Bioinformatics analysis of key genes and miRNAs associated with Stanford type A aortic dissection
Introduction
Aortic dissection (AD), characterized by tears in the aortic wall, is one of the most detrimental cardiovascular diseases. Based on the Stanford classification method, type A aortic dissection (AAD) involves the ascending aorta, while type B aortic dissection involves the descending aorta. AAD carries a high risk of mortality and morbidity (1). Epidemiological studies have shown that the death rate of untreated patients with AAD is 50% (36–72%) within 48 hours (2). Despite continuous advances in diagnostic methods, operative techniques, and perioperative care, AAD remains a major unresolved cardiovascular surgical challenge (3). A further understanding of the potential regulatory pathways and mechanisms of AAD may provide new insights into therapeutic strategies.
Previous studies have found unique microRNAs (miRNAs) and genes that may underlie the pathogenesis of AAD. Several miRNA-gene interactions and mechanisms in the development of AAD through combining miRNA and messenger RNA (mRNA) microarrays have been demonstrated with in vitro validation (4). MiRNA-320 was found to downregulate the expression of matrix metalloprotein by macrophages in AD patients (5). Although there are an increasing number of AAD related transcriptome profiles, there is no current comprehensive bioinformatics analysis to summarize the existing data.
Therefore, after searching for AAD gene expression datasets in the public database: Gene Expression Omnibus (GEO), and ArrayExpress, two microarray datasets were included in our study. Weighted gene co-expression network analysis (WGCNA) was used to construct a co-expression network, and the highly preserved modules with genes from the two datasets were selected. The MetaDE method in R language, which can conduct 12 primary meta-analysis methods (6), was implemented for identifying the differentially expressed genes (DEGs). After overlapping these significant consistency genes and the DEGs, we then further selected the differentially expressed miRNAs (DEmiRNAs). Subsequently, the interactions among the DEGs and DEmiRNAs were predicted and followed this with functional analysis of the genes. The in-depth analysis conducted in this study may provide novel insights into the pathogenesis of AAD, along with new biomarkers and therapeutic targets for human AAD. We present the following article in accordance with the MDAR reporting checklist (available at http://dx.doi.org/10.21037/jtd-20-1337).
Methods
Microarray data
This work used the public microarray data and does not include any human tissue. The ethical approval was not necessarily required.
We searched for the microarray data of AAD patients in the National Center for Biotechnology Information (NCBI) GEO (http://www.ncbi.nlm.nih.gov/geo/) and ArrayExpress (https://www.ebi.ac.uk/arrayexpress/). The inclusion criteria were as follows: (I) samples in the dataset were collected from the aorta; (II) the samples included AAD patients and healthy controls; (III) the dataset was based on human gene expression profiles. Two datasets, GSE98770 (n=11; DA, 6; control, 5), and GSE52093 (n=12; DA, 7; control, 5) that met the criteria were included in the present study.
For preprocessing of raw data, both datasets were downloaded from the NCBI GEO website and subsequently preprocessed using R software. The annotation and clinical traits information were downloaded using GEOquery package (7).
For the specific data preprocessing of GSE52093, we used the Illumina HumanHT-12 V4.0 platform, retrained the probes with detection P value less than 0.05 in more than 6 samples, and normalized by the oligo package (8). We preprocessed the GSE98770 gene and miRNA expression profile using the method for Agilent data in the limma package (9). After excluding the probes that are unable to be annotated, we combined the probes annotated with the same genes using the median method. Then, GSE52093 gene expression data was log2-transformed to achieve an approximate healthy distribution.
WGCNA construction and module selection process
WGCNA package (10) was required for the co-expression network construction. Firstly, we selected 5000 common genes with the most variance in both the GSE52093 and GSE98770 gene expression datasets. After evaluating the correlations between the two datasets using the verboseScatterplot function, we calculated the soft threshold value based on a scale-free topology criterion in GSE52093 (scale-free R2 =0.9). The weighted adjacency matrix was constructed using the soft-thresholding power. Relationships between one gene and all the other genes in the analysis were incorporated, and the adjacency matrix was transformed into the topological matrix (TOM). Subsequently, a hierarchical clustering analysis (6) of genes was performed using 1-TOM as the distance measure. To acquire a small number of large modules, modules were detected using a dynamic tree cut algorithm with a minimum module size of 50 and a minimum cut height of 0.99. Furthermore, module preservation between the two datasets was measured using the specific function of the WGCNA software package (10). After the clinical information was imported into the co-expression network, the module eigengene (ME) was calculated. ME is representative of the gene expression profiles in a module, illustrating the average expression level of genes in the module. The module could serve as a candidate if it had a high correlation value between ME valve and the clinical trait (10). We measured the correlation between the modules and clinical traits of samples such as gender and AAD by Pearson’s correlation analysis. Only the modules with both high preservation and high correlation were exported for further analysis.
Identification of DEGs and miRNAs (DEmiRNAs)
DEGs in the selected modules between AAD and healthy samples were screened in both datasets using the MetaDE package (11), which can utilize multi-core parallel processing for fast computation while generating informative outputs and visualization plots. We compared four meta methods [maxP, Fisher, roP, and adaptively weighted, (AW)] and chose the one that had the most DEGs identified. The threshold for DEGs was set as P<0.01 and the false detection rate was set as <0.01 (11). Principal component analysis (PCA) was performed for the miRNAs using factoextra package (12) before the DEmiRNAs were identified using Limma R package (9) between AAD and healthy samples. Correction for multiple testing was performed with the Benjamini-Hochberg (BH) method. DEmiRNAs were selected if the P value is less than 0.05. and heatmaps were generated using the pheatmap package (13).
Gene set enrichment analysis (GSEA) and gene set variation analysis (GSVA) on the selected modules
The GSEA desktop application was downloaded from GSEA website (http://software.broadinstitute.org/gsea/index.jsp) (14) and was used to associate the potential gene signature sets by comparing the AAD to the healthy samples. The expression profile of DEGs in selected modules of GSE52093 was exported and analyzed with version 4.0.3 of GSEA. The normalized enrichment score (NES) is the primary statistic for examining gene set enrichment results, and the nominal P value estimates the statistical significance of the enrichment score and therefore a gene set with nominal P≤0.05 was considered to be significantly enriched. We also used the GSVA in the R package GSVA (15) to explore variation in higher-order molecular traits. GSVA defines a set of synthetic traits based on a list of gene ontology (GO) terms. We chose the “h.all.v7.0.symbols.gmt” gene sets downloaded from “Molecular Signatures Database” as our terms. Each gene set’s value represents the extent to which genes labeled with a specific term tend to be up- or downregulated in that sample, with measurement being taken using a Kolmogorov–Smirnov-like random walk statistic. Coordinated regulation of genes in the same pathway or involved in similar processes is evidence for changes in the activity of specific cellular functions.
The construction of the DEmiRNA-DEG network
We predicted the DEmiRNA-DEG interactions using miRTarVis+ v.0.8.11 (http://sehilyi.com/mirtarvisplus/) (16) which implemented prediction algorithms based on both sequence and expression profile data and supports the two most cited sequence-based miRNA target prediction algorithms, TargetScan (17) and microRNA.org (18). After loading the expression profile of the DEGs of the selected modules and DEmiRNAs online, we obtained the common DEmiRNA-DEG interactions using both TargetScan and microRNA.org algorithms. The predicted genes were imported into STRING 11.0 (https://string-db.org/) to construct a protein-protein interaction (PPI) network. Both the PPI and DEmiRNA-DEG interactions were downloaded and visualized in Cytoscape. 3.7.2 software (19). We implemented the yFiles Layout Algorithms app (https://www.yworks.com/products/yfiles-layout-algorithms-for-cytoscape) to construct a circular layout. In the network, a node represents a gene or a miRNA; the undirected link between two nodes is an edge, denoting the interaction. The degree of a node corresponds to the number of gene to gene or gene to miRNA interactions in the network.
Function annotation and pathway enrichment analysis
To elucidate the possible biological roles of the selected genes, GO functional and Kyoto Encyclopedia of Genes and Genomes (KEGG) pathway enrichment analyses were performed using the clusterprofiler package (20). We focused on the GO terms biological process (BP). We analyzed the results with the Benjamini-Hochberg’s false discovery rate (FDR) correction, with the P=0.05 set as the significant difference threshold indicating a significant difference for GO terms. The results for KEGG pathway analysis were obtained with STRING 11.0 (https://string-db.org/).
Results
Preprocessing and clinical traits of included datasets
As illustrated in Figure 1, we downloaded and normalized the gene expression data using the aforementioned method. The expression profiles after normalization were demonstrated in Figure S1. We summarized the clinical traits of clustered samples as dendrograms (Figure 2A,B). Male patients constituted most of our study, and the GSM1259277 sample in GSE52093 was excluded from further analysis as an outlier. The median age for GSE98770 was 53, however GSE52093 was lacking such information.
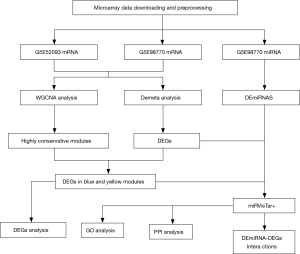
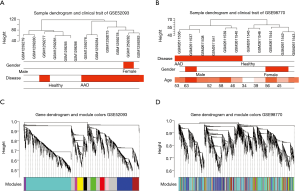
Identification of highly preserved WGCNA modules
Using WGCNA analysis processing, our study attempted to identify co-expression modules with high preservation in the GSE52093 and GSE98770 and strong AAD association. First, we assessed the correlation of average gene expression and overall connectivity between the two datasets. The correlation coefficients of genes were 0.56 with P values <1×10−200 and the connectivity was 0.099 with P value 2.3×10−12 (Figure S2), which suggests that our datasets were suitable for further analysis. Then, we set the parameter “deepSplit” =0 to achieve a small number of large modules (Figure S3). In the GSE52093 dataset, 11 modules are identified as the training set and were reconstructed as a validation set in the GSE98770 dataset (Figure 2C,D). These modules are illustrated in the branches of the dendrogram with unique colors (Figure 2C,D). We plotted the multi-dimensional scaling (MDS) and heatmap of expression data based on modules in the GSE52093 dataset, demonstrating that each module has unique expression patterns (Figures S4,S5). In 5 of the 11 modules, only the blue and yellow modules were well preserved, with Z-scores >10 and including 787 genes (Table 1). The correlation analysis also revealed that blue and yellow modules were highly correlated with disease state with r=0.91 and −0.63, respectively, and both P values =0 (Table 1). Thus, the blue and yellow modules were selected for further analysis.
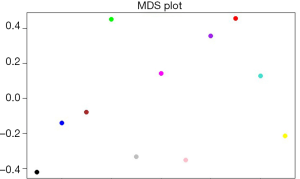
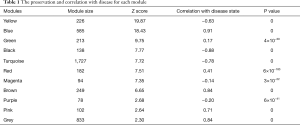
Full table
Screening for DEGs in blue and yellow modules and DEmiRNAs
After comparing the four statistical methods, we chose Fisher’s method to identify the DEGs. Of DEGs (Figure S6), 139 genes were in the yellow module, and 356 were in the blue module (Figure 3A). For miRNAs, we found a good distinguished miRNA expression pattern between the AAD and the healthy samples based on PCA analysis (Figure S7). We identified 46 DEmiRNAs and two heatmaps for these DEGs and these DEmiRNAs are visible in Figure 3B,C,D.
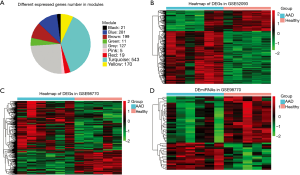
GSEA and GSVA
To further clarify the possible mechanisms of related DEGs in the blue and yellow modules, we performed GSEA analysis. As shown in the Table 2, for the AAD phenotype, GSEA analysis suggested that the genes in the blue module were enriched in gene sets such as GSE3982 Cent Memory CD4 T cell vs. Th1 DN, breast cancer basal up, GO negative regulation of hydrolase activity, TNF response not via p38 and GO DNA repair. Meanwhile, the yellow module was enriched in GO epithelium development, regulation of anatomical structure morphogenesis, GO regulation of vasculature development, GO negative regulation of response to stimulus and regulation of vasculature development, although some of the nominal P values suggested the enrichments were insignificant. Using GSVA, we considered the varying expression levels of these DEGs and found their biological roles in several other gene sets to be significant (P<0.001) (Figure 4). The up-regulated gene sets in the AAD samples included hypoxia, G2M checkpoint, PI3K/AKT motor signaling, and reactive oxygen species pathway. Meanwhile, the notch and KARS signaling pathways were down-regulated in AAD samples.
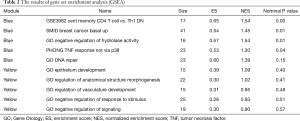
Full table
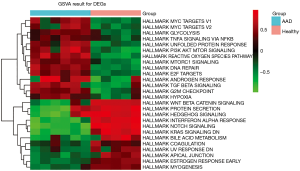
Construction of DEmiRNA-DEGs network
We uploaded the expression profile of the DEGs in the blue and yellow modules and DEmiRNAs to the miRTarVis+ website, and set it so that only the opposite direction between DEmiRNAs and DEGs to be presented. In microRNA.org and TargetScan database, 320 and 39 pairs of interactions were respectively detected. There were 25 common pairs of DEmiRNA-DEGs and 13 pairs of DEGs identified. The comprehensive interactions between 6 DEmiRNAs and 23 DEGs are illustrated in Figure 5. The genes in the blue module, which were outlined as a diamond and consistently upregulated, while the genes in the yellow module, which were rectangles, were down-regulated. Interestingly, the opposite expression level of hsa-miR-199b-5p and hsa-miR-199a-5p were identified. Meanwhile, the has-miR-199b-5p was the most connected miRNA (7 degrees) while IGF-1, TEK, KIT, and hsa-miR-152 all interacted with 6 other nodes.
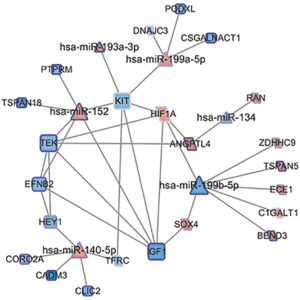
Function annotation and pathway enrichment analysis
In our study, GO analysis was used to perform the biological process analysis of the DEGs. As shown in Table 3, DEGs are highly enriched in biological processes such as epithelial cell proliferation, and positive regulation of vasculature development. We also performed KEGG pathways analysis, which found hypoxia-inducible factor 1 (HIF1) pathway to be highly enriched (Table 4). Pathways such as breast cancer, Rap1 signaling pathway, and Ras signaling pathway were also enriched.
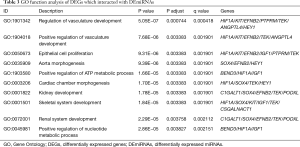
Full table
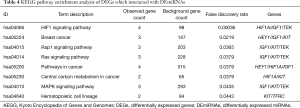
Full table
Discussion
Our study attempted to investigate the underlying pathogenic mechanisms of AAD by way of a comprehensive analysis of two GEO datasets, which contained AAD samples and healthy samples. In the WGCNA analysis, we identified two modules with high preservation in both datasets. Furthermore, our team identified the DEmiRNAs and DEGs in these two modules. By the expression of DEGs, we gained a comprehensive result showing the pathways they are involved in using GSEA and GSVA. An interaction network was constructed with 6 DEmiRNAs and 23 DEGs and functions of DEGs were annotated. Notably, the DEGs were highly concentrated in biological processes like regulation of vasculature development, positive regulation of vasculature development, and the HIF1 signaling pathway.
Interestingly, we found that in the regulatory network, the 23 DEGs in different modules had a distinctive expression profile, with up-regulated expression in the blue module and down-regulated expression in the yellow module. This result was partly expected since WGCNA analysis clusters the genes with similar expression profiles into one module. Nonetheless, regarding to the GSEA analysis, the blue module was highly enriched in the TNF response not via the p38 gene set, which suggests that the genes whose expression was altered in Calu-6 cells (lung cancer) by TNF-α were not affected by p38 inhibitor LY479754 (21). This gene set was generated from a study that asserted that despite p38 mitogen-activated protein kinase (MAPK) being activated after TNF-α stimulation, resulting in G2 DNA damage checkpoint-mediated cell cycle arrest, its activity is not necessary for the activation or maintenance of the G2 DNA damage checkpoint after inhibition. These pathways vary significantly in GSVA results with respect to the G2M checkpoint, TNF-α signaling via nuclear factor κB (NFκB), and DNA repair gene sets up-regulated in AAD samples compared with healthy samples. In other words, the function of the blue module highly correlates to the upregulation of cell cycle arrest through a pathway other than via MAPK. A similar mechanism was discovered by Rajan et al. (22), who showed that NFκB, but not p38 MAPK, is required for TNF-α-induced expression of cell adhesion molecules in endothelial cells (22).
On the other hand, the yellow module highly correlated with the negative regulation of vasculature development, which might be the consequence of the cell cycle arrest due to the blue module function. Previous studies have shown that the inhibitory effect of the Notch signaling cascade in endothelial cells induces differentiation-associated growth arrest through both MAPK and PI3K/Akt pathways (23), and is involved in angiogenesis and vasculature development (24,25). In tumor cells, activation of the PI3K/AKT pathway can also increase vascular endothelial growth factor secretion through HIF1 and affect vascular development (24). Meanwhile, the Notch is now considered to be a crucial player in vascular homeostasis and inflammatory response (26). Taking the function analysis for the two highly preserved modules together, we can surmise that inflammatory reaction and hypoxia caused DNA repair underlying the mechanism of AAD. Insights into how the Notch impacts vascular biology would be crucial for the design of therapeutic strategies for AAD.
It is acknowledged that vascular smooth muscle cells (VSMCs) act as a key member in the media of the human aorta, and which have two kinds of cell phenotypes, including contractile and synthetic status. In aortic dissection, contractile VSMCs can become synthetic VSMCs, which can trigger a cascade of events and induce dissection. Previous research has shown that, through the PI3K/AKT signaling pathway, increased expression of HIF1A in the media of the thoracic aortic dissection tissues, as compared with normal aortic tissues, associates with human aortic smooth muscle cell phenotype switch (27). Recently, macrophage reprogramming was proven to take part in the aggravation of aortic dissection by activating the HIF1A-related pathway (28). In our results, the HIF1 pathway is highlighted in the network of DEGs with the enrichment of HIF1A, IGF1, and TEK.
Angiopoietin-1 receptor (TEK/Tie-2) is a tyrosine-protein kinase that acts as a cell-surface receptor for angiopoietin-1 (ANG1), angiopoietin-2 (ANG2) and angiopoietin-4 (ANG4) which regulates angiogenesis, endothelial cell survival and maintenance of vascular quiescence. One study investigated the molecular basis by which HIF1 mediates the angiogenic response to hypoxia; it was concluded that ANG4 can function similarly to ANG1 and acts as a substitute for ANG1 to participate in hypoxia-induced angiogenesis (29). Importantly, activation of the angiopoietin/Tie-2 system may impact the ability of HIF1A to induce hypervascularity without excessive permeability. However, a more recent study (30) revealed that the genetic deletion of HIF2A but not HIF1A could harm the microvascular integrity in airways through endothelial angiopoietin-1/TIE2 signaling and Notch activity. The mechanisms of aortic dissection might correlate with novel interactions between angiopoietins, TEK receptors, and HIFs.
Noticeably, in our network, insulin-like growth factor 1 (IGF1), mast/stem cell growth factor receptor Kit (KIT), and TEK are present in several important pathways, such as the PI3K/AKT pathway, Rap1 signaling pathway, Ras signaling pathway, and the MAPK signaling pathway. A study by Weeks et al. has shown that IGF1/PI3K/Akt signaling pathway was activated by the exercise-induced cardiac hypertrophy and protection (31). In their experiment, mice with elevated IGF1/PI3K/Akt signaling displayed cardiac protection in settings of dilated cardiomyopathy, myocardial infarction, and pressure overload, whereas mice with reduced IGF1/PI3K/Akt signaling were more susceptible to pathological remodeling. However, in an earlier study (32), the elevated expression of IGF1 was speculated to correct the contractile defect due to the MYH11 mutation as a compensatory action of the aortic SMCs, leading to increased vascular volume in the vasa vasorum and AAD. A more thorough understanding of the role that IGF1 plays in the pathogenesis of AAD and vasculature development might help clinicians to reveal the key pathways and modulation patterns.
In terms of the DEmiRNAs, miR-199b-5p (MIMAT0000263) is a hot node with seven interactions. Sun et al. (33) reviewed the regulatory role of microRNAs in angiogenesis-related diseases and found miR-199b-5p could manage angiogenesis by targeting NAD-dependent protein deacetylase sirtuin-1 (SIRT1). It is classified with miRNAs that are modulated by pro- or antiangiogenic factors or hypoxia. In other studies (34), focusing on human intracranial aneurysms, the interaction of miR-199b-5p and HIF1A contributes to the response to oxidative stress. The role of miR-199b-5p in AAD has not yet been fully clarified. Another regulator of interest is miR-152 (MIMAT0026479) due to its association with KIT, TEK, and IGF1. However, only Liu et al. (35) found miR-199b-5p to be down-regulated in a rat model of abdominal aortic aneurysms. The possible effect that miR-152 makes together with KIT, TEK and IGF1 would be an intriguing topic to research in greater-depth. Moreover, a decreased level of miR-134-5p (MIMAT0000447) has already been proven to inhibit the VSMC phenotypic switch and migration in thoracic aortic dissection (36). Our study provides another perspective in which miR-134-5p might be a mediator in the AAD pathological process.
Our study had some limitations that should be addressed. Firstly, all the microarray datasets were obtained from pure public data and some inevitable biases, such as gender and age differences were present. Additionally, only a small number of datasets were analyzed in this study. Furtherly, genes altered with AAD need further examination to determine if overexpression/knockdown of such genes plays a significant role in AAD in vivo, which can also inspire new therapy potentially.
Conclusions
In summary, our study yielded a comprehensive interactive network of miRNAs and genes which tend to play an important role in the pathogenesis of AAD. The HIF pathway was highly enriched. Genes such as IGF1, TEK, KIT, and HIF1A, and miRNAs like miR-199b-5p could be promising targets for further analysis.
Acknowledgments
Funding: This work was supported by the National Natural Science Foundation of China (Grant No. 81700410), Sichuan Science and Technology Program (Grant No. 2019YFS0344, Grant No. 2019YFS0251).
Footnote
Reporting Checklist: The authors have completed the MDAR reporting checklist. Available at http://dx.doi.org/10.21037/jtd-20-1337
Conflicts of Interest: All authors have completed the ICMJE uniform disclosure form (available at http://dx.doi.org/10.21037/jtd-20-1337). The authors have no conflicts of interest to declare.
Ethical Statement: The authors are accountable for all aspects of the work in ensuring that questions related to the accuracy or integrity of any part of the work are appropriately investigated and resolved. This work used the public microarray data and does not include any human tissue. The ethical approval was not necessarily required.
Open Access Statement: This is an Open Access article distributed in accordance with the Creative Commons Attribution-NonCommercial-NoDerivs 4.0 International License (CC BY-NC-ND 4.0), which permits the non-commercial replication and distribution of the article with the strict proviso that no changes or edits are made and the original work is properly cited (including links to both the formal publication through the relevant DOI and the license). See: https://creativecommons.org/licenses/by-nc-nd/4.0/.
References
- Golledge J, Eagle KA. Acute aortic dissection. Lancet 2008;372:55-66. [Crossref] [PubMed]
- LeMaire SA, Russell L. Epidemiology of thoracic aortic dissection. Nat Rev Cardiol 2011;8:103-13. [Crossref] [PubMed]
- Chiu P, Miller DC. Evolution of surgical therapy for Stanford acute type A aortic dissection. Ann Cardiothorac Surg 2016;5:275-95. [Crossref] [PubMed]
- Kimura N, Futamura K, Arakawa M, et al. Gene expression profiling of acute type A aortic dissection combined with in vitro assessment. Eur J Cardiothorac Surg 2017;52:810-7. [Crossref] [PubMed]
- Liao M, Zou S, Bao Y, et al. Matrix metalloproteinases are regulated by MicroRNA 320 in macrophages and are associated with aortic dissection. Exp Cell Res 2018;370:98-102. [Crossref] [PubMed]
- Langfelder P, Horvath S. Fast R functions for robust correlations and hierarchical clustering. J Stat Softw 2012;46:1-17. [Crossref] [PubMed]
- Davis S, Meltzer PS. GEOquery: A bridge between the Gene Expression Omnibus (GEO) and BioConductor. Bioinformatics 2007;23:1846-7. [Crossref] [PubMed]
- Carvalho BS, Irizarry RA. A framework for oligonucleotide microarray preprocessing. Bioinformatics 2010;26:2363-7. [Crossref] [PubMed]
- Ritchie ME, Phipson B, Wu D, et al. Limma powers differential expression analyses for RNA-sequencing and microarray studies Nucleic Acids Res 2015;43:e47. [Crossref] [PubMed]
- Langfelder P, Horvath S. WGCNA: An R package for weighted correlation network analysis. BMC Bioinformatics 2008;9:559. [Crossref] [PubMed]
- Wang X, Kang DD, Shen K, et al. An r package suite for microarray meta-analysis in quality control, differentially expressed gene analysis and pathway enrichment detection. Bioinformatics 2012;28:2534-6. [Crossref] [PubMed]
- Kassambara A, Mundt F. Factoextra: Extract and visualize the results of multivariate data analyses 2017. R package version 2018;1.
- Kolde R. Pheatmap: pretty heatmaps R package version 1.0.12.
- Subramanian A, Tamayo P, Mootha VK, et al. Gene set enrichment analysis: A knowledge-based approach for interpreting genome-wide expression profiles Proc Natl Acad Sci U S A 2005;102:15545-50. [Crossref] [PubMed]
- Hänzelmann S, Castelo R, Guinney J. GSVA: Gene set variation analysis for microarray and RNA-Seq data BMC Bioinformatics 2013;14.7.
- L’Yi S, Jung D, Oh M, et al. miRTarVis+: Web-based interactive visual analytics tool for microRNA target predictions. Methods 2017;124:78-88. [Crossref] [PubMed]
- Lewis BP, Burge CB, Bartel DP. Conserved Seed Pairing, Often Flanked by Adenosines, Indicates that Thousands of Human Genes are MicroRNA Targets. Cell 2005;120:15-20. [Crossref] [PubMed]
- Betel D, Wilson M, Gabow A, et al. The microRNA.org resource: targets and expression. Nucleic Acids Res 2008;36:D149-53. [Crossref] [PubMed]
- Shannon P, Markiel A, Ozier O, et al. Cytoscape: A software environment for integrated models of biomolecular interaction networks. Genome Res 2003;13:2498-504. [Crossref] [PubMed]
- Yu G, Wang LG, Han Y, et al. ClusterProfiler: An R package for comparing biological themes among gene clusters. OMICS 2012;16:284-7. [Crossref] [PubMed]
- Phong MS, Van Horn RD, Li S, et al. p38 Mitogen-Activated Protein Kinase Promotes Cell Survival in Response to DNA Damage but Is Not Required for the G2 DNA Damage Checkpoint in Human Cancer Cells. Mol Cell Biol 2010;30:3816-26. [Crossref] [PubMed]
- Rajan S, Ye J, Bai S, et al. NF-κB, but not p38 MAP kinase, is required for TNF-α-induced expression of cell adhesion molecules in endothelial cells. J Cell Biochem 2008;105:477-86. [Crossref] [PubMed]
- Liu ZJ, Xiao M, Balint K, et al. Inhibition of endothelial cell proliferation by Notch1 signaling is mediated by repressing MAPK and PI3K/Akt pathways and requires MAML1. FASEB J 2006;20:1009-11. [Crossref] [PubMed]
- Karar J, Maity A. PI3K/AKT/mTOR Pathway in Angiogenesis. Front Mol Neurosci 2011;4:51. [Crossref] [PubMed]
- Dou GR, Wang L, Wang YS, et al. Notch signaling in ocular vasculature development and diseases. Mol Med 2012;18:47-55. [Crossref] [PubMed]
- Quillard T, Charreau B. Impact of Notch signaling on inflammatory responses in cardiovascular disorders. Int J Mol Sci 2013;14:6863-88. [Crossref] [PubMed]
- Liu K, Fang C, Shen Y, et al. Hypoxia-inducible factor 1α induces phenotype switch of human aortic vascular smooth muscle cell through PI3K/AKT/AEG-1 signaling. Oncotarget 2017;8:33343-52. [Crossref] [PubMed]
- Lian G, Li X, Zhang L, et al. Macrophage metabolic reprogramming aggravates aortic dissection through the HIF1α-ADAM17 pathway. EBioMedicine 2019;49:291-304. [Crossref] [PubMed]
- Yamakawa M, Liu LX, Date T, et al. Hypoxia-Inducible Factor-1 Mediates Activation of Cultured Vascular Endothelial Cells by Inducing Multiple Angiogenic Factors. Circ Res 2003;93:664-73. [Crossref] [PubMed]
- Jiang X, Tian W, Tu AB, et al. Endothelial Hypoxia-Inducible Factor-2 Is Required for the Maintenance of Airway Microvasculature. Circulation 2019;139:502-17. [Crossref] [PubMed]
- Weeks KL, Bernardo BC, Ooi JYY, et al. The IGF1-PI3K-Akt signaling pathway in mediating exercise-induced cardiac hypertrophy and protection. Adv Exp Med Biol 2017.187-210. [Crossref] [PubMed]
- Milewicz DM, Guo DC, Tran-Fadulu V, et al. Genetic Basis of Thoracic Aortic Aneurysms and Dissections: Focus on Smooth Muscle Cell Contractile Dysfunction. Annu Rev Genomics Hum Genet 2008;9:283-302. [Crossref] [PubMed]
- Sun LL, Li WD, Lei FR, et al. The regulatory role of microRNAs in angiogenesis-related diseases. J Cell Mol Med 2018;22:4568-87. [Crossref] [PubMed]
- Liu D, Han L, Wu X, et al. Genome-wide microRNA changes in human intracranial aneurysms. BMC Neurol 2014;14:188. [Crossref] [PubMed]
- Liu G, Huang Y, Lu X, et al. Identification and characteristics of microRNAs with altered expression patterns in a rat model of abdominal aortic aneurysms. Tohoku J Exp Med 2010;222:187-93. [Crossref] [PubMed]
- Wang Y, Dong CQ, Peng GY, et al. MicroRNA-134-5p Regulates Media Degeneration through Inhibiting VSMC Phenotypic Switch and Migration in Thoracic Aortic Dissection. Mol Ther Nucleic Acids 2019;16:284-94. [Crossref] [PubMed]