Long non-coding RNA expression profiles in peripheral blood mononuclear cells of patients with coronary artery disease
Introduction
Cardiovascular disease is a leading cause of death, with more than 7 million people dying from coronary artery disease (CAD) annually (1,2). Although great progress in the field of percutaneous coronary intervention and stent implantation, the number of serious treatment-related adverse events and mortality continue to grow worldwide. It is important for physicians to identify individuals who are at high risk of CAD and to develop appropriate prevention strategies (3,4). There are a number of well-established CAD risk factors, including age, sex, smoking, alcohol use, and comorbidities, such as diabetes mellitus, dyslipidemia, hypertensive disease, and atherosclerotic vascular disease (5). Despite increased awareness of risk factors for CAD, the molecular pathogenesis of CAD is complex and not well understood (6-8). Presently, clinicians use several biomarkers for the differential diagnosis of CAD, mainly including Cardiac troponin I, Cardiac troponin T, creatine kinase-MB and heart fatty acid binding protein. The value of these biomarkers in clinical assessment of CAD risk and decision on CAD treatment is not satisfactory, which is partly due to the clinical symptoms of CAD varies greatly. Therefore, there is an urgent need to explore non-invasive biomarkers for the diagnosis and evaluation of CAD in the early stages (9-11).
Long non-coding RNAs (lncRNAs) are transcripts at least 200 nucleotides in length with little or no coding potential, but exhibit a variety of important biologic processes (12-15). In addition, it has been demonstrated that lncRNAs exist in human body fluid at measurable levels with high stability, and can accurately reflect the situation in vivo, indicating their potential use as biomarkers (16,17). These advances harbor the potential to better elucidate the complex pathological process of CAD (18-20). Several freely circulating lncRNAs have been related to distinct cardiovascular disorders, indicating that altered lncRNAs have a significant impact on the pathogenesis of CAD (19,21-23). CAD is usually caused by the abnormal activity of biological processes, such as cell motility, immune response, metabolism or inflammation response, and lncRNAs are directly or indirectly involved in it (7,9). Inflammation plays a crucial role in the development of CAD and peripheral blood mononuclear cells (PBMC) are one of the main type of immune cells. However, no studies to date have specifically focused on assessing the role of lncRNA on these aspects.
Therefore, the aim of the current study was to explore the expression of lncRNAs in PBMC of CAD patients by high throughput sequencing, and to identify lncRNAs as potential biomarkers for clinical and subclinical CAD. These findings could also provide a useful basis for exploring the role of lncRNAs in CAD progression. We present the following article in accordance with the MDAR reporting checklist (available at http://dx.doi.org/10.21037/jtd-20-3105).
Methods
Participants
A total of 40 CAD patients and 10 controls who underwent diagnostic coronary angiography at the Meizhou Peoples’ Hospital (Huangtang Hospital, Meizhou, Guangdong Province, China) between January 2016 and January 2018 were enrolled in the present study. CAD diagnosis was based on the coronary angiography, electrocardiogram and serological examination on the discharged medical records, according to the European Society of Cardiology/American College of Cardiology. The 50 participants were classified into 5 groups: stable angina (SA; n=10), unstable angina (UA; n=10), ST-segment elevation acute myocardial infarction (STEMI; n=10), non-STEMI (n=10), and non-obstructive coronary atherosclerosis (NOCA; n=10). NOCA on coronary angiography conformed was define as controls. Exclusion criteria were patients previously diagnosed with malignancies, stroke, valvular heart diseases, autoimmune diseases, or other malignant disease. To validate the lncRNA profiling data, another 120 patients and 30 controls were also included. Demographic information, medical history, and medication data of all participants were obtained by face-to-face or telephone interviews and recorded on the questionnaire. The study was conducted in accordance with the Declaration of Helsinki (as revised in 2013). The present study was approved by the Ethics Committee for Research of Meizhou Peoples’ Hospital (No. MPH-HEC 2015-A-23). Signed informed consent was obtained from each participant at the time of enrollment.
RNA isolation
A total of 10 mL ethylenediaminetetraacetic acid-anticoagulated venous blood samples were collected from all participants. The sample was centrifuged at 3,000 ×g for 15 min, and supernatants were again centrifuged at 10,000 ×g for 20 min. The plasma interlayer was carefully transferred, aliquoted, and stored at –80 °C until use. Total RNA was extracted using the miRNeasy Mini Kit (Qiagen, Hilden, Germany), following the manufacturer’s protocols. The total RNA concentration and protein contamination were assessed using the NanoPhotometer spectrophotometer (Implen, Los Angeles, CA, USA). RNA quality and integrity were estimated using the Agilent Bioanalyzer 2100 (Agilent Technologies, Santa Clara, CA, USA).
LncRNA sequencing
Preparation for lncRNA sequencing library was based on the manufacturer’s standard protocols. In summary, ribosomal RNA was depleted from total RNA after enzymatic fragmentation by using the Ribo-Zero rRNA removal kit (EPICENTRE Biotechnologies, Madison, WI, USA). The resulting RNA pellet was then washed and recovered by alcohol precipitation. Libraries with high-strand specificity for sequencing were analyzed using the mRNA-ONLY Eukaryotic mRNA Isolation Kit (EPICENTRE Biotechnologies, USA). Samples for which the RNA integrity number was at least 8 were used to prepare a library of template molecules suitable for subsequent sequencing. The Illumina Hiseq 4000 sequencer (Illumina Inc., San Diego, CA, USA) was applied for sequencing, and a read length of 150 bp paired end was generated according to the standard protocol supplied by the manufacturer.
Mapping and identification of differently expressed genes
The sequencing raw reads were first converted into FASTQ format to filter out dirty raw reads, including sequence adaptors, reads containing ploy-N, and low-quality reads, prior to data analysis (24). The sequence reads were mapped onto the assembled genome, and transcript quantification and identification of differential gene expression was performed using the default parameters in Bowtie version 2.0.6 (http://bowtie-bio.sourceforge.net/index.shtml), TopHat version 2.0.9 (http://ccb.jhu.edu/software/tophat/index.shtml), and Cufflinks version 2.1.1 (http://cufflinks.cbcb.umd.edu/) (25). The read counts mapped to different isoforms of individual mRNA or lncRNA were pooled, and normalized and expressed as reads per kilobase per million mapped reads using the Cufflinks package. Differentially expressed genes were expressed as fold change with a false discovery rate (FDR) and corrected q-values. Only genes with an FDR <0.05 and |log2FC| >1 were identified as differentially expressed genes.
Functional enrichment analyses
Gene ontology (GO) enrichment analysis was performed to elucidate the biologic function of statistically significant genes, with GO annotations downloaded from GO (http://www.geneontology.org/) (26). Pathway analysis was used to analyze the potential functions in pathways where there was a significant enrichment of differentially expressed mRNAs based on the Kyoto Encyclopedia of Genes and Genomes (KEGG) database (http://www.genome.jp/kegg/pathway.html) (27). Fisher’s exact test was used in the GO and pathway analyses. Significance of data was set at P<0.05.
Quantitative real-time polymerase chain reaction (qRT-PCR)
LncRNA expression from the RNA sequencing data analysis was validated by qRT-PCR. A fixed volume of total RNA was subjected to reverse transcription using a PrimeScript RT Reagent Kit (Takara-Bio, Dalian, China) with a gDNA Eraser in accordance with the manufacturer’s protocol. The Premix Ex Taq II Reagent Kit (Takara-Bio, Dalian, China) and ABI 7500 Real-Time PCR thermocycler (Applied Biosystems, Carlsbad, CA, USA) were used for the qRT-PCR reactions. The primer sequences were listed in Table S1. Each reaction was conducted in triplicate. LncRNA expression was calculated using cycle threshold (Ct) values, and was internally normalized to glyceraldehyde 3-phosphate dehydrogenase using the 2−ΔΔCT equation method. All primer pairs are available upon request.
Statistical analysis
All statistical calculations were performed using SPSS version 19.0 (IBM, Armonk, NY, USA). Data for continuous variables are presented as means and standard deviations, and data for categorical variables are presented as numbers and percentages. The one-way analysis of variance comparison method was used to assess differences between different groups. P<0.05 indicated a statistically significant difference.
Results
Baseline clinical characteristics
A total of 160 CAD patients and 40 NOCA subjects were enrolled in the present. Characteristics of the study population are listed in Table 1. Statistically significant differences were not observed among the groups in terms of smoking, drinking, clopidogrel, and calcium blockers, as well as the level of systolic blood pressure (BP), diastolic BP, triglycerides, high-density lipoprotein cholesterol, and low-density lipoprotein cholesterol.
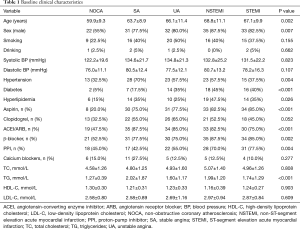
Full table
Quality assessment of lncRNA sequencing data
The cDNA sequencing libraries were constructed and sequenced through the Illumina HiSeq 4000 platform. Approximately 11.42 Gb sequence data were generated with the Q30, and averaged 94.14–95.32%, indicating high-quality data. Next, the clean reads were aligned to the human reference genome using TopHat version 2.0, where more than 93.96% of the total reads were useable reads that aligned uniquely to the reference genome with high uniformity. Detailed information on data quality and the mapping rate were summarized in Tables S2 and S3.
Four different algorithms including CPC (Coding Potential Calculator), CPAT (Coding Potential Assessment Tool), PFAM (Protein Family) and CNCI (Coding-Non-Coding Index) for calculating highly reliable potential non-coding transcripts were used. In the analysis, 2,925 candidate lncRNAs were detected, including 1,746 lincRNAs (59.7%) and 1,179 anti-sense lncRNAs (40.3%) (Figure 1). Compared with lncRNAs, mRNA expression levels were generally higher (Figure 2A). In addition, significant differences in length, open reading frame length and exon number between mRNAs and lncRNAs were also observed (Figure 2B,C,D).
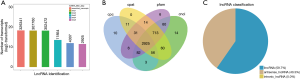
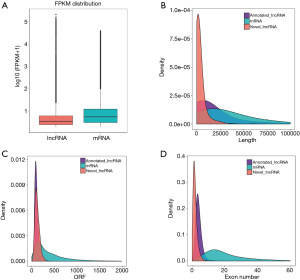
LncRNA expression profiles in PBMC
To specifically examine the entire repertoire of lncRNAs in PBMC, we determined the lncRNA expression profile from the PBMC of 40 CAD patients and 10 NOCA subjects. As shown in Figure 3, the unsupervised hierarchical clustering of the lncRNA and mRNA expression profiles showed that the overall changes from normal to clinical CAD were separated (upregulated genes are in red and downregulated genes are in green). These findings indicate an underlying dynamic change in gene expression signatures according to stage at diagnosis during CAD development.
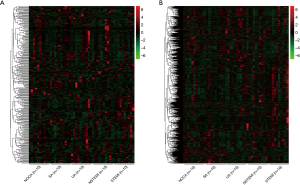
The Volcano plot of differential lncRNA expression in CAD subclinical patients and NOCA subjects are shown in Figure 4. Of these, the expressions of 83 lncRNAs were up-regulated, whereas the expressions of 114 lncRNAs were downregulated (P<0.05). In addition, a horizontal comparison of lncRNA expression was further performed. As shown in Figure 5, the change in the expression profile of 48 lncRNAs was found to be consistent with the degree of CAD, and could be used as potential biomarkers.
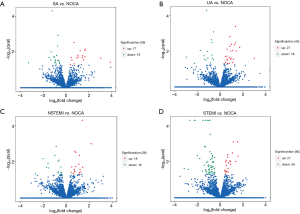
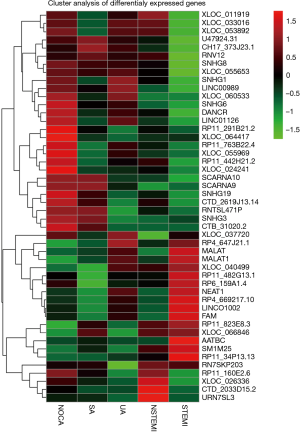
GO and KEGG pathway analyses
GO analysis was performed for gene and gene product enrichment based on biologic process, cellular component, and specific molecular function (Figure 6). We found that dysregulated lncRNA transcripts are associated with chromatin organization (ontology: biologic process), cell and cell part (ontology: cellular component), and protein heterodimerization activity (ontology: molecular function). In addition, KEGG pathway analysis was further performed; the findings indicated that the pathways mainly included viral carcinogenesis, systemic lupus erythematosus and alcoholism (Figure 7).
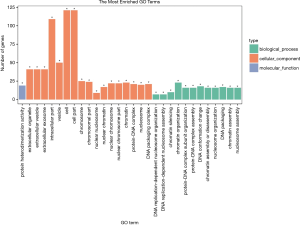
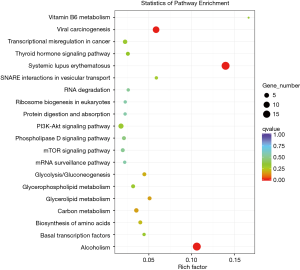
Evaluation of lncRNA expression via qRT-PCR analysis
To validate the expression levels of lncRNA identified through sequencing, 3 upregulated lncRNAs (RN7SKP203, SMIM25, and RP11-482G13.1) and 3 downregulated lncRNAs (RP11-763B22.4, XLOC_055653, and RN7SL471P) with only 1 transcript were randomly selected and evaluated. As shown in Figure 8, there was consistency between the qRT-PCR and RNA sequencing analysis results in the expression of 6× lncRNAs detected in CAD patients compared with NOCA subjects.
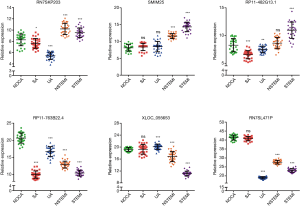
Discussion
CAD is a leading cause of death, and often presents as a complex systemic disease. Despite significant advances in the prevention and treatment of CAD, including antiplatelet agents, anticoagulants, lipid-lowering therapy, and percutaneous coronary intervention, the prevalence of CAD is still increasing rapidly (2,28-30). The identification of novel biomarkers could improve the management of CAD patients (7,31). Several lncRNAs have been shown to be involved in the physiological and pathophysiological processes of CAD, and have the potential to be used as biomarkers (10,32). However, the majority of them remain largely unknown. In the present study, we performed a systematic sequencing analysis of differentially expressed lncRNAs in different clinical CAD subtypes. Our findings indicated that the expression levels of lncRNAs and mRNAs significantly changed in the PBMC between the 40 CAD patients and 10 NOCA subjects. Interestingly, the expression levels of some lncRNAs were altered depending on the stage of subclinical coronary atherosclerosis.
LncRNAs are non-coding transcripts with a length >200 nt and lack protein-coding capacity. They have been found to play an important role in various important biologic processes and diverse human diseases (6,33). Although the functions of lncRNAs involved in the physiological and pathological processes of CAD are not fully understood, the findings of several previously published studies indicate their beneficial effects on cardiovascular disease. LncRNA-p21 enhances p53 activity and promotes the progression of atherosclerosis, including vascular intimal hyperplasia, vascular smooth muscle cell proliferation, and apoptosis (34). Smooth muscle enriched lncRNA (SMILR) has been identified as a driver of vascular smooth muscle cell proliferation, which may be a novel therapeutic strategy for vascular pathologies (35). LncRNA antisense non-coding RNA in the INK4 locus (ANRIL) has been identified as a prototype of a cirRNA regulating ribosome biogenesis and might promote antiatherogenic cell function (36). The lncRNA metastasis-associated lung adenocarcinoma transcript 1 (MALAT1) has been found to be involved in regulating endothelial cell function and angiogenesis (37), whereas the smooth muscle and endothelial cell-enriched migration/differentiation-associated long non-coding RNA (SENCR) mediates a repressive effect on the migration of smooth muscle cells (38).
In the present study, we investigated the expression profiles of lncRNAs in PBMC in the CAD subtypes of a Chinese population. We identified 83 upregulated and 114 downregulated lncRNAs that were differentially expressed between CAD patients and NOCA subjects. The expression profiles of lncRNAs in PBMC were found to alter depending on CAD stage. Our findings support those of previously published studies, in that lncRNA MALAT1 and KCNQ1OT1 expression levels increased, whereas ANRIL and long intergenic non-coding RNA predicting cardiac remodeling expression levels decreased (19,39,40). The lncRNA myocardial infarction-associated transcript (MIAT) has been reported as a biomarker for acute myocardial infarction (AMI), and our findings indicated that the MIAT expression level in patients with myocardial infarction and NOCA subjects was equivalent. Nevertheless, there was altered expression in the setting of AMI, and more specifically, patients with ST had lower levels of MIAT compared to those with non-ST, our results are consistent with the literature (40). These results indicate that these differentially expressed lncRNAs might be implicated in the development and progression of CAD; however, the molecular regulation remains to be elucidated. The altered expression levels of other lncRNAs, such as SENCR and SMILR, which have been reported to be associated with CAD, did not seem to be statistically significant in our study (35,38,41). The probable reason for the heterogeneity of the results may be because the lncRNAs detected differed between studies, whereas the lncRNAs in the study were mainly from PBMC. Therefore, lncRNA changes in PBMC could be related to the development and progression of these diseases. Our results provide supplementary data in this field.
The qRT-PCR results were in agreement with the initial RNA sequencing data in the present study. Interestingly, the upregulated or downregulated expression profiles observed in 48 lncRNAs were in accordance with the subclinical CAD stages. We speculated that these lncRNAs and their co-expressed mRNAs may play an important in CAD development and progression. In addition, the findings of our study indicated the potential of these lncRNAs as candidate biomarkers in clinical use. However, future studies on the molecular mechanism of these lncRNAs are warranted.
Despite providing informative expression profiles of lncRNAs, the present study has several limitations that need to be acknowledged. The participants in our study were from a single center with a high geographic concentration of participants, and the data should be extrapolated to other regions and ethnic groups with caution. Therefore, these results need to be validated using a more extensive population. Also, lncRNA mechanisms in CAD have not been fully elucidated, and further research is warranted to elucidate their biologic functions.
Conclusions
Our preliminary findings indicate that lncRNAs could be used as potential biomarkers of subclinical cardiac abnormalities in the PBMC of CAD patients using the RNA sequencing approach. Our data might provide valuable insight into transcriptome and related regulatory mechanisms in CAD progression. However, further studies are needed to verify our findings and hypothesis.
Acknowledgments
Funding: The present study was supported by the Science and Technology Program of Meizhou (grant no. 2018B024) and the Key Scientific and Technological Project of Meizhou People’s Hospital (grant no. MPHKSTP-20180101 and MPHKSTP-20170102).
Footnote
Reporting Checklist: The authors have completed the MDAR reporting checklist. Available at http://dx.doi.org/10.21037/jtd-20-3105
Data Sharing Statement: Available at http://dx.doi.org/10.21037/jtd-20-3105
Conflicts of Interest: All authors have completed the ICMJE uniform disclosure form (available at http://dx.doi.org/10.21037/jtd-20-3105). The authors have no conflicts of interest to declare.
Ethical Statement: The authors are accountable for all aspects of the work in ensuring that questions related to the accuracy or integrity of any part of the work are appropriately investigated and resolved. The study was conducted in accordance with the Declaration of Helsinki (as revised in 2013). The present study was approved by the Ethics Committee for Research of Meizhou Peoples’ Hospital (No. MPH-HEC 2015-A-23). Signed informed consent was obtained from each participant at the time of enrollment.
Open Access Statement: This is an Open Access article distributed in accordance with the Creative Commons Attribution-NonCommercial-NoDerivs 4.0 International License (CC BY-NC-ND 4.0), which permits the non-commercial replication and distribution of the article with the strict proviso that no changes or edits are made and the original work is properly cited (including links to both the formal publication through the relevant DOI and the license). See: https://creativecommons.org/licenses/by-nc-nd/4.0/.
References
- Cardiovascular disease in Europe 2016: an epidemiological update. Eur Heart J 2016;37:3182-3. [Crossref] [PubMed]
- Libby P, Bornfeldt KE, Tall AR. Atherosclerosis: Successes, Surprises, and Future Challenges. Circ Res 2016;118:531-4. [Crossref] [PubMed]
- Mathers CD, Loncar D. Projections of global mortality and burden of disease from 2002 to 2030. PLoS Med 2006;3:e442. [Crossref] [PubMed]
- Khyzha N, Alizada A, Wilson MD, et al. Epigenetics of Atherosclerosis: Emerging Mechanisms and Methods. Trends Mol Med 2017;23:332-47. [Crossref] [PubMed]
- Mack M, Gopal A. Epidemiology, Traditional and Novel Risk Factors in Coronary Artery Disease. Heart Fail Clin 2016;12:1-10. [Crossref] [PubMed]
- Wang K, Liu CY, Zhou LY, et al. APF lncRNA regulates autophagy and myocardial infarction by targeting miR-188-3p. Nat Commun 2015;6:6779. [Crossref] [PubMed]
- Voelter-Mahlknecht S. Epigenetic associations in relation to cardiovascular prevention and therapeutics. Clin Epigenetics 2016;8:4. [Crossref] [PubMed]
- Agarwal MA, Garg L, Lavie CJ, et al. Impact of family history of coronary artery disease on in-hospital clinical outcomes in ST-segment myocardial infarction. Ann Transl Med 2018;6:3. [Crossref] [PubMed]
- Devaux Y, Zangrando J, Schroen B, et al. Long noncoding RNAs in cardiac development and ageing. Nat Rev Cardiol 2015;12:415-25. [Crossref] [PubMed]
- Thum T, Condorelli G. Long noncoding RNAs and microRNAs in cardiovascular pathophysiology. Circ Res 2015;116:751-62. [Crossref] [PubMed]
- Souza PAL, Marcadenti A, Portal VL. Effects of Olive Oil Phenolic Compounds on Inflammation in the Prevention and Treatment of Coronary Artery Disease. Nutrients 2017;9:1087. [Crossref] [PubMed]
- Iyer MK, Niknafs YS, Malik R, et al. The landscape of long noncoding RNAs in the human transcriptome. Nat Genet 2015;47:199-208. [Crossref] [PubMed]
- Zhou Y, Wang X, Zhu X, et al. Bioinformatic analysis of long non-coding RNA-associated competing endogenous RNA network in adrenocortical carcinoma. Transl Cancer Res 2019;8:2175-86. [Crossref]
- Reon BJ, Dutta A. Biological Processes Discovered by High-Throughput Sequencing. Am J Pathol 2016;186:722-32. [Crossref] [PubMed]
- Vennin C, Adriaenssens E.. Long non-coding RNA and messenger RNA—the meeting of two worlds. J Thorac Dis 2018;10:544-6. [Crossref] [PubMed]
- Jarroux J, Morillon A, Pinskaya M. History, Discovery, and Classification of lncRNAs. Adv Exp Med Biol 2017;1008:1-46. [Crossref] [PubMed]
- Zhang Y, Sun L, Xuan L, et al. Reciprocal Changes of Circulating Long Non-Coding RNAs ZFAS1 and CDR1AS Predict Acute Myocardial Infarction. Sci Rep 2016;6:22384. [Crossref] [PubMed]
- Ishii N, Ozaki K, Sato H, et al. Identification of a novel non-coding RNA, MIAT, that confers risk of myocardial infarction. J Hum Genet 2006;51:1087-99. [Crossref] [PubMed]
- Kumarswamy R, Bauters C, Volkmann I, et al. Circulating long noncoding RNA, LIPCAR, predicts survival in patients with heart failure. Circ Res 2014;114:1569-75. [Crossref] [PubMed]
- de Gonzalo-Calvo D, Kenneweg F, Bang C, et al. Circulating long-non coding RNAs as biomarkers of left ventricular diastolic function and remodelling in patients with well-controlled type 2 diabetes. Sci Rep 2016;6:37354. [Crossref] [PubMed]
- Yang Y, Cai Y, Wu G, et al. Plasma long non-coding RNA, CoroMarker, a novel biomarker for diagnosis of coronary artery disease. Clin Sci (Lond) 2015;129:675-85. [Crossref] [PubMed]
- Wu T, Wu HD, Xu ZX, et al. Abnormal expression of long non-coding RNAs in myocardial infarction. Heart Vessels 2017;32:1253-61. [Crossref] [PubMed]
- Kiliszek M, Szpakowicz A, Franaszczyk M, et al. The 9p21 polymorphism is linked with atrial fibrillation during acute phase of ST-segment elevation myocardial infarction. Heart Vessels 2016;31:1590-4. [Crossref] [PubMed]
- Cock PJ, Fields CJ, Goto N, et al. The Sanger FASTQ file format for sequences with quality scores, and the Solexa/Illumina FASTQ variants. Nucleic Acids Res 2010;38:1767-71. [Crossref] [PubMed]
- Trapnell C, Williams BA, Pertea G, et al. Transcript assembly and quantification by RNA-Seq reveals unannotated transcripts and isoform switching during cell differentiation. Nat Biotechnol 2010;28:511-5. [Crossref] [PubMed]
- Young MD, Wakefield MJ, Smyth GK, et al. Gene ontology analysis for RNA-seq: accounting for selection bias. Genome Biol 2010;11:R14. [Crossref] [PubMed]
- Kanehisa M, Araki M, Goto S, et al. KEGG for linking genomes to life and the environment. Nucleic Acids Res 2008;36:D480-4. [Crossref] [PubMed]
- Laufs U, Karmann B, Pittrow D. Atorvastatin treatment and LDL cholesterol target attainment in patients at very high cardiovascular risk. Clin Res Cardiol 2016;105:783-90. [Crossref] [PubMed]
- Davi G, Patrono C. Platelet activation and atherothrombosis. N Engl J Med 2007;357:2482-94. [Crossref] [PubMed]
- Jeong YH, Kim IS, Park Y, et al. Carriage of cytochrome 2C19 polymorphism is associated with risk of high post-treatment platelet reactivity on high maintenance-dose clopidogrel of 150 mg/day: results of the ACCEL-DOUBLE (Accelerated Platelet Inhibition by a Double Dose of Clopidogrel According to Gene Polymorphism) study. JACC Cardiovasc Interv 2010;3:731-41. [Crossref] [PubMed]
- Gomes CPC, Spencer H, Ford KL, et al. The Function and Therapeutic Potential of Long Non-coding RNAs in Cardiovascular Development and Disease. Mol Ther Nucleic Acids 2017;8:494-507. [Crossref] [PubMed]
- Busch A, Eken SM, Maegdefessel L. Prospective and therapeutic screening value of non-coding RNA as biomarkers in cardiovascular disease. Ann Transl Med 2016;4:236. [Crossref] [PubMed]
- Mercer TR, Mattick JS. Structure and function of long noncoding RNAs in epigenetic regulation. Nat Struct Mol Biol 2013;20:300-7. [Crossref] [PubMed]
- Wu G, Cai J, Han Y, et al. LincRNA-p21 regulates neointima formation, vascular smooth muscle cell proliferation, apoptosis, and atherosclerosis by enhancing p53 activity. Circulation 2014;130:1452-65. [Crossref] [PubMed]
- Ballantyne MD, Pinel K, Dakin R, et al. Smooth Muscle Enriched Long Noncoding RNA (SMILR) Regulates Cell Proliferation. Circulation 2016;133:2050-65. [Crossref] [PubMed]
- Holdt LM, Stahringer A, Sass K, et al. Circular non-coding RNA ANRIL modulates ribosomal RNA maturation and atherosclerosis in humans. Nat Commun 2016;7:12429. [Crossref] [PubMed]
- Michalik KM, You X, Manavski Y, et al. Long noncoding RNA MALAT1 regulates endothelial cell function and vessel growth. Circ Res 2014;114:1389-97. [Crossref] [PubMed]
- Bell RD, Long X, Lin M, et al. Identification and initial functional characterization of a human vascular cell-enriched long noncoding RNA. Arterioscler Thromb Vasc Biol 2014;34:1249-59. [Crossref] [PubMed]
- Holdt LM, Beutner F, Scholz M, et al. ANRIL expression is associated with atherosclerosis risk at chromosome 9p21. Arterioscler Thromb Vasc Biol 2010;30:620-7. [Crossref] [PubMed]
- Vausort M, Wagner DR, Devaux Y. Long noncoding RNAs in patients with acute myocardial infarction. Circ Res 2014;115:668-77. [Crossref] [PubMed]
- Zhang Z, Gao W, Long QQ, et al. Increased plasma levels of lncRNA H19 and LIPCAR are associated with increased risk of coronary artery disease in a Chinese population. Sci Rep 2017;7:7491. [Crossref] [PubMed]