anticoagulation tubes. The differential white blood cell count was determined using a Coulter instrument (Sysmex-XE2100, Kobe, Japan). Serum samples were stored at −80 °C for subsequent detection.
Sputum collection and processing
Sputum induction was performed according to the guidelines suggested by the Task Force of the European Respiratory Society (40). We used a two-step procedure to process the sputum as previously reported (41,42). Selected and weighed sputum specimens were placed in a centrifuge tube and incubated with an 8× volume of phosphate-buffered saline (PBS), placed on ice on a bench rocker for 15 min, then rocked for 15 s every 5 min during an ice bath. The mixture was centrifuged at 1,000 ×g for 10 min using a low-temperature centrifuge at 4 °C. The suspension was filtered through a 0.22-µm bacterial filter to remove bacteria, then a 4× volume of the supernatant was aspirated and stored in frozen storage tubes at −80 °C for subsequent detection. Then, a 2× volume of 0.3% dithiothreitol (DTT) was added to the remaining sputum solute for cell dispersion. Slides were prepared for differential cell counts staining with hematoxylin-eosin. The percentages of neutrophils, eosinophils, macrophages and lymphocytes were quantified respectively (43).
Autoantibody detection
As mentioned above, we selected 13 autoantigens, which included: Collagen I, Collagen II, Collagen IV, Aggrecan, elastin, Aβ, HSP47, HSP70, Cytokeratin 18, CENP-B, Cytochrome C, La and Vimentin. Both IgG and IgM antibody levels against the above autoantigens in the sputum supernatant and serum samples were detected in triplicate by using a customized protein microarray (Human Autoantigen Protein Array G1; RayBiotech, Inc. Norcross, GA, USA). Given that the detection of autoantibodies in sputum is not a routine test, we validated the reproducibility of the autoantibody measurements in the sputum supernatant of healthy and stable COPD subjects in our recent publication (42). Briefly, the detection procedure included the following steps: (I) equilibrating the kit to room temperature; (II) air-drying the glass slide; (III) array blocking; (IV) sample incubation; (V) array washing; (VI) incubation with biotin-conjugated anti-human IgG/IgM; (VII) array washing; (VIII) incubation with Cy3-conjugated streptavidin; (IX) array washing and drying; (X) fluorescence detection (laser scanning) and data extraction; (XI) data analysis. The detailed protocol can be downloaded (https://www.raybiotech.com/files/manual/Protein-Array/PAH-AGN-G1.pdf).
Statistical analysis
Data are expressed as the mean ± SD for continuous variables. Variables with a skewed distribution are expressed as the median (interquartile range). COPD airway inflammatory phenotypes were classified using sputum white cell counts as follows: eosinophilic COPD was defined as ≥3% sputum eosinophils, and non-eosinophilic COPD was defined as <3% sputum eosinophils (5-8). For categorical variables, the number of observations and percentages were given for each category. Shapiro-Wilk test was performed to access the normality of distribution of each continuous variable, and depending on the distribution of the data, ANOVA or Kruskal-Wallis test were used to compare variables among groups. Then, Fisher’s Least Significant Difference (LSD) test or the Nemenyi test was performed to analyse the differences between groups. All statistical analyses were performed using the SPSS software (version 19.0). Spearman correlation networks integrating clinical and molecular parameters were then constructed using Gephi software (version 0.9.1). Network topological analysis was performed using the corresponding functions contained in Gephi.
Results
The demographics and clinical characteristics of all subjects are shown in Table 1. The COPD groups had lower lung function than the healthy control group. There were no significant differences in body mass index (BMI), lung function, CAT score or mMRC between the two COPD groups.
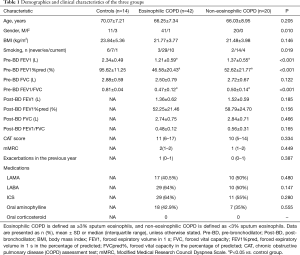
Full table
Autoantibody levels in the three groups
When comparing autoantibody levels, just a few autoantibodies were significantly different among groups. Significantly higher levels of the sputum autoantibodies, anti-CytochromeC_IgG and anti-Aggrecan_IgM, were detected in the non-eosinophilic COPD cases than in the controls, and significantly lower levels of the anti-CollagenII_IgG and anti-CollagenIV_IgG antibodies were detected in the non-eosinophilic COPD cases than in the controls. Anti-CollagenII_IgG and anti-HSP47_IgG levels were also significantly lower in the eosinophilic COPD cases than in the controls, and anti-Aggrecan_IgM levels were significantly higher in eosinophilic COPD cases than in the controls (Table 2 and Figure 1A,B,C,D,E).
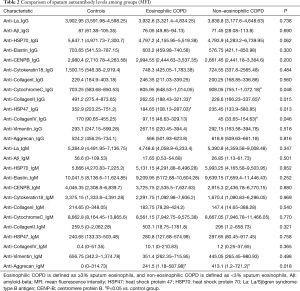
Full table
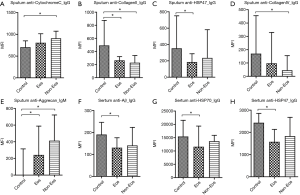
Regarding the levels of autoantibodies in serum, anti- Aβ_IgG, anti-HSP70_IgG and anti-HSP47_IgG levels were significantly lower in eosinophilic COPD cases than in the controls (Table 3 and Figure 1F,G,H). Interestingly, there was no significant difference between eosinophilic COPD and non-eosinophilic COPD in terms of IgG and IgM autoantibody levels (Table 3 and Figure 1).
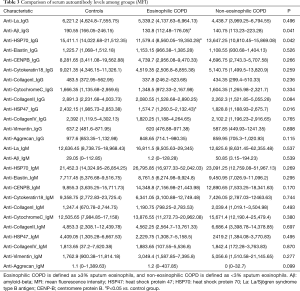
Full table
Correlation patterns of clinical and autoantibody profiles in the three groups
Figure 2 shows the Spearman correlation networks integrating autoantibody profiles and clinical parameters in the three groups, and Table 4 shows their comparisons with respect to the topological properties of the networks.
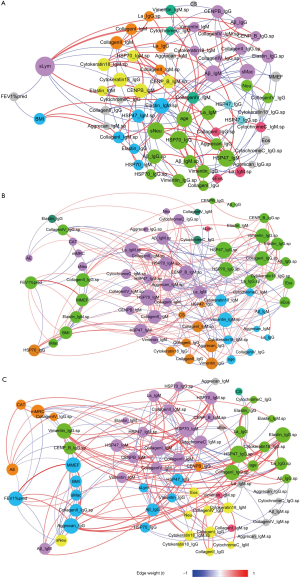
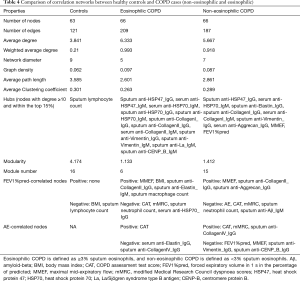
Full table
In the normal controls, the forced expiratory volume in 1 s in the percentage of predicted (FEV1%pred) only negatively correlated with BMI and the sputum lymphocyte count. (Figure 2A and Table 4) In eosinophilic COPD cases, FEV1%pred positively correlated with the maximal mid-expiratory flow (MMEF), BMI, sputum macrophage count, sputum anti-CollagenII_IgG and sputum anti-Elastin_IgM, and negatively correlated with the CAT score, mMRC, sputum neutrophil count and serum anti-HSP70_IgG. Prior exacerbations positively correlated only with the CAT score, and negatively correlated with sputum anti-CollagenIV_IgG and serum anti-Elastin_IgG (Figure 2B). Interestingly, there was no significant correlation between prior exacerbation and lung function parameters (FEV1%pred and MMEF) (Figure 2B and Table 4).
In non-eosinophilic COPD, FEV1%pred positively correlated with MMEF, BMI, sputum macrophage count, sputum anti-CollagenII_IgG, serum anti- Aggrecan_IgG, and negatively correlated with prior exacerbation, CAT score, mMRC, sputum neutrophil count and serum anti- Aβ_IgM. Prior exacerbation positively correlated with CAT score, mMRC and sputum anti-CollagenIV_IgG, and negatively correlated with FEV1%pred, MMEF, sputum anti-Vimentin_IgG and sputum anti- CENP-B_IgG (Figure 2C and Table 4).
The distinct correlation patterns described above resulted in distinct topological properties in three networks, as shown in Table 4. Notable observations were that both COPD groups had higher network densities and more hubs than the controls, and sputum anti-HSP47_IgG, serum anti-HSP70_IgM, sputum anti-CollagenI_IgG and sputum anti-Vimentin_IgG were hubs in both of the COPD groups. Lung function parameters (FEV1%pred and MMEF) were hubs in non-eosinophilic COPD but not in eosinophilic COPD (Table 4).
Discussion
To the best of our knowledge, this is the first study to demonstrate (I) the detection of autoantibodies against ECM proteins, DAMPs and many other autoantigens in serum and sputum simultaneously using a high-throughput method; (II) analysis of the correlation patterns among autoantibodies and clinical parameters in each inflammatory phenotype of COPD, as well as a comparison of the network topological features. Our main findings were as follows: (I) Although the clinical features were similar between the two COPD groups, the correlation patterns between the clinical parameters and autoantibody levels were markedly different. (II) In non-eosinophilic COPD, prior exacerbation was negatively associated with lung function (FEV1%pred and MMEF) and positively associated with CAT score, mMRC and sputum anti-CollagenIV IgG, but in eosinophilic COPD, no significant correlation between prior exacerbation and lung function was detected. (III) Topological analysis identified groups of highly connected nodes/parameters (hubs): the network of eosinophilic COPD had 12 hubs all comprising autoantibodies, while the network of non-eosinophilic COPD comprised two lung function parameters and only seven autoantibodies.
Previous studies reported the relationship between COPD and many autoantibodies and assessed the correlation between autoantibodies and clinical parameters, but few studies have addressed the correlation patterns between autoantibodies and clinical parameters in different airway inflammatory phenotypes of COPD. Lee and colleagues reported that anti-elastin antibodies, but not anti-collagen antibodies, were higher in patients with emphysema than in healthy controls (38), although subsequent studies failed to show that the levels of both anti-elastin and anti-collagen antibodies were higher in COPD patients than in healthy controls (32,44). A lower antibody titre of anti-elastin antibodies was reported to be associated with emphysema and more severe disease (22). Newkirk and co-workers reported that 85% of COPD cases were positive for IgM anti-HSP70 autoantibody, whereas this antibody was undetectable or present at minimal levels in healthy controls (37). Koji and colleagues assessed serum autoantibody profiles and their association with blood eosinophils in a small group of COPD patients, but they did not find a link between serum autoantibodies and blood eosinophils (12). In the current study, we found that sputum differential autoantibodies exist in both eosinophilic and non-eosinophilic COPD, and serum differential autoantibodies only exist in eosinophilic COPD, indicating that abnormal localized autoimmunity is involved in both eosinophilic and non-eosinophilic COPD, but abnormal systemic autoimmunity is only involved in eosinophilic COPD. From this comparison, we found that six autoantibodies were decreased in COPD groups: sputum anti-HSP47_IgG, serum anti-Aβ_IgG, serum anti-HSP70_IgG, serum anti-HSP47_IgG, sputum anti-CollagenII_IgG and sputum anti-CollagenIV_IgG. Several previous studies reported lower levels of serum autoantibodies in COPD patients. Wood et al. demonstrated that serum anti-elastin autoantibody levels in COPD patients were significantly lower than those in healthy controls or in subjects with a1-antitrypsin deficiency (AATD) (33). Rinaldi et al. found that plasma anti-elastin autoantibody levels in COPD patients were significantly lower than those in healthy controls (22). Daffa et al. reported that serum anti-Collagen-1 autoantibody levels in smokers with COPD were significantly lower than those in smokers without COPD and those in non-smokers (35). The lower autoantibody levels in COPD groups may indicate the abnormal consumption of autoantibodies. This may be due to ongoing or increasing autoantibody consumption via binding to lung tissue or to circulating free self-antigens. In the current study, we identified three serum autoantibodies with lower levels in COPD patients than in healthy controls: anti-Aβ_IgG, anti-HSP70_IgG, and anti-HSP47_IgG. This is inconsistent with reports from previous studies that found higher levels of circulating target antigens (Aβ and HSP70) in patients with COPD and other chronic lung diseases as compared with healthy controls (45-47). There are no prior reports about HSP47 level in COPD patients, but HSP47 is a collagen-specific molecular chaperone (48), so this could partly explain why the lower anti-HSP47 level in COPD shows the same trend as anti-Collagen levels. However, there are other possibilities for the decreased autoantibody levels: (I) the presence of proteases that degrade IgG/IgM; (II) the presence of IgA that competes for binding but was not detected.
The current study expands our knowledge of the autoantibody responses and their correlation patterns between different airway inflammatory phenotypes in COPD, and raises several important points for discussion. First, in eosinophilic COPD, all hubs are autoantibodies, and most are sputum autoantibodies; based on this, it is reasonable to assume that these patients would benefit from ICS, which is consistent with previous clinical trials (5). There are 12 autoantibody hubs in eosinophilic COPD compared with only seven in non-eosinophilic COPD, therefore, eosinophilic COPD may be more relevant to autoimmunity than non-eosinophilic COPD. Second, lung functions, including large airway (FEV1%pred) and small airway (MMEF) parameters, are all hubs and negatively correlate with prior exacerbations in non-eosinophilic COPD, while this is not the case in eosinophilic COPD, indicating the clinical heterogeneity between these two COPD groups. Therefore, it is reasonable to assume that non-eosinophilic COPD cases would gain more benefit from bronchodilator treatment to reduce the exacerbation risk. Third, many anti-HSP antibodies are hubs in both COPD groups (Table 4), whereas this is not the case for normal controls. This indicated that autoimmunity to HSP may play a critical role in COPD pathogenesis, which is supported by evidence from other inflammatory disorders (49-51). In eosinophilic COPD, serum anti-HSP70 IgG negatively correlated with FEV1%pred, and its level was significantly lower than that in the healthy controls (Table 3), demonstrating a complex relationship between anti-HSP70 autoimmunity and lung function impairment in COPD. Yokota and colleagues reported that murine anti-HSP70 antibody could enhance HSP-induced proinflammatory cytokine and chemokine production via Toll-like receptor signalling in human peripheral blood mononuclear cells and human monocytic cells lines (49). Therefore, we inferred that anti-HSP autoimmunity may play a role in the pathogenesis of COPD, but that the network correlation patterns are different in different airway inflammatory phenotypes. Fourth, serum anti-Elastin_IgG and sputum anti-CollagenIV_IgG negatively correlated with prior exacerbation in eosinophilic COPD, whereas in non-eosinophilic COPD, prior exacerbation was positively correlated with sputum anti-Collagen-IV_IgG and negatively correlated with sputum anti-Vimentin_IgG and sputum anti-CENP-B_IgG, suggesting that autoantibody responses were heterogeneous and differentially associated with the exacerbation risk in the different airway inflammatory phenotypes of COPD.
Because the autoantibody responses (either natural or pathogenic) and their interrelationships in the airway and circulation are extremely complicated, the differential levels of autoantibody present in COPD patients do not necessarily indicate that these autoantibodies are pathogenic (52,53). Although the network medicine results cannot be used to draw exact conclusions about the biological mechanisms, they still provide insights that could help shape future clinical practice. First, according to the network theory, the hubs are a few highly connected nodes in the network that usually play a key role in the network (23,54). If a hub is damaged, the functionality of the entire network is likely to be jeopardised (55), so these points would be ideal candidate targets for potential treatments (56,57). Therefore, the hubs of lung function parameters in non-eosinophilic COPD and the hubs of autoantibodies are plausible targets that could potentially benefit from bronchodilator and ICS treatment, respectively. Second, the correlation patterns in different COPD groups will allow the identification of potential biomarkers for personalized predictions of the exacerbation risk, such as the autoantibodies indicative of eosinophilic COPD and the lung function parameters indicative of non-eosinophilic COPD. However, these preliminary results need to be validated by a longitudinal study with a large sample size.
The current study had two main strengths. First, we detected many previously reported autoantibodies related to COPD in the sputum and serum simultaneously. Second, we used network analysis to explore the interrelationships between autoantibodies, and cytological and clinical parameters in each of the groups. However, several limitations of our study should also be noted. First, it was a cross-sectional study; therefore, we must validate exacerbation-related factors using longitudinal cohort data. However, previous studies showed that the type of inflammatory responses during exacerbation may depend on the patient phenotype during stable disease, suggesting that patient parameters in stable disease and exacerbation are closely related. Second, due to the invasiveness of the procedure, we did not perform biopsies to confirm the airway inflammatory patterns. Third, owing to financial and sample volume constraints, the levels of IgA autoantibody were not evaluated. Fourth, the sample size of health control group was small, because it is difficult to collect sufficient sputum from healthy people. However, a convincing study with similar comparison had a smaller sample size in control group (58), so we think that this sample size may be acceptable. Fifth, the COPD subjects in the present study were predominantly male, and this skew toward more male patients may have resulted from the uneven sex distribution of the most important COPD risk factor. According to the Global Adults Tobacco Survey of 2018, 50.5% of males and 2.1% of females in China were current smokers (59). Cigarette smoking is the main risk factor for COPD in China, although in rural southern China the main COPD risk factor is exposure to biomass fuel (60). Patients in the current study were recruited from an affiliated hospital of a medical university in Guangzhou (the largest city in southern China). Thus, cigarette smoking would have been a major risk factor for COPD in this population, but because many more males than females are smokers, there was a corresponding sexual bias in our study subjects. Sixth, the number of current smokers in our control group is very small. It is possible that the observed differences in autoantibody levels between the COPD patients and controls may be due to the difference in smoking status between these groups. However, the goal of the current study was to compare the autoantibody profiles and their clinical associations in stable COPD patients with and without eosinophilic airway inflammation. As such, we do not think this limitation affects the main conclusions of our study. However, avoiding this limitation will be very important in the design of future research.
In conclusion, although the design of our study cannot draw conclusions about the cause-and-effect of the production of these autoantibodies in COPD patients, it shows that (I) autoantibody responses are heterogeneous and differentially associated with the exacerbation risk in different airway inflammatory phenotypes of COPD; (II) distinct correlation patterns among autoantibodies, the exacerbation risk and lung functions in different phenotypes provide useful insight into the need for personalized management for preventing exacerbations of COPD. However, further validation is needed by longitudinal study with large sample size.
Acknowledgments
Funding: This study was supported by the National Key R&D Program of China (2017YFC1310600, 2018YFC1311900 and 2016YFC0903700); Guangzhou Healthcare Collaborative Innovation Major Project (201604020012); Medical Scientific Research Foundation of Guangdong Province (A2019459, C2017050); and 111 Project (D18010).
Footnote
Conflicts of Interest: All authors have completed the ICMJE uniform disclosure form (available at http://dx.doi.org/10.21037/JTD-20-545). All authors report grants from National Key R&D Program of China (2017YFC1310600, 2018YFC1311900, 2016YFC0903700), grants from Medical Scientific Research Foundation of Guangdong Province (A2019459, C2017050), grants from Guangzhou Healthcare Collaborative Innovation Major Project (201604020012), grants from 111 Project (D18010) during the conduct of the study. RC serves as an unpaid Associate Editor-in-Chief of Journal of Thoracic Disease from Feb 2016 to Jan 2022.
Ethical Statement: The authors are accountable for all aspects of the work in ensuring that questions related to the accuracy or integrity of any part of the work are appropriately investigated and resolved. Written informed consent was obtained from all subjects. This study was conducted in accordance with the Declaration of Helsinki and approved by the ethics committee of the First Affiliated Hospital of Guangzhou Medical University (approval No.: 2017-22).
Open Access Statement: This is an Open Access article distributed in accordance with the Creative Commons Attribution-NonCommercial-NoDerivs 4.0 International License (CC BY-NC-ND 4.0), which permits the non-commercial replication and distribution of the article with the strict proviso that no changes or edits are made and the original work is properly cited (including links to both the formal publication through the relevant DOI and the license). See: https://creativecommons.org/licenses/by-nc-nd/4.0/.
References
- Vogelmeier CF, Criner GJ, Martinez FJ, et al. Global Strategy for the Diagnosis, Management, and Prevention of Chronic Obstructive Lung Disease 2017 Report. GOLD Executive Summary. Am J Respir Crit Care Med 2017;195:557-82. [Crossref] [PubMed]
- Brusselle GG, Joos GF, Bracke KR. New insights into the immunology of chronic obstructive pulmonary disease. Lancet 2011;378:1015-26. [Crossref] [PubMed]
- Tworek D, Antczak A. Eosinophilic COPD - a distinct phenotype of the disease. Adv Respir Med 2017;85:271-6. [Crossref] [PubMed]
- Saetta M, Di Stefano A, Maestrelli P, et al. Airway eosinophilia in chronic bronchitis during exacerbations. Am J Respir Crit Care Med 1994;150:1646-52. [Crossref] [PubMed]
- Leigh R, Pizzichini MM, Morris MM, et al. Stable COPD: predicting benefit from high-dose inhaled corticosteroid treatment. Eur Respir J 2006;27:964-71. [Crossref] [PubMed]
- Brightling CE, McKenna S, Hargadon B, et al. Sputum eosinophilia and the short-term response to inhaled mometasone in chronic obstructive pulmonary disease. Thorax 2005;60:193-8. [Crossref] [PubMed]
- Brightling CE, Monteiro W, Ward R, et al. Sputum eosinophilia and short-term response to prednisolone in chronic obstructive pulmonary disease: a randomised controlled trial. Lancet 2000;356:1480-5. [Crossref] [PubMed]
- Pizzichini E, Pizzichini MM, Gibson P, et al. Sputum eosinophilia predicts benefit from prednisone in smokers with chronic obstructive bronchitis. Am J Respir Crit Care Med 1998;158:1511-7. [Crossref] [PubMed]
- Cosio MG, Saetta M, Agusti A. Immunologic aspects of chronic obstructive pulmonary disease. N Engl J Med 2009;360:2445-54. [Crossref] [PubMed]
- Kheradmand F, Shan M, Xu C, et al. Autoimmunity in chronic obstructive pulmonary disease: clinical and experimental evidence. Expert Rev Clin Immunol 2012;8:285-92. [Crossref] [PubMed]
- Mukherjee M, Bulir DC, Radford K, et al. Sputum autoantibodies in patients with severe eosinophilic asthma. J Allergy Clin Immunol 2018;141:1269-79. [Crossref] [PubMed]
- Tamai K, Yoshimatsu H, Saito T, et al. Autoantibody profiles and their association with blood eosinophils in asthma and COPD. Allergol Int 2017;66:332-7. [Crossref] [PubMed]
- Durham AL, Caramori G, Chung KF, et al. Targeted anti-inflammatory therapeutics in asthma and chronic obstructive lung disease. Transl Res 2016;167:192-203. [Crossref] [PubMed]
- Lee J, Machin M, Russell KE, et al. Corticosteroid modulation of immunoglobulin expression and B-cell function in COPD. FASEB J 2016;30:2014-26. [Crossref] [PubMed]
- Caramori G, Ruggeri P, Di Stefano A, et al. Autoimmunity and COPD: Clinical Implications. Chest 2018;153:1424-31. [Crossref] [PubMed]
- Garudadri S, Woodruff PG. Targeting Chronic Obstructive Pulmonary Disease Phenotypes, Endotypes, and Biomarkers. Ann Am Thorac Soc 2018;15:S234-8. [Crossref] [PubMed]
- Bihlet AR, Karsdal MA, Sand JM, et al. Biomarkers of extracellular matrix turnover are associated with emphysema and eosinophilic-bronchitis in COPD. Respir Res 2017;18:22. [Crossref] [PubMed]
- Persson C, Uller L. Theirs but to die and do: primary lysis of eosinophils and free eosinophil granules in asthma. Am J Respir Crit Care Med 2014;189:628-33. [Crossref] [PubMed]
- Bhalla A, Mukherjee M, Nair P. Airway Eosinophilopoietic and Autoimmune Mechanisms of Eosinophilia in Severe Asthma. Immunol Allergy Clin North Am 2018;38:639-54. [Crossref] [PubMed]
- Ilmarinen P, Moilanen E, Kankaanranta H. Mitochondria in the center of human eosinophil apoptosis and survival. Int J Mol Sci 2014;15:3952-69. [Crossref] [PubMed]
- Packard TA, Li QZ, Cosgrove GP, Bowler RP, Cambier JC. COPD is associated with production of autoantibodies to a broad spectrum of self-antigens, correlative with disease phenotype. Immunol Res 2013;55:48-57. [Crossref] [PubMed]
- Rinaldi M, Lehouck A, Heulens N, et al. Antielastin B-cell and T-cell immunity in patients with chronic obstructive pulmonary disease. Thorax 2012;67:694-700. [Crossref] [PubMed]
- Barabási AL, Gulbahce N, Loscalzo J. Network medicine: a network-based approach to human disease. Nat Rev Genet 2011;12:56-68. [Crossref] [PubMed]
- Diez D, Agusti A, Wheelock CE. Network analysis in the investigation of chronic respiratory diseases. From basics to application. Am J Respir Crit Care Med 2014;190:981-8. [Crossref] [PubMed]
- Noell G, Faner R, Agustí A. From systems biology to P4 medicine: applications in respiratory medicine. European Respiratory Review 2018;27:170110. [Crossref] [PubMed]
- Roca J, Vargas C, Cano I, et al. Chronic Obstructive Pulmonary Disease heterogeneity: challenges for health risk assessment, stratification and management. J Transl Med 2014;12 Suppl 2:S3. [Crossref] [PubMed]
- Agustí A, Celli B, Faner R. What does endotyping mean for treatment in chronic obstructive pulmonary disease? Lancet 2017;390:980-7. [Crossref] [PubMed]
- Hu JX, Thomas CE, Brunak S. Network biology concepts in complex disease comorbidities. Nat Rev Genet 2016;17:615-29. [Crossref] [PubMed]
- Grosdidier S, Ferrer A, Faner R, et al. Network medicine analysis of COPD multimorbidities. Respir Res 2014;15:111. [Crossref] [PubMed]
- Divo MJ, Casanova C, Marin JM, et al. COPD comorbidities network. Eur Respir J 2015;46:640-50. [Crossref] [PubMed]
- Faner R, Gutierrez-Sacristan A, Castro-Acosta A, et al. Molecular and clinical diseasome of comorbidities in exacerbated COPD patients. Eur Respir J 2015;46:1001-10. [Crossref] [PubMed]
- Brandsma CA, Kerstjens HA, Geerlings M, et al. The search for autoantibodies against elastin, collagen and decorin in COPD. Eur Respir J 2011;37:1289-92. [Crossref] [PubMed]
- Wood AM, de Pablo P, Buckley CD, Ahmad A, Stockley RA. Smoke exposure as a determinant of autoantibody titre in alpha(1)-antitrypsin deficiency and COPD. Eur Respir J 2011;37:32-8. [Crossref] [PubMed]
- Bagdonas E, Raudoniute J, Bruzauskaite I, et al. Novel aspects of pathogenesis and regeneration mechanisms in COPD. Int J Chron Obstruct Pulmon Dis 2015;10:995-1013. [PubMed]
- Daffa NI, Tighe PJ, Corne JM, Fairclough LC, Todd I. Natural and disease-specific autoantibodies in chronic obstructive pulmonary disease. Clin Exp Immunol 2015;180:155-63. [Crossref] [PubMed]
- Kuo YB, Chang CA, Wu YK, et al. Identification and clinical association of anti-cytokeratin 18 autoantibody in COPD. Immunol Lett 2010;128:131-6. [Crossref] [PubMed]
- Newkirk MM, Mitchell S, Procino M, et al. Chronic smoke exposure induces rheumatoid factor and anti-heat shock protein 70 autoantibodies in susceptible mice and humans with lung disease. Eur J Immunol 2012;42:1051-61. [Crossref] [PubMed]
- Lee SH, Goswami S, Grudo A, et al. Antielastin autoimmunity in tobacco smoking-induced emphysema. Nat Med 2007;13:567-9. [Crossref] [PubMed]
- Miller MR, Hankinson J, Brusasco V, et al. Standardisation of spirometry. Eur Respir J 2005;26:319-38. [Crossref] [PubMed]
- Paggiaro PL, Chanez P, Holz O, et al. Sputum induction. Eur Respir J Suppl 2002;37:3s-8s. [PubMed]
- Bafadhel M, McCormick M, Saha S, et al. Profiling of sputum inflammatory mediators in asthma and chronic obstructive pulmonary disease. Respiration 2012;83:36-44. [Crossref] [PubMed]
- Wang F, Liang Z, Yang Y, et al. Reproducibility of fluid-phase measurements in PBS-treated sputum supernatant of healthy and stable COPD subjects. Int J Chron Obstruct Pulmon Dis 2019;14:835-52. [Crossref] [PubMed]
- Simpson JL, Scott R, Boyle MJ, et al. Inflammatory subtypes in asthma: assessment and identification using induced sputum. Respirology 2006;11:54-61. [Crossref] [PubMed]
- Greene CM, Low TB, O'Neill SJ, et al. Anti-proline-glycine-proline or antielastin autoantibodies are not evident in chronic inflammatory lung disease. Am J Respir Crit Care Med 2010;181:31-5. [Crossref] [PubMed]
- Dong J, Guo L, Liao Z, et al. Increased expression of heat shock protein 70 in chronic obstructive pulmonary disease. Int Immunopharmacol 2013;17:885-93. [Crossref] [PubMed]
- Hacker S, Lambers C, Hoetzenecker K, et al. Elevated HSP27, HSP70 and HSP90 alpha in chronic obstructive pulmonary disease: markers for immune activation and tissue destruction. Clin Lab 2009;55:31-40. [PubMed]
- Bu XL, Cao GQ, Shen LL, et al. Serum Amyloid-Beta Levels are Increased in Patients with Chronic Obstructive Pulmonary Disease. Neurotox Res 2015;28:346-51. [Crossref] [PubMed]
- Nagata K. Hsp47: a collagen-specific molecular chaperone. Trends Biochem Sci 1996;21:22-6. [Crossref] [PubMed]
- Yokota S, Minota S, Fujii N. Anti-HSP auto-antibodies enhance HSP-induced pro-inflammatory cytokine production in human monocytic cells via Toll-like receptors. Int Immunol 2006;18:573-80. [Crossref] [PubMed]
- Tukaj S, Gorog A, Kleszczynski K, et al. Autoimmunity to heat shock proteins and vitamin D status in patients with celiac disease without associated dermatitis herpetiformis. J Steroid Biochem Mol Biol 2017;173:23-27. [Crossref] [PubMed]
- Yokota S, Fujii N. Immunomodulatory activity of extracellular heat shock proteins and their autoantibodies. Microbiol Immunol 2010;54:299-307. [PubMed]
- Byrne R, Todd I, Tighe PJ, et al. Autoantibodies in chronic obstructive pulmonary disease: A systematic review. Immunol Lett 2019;214:8-15. [Crossref] [PubMed]
- Duncan SR. Perspective: Clues, not conclusions. Nature 2012;489:S15. [Crossref] [PubMed]
- Benson M, Breitling R. Network theory to understand microarray studies of complex diseases. Curr Mol Med 2006;6:695-701. [Crossref] [PubMed]
- Faner R, Cruz T, Lopez-Giraldo A, et al. Network medicine, multimorbidity and the lung in the elderly. Eur Respir J 2014;44:775-88. [Crossref] [PubMed]
- Vitali F, Mulas F, Marini P, et al. Network-based target ranking for polypharmacological therapies. J Biomed Inform 2013;46:876-81. [Crossref] [PubMed]
- Barneh F, Jafari M, Mirzaie M. Updates on drug-target network; facilitating polypharmacology and data integration by growth of DrugBank database. Brief Bioinform 2016;17:1070-80. [PubMed]
- Mukherjee M, Thomas SR, Radford K, et al. Sputum Antineutrophil Cytoplasmic Antibodies in Serum Antineutrophil Cytoplasmic Antibody-Negative Eosinophilic Granulomatosis with Polyangiitis. Am J Respir Crit Care Med 2019;199:158-70. [Crossref] [PubMed]
- World Health Organization. Global Adult Tobacco Survey (GATS) (China 2018), 2019.
- Liu S, Zhou Y, Wang X, et al. Biomass fuels are the probable risk factor for chronic obstructive pulmonary disease in rural South China. Thorax 2007;62:889-97. [Crossref] [PubMed]