Development of a nomogram for preoperative prediction of lymph node metastasis in non-small cell lung cancer: a SEER-based study
Introduction
Lung cancer remains the most frequent malignancy and one of the leading causes of cancer-specific morbidity and mortality among men and women worldwide (1). Non-small cell lung cancer (NSCLC) is the most common histology type and represents 85% of all newly diagnosed lung cancer cases (2). Five-year survival rate of lung cancer is approximately 17% for all stages according to the latest cancer statistics, even though targeted therapy and immunotherapy has started a revolution in management and achieved remarkable progress in recent years (3,4). Considering the relatively low survival rate, a better understanding of cancer biology, earlier and more precise diagnosis, improved staging system and novel therapy is all in urgent need for better outcomes of patients in the future.
Accurate staging is a crucial step after diagnosis to provide optimal treatment and metastases to lymph nodes is one of the key factors in the staging system which is closely related to prognosis (5). PET-CT scan has gradually become the most important examination for lymph node status. Nodes showing greater 18F-FDG uptake at PET without benign calcification or high attenuation >70 household unit (HU) at unenhanced CT were regarded as being positive for malignancy. PET-CT has improved specificity and negative predictive value and help surgeons to determine patients who will benefit the most from the surgery. However high specificity comes with a price of high rate of false positives and possible histological confirmation is recommended according to previous studies (6). Assessment of lymph node metastasis also relies on traditional computed tomography (CT) results and lymph nodes present with short-axis diameters of >1 cm are generally defined as metastatic lesions (7). However, predicting accuracy of CT scan is not satisfactory enough as sensitivity is 43–86% and specificity is 59–83% (8). Other invasive diagnostic methods such as mediastinoscopy and endoscopic ultrasound-guided fine-needle aspiration are valuable but not routinely used in clinical practice (9).
Complete surgery resection is standard care for early stage NSCLC patients confirmed with no distant metastasis (10). Pulmonary and mediastinal lymph nodes dissection is widely performed for patients suspected with lymph node metastases and is believed to be beneficial supported by abundant evidence (11,12). Since evaluation of CT results had its limitation, 15–17% of patients diagnosed as N0 via preoperative CT scan was proved to be N2 according to pathological examination while some other patients classified as N0 came back with positive lymph nodes (13). To decide the risk of lymph node involvement and therefore the extent of lymph node dissection during operation, accurate assessment before surgery become necessary.
Several factors have been revealed to be related with lymph node metastasis by previous studies, including age, tumor size, pleural invasion etc. (14-16). However, there is no convenient clinical model for risk prediction so far. We intended to build a model with clinicopathological factors to predict the risk of lymph node metastasis for patients with NSCLC. In the present study, we did a retrospective research on data released from SEER database to identify clinicopathologic features correlating with lymph node metastasis in NSCLC patients. Afterwards a nomogram was developed for the first time based on our knowledge of literature to predict the risk of lymph node involvement prior to surgical intervention. We present the following article in accordance with the STROBE reporting checklist (available at http://dx.doi.org/10.21037/jtd-20-601).
Methods
Ethical statement
The trial was conducted in accordance with the Declaration of Helsinki and the Harmonized Tripartite Guideline for Good Clinical Practice from the International Conference on Harmonization. This study was approved by Ethical Committee of Shanghai Cancer Center, Fudan University. Patient consent form was not required for data from SEER database as all data was deidentified before release and contained no personally identifying information of patients. No specific funding from public, commercial agencies or individual was received in the present study.
Patient population
Surveillance, Epidemiology, and End Results (SEER) database collects cancer patient information from 18 separate cancer registries all across the United States and covers about one quarter population of the whole country (17). The population entered the database are considered to be able to represent the overall population. SEER*Stat version 8.3.5 was used to generate a case listing file. A general description of our study design was present in Figure 1.
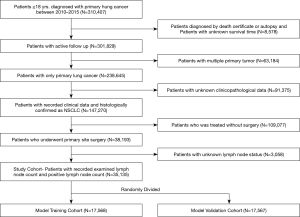
Patient selection
A total of 35,138 patients were selected into our study cohort. Inclusion criteria was as followed: (I) patients over 20 years old at diagnosis (II) patients diagnosed with primary lung cancer between 2010 to 2015 with site codes as C34.0-C34.9 (lung and bronchus). (III) patients who received a primary site surgery (IV) positive pathologic confirmation of histologic type as adenocarcinoma (8140–8147, 8255, 8260, 8310, 8323, 8480, 8481, 8490, 8550, 8572), squamous cell carcinoma (8050–8052, 8070–8078), or other (8010, 8012, 8014–8015, 8020–8022, 8030, 8036) (V) active follow up (VI) survival time over 1 month after surgery. Exclusion criteria included (I) diagnoses made by autopsy or death certificate (II) multiple primary tumor (III) no lymph node examined (VI) unknown disease stage at diagnosis. Patients with stage IIIB or stage IV disease were also excluded since surgery was no standard procedure for these patients. Clinicopathologic features extracted were age at diagnosis, sex, race, histology type, clinical stage, T status, tumor size, histologic grade, laterality. We randomly divided all population into two separate sets: a training set with 17,568 patients and a validation set with 17,567 patients.
Statistical analysis
Statistical analyses were conducted using SPSS for windows (version 24.0, SPSS Inc., Chicago, IL, USA) and RStudio for windows supported by R programming language and environment version 3.5.1. Pearson’s chi-square test or Fisher’s exact test when needed were applied to compare all variables between training set and validation set. To identify risk factors for lymph node metastasis, multivariable analysis was performed by binary logistic regression analysis. All features available for preoperative assessment were included into the multivariable analysis.
A logistic regression model-based nomogram was developed using the training set. All variables with a P value <0.05 were included into model construction unless specifically stated otherwise. Nomogram performance was evaluated by both internal validation in the training set and external validation in the validation set. We used bootstrapping method (1,000 repetitions) to generate a calibration curve plotted with observed outcome frequencies and predicted probabilities of lymph node metastasis. Discrimination ability was measured by means of the area under the receiver operating characteristic (ROC) curve (AUC or C-index). All tests were two-sided and P value <0.05 was considered as significant.
Results
Patient characteristics
The clinicopathologic features of all patients were listed in Table 1. After selection 35,138 patients were eligible for our study. For all patients, median follow-up time was 16 months (with a range from1–44 months) 42.2% of all patients were over 70 years old and 57.8% were under. As for race, the majority of the population were white and other races counted for 15.87%. Patients distributed evenly over the diagnosis years. Male and Female patients distributed almost evenly with percentage of 48.32% and 51.68%. 68.03% of patients were histologically classified as adenocarcinoma and 29.16% were squamous cell carcinoma. 41.89% of all lung tumors were located on the left size and 36.76% were small lesions with a diameter under 20 mm. In post operational pathologic examination, 21.83% patients were found with positive lymph node metastasis. There was no significant difference between training and validation set which was consistent with our method of random sampling. We also investigated how different types of surgery will influence the results of lymph node status. The results were presented in Table 2. Most patients went through Resection of one lobe or bilobectomy with mediastinal lymph node dissection, however this procedure held the lowest average positive rate.
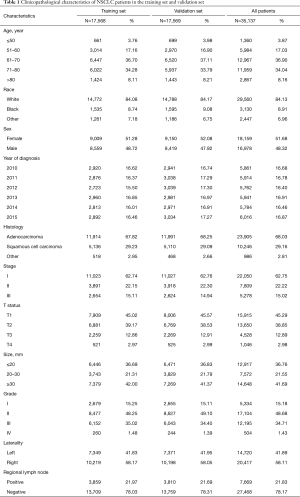
Full table
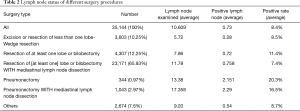
Full table
Identification of risk factors corelating with lymph node metastasis
To reveal factors which have influence on lymph node metastasis, we first performed a univariant analysis in both training and validation set as Table 3 summarized the results. The results showed that factors most strongly related to lymph node metastasis were age at diagnosis, sex, stage, T status, tumor size, grade and laterality. Notably, histology type, which is usually an important indicator for staging and prognosis, had no significant influence on lymph node involvement.
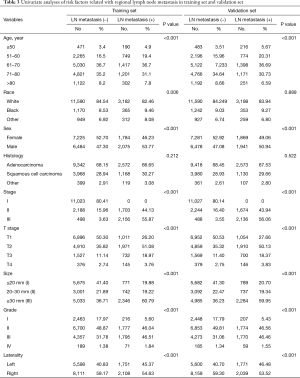
Full table
Considering our predictive model will be used before surgery, we entered all features that would be available in preoperational evaluation into a binary logistic regression analysis. These factors included age, sex, race, histology, T status, tumor size, grade and laterality and analysis results were presented in Table 4. According to this multivariant analysis results, age, sex, T status, tumor size, grade and laterality were independent variables that cast significant influence on lymph node positivity and were furthered taken into nomogram development.
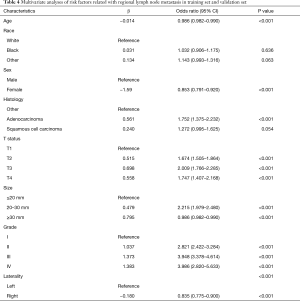
Full table
Nomogram construction
We then constructed a nomogram on the basis of a logistic regression model developed by our training set data. Significant risk factors indicated by the logistic regression analysis were fit into the model. The final nomogram was presented in Figure 2.
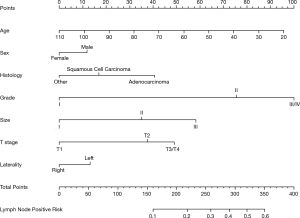
Internal and external validation of the nomogram
Validation was first performed in training set internally. The model had an AUC of 0.696 (95% CI, 0.617 to 0.775), which showed good discrimination. Bootstrapping method (1,000 repetitions) was used and a calibration curve was illustrated in Figure 3. There was no obvious deviation between model predicted risk and actual observed risk curve, meaning the model was well calibrated. We further validated the model in the validation set using the same method. Good calibration was observed and the AUC was 0.693 (95% CI, 0.628 to 0.758) demonstrating the nomogram was well fitted.
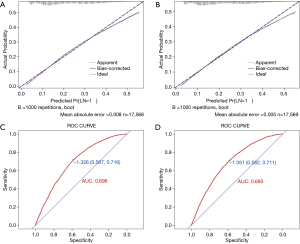
Discussion
Due to rapid development of novel screening approaches such as PET-CT and widely applied annual health check, more and more lung cancer patients were detected at an early stage of disease (18). Surgery remains the most effective and possible curable method for these patients and lobectomy with systematic lymph node dissection (LND) is the most common procedure. Traditionally, LND is believed to be capable of providing more accurate stage, detecting occult lesions and improving overall survival (19). However, with the appearance of more early stage lung cancer patients, how to minimize the surgical trauma and shorten the hospital stay should also be taken into consideration. Also, it is not a rare scenario that postoperative pathologic examination reveals no lymph node metastasis. Overly removal of intact lymph nodes will cause longer surgery time, more blood loss and impaired regional immune function which can be harmful for elderly patients and patients with defective pulmonary function (20,21). There is a rising opinion that selective lymph node dissection (SLND) may be a better alternative for systematic lymph node dissection. Han et al. proposed that SLND will be a vital component of minimally invasive surgical treatment and may lead to better life quality afterwards (22). Therefore, it’s not hard to understand that preoperative lymph node status assessment is of great importance to determine the best lymph node dissection pattern.
Several factors were reported to be relevant to lymph node metastasis in lung cancer. Xia et al. revealed that young age increased risk of lymph node involvement and Ding et al. reported that tumor size, histologic differentiation and smoking status were corelated to LN positivity (14,23). So far there is no easy to use clinical tool to estimate individual risk of lymph node metastasis. Nomogram is a pictorial presentation of complex mathematic regression model. It has emerged has a simpler and more advanced method for clinical event prediction and gradually become an important component of decision making (24). Hence, we aimed to develop a nomogram combining clinicopathologic factors to serve as a comprehensive, user friendly, effective clinical prediction model.
In the present study, we utilized data released from large population cancer database SEER and 35,138 patients fit into our selection criteria mentioned above and was included into our study cohort. We first performed univariant and multivariant analysis and age, sex, T status, tumor size, grade and laterality were distinguished as independent variables involving the lymph node metastasis. Patients with younger age, poorly differentiation, higher T stage and larger size tumor are facing higher risk. We took these preoperative assessment available factors into our nomogram construction and the model showed good discrimination and calibration. When using the nomogram for a specific patient, first collect all the clinicopathologic feature listed and put them into the scale and get a score for each variable. Next add all scores up to get a total score and match with a probability on the risk scale. This model enables physicians to calculate a specific lymph node metastasis risk for each patient and help them decide which patients will possibly benefit most from more extensive lymph node dissection and make better surgery plan.
Recent research has put much effort into understanding the molecular mechanism underlying lymph node metastasis in lung cancer. Moriya et al. reported a gene profiling method and selected sets of gene predictor for lymph node metastasis (21). Gomez et al. reported higher expression of E-cadherin, γ-catenin, p27, and p53 in patients with positive lymph node while p16 and Rb were expressed in negative cases (25). With more and more clear interpretation of lymph node metastasis molecular biology in the future, our model would serve as a basic platform to identify high risk patients for further gene or protein level profiling examinations to achieve more accurate staging, more individualized management and hopefully better outcomes.
There are certain limitations of our study that we need to point out. Firstly, as this is a retrospective study, selection bias should be considered. White people constituted vast majority of the study cohort which can make prediction less accurate for people of other races and influence the model application in other countries. Secondly, There is some missing detailed information in SEER database like smoking history, genetic mutations, accurate recording of positive lymph node location and any preoperative chemotherapy or regional radiotherapy which will affect lymph node status. EGFR mutation is a very important factor in lung cancer management today and may have certain effect on lymph node status. Also, it would be better if PET scan results are also taken into consideration. These variables will be a major part of our future research to improve this model. Thirdly, further external validation of more multi-institution cohorts from around the world are required to confirm our results.
Acknowledgments
Funding: None.
Footnote
Reporting Checklist: The authors have completed the STROBE reporting checklist. Available at http://dx.doi.org/10.21037/jtd-20-601
Conflicts of Interest: All authors have completed the ICMJE uniform disclosure form (available at http://dx.doi.org/10.21037/jtd-20-601). The authors have no conflicts of interest to declare.
Ethical Statement: The authors are accountable for all aspects of the work in ensuring that questions related to the accuracy or integrity of any part of the work are appropriately investigated and resolved. The trial was conducted in accordance with the Declaration of Helsinki (as revised in 2013) and the Harmonized Tripartite Guideline for Good Clinical Practice from the International Conference on Harmonization. This study was approved by Ethical Committee of Shanghai Cancer Center, Fudan University. Patient consent form was not required for data from SEER database as all data was deidentified before release and contained no personally identifying information of patients.
Open Access Statement: This is an Open Access article distributed in accordance with the Creative Commons Attribution-NonCommercial-NoDerivs 4.0 International License (CC BY-NC-ND 4.0), which permits the non-commercial replication and distribution of the article with the strict proviso that no changes or edits are made and the original work is properly cited (including links to both the formal publication through the relevant DOI and the license). See: https://creativecommons.org/licenses/by-nc-nd/4.0/.
References
- Gridelli C, Rossi A, Carbone DP, et al. Non-small-cell lung cancer. Nat Rev Dis Primers 2015;1:15009. [Crossref] [PubMed]
- Jamal-Hanjani M, Wilson GA, McGranahan N, et al. Tracking the Evolution of Non-Small-Cell Lung Cancer. N Engl J Med 2017;376:2109-21. [Crossref] [PubMed]
- Herzberg B, Campo MJ, Gainor JF. Immune checkpoint inhibitors in non-small cell lung cancer. Oncologist 2017;22:81-8. [Crossref] [PubMed]
- Masri M, McManus M, Mudad R. Treatment of Advanced Non-Small Cell Lung Cancer in the Era of Targeted Therapy. Curr Pulmonol Rep 2018;7:79-91. [Crossref]
- Reck M, Rabe KF. Precision diagnosis and treatment for advanced non–small-cell lung cancer. N Engl J Med 2017;377:849-61. [Crossref] [PubMed]
- de Castro ABG, Domínguez JF, Bolton RD, et al. PET-CT in presurgical lymph node staging in non-small cell lung cancer: The importance of false-negative and false-positive findings. Radiologia 2017;59:147-58. [PubMed]
- El-Sherief AH, Lau CT, Obuchowski NA, et al. Cross-Disciplinary Analysis of Lymph Node Classification in Lung Cancer on CT Scanning. Chest 2017;151:776-85. [Crossref] [PubMed]
- Peng Z, Liu Q, Li M, et al. Comparison of (11)C-choline PET/CT and enhanced CT in the evaluation of patients with pulmonary abnormalities and locoregional lymph node involvement in lung cancer. Clin Lung Cancer 2012;13:312-20. [Crossref] [PubMed]
- Eloubeidi MA, Cerfolio RJ, Chen VK, et al. Endoscopic ultrasound-guided fine needle aspiration of mediastinal lymph node in patients with suspected lung cancer after positron emission tomography and computed tomography scans. Ann Thorac Surg 2005;79:263-8. [Crossref] [PubMed]
- Gagliasso M, Migliaretti G, Ardissone F. Assessing the prognostic impact of the International Association for the Study of Lung Cancer proposed definitions of complete, uncertain, and incomplete resection in non-small cell lung cancer surgery. Lung Cancer 2017;111:124-30. [Crossref] [PubMed]
- Keller SM, Adak S, Wagner H, et al. Mediastinal lymph node dissection improves survival in patients with stages II and IIIa non-small cell lung cancer. Ann Thorac Surg 2000;70:358-65. [Crossref] [PubMed]
- Lardinois D, Suter H, Hakki H, et al. Morbidity, survival, and site of recurrence after mediastinal lymph-node dissection versus systematic sampling after complete resection for non-small cell lung cancer. Ann Thorac Surg 2005;80:268-74;discussion 274-5. [Crossref] [PubMed]
- Park HK, Jeon K, Koh WJ, et al. Occult nodal metastasis in patients with non-small cell lung cancer at clinical stage IA by PET/CT. Respirology 2010;15:1179-84. [Crossref] [PubMed]
- Ding N, Mao Y, Gao S, et al. Predictors of lymph node metastasis and possible selective lymph node dissection in clinical stage IA non-small cell lung cancer. J Thorac Dis 2018;10:4061-8. [Crossref] [PubMed]
- Xia W, Wang A, Jin M, et al. Young age increases risk for lymph node positivity but decreases risk for non-small cell lung cancer death. Cancer Manag Res 2018;10:41-8. [Crossref] [PubMed]
- Yu X, Li Y, Shi C, et al. Risk factors of lymph node metastasis in patients with non-small cell lung cancer ≤ 2 cm in size: A monocentric population-based analysis. Thorac Cancer 2018;9:3-9. [Crossref] [PubMed]
- Soneji S, Beltran-Sanchez H, Sox HC. Assessing progress in reducing the burden of cancer mortality, 1985-2005. J Clin Oncol 2014;32:444-8. [Crossref] [PubMed]
- Senan S, Paul MA, Lagerwaard FJ. Treatment of early-stage lung cancer detected by screening: surgery or stereotactic ablative radiotherapy? Lancet Oncol 2013;14:e270-4. [Crossref] [PubMed]
- Doddoli C, Aragon A, Barlesi F, et al. Does the extent of lymph node dissection influence outcome in patients with stage I non-small-cell lung cancer? Eur J Cardiothorac Surg 2005;27:680-5. [Crossref] [PubMed]
- Ishiguro F, Matsuo K, Fukui T, et al. Effect of selective lymph node dissection based on patterns of lobe-specific lymph node metastases on patient outcome in patients with resectable non–small cell lung cancer: A large-scale retrospective cohort study applying a propensity score. J Thorac Cardiovasc Surg 2010;139:1001-6. [Crossref] [PubMed]
- Moriya Y, Iyoda A, Kasai Y, et al. Prediction of lymph node metastasis by gene expression profiling in patients with primary resected lung cancer. Lung Cancer 2009;64:86-91. [Crossref] [PubMed]
- Han H, Chen H. Selective lymph node dissection in early-stage non-small cell lung cancer. J Thorac Dis 2017;9:2102-7. [Crossref] [PubMed]
- Zhao F, Zhou Y, Ge PF, et al. A prediction model for lymph node metastases using pathologic features in patients intraoperatively diagnosed as stage I non-small cell lung cancer. BMC Cancer 2017;17:267. [Crossref] [PubMed]
- Balachandran VP, Gonen M, Smith JJ, et al. Nomograms in oncology: more than meets the eye. Lancet Oncol 2015;16:e173-80. [Crossref] [PubMed]
- Gomez AM, Jarabo Sarceda JR, Garcia-Asenjo JA, et al. Relationship of immunohistochemical biomarker expression and lymph node involvement in patients undergoing surgical treatment of NSCLC with long-term follow-up. Tumour Biol 2014;35:4551-9. [Crossref] [PubMed]