Persistent pulmonary subsolid nodules with a solid component smaller than 6 mm: what do we know?
We thank Prof. Park et al. from Korea very much for their wonderful comments on our recent article (1). Our group published a study in the Journal of Thoracic Disease, which explored the role of qualitative and quantitative imaging features of pulmonary subsolid nodules (SSNs) in differentiating invasive adenocarcinoma (IAC) from minimally invasive adenocarcinoma and preinvasive lesions (2). In this retrospective single-center study, 316 surgically resected SSNs [260 pure ground-glass nodules (pGGNs), 47 part-solid nodules (PSNs) with solid components ≤5 mm, and 9 ground-glass nodules with cystic airspaces] from 287 patients were included. We reported that the IAC was the most common pathological type among these enrolled SSNs. SSN mass was a significant predictor of IAC (odds ratio, 1.007; P<0.001), with an optimal cutoff value of 283.2 mg (area under curve: 0.859; sensitivity: 68.7%; specificity: 92.9%) (2).
To date, there are still dilemmas in clinical management of pulmonary SSNs, primarily because most persistent pulmonary SSNs pathologically represent pre-invasive or invasive adenocarcinomas (2) and ironically showed an indolent course (3,4). The current guidelines for management of incidental pulmonary nodules recommend that long-term follow-up rather than immediate surgery should be considered for pGGNs and PSNs with a solid component smaller than 6 mm (5). These two previous studies have shown that “follow-up until interval growth” for PSNs with a solid component of ≤5 mm or pGGNs will not negatively influence the patients’ prognosis (6,7). Additionally, we found that IACs appearing as SSNs, with the mean volume doubling time of 1,436.0±1,188.2 days, showed an indolent clinical course, which is under review by European Radiology. Therefore, although our study reported that IAC was the most common pathological type among pGGNs and PSNs with solid components ≤5 mm (2), we fully agree with the “follow-up until interval growth” policy for SSNs with solid portion smaller than 6 mm.
However, in clinical practice, we found that many patients with these SSNs choose surgical resection, mainly in Asia, which may be related to the health insurance policy and patients’ anxiety. Moreover, numerous studies have demonstrated that pulmonary SSNs with a solid component smaller than 6 mm could be IACs in histopathology. Thus, prediction of IAC likelihood among these patients who choose surgery would be very helpful in deciding the optimal surgical method and evaluating prognosis, which was the purpose of many previous studies.
The proportions of IACs in SSNs were different among various studies. Interestingly, the proportion of IACs in pGGNs was quite high in these Asian studies (2,8-11), ranging from 35% to 61.6%. The reasons for the higher proportion have been explained in our article (2). However, as mentioned in Prof. Park’s commentary (1), intra- or inter-reader variability should also be taken into account. As we all know, not only nodule measurement and evaluations of morphological features, but also nodule classification (pGGN or PSN) was vulnerable to inter- and intra-reader variability, which will affect the interpretation of research results. It is a pity that neither of them has been evaluated in most previous studies, including our study. This should arouse the attention of all scholars in the future research. Kindly reminder, in our study, the two doctors who were mainly responsible for image analysis had relatively rich clinical experience and special training in pulmonary SSNs; disagreements were resolved through consultation with a senior radiologist (2).
Numerous prediction models about pathological classification of SSNs have been reported, but as far as we know, only few studies took SSN mass into account (2,8,9,11). Mass measurement can simultaneously reflect SSN volume and density, and the variability of mass measurement was significantly smaller than that of volume measurement (4). Nevertheless, it is undeniable that the use of contrast media will affect the measurement of SSN density and mass. However, thoracic surgeons usually use enhanced CT to clearly observe vessels and plan sublobectomy before operation. Thus, in order to minimize the impact of contrast media, as described in the Image acquisition and analysis section of our article, we excluded portions of apparent vessels to measure SSN density and mass (2). In addition, enhanced CT images were obtained 35 s after the intravenous injection in our study, at which time the contrast media had relatively little effect on the SSN analysis. Perhaps deep-learning technique can automatically detect and eliminate blood vessels passing through SSNs, thereby helping to accurately measure the SSN density and mass, which requires further study.
In recent years, deep-learning techniques, which can automatically acquire features for nodule detection and classification, have been employed to differentiate IACs among pulmonary SSNs (12-15). The performance of deep-learning techniques and quantitative CT in differentiating IACs among SSNs is comparatively summarized in Figure 1. We found that compared to quantitative CT, the deep-learning technique obtained better or similar performance; it also yielded higher performance than radiologists in predicting IACs among SSNs (12,13). The deep-learning technique has many advantages in this field, superior reproducibility and not limited by the radiologists’ experience. However, the deep-learning technique also has many shortcomings, and it needs a large amount of manual label data to train the model and test the results, which is expensive, time-consuming, and laborious, greatly increased the cost of system construction.
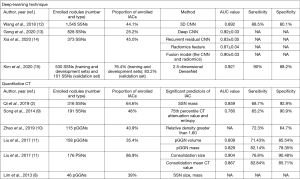
However, most of these prediction models on this topic have not been validated in an external cohort or an in-house prospective cohort. As far as we know, in the current clinical practice, differentiating IACs from minimally/pre-IACs among SSNs is still mainly based on the size of whole nodule, solid portion and radiologists’ experience. We will prospectively validate the model reported by our study in future clinical practice. For these prediction models based on traditional 3D measurements, there is still much work to be done to apply them to clinical work. In contrast, we believe that these prediction models based on deep-learning techniques may be applied to clinical practice in the near future.
Additionally, many studies have explored the relationship between the clinical and radiologic characteristics of SSNs and the patients’ survival. However, to our knowledge, SSN mass has not been taken into account. As mentioned in Prof. Park’s commentary, this is an excellent direction. We are very willing to pay close attention to the prognosis of these enrolled patients and explore the correlation between SSN mass and the patients’ survival. Moreover, we believe that deep-learning technique may be another great way to evaluate prognosis.
In summary, we believe that long-term follow-up rather than immediate surgery should be recommended for SSNs with a solid component smaller than 6 mm, even if some of them will progress into IACs. In some Asian studies, IACs accounted for a relatively high proportion in SSNs with a solid composition smaller than 6mm, which may be due to several reasons. A large-scale and multi-center study may be needed to verify this, and the participation of pathologists is also necessary. Furthermore, deep-learning techniques may be very helpful in the detection, follow-up, classification, and prognosis assessment of pulmonary SSNs.
Acknowledgments
Funding: This work was supported by the National Key R&D Program of China [grant number 2017YFC1308700]; the National Natural Science Foundation of China [grant numbers 81171344, 81971616]; and Chinese Academy of Medical Sciences Initiative for Innovative Medicine [grant numbers 2017-I2M-1-005, 2019-12M-2-002].
Footnote
Conflicts of Interest: The authors have completed the ICMJE uniform disclosure from (available at http://dx.doi.org/10.21037/jtd-20-1972). The authors have no conflicts of interests to declare.
Ethical Statement: The authors are accountable for all aspects of the work in ensuring that questions related to the accuracy or integrity of any part of the work are appropriately investigated and resolved.
Open Access Statement: This is an Open Access article distributed in accordance with the Creative Commons Attribution-NonCommercial-NoDerivs 4.0 International License (CC BY-NC-ND 4.0), which permits the non-commercial replication and distribution of the article with the strict proviso that no changes or edits are made and the original work is properly cited (including links to both the formal publication through the relevant DOI and the license). See: https://creativecommons.org/licenses/by-nc-nd/4.0/.
References
- Lee JH, Park CM. Differentiation of persistent pulmonary subsolid nodules with a solid component smaller than 6 mm: to be invasive adenocarcinoma or not to be? J Thorac Dis 2020;12:1754-7. [Crossref] [PubMed]
- Qi L, Lu W, Yang L, et al. Qualitative and quantitative imaging features of pulmonary subsolid nodules: differentiating invasive adenocarcinoma from minimally invasive adenocarcinoma and preinvasive lesions. J Thorac Dis 2019;11:4835-46. [Crossref] [PubMed]
- Qi LL, Wu BT, Tang W, et al. Long-term follow-up of persistent pulmonary pure ground-glass nodules with deep learning-assisted nodule segmentation. Eur Radiol 2020;30:744-55. [Crossref] [PubMed]
- Song YS, Park CM, Park SJ, et al. Volume and mass doubling times of persistent pulmonary subsolid nodules detected in patients without known malignancy. Radiology 2014;273:276-84. [Crossref] [PubMed]
- MacMahon H, Naidich DP, Goo JM, et al. Guidelines for management of incidental pulmonary nodules detected on CT images: from the Fleischner Society 2017. Radiology 2017;284:228-43. [Crossref] [PubMed]
- Lee JH, Park CM, Kim H, et al. Persistent part-solid nodules with solid part of 5 mm or smaller: Can the 'follow-up and surgical resection after interval growth' policy have a negative effect on patient prognosis? Eur Radiol 2017;27:195-202. [Crossref] [PubMed]
- Yankelevitz DF, Yip R, Smith JP, et al. CT screening for lung cancer: Nonsolid nodules in baseline and annual repeat rounds. Radiology 2015;277:555-64. [Crossref] [PubMed]
- Lim HJ, Ahn S, Lee KS, et al. Persistent pure ground-glass opacity lung nodules ≥10 mm in diameter at CT scan: histopathologic comparisons and prognostic implications. Chest 2013;144:1291-9. [Crossref] [PubMed]
- Son JY, Lee HY, Lee KS, et al. Quantitative CT analysis of pulmonary ground-glass opacity nodules for the distinction of invasive adenocarcinoma from pre-invasive or minimally invasive adenocarcinoma. PLoS One 2014;9:e104066. [Crossref] [PubMed]
- Zhao Q, Wang JW, Yang L, et al. CT diagnosis of pleural and stromal invasion in malignant subpleural pure ground-glass nodules: an exploratory study. Eur Radiol 2019;29:279-86. [Crossref] [PubMed]
- Liu Y, Sun H, Zhou F, et al. Imaging features of TSCT predict the classification of pulmonary preinvasive lesion, minimally and invasive adenocarcinoma presented as ground glass nodules. Lung Cancer 2017;108:192-7. [Crossref] [PubMed]
- Wang S, Wang R, Zhang S, et al. 3D convolutional neural network for differentiating pre-invasive lesions from invasive adenocarcinomas appearing as ground-glass nodules with diameters ≤3 cm using HRCT. Quant Imaging Med Surg 2018;8:491-9. [Crossref] [PubMed]
- Gong J, Liu J, Hao W, et al. A deep residual learning network for predicting lung adenocarcinoma manifesting as ground-glass nodule on CT images. Eur Radiol 2020;30:1847-55. [Crossref] [PubMed]
- Xia X, Gong J, Hao W, et al. Comparison and fusion of deep learning and radiomics features of ground-glass nodules to predict the invasiveness risk of stage-I lung adenocarcinomas in CT scan. Front Oncol 2020;10:418. [Crossref] [PubMed]
- Kim H, Lee D, Cho WS, et al. CT-based deep learning model to differentiate invasive pulmonary adenocarcinomas appearing as subsolid nodules among surgical candidates: comparison of the diagnostic performance with a size-based logistic model and radiologists. Eur Radiol 2020;30:3295-305. [Crossref] [PubMed]