Technologic advances in the assessment and management of obstructive sleep apnoea beyond the apnoea-hypopnoea index: a narrative review
Introduction
Obstructive sleep apnoea (OSA) is a serious and worldwide health problem that affects at least 10% of the general population (1,2), although a large percentage of patients with moderate to severe disease remain undiagnosed (3,4). OSA is highly prevalent in adults (14% in men, 5% in females), especially mild-moderate severity and is becoming more prevalent due in part to the increasing prevalence of obesity in the developed world (5,6). The global prevalence is thought to be 1 billion, with general population prevalence estimates exceeding 50% in some countries (7). This represents a challenge for diagnosis, as the current gold standard diagnostic test is overnight polysomnography (PSG) in a sleep laboratory, which is labour intensive and expensive (8).
OSA is characterised by recurrent upper airway obstruction (UAO) during sleep triggering episodes of apnoea and hypopnoea that leads to sleep fragmentation, non-restorative sleep and excessive daytime somnolence (EDS) (9). The cessation in airflow causes intermittent hypoxia (IH) (10) which is thought to be a major pathogenic mechanism for the adverse complications associated with OSA (11) including cardiovascular complications and increased mortality (12,13). Increasingly, it is recognised that various phenotypic traits are important contributors to OSA for most patients, which include a low respiratory arousal threshold, loop gain, and anatomical factors that narrow the upper airway (14). Clinical symptoms, the presence of certain biomarkers and co-morbidities are also significant variables (15).
OSA contributes to a significant health burden in society due to the strong association with cardiovascular disease [hypertension (HTN), coronary artery disease, congestive cardiac failure], stroke, metabolic syndrome and type 2 diabetes mellitus (12,16). OSA, independent of age, sex and obesity is also associated with reduced quality of life secondary to EDS (17), motor vehicle accidents (18), depression and cognitive decline (19).
Currently, disease severity is measured using the apnoea-hypopnoea index (AHI) as determined from a sleep study. However, recent evidence suggests a poor association between daytime symptoms (e.g., EDS) and the severity of OSA recorded in a sleep study (20), and there is a growing call to move away from the AHI as the principle measure of OSA severity towards a more personalised approach to OSA diagnosis and treatment (15). The global individual and socioeconomic burden of OSA, evidence for poor correlation of daytime symptoms with AHI, and limitations of AHI in terms of disease severity has led to emerging trends for the practice of personalized medicine within OSA and the development of simpler, more convenient objective methods of diagnosis. Recent reviews (15,21,22) highlight the need to move away from sole use of AHI as indicator and predictor of adverse outcome in OSA and instead consider individual risk factors, clinical history and co-morbid disease in the diagnosis and treatment of OSA. Other signals such as heart rate variability (HRV), pulse transit time (PTT), oximetry and the use of biomotion sensors may allow for an improved and phenotypic diagnosis. Home sleep apnoea testing (HSAT) and wearable technologies may help improve access to diagnosis and treatment adherence. Finally, technological advances in telemedicine may strengthen inter-departmental collaboration to improve overall care of OSA patients.
In this paper, we review the current diagnostic strategies for OSA in the context of their limitations, the role of emerging technology and potential physiological targets. We review the current evidence for using newer technologies in OSA diagnosis such as smartphone applications and wearable technology.
We present the following article/case in accordance with the Narrative Review Checklist (available at http://dx.doi.org/10.21037/jtd-sleep-2020-003).
Clinical and pathophysiologic phenotypes
OSA patients typically present with EDS, frequent awakenings, bed partner reports of frequent choking/gasping during sleep, and loud snoring (8). Patients often complain of morning headache, dry mouth and nonrestorative sleep, and may have a diagnosis of systolic HTN or co-morbid cardiovascular disease. Evidence from epidemiological studies indicate that not all patients complain of EDS or fatigue and some patients report only minimal symptoms (23). Furthermore, clinical history and symptoms are now recognised as an important phenotypic variable in the individualised approach to the diagnosis and treatment of OSA (24) with different characteristics requiring tailored treatment plans (15,21). Nonetheless, undiagnosed and untreated OSA is a significant burden on the healthcare system, with increased healthcare utilization seen in those with untreated disease (25).
More recently, Randerath et al. (15) presented conclusions from an expert review of the challenges in OSA management. The expert consensus was that a revision to the diagnostic criteria for OSA is required to include factors that reflect different clinical and pathophysiological phenotypes, and relevant comorbidities such as non-dipping nocturnal blood pressure (BP), which are not currently part of the diagnostic criteria. PSG will objectively measure AHI but fails to demonstrate an individual’s susceptibility for systemic effects of recurrent hypoxia due to OSA or the underlying pathophysiology. For instance, in older adults, airway anatomy/collapsibility plays a relatively greater pathogenetic role (26). Molecular markers or biomarkers that result from end-organ strain or damage inflicted by factors such as IH represent another variable to consider. These differences have clinical relevance and may influence treatment options (14). Going forward, management should be linked to the underlying clinical and pathophysiological phenotype and assess additional factors to the AHI such as acute systemic effects and associated relevant comorbidity (Figure 1).
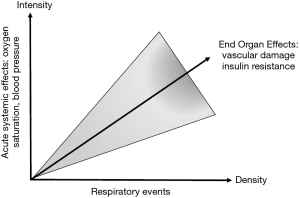
Diagnostic strategies in OSA
Traditional measures
Current guidelines describe optimum methods for testing of patients suspected of OSA (8). Patients presenting with symptoms of EDS and clinical features as highlighted above should be suspected as having OSA, particularly in the setting of risk factors such as advanced age, male gender and obesity (5,8). PSG in conjunction with a sleep-focused clinical review is the gold standard for the diagnosis of OSA and represents the reference method for the quantitative study of sleep (8). PSG is labour intensive, involving the continuous recording of electroencephalography, electrooculography, electromyography, and electrocardiography (ECG) to determine sleep stages, arousals, movement and sleep related cardiac arrhythmia, respectively. A nasal pressure sensor and a thermistor detect airflow and respiratory inductive plethysmography detect thoracic movements. Pulse oximetry is also utilised to monitor oxygen saturations and heart rate (HR). PSG is typically performed in a sleep laboratory setting with a technician in attendance. The increasing prevalence of OSA (5,27), cumbersome set up, and concern that PSG testing is not cost effective (28) has led to the emergence of alternative types of testing including portable HSAT in recent years.
HSAT
HSAT can be performed in the patient’s home, is cheaper and convenient. HSAT will record between four and seven variables that include respiratory effort, airflow, HR or ECG, arterial oxygen saturation, snoring, body position and movement. Newer devices have improved diagnostic accuracy and many devices have now been validated against PSG (29), thus providing a more convenient and cost-effective diagnostic option. Also, HSAT can record several nights of sleep, which is advantageous as night-to-night variability is a feature of OSA and tends to be most relevant in patients with mild disease (30,31).
Despite the convenience of HSAT, these devices typically underestimate the AHI, increasing the likelihood of a false negative result due to the inability of HSAT to record total sleep time (TST) and detect arousals (32,33). Fewer physiological variables are measured which can lead to misinterpretation of results and may miss coexisting sleep disorders such as insomnia, periodic limb movements and parasomnias. The American Academy of Sleep Medicine (AASM) recommends the use of HSAT for OSA diagnosis in selected populations, but advises against its use as a population screening tool (34).
However, current OSA severity thresholds based on AHI level also need to be revised, especially since recent general population studies suggest that up to 50% of the general population have significant OSA based on AHI (23). Furthermore, the disparity between AHI and symptom level evokes concerns regarding the sole assessment of OSA by PSG. Thus, a move toward multi-modality testing using novel technologies that encompass clinical, phenotypical and objective measurements is needed, employing additional markers of disease severity than the AHI.
Technological developments in sleep assessment
Emerging innovative technology allows more in-depth information to be gathered from more sophisticated algorithms and systems. Traditional PSG remains the “gold standard”, but new technologies such as novel sensors, WiFI, remote monitoring and wearable devices provide exciting potential alternatives to PSG (Figure 2). Integration of these technologies has the potential to improve patient care and allow more readable accessibility of diagnostic tests to allow quicker diagnosis and ultimately treatment of patients with OSA.
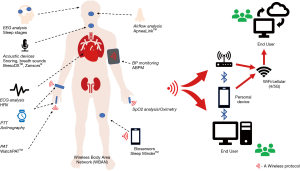
Novel approaches to evaluating oxygen saturation metrics
Overnight oximetry (ONO), typically using a pulse oximeter has long being proposed as an accessible, uncomplicated and reasonably reliable technique for OSA diagnosis, especially in severe cases (35,36). In OSA, recording of the arterial oxygen saturation (SpO2) requires a high sampling rate (>0.5 Hz) for an overnight period to ensure adequate detection of intermittent oxygen desaturations. In clinical practice, several relevant data can be obtained from the recordings, including the oxygen desaturation index (ODI) [number of desaturations per hour which drop 3% (ODI3) or 4% (ODI4) below baseline], the cumulative time with a saturation below a predetermined level, usually 90% (T90), and signal variability, which may be referred to as the delta index (8,37). In OSA, the common “saw tooth” pattern of transient oxygen desaturation is seen, especially in severe cases, which gives an instant visual picture of the disease that is unique to OSA. Simple indices fail to capture all of the important and potentially diagnostic pathophysiological characteristics (36) and novel strategies for analysis of oximetry have concentrated on the use of automation (38-40) to maximise the diagnostic potential of these data.
Application of computer-assisted quantification of the time, number and severity of desaturations aids detection of OSA (39,41). Automation of desaturation events facilitates a better description of events, to include desaturations of >4%, >5% and more, with desaturation duration and depth also calculable (42). Time series variables such as mean SpO2, variance of SpO2, minimum SpO2 and cumulative time with a saturation below a predetermined level can be measured and plotted graphically (43).
Automated signal processing plays an important role in optimizing the use of ONO in the context of OSA diagnosis. Several studies have focused on automated signal processing which look at the time/frequency dynamics of ONO (44), morphological features (45), and analysis of repetitive apnoeic/hypopnoeic events (39,42). Non-linear analysis, which quantifies information related to the temporal order of values in the oximetry signal, has also been utilized (39,42) in characterizing the changes in ONO. Several devices that encompass these technologies have been developed and most include a sensor that sends data to be examined by Bluetooth to a mobile phone. OSA detecting algorithms compute this data to diagnose OSA. Other devices use smartphones or personal digital assistant (PDA) as the receiver. Algorithm analysis and statistical analysis of time and frequency allow in depth processing to occur with encouraging potential for home testing (46-48). Wireless approaches are also available (48), which may assist in OSA diagnosis and prioritise cases for more detailed study, and may also have a role in treatment follow-up (49).
Oximetry and AHI
ODIs can be directly used as a substitute for the AHI to estimate the number of respiratory events during the night. However, ODIs (>4%) often underestimate the severity of OSA, which can have significant clinical consequences (12,13) and influences treatment decisions (8). Different approaches have been utilised to combat this limitation, including regression analysis (45,50) and machine learning algorithms (51), with average diagnostic accuracy of 96.7% reported. The diagnostic potential of ONO compared to AHI is emphasised by several reports indicating that ODI is a superior predictor of co-morbidity than AHI in OSA patients regarding co-morbid HTN (52) and diabetes mellitus (53).
Oximetry in patients with co-morbidity
Some work has been conducted on the use of portable ONO to diagnose sleep disordered breathing (SDB) in patients with co-morbidities including stoke (54), heart failure (55), morbid obesity (56,57) and COPD (58). In stroke, OSA is associated with impaired recovery and an increased risk of mortality (59). In stroke patients undergoing rehabilitation, >15 events/hour reached 77% sensitivity and 100% specificity in the prediction of an AHI >15 events/h and the authors concluded that ONO could be used as a diagnostic tool for OSA in stroke patients (54). In the setting of congestive cardiac failure, one study found that ONO achieved high sensitivity (97%) but lacked specificity (32%) making it a useful rule out test, but was not diagnostic for OSA in this population (55). In obesity, ONO performs better, with conventional oximetry alone achieving 100% sensitivity and 93% specificity when used alone at home for the diagnosis of severe OSA in patients with morbid obesity (56). ODI4 was shown to be predictive of mild, moderate and severity OSA in a further study of 475 surgical patients (57).
Both OSA and nocturnal hypoxemia are commonly seen in chronic obstructive pulmonary disease (COPD) patients (58,60) and treatment with CPAP as compared to LTOT alone is associated with higher survival rates in patients with OSA-COPD overlap (61). ONO alone in diagnosing patients with the overlap syndrome is limited by low sensitivity (59%) and specificity (60%) (58). However, oximetry when combined with novel technology was able to identify moderate-severe OSA regardless of the presence of COPD (62).
Future direction
Advances in technology and signal processing have expanded the role of ONO but controversy persists. Oximetry alone demonstrates significant variability in diagnostic accuracy, with reported sensitivity ranging from 31–98% and specificity 41–100% (38). Part of this variability may be accounted by the range in sampling frequency and amplitude resolution seen in currently available devices (39) and also without automation, variability in how physicians interpret oximetry tracing (63). Thus, further research is needed to support the use of oximetry at home as a single diagnostic test for OSA, particularly in those patients with comorbidities.
Cardiac based measures
ECG analysis: monitoring of HRV, ECG and respiration
Monitoring of HRV by overnight ECG has long been recognized as a potential diagnostic tool in patients with SDB (64). Under standard conditions, a single lead ECG is recorded during PSG to measure HR and rhythm. Use of dedicated software allows analysis of HRV (65) which can provide insight into autonomic activity and may even distinguish sleep stages (66). Additional information may be obtained from fluctuations in QRS amplitude relating to rib cage movement during respirations. The combination of ECG-derived respiration and sleep apnoea related HRV has the potential to be a reliable predictor of OSA (67) and has potential for screening in the cardiologist’s office prior to referral.
PTT
The PTT is derived from the ECG and the photoplethysmographic arterial pressure wave measured by a finger probe, which has been shown to reflect inspiratory effort (68). PTT measures the time required for the arterial pulse wave to travel between two points in the arterial tree: from the moment when the pulse leaves the aortic valve (R-wave on ECG) to the time when it reaches the vessels in the finger as identified by pulse oximetry. Pulse wave speed depends on the vessel stiffness, which is influenced by BP levels. PTT fluctuates with changes in arterial wall stiffness and has the potential to identify apnoea and arousals (68).
Peripheral arterial tonometry (PAT) (the pulse wave as a measure)
PAT allows a closer look at the pulse wave and is one of the more robust diagnostic tools available. Amplitude of the wave is modulated by sympathetic tone, and arousals can be estimated by drops in pulse amplitude. Sleep stages may also be estimated by pulse rate analysis (69). The WatchPATTM device (Itamar Medical, Ltd) uses a proprietary algorithm that combines PAT, oximetry, HR monitoring, and actigraphy to provide an estimate of TST and to calculate a respiratory disturbance index (70). It has been validated in a number of studies (69-71) and the overall agreement between sleep indexes calculated by in-lab PSG and those calculated using PAT devices have a reasonable correlation (r=0.889) (72), making it an attractive and reasonably reliable diagnostic tool for OSA.
BP monitoring as a potential diagnostic tool
OSA is a known independent risk factor for daytime HTN with apnoeic episodes during sleep also recognized to result in acute BP elevation (5,73). Meta-analysis suggests that OSA increases the likelihood of non-dipping blood pressure (NDBP) by approximately 1.5 times (74), suggesting ambulatory BP monitoring may serve as a surrogate marker of OSA, An increased frequency of reverse systolic dippers (73.5%), which is also independently associated with OSA is also seen (75). A recent report from this department has indicated a high predictive value for moderate to severe OSA in unselected patients recruited from a HTN clinic who demonstrated a non-dipping pattern of nocturnal BP (76).
However, standard ambulatory BP measurement with a pneumatic cuff may cause arousals during sleep and cannot track the rapid BP changes that are observed in OSA (77,78). Continuous BP measurement can be conducted by finger photoplethysmography, whereby a miniature cuff fits on the finger and provides a continuous signal. This provides beat-by-neat pressure curves, allowing assessment of BP variability which can be increased in OSA (79). A novel smart-watch, CareUpTM (Farasha Labs, Paris, France) has been developed for estimating BP in real time (80). This watch has been validated in 44 subjects, representing a promising tool for home monitoring of BP and may serve as a screening tool to identify patients with OSA, whilst also identifying and monitoring BP variability which could help improve the management of OSA and reduce cardiovascular risk in these patients (77). Cardiac-based signals potentially useful in the diagnosis and monitoring of OSA are summarised in Table 1.
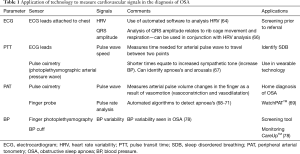
Full table
Biomarkers in OSA and associated comorbidities
Markers of inflammation or oxidative stress, metabolic markers, novel exhaled breath analysis have potential as novel biomarkers in OSA. Chronic hypoxic stress may increase circulating levels of biochemical mediators of inflammation such as C-reactive protein (CRP), tumour necrosis factor-α (TNF-α), interleukin-6 (IL-6), and interleukin-8 (IL-8) which could have potential as markers of disease severity and likelihood of associated comorbidity (81,82). However, no studies have identified an ideal biomarker. Furthermore, certain co-morbidities such as non-dipping nocturnal BP have potential as a biomarker in OSA (76).
Exhaled breath analysis is an innovative and non-invasive approach that allows collection of a wide range of substances that provide information on pathophysiological and metabolic processes in a wide range of disorders. In sleep disorders, exhaled breath analysis has allowed insight in to the metabolomics of OSA and its consequences. Most studies focus on markers of inflammation and oxidative stress (IL-6, TNF-α) which can correlate with measures of OSA severity. Further research into this area may facilitate earlier diagnosis and assist therapeutic monitoring (83).
Acoustic and airflow devices
Acoustic devices
Despite snoring being a common finding in OSA, on its own, snoring is probably of limited value in the assessment of OSA, due to its weak relationship with AHI (84). However, breath sounds are capable of being recorded and may offer an alternative home measure of AHI. BresoDXTM (BresoTec Inc., Toronto, Ontario, Canada) is a portable device consisting of an open lightweight face frame, embedded electronic module and a microphone. Breath sounds are stored continuously for up to 8 hours and can be transferred to a central server for acoustic analysis (85,86). In this context, an intermittent pattern of snoring is likely to be the most useful predictive pattern for OSA. AHI determination using BresoDXTM in one study of 135 subjects showed good correlation to PSG with a diagnostic accuracy ranging between 88.9% and 93.3% at AHI cut-offs of 5–15 (87).
More recently Zansors® has developed a small, wireless wearable patch that measures sleep breathing patterns using an inbuilt microphone and an accelerometer to record movement. In a pilot study the device demonstrated 75% sensitivity and 71% specificity for detecting sleep apnoea events compared to gold standard PSG. Further results are awaited, but highlights the potential for an acoustic sensor to have improved diagnostic performance when used with other signals (88).
Airflow devices
Home single-channel nasal pressure (HNP) devices such as ApneaLinkTM (Resmed, Sydney, Australia) (89) have been proposed as a cheaper alternative to PSG for OSA diagnosis. ApneaLinkTM is a portable, battery powered, single-channel nasal pressure device that measures airflow through a nasal cannula. Sophisticated algorithms calculate the AHI (90). When compared to PSG, home ApneaLinkTM recordings have a 73.1% sensitivity and 91% specificity for detecting an AHI >15 in at risk populations (89), with similar results reported in other studies (90,91). These results suggest a promising role for devices that measure one or two signals only, with appropriate pre-selection of patients, but further study is needed to fully validate their role.
Actigraphy
Immobility is a predictable and distinctive feature of sleep compared to wakefulness. Actigraphy involves the collection of data representing body movement (often an accelerometer built into a wristwatch) over time. Actigraphs can display daily sleep-wake cycles, which may be useful in the diagnosis and evaluation of several clinical sleep disorders. In 1995, the AASM concluded that actigraphy was useful as a research tool in sleep (92) and since, advancements in technology have allowed for cheaper and smaller devices that can unobtrusively measure sleep in large-scale, real life settings (see section “Use of technology to improve adherence in OSA”). In 2007, actigraphy was accepted by the American Academy of Sleep Medicine (AASM) as an alternative to PSG to allow prolonged monitoring of sleep (93).
Previous reviews (94-96) have noted that actigraphy can provide useful information about sleep in the natural sleep environment, measures of basic sleep indices and/or inform when extended monitoring is clinically indicated. However, actigraphy is not able to accurately detect sleep stage and has low specificity (97). When compared to PSG, actigraphy tends to overestimate TST and underestimate wake time (98). Actigraphy is limited by an indirect signal, namely motion, and estimations of sleep patterns are only inferential. However, with technological improvements, the role of actigraphy is likely to evolve as a screening tool and become part of more complex systems to evaluate OSA.
Wireless systems: biosensors in OSA
Sleep can be monitored wirelessly. The first device was constructed in Finland in the 1980s using pressure sensitive foils in the bed of the sleeping patient, which recorded sleep, HR and respiration (99). These have evolved substantially with the introduction of digital technology for signal processing and signal acquisition, and by the inclusion of oximetry.
Radio frequency waves, similar to radar technology, can detect very small body movements, as caused by respiration and heartbeat, even through the bed clothes of a sleeping patient, thus allowing the clinician to distinguish sleep and wakefulness, sleep apnoea and normal breathing (100,101). SleepMinder™ (ResMed Sensor Technologies, Dublin, Ireland) is a device that estimates the severity of OSA using a novel method of interpreting the breathing pattern of subjects during sleep (102). The device is non-contact, portable and easy to use. It is based on a multi-channel biomotion sensor and integrated analysis software. The biomotion sensor uses an ultralow power radiofrequency transceiver to measure the biomotion due to breathing and body movement of the subject in bed. Algorithms are used to perform signal analysis, including respiration analysis, sleep quality measurement and sleep apnoea assessment. The device has been shown to correlate well with PSG in the determination of AHI (103) and sleep efficiency (SE) (104) during controlled laboratory settings. More recently, the device was shown to be effective as a screening tool in high risk patients (but demonstrated lower accuracy in mild OSA (105). The device also allowed monitoring over seven nights, which may provide further information on the patient’s disorder. Further validation studies are still needed on various populations, but it is a promising and simple home test.
Smartphone technology
PSG, although well studied and the gold standard in diagnosis of OSA, is not a set up that is well suited to ambulatory assessment. In contrast, smartphones are widely available and potentially can track sleep and sleep behaviour in a non-invasive manner. Most smartphones have embedded sensors (Figure 3) that can facilitate data acquisition (e.g., accelerometer, microphone), offering novel opportunities to passively monitor patients in their natural environments (106). The majority boast a wide array of computational power which can be fed to a cloud computing database and sophisticated algorithms employed to analyse and score sleep data (107).
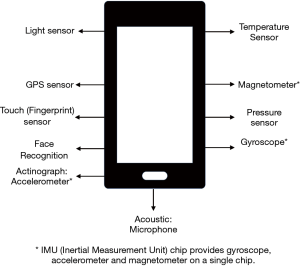
The concepts underpinning actigraphy are important as most smartphones rely on sleep assessment methods that involve movement detection, in addition to audio and video recording in some applications. The inbuilt accelerometer act as a modern actigraph to distinguish wake and sleep from the movement detected by the phone’s embedded sensors. More novel devices utilise sound recordings (e.g., snoring) to aid detection of SDB. More recently, advances have allowed sensors devices (HR recording, breathing frequency) to be connected to the smartphone device permitting the assessment of more biological signals (106). Given the wide availability, relatively low cost and ease of use, smartphones are considered by many as the best strategy for monitoring sleep outside the traditional PSG set up (108,109), and may have potential as screening tools for SDB (Table 2).
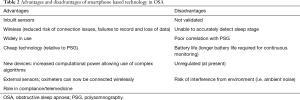
Full table
Applications or “apps” in sleep medicine
Smartphones allow consumers to download third-party applications (apps) through an online mobile store. Mobile apps are the most popular form of consumer technology, running on smart phones or tablets and are common in the area of sleep and sleep hygiene. They can analyse data online and provide real-time feedback to the consumer, functioning through the support of in built sensors (110). The apps monitor sleep tracking, facilitate sleep and dream logging, and may have alarm functions. They allow sleep analysis and are increasing in number (111). Sound recording can also be added to detect snoring (112).
Consumers often use these “apps” to improve or self-monitor sleep. However, robust evidence for their use is lacking (108,113,114) and there is a paucity of clinical validation with traditional sleep technologies (PSG, HSAT, actigraphy) (107,115,116). A review of 51 such “apps” in 2016 (117) found inconsistencies and lack of any scientific publications to back up the accuracy of the apps. Every app reported sleep duration but reporting data on sleep structure (time in light sleep, deep sleep, REM) was unreliable. App’s were reliant on inbuilt accelerometers (actigraphy), but each app had its own proprietary algorithm to relate the amount of movement detected to specific sleep stages.
Nonetheless, sleep tracking products remain very popular consumer products and are widely used for sleep tracking (117). One of the top 5 paid apps in 2014 on iTunes was a sleep tracker and alarm clock (106). Data from these apps are currently not regulated for use as medical devices and are used without prescription or clinical guidance. To date, the accuracy of these data when used as healthcare related data remains to be determined (108).
Smartphone vs. PSG
Few studies have compared sleep apps with PSG and most relate to sleep tracking measures. In one study of the sleep app, Sleep TimeTM (Azumio, Inc., Palo Alto, CA, USA), no correlation was found with PSG regarding sleep efficiency, light sleep percentage, deep sleep percentage, or sleep latency (SL) (115). Furthermore, the app had poor specificity, similar to previous reports (96,118). Similar poor correlations with PSG are reported for the apps Sleep CycleTM (Northcube, Goteborg, Sweden) (119), and Motion X 24/7TM (Fullpower technologies, Inc. Santa Cruz, CA, USA) (116), and are not recommended as a diagnostic tool.
A more recent study (120) compared four smartphone apps (Sleep Cycle-AccelerometerTM (Northcube, Goteburg, Sweden), Sleep Cycle-MicrophoneTM (Northcube, Goteburg, Sweden), SenseTM (Hello, San Francisco, USA), Smart AlarmTM (Plus Sports, USA) with PSG. A combination of sound and movement sensors, either in built or external were used for sleep-wake detection and determining sleep stages. In keeping with previous studies (115,116,119) and a recent systematic review (114), the apps compared poorly to PSG in determining sleep stage and at present are not suitable alternatives to PSG in the diagnosis of SDB. The authors concluded that further validation studies involving accelerometer-based apps are needed.
In contrast, Tal et al. (121) evaluated a contact free monitoring system, EarlySenseTM (Ltd., Israel) which consists of an under the mattress piezoelectric sensor and a smartphone app, to identify sleep stages in 43 adults compared to in-laboratory PSG. An overall sleep accuracy of 88.5% was found. EarlySenseTM also detected snoring and integrated data from multiple signals including HR, RR, and motion detection, suggesting that multiple signals are required to accurately distinguish sleep stages.
Thus, clinicians need to be aware of the limitations of actigraphy as outlined previously, and especially its limitations when used within Smartphone Apps. More research into the measurement validity of these apps and development of enhanced and more sophisticated sleep algorithms are needed, which may in the future improve the diagnostic utility and reliability of these applications. Examples of smartphone apps that may have a role in screening for sleep disorders and SDB are given in Table 3.
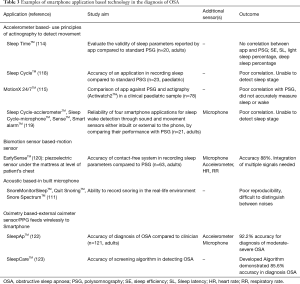
Full table
Snoring and SDB via smartphone apps
Detection of snoring
Recording sounds during sleep allows physicians to potentially screen patients who are unaware of snoring, which is a risk factor for cardiovascular disease and possibly points to OSA (124). Nakano et al. (125) used a smartphone to monitor and quantify snoring and OSA severity using a built-in microphone and analysed information obtained using a signal processing procedure like that developed for tracheal sound monitoring to detect OSA. Snoring time correlated well with PSG findings. The diagnostic sensitivity and specificity for diagnosing OSA (AHI ≥15) was 0.70 and 0.94, respectively, within laboratory settings.
However, Stippig et al. (112) looked at the accuracy of three available apps; SnoreMonitorSleepLabTM (Adactive AB, Sweden), Quit SnoringTM (Pointer Software Systems, Ltd. CA, USA) and Snore SpectrumTM (Zurlin Technologies, Melbourne, Australia) to determine whether this technology could be used in a noisy home environment. The apps demonstrated difficulty distinguishing between snoring sounds and other noises in the bedroom or close by, with both environmental and device variability noted. The authors concluded that the audio recordings were good, but ability to analyse and draw conclusions from the data was limited due to poor reproducibility and poor reliability and cannot replace current screening devices.
Snoring and SDB
The addition of oximetry should enable better diagnostic utility of smartphones for the diagnosis of OSA and systems have been designed to fit this purpose (126). However, as shown previously, continuous monitoring of oximetry is required and such systems require increased processing power, longer battery life and more detailed algorithms. Al-Mardini et al. (122) combined an external oximetry sensor with an in-built accelerometer to detect movement and a microphone to detect respiratory effort with a smartphone application to detect OSA in adults. Testing on a small group (15) demonstrated 100% sensitivity and 85.7% specificity in classifying OSA when compared to PSG, while severity of disease was accurately diagnosed in 87.5%. The authors designed an OSA detection technique that utilised the built-in sensors in the smartphone. The detection platform extracted and analysed physiological signals (SpO2, movement, respiratory effort) using the smartphones computational power and in-built algorithms.
SleepApTM was developed for the purpose of screening and monitoring OSA (123). Internal phone sensors and an external pulse oximeter are used to record audio, body position, activity and oxygen saturation during sleep. The clinically validated STOP-BANG questionnaire was also used (127). Specific algorithms used by the app are based on signal processing and machine learning algorithms developed from a larger database. The app classifies the user as either: Non OSA or OSA (mild, moderate, severe) and performed well compared to clinicians with an accuracy of up to 92.2% for those with moderate-severe OSA. Less robust data exists for those with mild OSA, where the app performs less well. Similarly, Nakano et al. (125) showed high correlation between smartphone and PSG in terms of total snore time (r=0.93) and AHI (r=0.94) after developing a specific algorithm using a test population of 10 patients.
Daly et al. (128) tested SleepCareTM (Sleepcare Technologies Inc., Canada): which comprised a smartphone app, a pulse oximeter, and an online server. The screening algorithms demonstrated 85.6% accuracy in a test database. Strengthened algorithms and use of more than one sensor led to improved detection of OSA and suggests that in order to produce a robust and reliable app, combining more than one sensor and use of objective and subjective sleep assessment maybe a more reliable screening tool. Further work is still needed to validate these systems in the real-world setting.
Wearable technology
Smart watches, fitness trackers and photoplethysmography
Wearable health monitoring technologies such as smart watches and fitness trackers have recently gained popularity (129). These devices are novel in that they are worn on the wrist and can pick up physiological signals directly without additional sensors being attached. The market is growing. In 2016 it was valued at over $13.2 billion and is forecast to reach $34 billion by the end of 2020. Despite the popularity of these device, only limited data are available on how these devices compare to each other and how valid and reliable they are when compared to traditional diagnostic methods (106,130).
The most common sensor technology employed in wearable devices is motion sensing via accelerometery, similar to actigraphy (95). Along with built-in accelerometers, cardiac monitoring is increasingly being used as an additional sensor, through optical and photoplethysmography methods (130). Light sensors on the device record pulse wave signal, with sophisticated algorithms capable of extracting HR and SpO2 data making these devices ideal for sleep monitoring. However, to date the data suggests these devices have limited accuracy and reliability.
Wearable devices vs. PSG
Seventeen studies have examined the accuracy of consumer-targeted wrist-worn or arm-band accelerometers that include FitBitTM (Fitbit Inc, CA, USA), JawboneTM (Jawbone Inc, CA, USA), and SensewearTM (Bodymedia, USA). An early study comparing FitbitTM with PSG demonstrated that the device overestimated TST by 63 minutes compared to PSG and by 25 minutes compared to actigraphy (131). The more recently developed Fitbit FlexTM (Fitbit, CA, USA) demonstrated more favourable results with an overestimation of sleep duration by 6.5 minutes in good sleepers and by 32.9 minutes in insomniac patients (132). This better performance in good sleepers is expected, given sleep research consistently shows that wrist accelerometry has a high sensitivity for sleep (~90%; i.e., a true sleep epoch is recorded as sleep), while having much lower specificity for sleep (~50%) (130).
Mantua et al. (133) compared four devices [Basis Health TrackerTM (Intel Corp, CA, USA), Misfit ShineTM (Misfit Wearables, CA, USA), Fitbit FlexTM (Fitbit Inc, CA, USA) and Withings Pulse O2TM (Withings, Issy-les-Moulineaux, France)] to Actiwatch SpectrumTM (Philips Respironics, OR, USA) and PSG in 40 patients. Their analysis found that the only parameter that was reliably measured for all devices was time to sleep. Another study compared nine devices for accuracy in a 24-h activity measurement. Error rates ranging from 8–16.92% were reported when compared with EEG based sleep duration (134), indicating none were capable of accurately capturing activity data across the entire day.
Combined use of photoplethysmographic methods (HR, SpO2) may improve diagnostic capabilities and determinants of sleep stage. However, the FitBit Charge 2TM (Fitbit Inc. CA, USA) underestimated sleep stage transition dynamics compared with PSG, although performed better in estimating TST and sleep efficiency (135,136). A recent meta- analysis of 22 reports evaluating FitBitTM compared to PSG confirmed that FitbitTM models overestimate TST and SE (137). Predictably, newer devices that analyze more physiological signals (HR, body movement) performed better but sleep-staging devices were limited by low specificity (137).
Reliability of HR measurement
All wrist worn activity trackers rely on photoplethysmography and use proprietary HR-derived algorithms. Several recent studies investigated the accuracy of wearable devices for measuring HR. Benedetto et al. (138) reported that the Fitbit Charge 2TM (Fitbit Inc, CA, USA) underestimates the HR by up to 30 bpm. Another report (139) evaluated the HR accuracy of Apple Watch 3TM (Apple Inc, CA, USA) and Fitbit Charge 2TM compared with ambulatory ECG and reported acceptable HR accuracy (<±10%). However, both slightly underestimated HR and measurements were influenced by wrist movement and higher HR.
Wearable devices vs. actigraphy
The accuracy of three consumer wearable devices [Fitbit FlexTM, Jawbone Up24TM (Jawbone, CA, USA), Misfit ShineTM] was compared to the well validated actigraphy device, Actiwatch-2TM (Philips Respironics, OR, USA). Users wore all devices on the same wrist for seven consecutive 24-hour periods. Overall, both devices were comparable to actigraphy in establishing TST and wake after sleep onset (WASO). Kanady et al. (140) compared a consumer grade wearable sleep monitor (Basis B1TM (Basis Science Inc. CA, USA) in the assessment of sleep patterns in normal adults to PSG and research grade actigraphy. The device performed similarly to actigraphy for estimation of TST, but was not accurate for sleep stages and was not a substitute for PSG.
Role in detection of OSA
Wearable sleep trackers are not reliable in the diagnosis of OSA. The ability of two wrist worn sleep trackers; Withings pulse 02TM and Jawbone UpTM and one actigraph (Bodymedia SenseWear Pro ArmbandTM) to measure sleep architecture and sleep quantity in patients diagnosed with OSA assessed during one polysomnographic recording night in a general sleep laboratory was evaluated in 32 patients (141). Both sleep trackers were unable to accurately measure SE, SL or awakenings, could not estimate deep sleep properly and demonstrated poor intra-class correlation, confirming the limited performance of wearable devices in the evaluation of sleep in OSA patients.
Use of technology to improve adherence in OSA
Despite the technological advancements in CPAP devices, including improvements in comfort (humidifiers, pressure ramp features) and more discreet machines over the last 20–30 years, adherence to therapy has not significantly improved and remains a significant stumbling block in the management of OSA patients (142). This data was provided via a systematic review of clinical trials evaluating adherence, but may not be fully reflective of real world practice and in general adherence rates fluctuate widely (50–80%). Traditional intensive support strategies involve regular nursing visits and detailed education, which is time consuming, costly, labour intensive and produces variable results (143).
However, CPAP devices already objectively record usage data and now with emergence of cloud-based technologies, a unique way of analysing adherence in patients with OSA, in a real-world setting exists. Cistulli et al. (144) evaluated 2.62 million patients, using real world data with an observed 90-day adherence rate of 75%, higher than previously reported (142). Still, we can and should do better and new technology also affords development of patient engagement strategies to improve compliance.
Patients may now engage in their own care. Most CPAP machines can display previous nights’ usage, and this can facilitate direct feedback to the patient in real time. Cloud-based platforms, similarly, can be used to update physicians on patient’s adherence and allow remote monitoring, facilitating better care and be cost efficient (145). Cloud-based technology can also be developed to provide real time feedback to the patient. Malhotra et al. (146) compared CPAP adherence among patients who were provided with active patient engagement (APE) technology versus those who received usual care monitoring (UCM). APE was associated with improved adherence (87.3%) compared with UCM patients (70.4%; P<0.001).
Smartphone applications may also improve patient adherence to CPAP. SleepWell24TM (ASU, Arizona, USA) is a multicomponent evidence-based smartphone app that is designed to deliver biofeedback about CPAP use and sleep patterns by combining cloud-based CPAP data and wearable sensor data. It remains to be seen whether this app will be effective in improving adherence and will be evaluated for efficacy compared to UCM in an upcoming RCT (147).
Conclusions
The growing recognition that AHI represents a poor metric to assess the presence of a clinically significant OSA syndrome, together with the move towards ambulatory monitoring of patients for diagnosis and treatment has resulted in an increasing desire to identify biological variables that identify patients with a clinically significant disorder. It is likely that smartphone and wearable technologies will play a significant role in these developments, but currently available technologies have not yet reached that goal. Other strategies such as biomarkers will also likely play a role, especially in the identification of relevant comorbidity. Combinations of signals that can be non-invasively measured in the ambulatory setting are most likely to succeed in replacing conventional testing such as PSG for most patients.
Acknowledgments
Funding: None.
Footnote
Provenance and Peer Review: This article was commissioned by the Guest Editor (Walter McNicholas) for the series “Sleep Section” published in Journal of Thoracic Disease. The article was sent for external peer review organized by the editorial office.
Reporting Checklist: The authors have completed the Narrative Review Checklist. Available at http://dx.doi.org/10.21037/jtd-sleep-2020-003
Conflicts of Interest: All authors have completed the ICMJE uniform disclosure form (Available at http://dx.doi.org/10.21037/jtd-sleep-2020-003). JFG reports that he has been a member of the European medical advisory board for BresoTec. WTM McNicholas serves as an unpaid Section Director of Sleep Section and an unpaid editorial board member of Journal of Thoracic Disease. The authors have no other conflicts of interest to declare.
Ethical Statement: The authors are accountable for all aspects of the work in ensuring that questions related to the accuracy or integrity of any part of the work are appropriately investigated and resolved.
Open Access Statement: This is an Open Access article distributed in accordance with the Creative Commons Attribution-NonCommercial-NoDerivs 4.0 International License (CC BY-NC-ND 4.0), which permits the non-commercial replication and distribution of the article with the strict proviso that no changes or edits are made and the original work is properly cited (including links to both the formal publication through the relevant DOI and the license). See: https://creativecommons.org/licenses/by-nc-nd/4.0/.
References
- Young T, Peppard PE, Gottlieb DJ. Epidemiology of obstructive sleep apnea: a population health perspective. Am J Respir Crit Care Med 2002;165:1217-39. [Crossref] [PubMed]
- Lévy P, Kohler M, McNicholas WT, et al. Obstructive sleep apnoea syndrome. Nat Rev Dis Primers 2015;1:15015. [Crossref] [PubMed]
- Kapur V, Strohl KP, Redline S, et al. Underdiagnosis of sleep apnea syndrome in U.S. communities. Sleep Breath 2002;6:49-54. [Crossref] [PubMed]
- Young T, Skatrud J, Peppard PE. Risk factors for obstructive sleep apnea in adults. JAMA 2004;291:2013-6. [Crossref] [PubMed]
- Peppard PE, Young T, Barnet JH, et al. Increased prevalence of sleep-disordered breathing in adults. Am J Epidemiol 2013;177:1006-14. [Crossref] [PubMed]
- Franklin KA, Lindberg E. Obstructive sleep apnea is a common disorder in the population-a review on the epidemiology of sleep apnea. J Thorac Dis 2015;7:1311-22. [PubMed]
- Benjafield AV, Ayas NT, Eastwood PR, et al. Estimation of the global prevalence and burden of obstructive sleep apnoea: a literature-based analysis. Lancet Respir Med 2019;7:687-98. [Crossref] [PubMed]
- Kapur VK, Auckley DH, Chowdhuri S, et al. Clinical Practice Guideline for Diagnostic Testing for Adult Obstructive Sleep Apnea: An American Academy of Sleep Medicine Clinical Practice Guideline. J Clin Sleep Med 2017;13:479-504. [Crossref] [PubMed]
- Malhotra A, White DP. Obstructive sleep apnoea. Lancet 2002;360:237-45. [Crossref] [PubMed]
- Dempsey JA, Veasey SC, Morgan BJ, et al. Pathophysiology of sleep apnea. Physiol Rev 2010;90:47-112. [Crossref] [PubMed]
- Dewan NA, Nieto FJ, Somers VK. Intermittent hypoxemia and OSA: implications for comorbidities. Chest 2015;147:266-74. [Crossref] [PubMed]
- McNicholas WT, Bonsigore MR. Sleep apnoea as an independent risk factor for cardiovascular disease: current evidence, basic mechanisms and research priorities. Eur Respir J 2007;29:156-78. [Crossref] [PubMed]
- Lam JC, Mak JC, Ip MS. Obesity, obstructive sleep apnoea and metabolic syndrome. Respirology 2012;17:223-36. [Crossref] [PubMed]
- Carberry JC, Amatoury J, Eckert DJ. Personalized Management Approach for OSA. Chest 2018;153:744-55. [Crossref] [PubMed]
- Randerath W, Bassetti CL, Bonsignore MR, et al. Challenges and perspectives in obstructive sleep apnoea: Report by an ad hoc working group of the Sleep Disordered Breathing Group of the European Respiratory Society and the European Sleep Research Society. Eur Respir J 2018;52:1702616. [Crossref] [PubMed]
- Kent BD, McNicholas WT, Ryan S. Insulin resistance, glucose intolerance and diabetes mellitus in obstructive sleep apnoea. J Thorac Dis 2015;7:1343-57. [PubMed]
- Vinnikov D, Blanc PD, Alilin A, et al. Fatigue and sleepiness determine respiratory quality of life among veterans evaluated for sleep apnea. Health Qual Life Outcomes 2017;15:48. [Crossref] [PubMed]
- Bioulac S, Micoulaud-Franchi JA, Arnaud M, et al. Risk of Motor Vehicle Accidents Related to Sleepiness at the Wheel: A Systematic Review and Meta-Analysis. Sleep 2017.40. [PubMed]
- Kerner NA, Roose SP. Obstructive Sleep Apnea is Linked to Depression and Cognitive Impairment: Evidence and Potential Mechanisms. Am J Geriatr Psychiatry 2016;24:496-508. [Crossref] [PubMed]
- Deegan PC, McNicholas WT. Predictive value of clinical features for the obstructive sleep apnoea syndrome. Eur Respir J 1996;9:117-24. [Crossref] [PubMed]
- Hilbert J, Yaggi HK. Patient-centered care in obstructive sleep apnea: A vision for the future. Sleep Med Rev 2018;37:138-47. [Crossref] [PubMed]
- Sânchez-de-la-Torre M, Gozal D. Obstructive sleep apnea: in search of precision. Expert Rev Precis Med Drug Dev 2017;2:217-28. [Crossref] [PubMed]
- Heinzer R, Vat S, Marques-Vidal P, et al. Prevalence of sleep-disordered breathing in the general population: the HypnoLaus study. Lancet Respir Med 2015;3:310-8. [Crossref] [PubMed]
- Gagnadoux F, Le Vaillant M, Paris A, et al. Relationship Between OSA Clinical Phenotypes and CPAP Treatment Outcomes. Chest 2016;149:288-90. [Crossref] [PubMed]
- Kapur V, Blough DK, Sandblom RE, et al. The medical cost of undiagnosed sleep apnea. Sleep 1999;22:749-55. [Crossref] [PubMed]
- Edwards BA, Wellman A, Sands SA, et al. Obstructive sleep apnea in older adults is a distinctly different physiological phenotype. Sleep 2014;37:1227-36. [Crossref] [PubMed]
- Young T, Palta M, Dempsey J, et al. The occurrence of sleep-disordered breathing among middle-aged adults. N Engl J Med 1993;328:1230-5. [Crossref] [PubMed]
- Walsleben JA, Kapur VK, Newman AB, et al. Sleep and reported daytime sleepiness in normal subjects: the Sleep Heart Health Study. Sleep 2004;27:293-8. [Crossref] [PubMed]
- Collop NA, Tracy SL, Kapur V, et al. Obstructive sleep apnea devices for out-of-center (OOC) testing: technology evaluation. J Clin Sleep Med 2011;7:531-48. [Crossref] [PubMed]
- Bittencourt LR, Suchecki D, Tufik S, et al. The variability of the apnoea-hypopnoea index. J Sleep Res 2001;10:245-51. [Crossref] [PubMed]
- Ahmadi N, Shapiro GK, Chung SA, et al. Clinical diagnosis of sleep apnea based on single night of polysomnography vs. two nights of polysomnography. Sleep Breath 2009;13:221-6. [Crossref] [PubMed]
- Zeidler MR, Santiago V, Dzierzewski JM, et al. Predictors of Obstructive Sleep Apnea on Polysomnography after a Technically Inadequate or Normal Home Sleep Test. J Clin Sleep Med 2015;11:1313-8. [Crossref] [PubMed]
- Escourrou P, Grote L, Penzel T, et al. The diagnostic method has a strong influence on classification of obstructive sleep apnea. J Sleep Res 2015;24:730-8. [Crossref] [PubMed]
- Collop NA, Anderson WM, Boehlecke B, et al. Clinical guidelines for the use of unattended portable monitors in the diagnosis of obstructive sleep apnea in adult patients. Portable Monitoring Task Force of the American Academy of Sleep Medicine. J Clin Sleep Med 2007;3:737-47. [Crossref] [PubMed]
- McNicholas WT. Diagnosis of Obstructive Sleep Apnea in Adults. Proc Am Thorac Soc 2008;5:154-60. [Crossref] [PubMed]
- Netzer N, Eliasson AH, Netzer C, et al. Overnight pulse oximetry for sleep-disordered breathing in adults: a review. Chest 2001;120:625-33. [Crossref] [PubMed]
- Berry RB, Budhiraja R, Gottlieb DJ, et al. Rules for scoring respiratory events in sleep: update of the 2007 AASM Manual for the Scoring of Sleep and Associated Events. Deliberations of the Sleep Apnea Definitions Task Force of the American Academy of Sleep Medicine. J Clin Sleep Med 2012;8:597-619. [Crossref] [PubMed]
- Uddin MB, Chow CM, Su SW. Classification methods to detect sleep apnea in adults based on respiratory and oximetry signals: a systematic review. Physiol Meas 2018;39:03tr1.
- Del Campo F, Crespo A, Cerezo-Hernandez A, et al. Oximetry use in obstructive sleep apnea. Expert Rev Respir Med 2018;12:665-81. [Crossref] [PubMed]
- Mendonca F, Mostafa SS, Ravelo-Garcia AG, et al. A Review of Obstructive Sleep Apnea Detection Approaches. IEEE J Biomed Health Inform 2019;23:825-37. [Crossref] [PubMed]
- Mendonça F, Mostafa SS, Ravelo-Garcia AG, et al. Devices for home detection of obstructive sleep apnea: A review. Sleep Med Rev 2018;41:149-60. [Crossref] [PubMed]
- Terrill PI. A review of approaches for analysing obstructive sleep apnoea-related patterns in pulse oximetry data. Respirology 2020;25:475-85. [Crossref] [PubMed]
- Deviaene M, Testelmans D, Buyse B, et al. Automatic Screening of Sleep Apnea Patients Based on the SpO2 Signal. IEEE J Biomed Health Inform 2019;23:607-17. [Crossref] [PubMed]
- Álvarez D, Hornero R, Marcos JV, et al. Feature selection from nocturnal oximetry using genetic algorithms to assist in obstructive sleep apnoea diagnosis. Med Eng Phys 2012;34:1049-57. [Crossref] [PubMed]
- Jung DW, Hwang SH, Cho JG, et al. Real-Time Automatic Apneic Event Detection Using Nocturnal Pulse Oximetry. IEEE Trans Biomed Eng 2018;65:706-12. [Crossref] [PubMed]
- Burgos A, Goni A, Illarramendi A, et al. Real-time detection of apneas on a PDA. IEEE Trans Inf Technol Biomed 2010;14:995-1002. [Crossref] [PubMed]
- Zhang J, Zhang Q, Wang Y, et al. A real-time auto-adjustable smart pillow system for sleep apnea detection and treatment. Proceedings of the 12th international conference on Information processing in sensor networks; Philadelphia, Pennsylvania, USA: Association for Computing Machinery; 2013:179-90.
- Angius G, Raffo L. A sleep apnoea keeper in a wearable device for Continuous detection and screening during daily life. 2008 Computers in Cardiology 2008;2008:14-17.
- Mendonça F, Mostafa SS, Morgado-Dias F, et al. An Oximetry Based Wireless Device for Sleep Apnea Detection. Sensors (Basel) 2020;20:888. [Crossref] [PubMed]
- Ebben MR, Krieger AC. Diagnostic accuracy of a mathematical model to predict apnea-hypopnea index using nighttime pulse oximetry. J Biomed Opt 2016;21:35006. [Crossref] [PubMed]
- Marcos JV, Hornero R, Alvarez D, et al. Automated prediction of the apnea-hypopnea index from nocturnal oximetry recordings. IEEE Trans Biomed Eng 2012;59:141-9. [Crossref] [PubMed]
- Tkacova R, McNicholas WT, Javorsky M, et al. Nocturnal intermittent hypoxia predicts prevalent hypertension in the European Sleep Apnoea Database cohort study. Eur Respir J 2014;44:931-41. [Crossref] [PubMed]
- Kent BD, Grote L, Bonsignore MR, et al. Sleep apnoea severity independently predicts glycaemic health in nondiabetic subjects: the ESADA study. Eur Respir J 2014;44:130-9. [Crossref] [PubMed]
- Aaronson JA, van Bezeij T, van den Aardweg JG, et al. Diagnostic accuracy of nocturnal oximetry for detection of sleep apnea syndrome in stroke rehabilitation. Stroke 2012;43:2491-3. [Crossref] [PubMed]
- Ward NR, Cowie MR, Rosen SD, et al. Utility of overnight pulse oximetry and heart rate variability analysis to screen for sleep-disordered breathing in chronic heart failure. Thorax 2012;67:1000-5. [Crossref] [PubMed]
- Malbois M, Giusti V, Suter M, et al. Oximetry alone versus portable polygraphy for sleep apnea screening before bariatric surgery. Obes Surg 2010;20:326-31. [Crossref] [PubMed]
- Chung F, Liao P, Elsaid H, et al. Oxygen desaturation index from nocturnal oximetry: a sensitive and specific tool to detect sleep-disordered breathing in surgical patients. Anesth Analg 2012;114:993-1000. [Crossref] [PubMed]
- Scott AS, Baltzan MA, Wolkove N. Examination of pulse oximetry tracings to detect obstructive sleep apnea in patients with advanced chronic obstructive pulmonary disease. Can Respir J 2014;21:171-5. [Crossref] [PubMed]
- Bassetti CLA, Randerath W, Vignatelli L, et al. EAN/ERS/ESO/ESRS statement on the impact of sleep disorders on risk and outcome of stroke. Eur Respir J 2020;55:1901104. [Crossref] [PubMed]
- McNicholas WT. COPD-OSA overlap syndrome: Evolving evidence regarding epidemiology, clinical consequences, and management. Chest 2017;152:1318-26. [Crossref] [PubMed]
- Machado MC, Vollmer WM, Togeiro SM, et al. CPAP and survival in moderate-to-severe obstructive sleep apnoea syndrome and hypoxaemic COPD. Eur Respir J 2010;35:132-7. [Crossref] [PubMed]
- Andrés-Blanco AM, Alvarez D, Crespo A, et al. Assessment of automated analysis of portable oximetry as a screening test for moderate-to-severe sleep apnea in patients with chronic obstructive pulmonary disease. PLoS One 2017;12:e0188094. [Crossref] [PubMed]
- Ramsey R, Mehra R, Strohl KP. Variations in physician interpretation of overnight pulse oximetry monitoring. Chest 2007;132:852-9. [Crossref] [PubMed]
- Guilleminault C, Connolly S, Winkle R, et al. Cyclical variation of the heart rate in sleep apnoea syndrome. Mechanisms, and usefulness of 24 h electrocardiography as a screening technique. Lancet 1984;1:126-31. [Crossref] [PubMed]
- Penzel T, McNames J, Murray A, et al. Systematic comparison of different algorithms for apnoea detection based on electrocardiogram recordings. Med Biol Eng Comput 2002;40:402-7. [Crossref] [PubMed]
- Penzel T. Is heart rate variability the simple solution to diagnose sleep apnoea? Eur Respir J 2003;22:870-1. [Crossref] [PubMed]
- Heneghan C, de Chazal P, Ryan S, et al. Electrocardiogram recording as a screening tool for sleep disordered breathing. J Clin Sleep Med 2008;4:223-8. [Crossref] [PubMed]
- Pépin JL, Delavie N, Pin I, et al. Pulse transit time improves detection of sleep respiratory events and microarousals in children. Chest 2005;127:722-30. [Crossref] [PubMed]
- Bar A, Pillar G, Dvir I, et al. Evaluation of a portable device based on peripheral arterial tone for unattended home sleep studies. Chest 2003;123:695-703. [Crossref] [PubMed]
- O'Donnell CP, Allan L, Atkinson P, et al. The effect of upper airway obstruction and arousal on peripheral arterial tonometry in obstructive sleep apnea. Am J Respir Crit Care Med 2002;166:965-71. [Crossref] [PubMed]
- Dvir I, Adler Y, Freimark D, et al. Evidence for fractal correlation properties in variations of peripheral arterial tone during REM sleep. Am J Physiol Heart Circ Physiol 2002;283:H434-9. [Crossref] [PubMed]
- Yalamanchali S, Farajian V, Hamilton C, et al. Diagnosis of obstructive sleep apnea by peripheral arterial tonometry: meta-analysis. JAMA Otolaryngol Head Neck Surg 2013;139:1343-50. [Crossref] [PubMed]
- Crinion SJ, Ryan S, McNicholas WT. Obstructive sleep apnoea as a cause of nocturnal nondipping blood pressure: recent evidence regarding clinical importance and underlying mechanisms. Eur Respir J 2017;49:1601818. [Crossref] [PubMed]
- Cuspidi C, Tadic M, Sala C, et al. Blood Pressure Non-Dipping and Obstructive Sleep Apnea Syndrome: A Meta-Analysis. J Clin Med 2019;8:1367. [Crossref] [PubMed]
- Genta-Pereira DC, Furlan SF, Omote DQ, et al. Nondipping Blood Pressure Patterns Predict Obstructive Sleep Apnea in Patients Undergoing Ambulatory Blood Pressure Monitoring. Hypertension 2018;72:979-85. [Crossref] [PubMed]
- Crinion SJ, Ryan S, Kleinerova J, et al. Nondipping Nocturnal Blood Pressure Predicts Sleep Apnea in Patients With Hypertension. J Clin Sleep Med 2019;15:957-63. [Crossref] [PubMed]
- Marrone O, Bonsignore MR. Blood-pressure variability in patients with obstructive sleep apnea: current perspectives. Nat Sci Sleep 2018;10:229-42. [Crossref] [PubMed]
- Davies RJ, Jenkins NE, Stradling JR. Effect of measuring ambulatory blood pressure on sleep and on blood pressure during sleep. BMJ 1994;308:820-3. [Crossref] [PubMed]
- Davies RJ, Crosby J, Vardi-Visy K, et al. Non-invasive beat to beat arterial blood pressure during non-REM sleep in obstructive sleep apnoea and snoring. Thorax 1994;49:335-9. [Crossref] [PubMed]
- Lazazzera R, Belhaj Y, Carrault G. A New Wearable Device for Blood Pressure Estimation Using Photoplethysmogram. Sensors (Basel) 2019;19:2557. [Crossref] [PubMed]
- De Luca Canto G, Pachêco-Pereira C, Aydinoz S, et al. Biomarkers associated with obstructive sleep apnea and morbidities: a scoping review. Sleep Medicine 2015;16:347-57. [Crossref] [PubMed]
- Archontogeorgis K, Nena E, Papanas N, et al. Biomarkers to improve diagnosis and monitoring of obstructive sleep apnea syndrome: current status and future perspectives. Pulm Med 2014;2014:930535. [Crossref] [PubMed]
- Schwarz EI, Engler A, Kohler M. Exhaled breath analysis in obstructive sleep apnea. Expert Rev Respir Med 2017;11:631-9. [Crossref] [PubMed]
- Alshaer H, Hummel R, Mendelson M, et al. Objective Relationship Between Sleep Apnea and Frequency of Snoring Assessed by Machine Learning. J Clin Sleep Med 2019;15:463-70. [Crossref] [PubMed]
- Alshaer H, Fernie GR, Maki E, et al. Validation of an automated algorithm for detecting apneas and hypopneas by acoustic analysis of breath sounds. Sleep Med 2013;14:562-71. [Crossref] [PubMed]
- Alshaer H, Levchenko A, Bradley TD, et al. A system for portable sleep apnea diagnosis using an embedded data capturing module. J Clin Monit Comput 2013;27:303-11. [Crossref] [PubMed]
- Alshaer H, Fernie GR, Tseng WH, et al. Comparison of in-laboratory and home diagnosis of sleep apnea using a cordless portable acoustic device. Sleep Med 2016;22:91-6. [Crossref] [PubMed]
- Abbasi J. In-home, Over-the-counter Sleep Apnea Sensor on the Horizon. JAMA 2017;317:2271. [PubMed]
- Masa JF, Duran-Cantolla J, Capote F, et al. Effectiveness of home single-channel nasal pressure for sleep apnea diagnosis. Sleep 2014;37:1953-61. [Crossref] [PubMed]
- Erman MK, Stewart D, Einhorn D, et al. Validation of the ApneaLink for the screening of sleep apnea: a novel and simple single-channel recording device. J Clin Sleep Med 2007;3:387-92. [Crossref] [PubMed]
- Oktay B, Rice TB, Atwood CW Jr, et al. Evaluation of a single-channel portable monitor for the diagnosis of obstructive sleep apnea. J Clin Sleep Med 2011;7:384-90. [Crossref] [PubMed]
- Practice parameters for the use of actigraphy in the clinical assessment of sleep disorders. American Sleep Disorders Association. Sleep 1995;18:285-7. [Crossref] [PubMed]
- Morgenthaler T, Alessi C, Friedman L, et al. Practice parameters for the use of actigraphy in the assessment of sleep and sleep disorders: an update for 2007. Sleep 2007;30:519-29. [Crossref] [PubMed]
- Sadeh A, Hauri PJ, Kripke DF, et al. The role of actigraphy in the evaluation of sleep disorders. Sleep 1995;18:288-302. [Crossref] [PubMed]
- Sadeh A. The role and validity of actigraphy in sleep medicine: an update. Sleep Med Rev 2011;15:259-67. [Crossref] [PubMed]
- Martin JL, Hakim AD. Wrist actigraphy. Chest 2011;139:1514-27. [Crossref] [PubMed]
- Goldstone A, Baker FC, de Zambotti M. Actigraphy in the digital health revolution: still asleep? Sleep 2018.41. [PubMed]
- Marino M, Li Y, Rueschman MN, et al. Measuring sleep: accuracy, sensitivity, and specificity of wrist actigraphy compared to polysomnography. Sleep 2013;36:1747-55. [Crossref] [PubMed]
- Salmi T, Telakivi T, Partinen M. Evaluation of automatic analysis of SCSB, airflow and oxygen saturation signals in patients with sleep related apneas. Chest 1989;96:255-61. [Crossref] [PubMed]
- Pallin M, O'hare E, Zaffaroni A, et al. Comparison of a novel non-contact biomotion sensor with wrist actigraphy in estimating sleep quality in patients with obstructive sleep apnoea. J Sleep Res 2014;23:475-84. [Crossref] [PubMed]
- De Chazal P, Fox N, O'Hare E, et al. Sleep/wake measurement using a non-contact biomotion sensor. J Sleep Res 2011;20:356-66. [Crossref] [PubMed]
- Zaffaroni A, de Chazal P, Heneghan C, et al. SleepMinder: an innovative contact-free device for the estimation of the apnoea-hypopnoea index. Conf Proc IEEE Eng Med Biol Soc 2009;2009:7091-4. [PubMed]
- Zaffaroni A, Kent B, O'Hare E, et al. Assessment of sleep-disordered breathing using a non-contact bio-motion sensor. J Sleep Res 2013;22:231-6. [Crossref] [PubMed]
- Pallin M, O'Hare E, Zaffaroni A, et al. Comparison of a novel non-contact biomotion sensor with wrist actigraphy in estimating sleep quality in patients with obstructive sleep apnoea. J Sleep Res 2014;23:475-84. [Crossref] [PubMed]
- Crinion SJ, Tiron R, Lyon G, et al. Ambulatory detection of sleep apnea using a non-contact biomotion sensor. J Sleep Res 2020;29:e12889. [Crossref] [PubMed]
- Ko PR, Kientz JA, Choe EK, et al. Consumer Sleep Technologies: A Review of the Landscape. J Clin Sleep Med 2015;11:1455-61. [Crossref] [PubMed]
- Natale V, Drejak M, Erbacci A, et al. Monitoring sleep with a smartphone accelerometer. Sleep and Biological Rhythms 2012;10:287-92. [Crossref]
- Penzel T, Schobel C, Fietze I. New technology to assess sleep apnea: wearables, smartphones, and accessories. F1000Res 2018;7:413. [Crossref] [PubMed]
- Behar J, Roebuck A, Domingos JS, et al. A review of current sleep screening applications for smartphones. Physiol Meas 2013;34:R29-46. [Crossref] [PubMed]
- Van de Water AT, Holmes A, Hurley DA. Objective measurements of sleep for non-laboratory settings as alternatives to polysomnography--a systematic review. J Sleep Res 2011;20:183-200. [Crossref] [PubMed]
- Van den Bulck J. Sleep apps and the quantified self: blessing or curse? J Sleep Res 2015;24:121-3. [Crossref] [PubMed]
- Stippig A, Hubers U, Emerich M. Apps in sleep medicine. Sleep Breath 2015;19:411-7. [Crossref] [PubMed]
- Baron KG, Duffecy J, Berendsen MA, et al. Feeling validated yet? A scoping review of the use of consumer-targeted wearable and mobile technology to measure and improve sleep. Sleep Med Rev 2018;40:151-9. [Crossref] [PubMed]
- Choi YK, Demiris G, Lin SY, et al. Smartphone Applications to Support Sleep Self-Management: Review and Evaluation. J Clin Sleep Med 2018;14:1783-90. [Crossref] [PubMed]
- Bhat S, Ferraris A, Gupta D, et al. Is There a Clinical Role For Smartphone Sleep Apps? Comparison of Sleep Cycle Detection by a Smartphone Application to Polysomnography. J Clin Sleep Med 2015;11:709-15. [Crossref] [PubMed]
- Toon E, Davey MJ, Hollis SL, et al. Comparison of Commercial Wrist-Based and Smartphone Accelerometers, Actigraphy, and PSG in a Clinical Cohort of Children and Adolescents. J Clin Sleep Med 2016;12:343-50. [Crossref] [PubMed]
- Ong AA, Gillespie MB. Overview of smartphone applications for sleep analysis. World J Otorhinolaryngol Head Neck Surg 2016;2:45-9. [Crossref] [PubMed]
- Paquet J, Kawinska A, Carrier J. Wake detection capacity of actigraphy during sleep. Sleep 2007;30:1362-9. [Crossref] [PubMed]
- Patel P, Kim JY, Brooks LJ. Accuracy of a smartphone application in estimating sleep in children. Sleep Breath 2017;21:505-11. [Crossref] [PubMed]
- Fino E, Plazzi G, Filardi M, et al. (Not so) Smart sleep tracking through the phone: Findings from a polysomnography study testing the reliability of four sleep applications. J Sleep Res 2020;29:e12935. [Crossref] [PubMed]
- Tal A, Shinar Z, Shaki D, et al. Validation of Contact-Free Sleep Monitoring Device with Comparison to Polysomnography. J Clin Sleep Med 2017;13:517-22. [Crossref] [PubMed]
- Al-Mardini M, Aloul F, Sagahyroon A, et al. Classifying obstructive sleep apnea using smartphones. J Biomed Inform 2014;52:251-9. [Crossref] [PubMed]
- Behar J, Roebuck A, Shahid M, et al. SleepAp: an automated obstructive sleep apnoea screening application for smartphones. IEEE J Biomed Health Inform 2015;19:325-31. [Crossref] [PubMed]
- Endeshaw Y, Rice TB, Schwartz AV, et al. Snoring, daytime sleepiness, and incident cardiovascular disease in the health, aging, and body composition study. Sleep 2013;36:1737-45. [Crossref] [PubMed]
- Nakano H, Hirayama K, Sadamitsu Y, et al. Monitoring sound to quantify snoring and sleep apnea severity using a smartphone: proof of concept. J Clin Sleep Med 2014;10:73-8. [Crossref] [PubMed]
- Petersen CL, Chen TP, Ansermino JM, et al. Design and evaluation of a low-cost smartphone pulse oximeter. Sensors (Basel) 2013;13:16882-93. [Crossref] [PubMed]
- Nagappa M, Liao P, Wong J, et al. Validation of the STOP-Bang Questionnaire as a Screening Tool for Obstructive Sleep Apnea among Different Populations: A Systematic Review and Meta-Analysis. PLoS One 2015;10:e0143697. [Crossref] [PubMed]
- Daly J, Roebuck A, Palmius N, et al., editors. Sleepcare: obstructive sleep apnoea screening using mobile health technology. Appropriate Healthcare Technologies for Low Resource Settings (AHT 2014); 2014 17-18 Sept. 2014.
- Kolla BP, Mansukhani S, Mansukhani MP. Consumer sleep tracking devices: a review of mechanisms, validity and utility. Expert Rev Med Devices 2016;13:497-506. [Crossref] [PubMed]
- Bianchi MT. Sleep devices: wearables and nearables, informational and interventional, consumer and clinical. Metabolism 2018;84:99-108. [Crossref] [PubMed]
- Montgomery-Downs HE, Insana SP, Bond JA. Movement toward a novel activity monitoring device. Sleep Breath 2012;16:913-7. [Crossref] [PubMed]
- Kang SG, Kang JM, Ko KP, et al. Validity of a commercial wearable sleep tracker in adult insomnia disorder patients and good sleepers. J Psychosom Res 2017;97:38-44. [Crossref] [PubMed]
- Mantua J, Gravel N, Spencer RM. Reliability of Sleep Measures from Four Personal Health Monitoring Devices Compared to Research-Based Actigraphy and Polysomnography. Sensors (Basel) 2016;16:646. [Crossref] [PubMed]
- Rosenberger ME, Buman MP, Haskell WL, et al. Twenty-four Hours of Sleep, Sedentary Behavior, and Physical Activity with Nine Wearable Devices. Med Sci Sports Exerc 2016;48:457-65. [Crossref] [PubMed]
- de Zambotti M, Goldstone A, Claudatos S, et al. A validation study of Fitbit Charge 2 compared with polysomnography in adults. Chronobiol Int 2018;35:465-76. [Crossref] [PubMed]
- Liang Z, Chapa-Martell MA. Accuracy of Fitbit Wristbands in Measuring Sleep Stage Transitions and the Effect of User-Specific Factors. JMIR Mhealth Uhealth 2019;7:e13384. [Crossref] [PubMed]
- Haghayegh S, Khoshnevis S, Smolensky MH, et al. Accuracy of Wristband Fitbit Models in Assessing Sleep: Systematic Review and Meta-Analysis. J Med Internet Res 2019;21:e16273. [Crossref] [PubMed]
- Benedetto S, Caldato C, Bazzan E, et al. Assessment of the Fitbit Charge 2 for monitoring heart rate. PLoS One 2018;13:e0192691. [Crossref] [PubMed]
- Nelson BW, Allen NB. Accuracy of Consumer Wearable Heart Rate Measurement During an Ecologically Valid 24-Hour Period: Intraindividual Validation Study. JMIR Mhealth Uhealth 2019;7:e10828. [Crossref] [PubMed]
- Kanady JC, Ruoff L, Straus LD, et al. Validation of Sleep Measurement in a Multi-Sensor Consumer Grade Wearable Device in Healthy Young Adults. J Clin Sleep Med 2020;16:917-24. [Crossref] [PubMed]
- Gruwez A, Bruyneel AV, Bruyneel M. The validity of two commercially-available sleep trackers and actigraphy for assessment of sleep parameters in obstructive sleep apnea patients. PLoS One 2019;14:e0210569. [Crossref] [PubMed]
- Rotenberg BW, Murariu D, Pang KP. Trends in CPAP adherence over twenty years of data collection: a flattened curve. J Otolaryngol Head Neck Surg 2016;45:43. [Crossref] [PubMed]
- Haniffa M, Lasserson TJ, Smith I. Interventions to improve compliance with continuous positive airway pressure for obstructive sleep apnoea. Cochrane Database Syst Rev 2004.CD003531. [PubMed]
- Cistulli PA, Armitstead J, Pepin JL, et al. Short-term CPAP adherence in obstructive sleep apnea: a big data analysis using real world data. Sleep Med 2019;59:114-6. [Crossref] [PubMed]
- Lugo VM, Garmendia O, Suarez-Giron M, et al. Comprehensive management of obstructive sleep apnea by telemedicine: Clinical improvement and cost-effectiveness of a Virtual Sleep Unit. A randomized controlled trial. PLoS One 2019;14:e0224069. [Crossref] [PubMed]
- Malhotra A, Crocker ME, Willes L, et al. Patient Engagement Using New Technology to Improve Adherence to Positive Airway Pressure Therapy: A Retrospective Analysis. Chest 2018;153:843-50. [Crossref] [PubMed]
- Petrov ME, Hasanaj K, Hoffmann CM, et al. Rationale, design, and development of SleepWell24: A smartphone application to promote adherence to positive airway pressure therapy among patients with obstructive sleep apnea. Contemp Clin Trials 2020;89:105908. [Crossref] [PubMed]