Bioinformatics analysis of differentially expressed genes in tumor and paracancerous tissues of patients with lung adenocarcinoma
Introduction
Lung cancer is the most common and deadly malignant tumor in humans with an incidence and mortality rate of 11.6% and 18.4% respectively (1). Non-small cell lung cancers (NSCLC) are the most common type of lung cancer, of which lung adenocarcinoma is the main pathologic type, accounting for 40% of lung cancer (2). Most lung adenocarcinoma is identified in the late stage, and the 5-year survival rate is low (3), possibly because there is currently no specific biomarker to facilitate early diagnosis and prognosis prediction (3). A reliable prognostic biomarker would help assess prognosis, evaluate therapeutic effects, and clarify the mechanism of lung cancer. The gene chip is a systematic high-throughput method for detecting and analyzing differentially expressed genes in different tissues, which can help to find prognostic genes or biomarkers for cancers. With the rapid development and application of gene chip technology, a large amount of gene data have been accumulated and the best methods how to mine genes from gene data has become a hot research topic (4). In this study, we screened the core genes of lung adenocarcinoma by the bioinformatic analysis of gene chip data of tumor tissue and matched paracancerous tissue from the gene chip public database Gene Expression Omnibus (GEO). These core genes can be used to determine disease diagnosis, prognosis judgment, and the mechanism research of lung adenocarcinoma in the future. We present the following article in accordance with the MDAR reporting checklist (available at http://dx.doi.org/10.21037/jtd-20-3453).
Methods
Microarray data information
The GEO database (https://www.ncbi.nlm.nih.gov/) is a free and open public database. The data of three gene expression profiles (GSE7670, GSE27262, GSE32863) were downloaded from the database and included 26, 25, and 58 pairs of lung adenocarcinoma and matched paracancerous normal lung tissue samples, respectively. The study was conducted in accordance with the Declaration of Helsinki (as revised in 2013).
Screening of differentially expressed genes (DEGs)
Data of the three profiles were analyzed using an online analysis program based on the R language, GEO2R. Venn software was used to analyze the differential genes of the profiles, and commonly up- and down-regulated differential genes were obtained.
Gene ontology analysis (GO) and Kyoto Encyclopedia of gene and genome (KEGG) pathways analysis
The common DEGs selected from the above three groups were uploaded to the online analysis platform DAVID (https://david.ncifcrf.gov/) for GO and KEGG pathway analysis. The encoded proteins of DEGs were analyzed by molecular function (MF), cellular component (CC), and biological process (BP) to study their GO functional and pathway enrichment. Differences with P<0.05 were considered statistically significant.
Protein-protein interaction (PPI) network and core gene screening
A PPI network was constructed by analyzing the PPI relationship between DEGs using the STRING database, and the threshold condition was a confidence score ≥0.4 (5). The results of analyzed data were then imported into Cytoscape for visualization analysis, and node connectivity was calculated with the plug-in MCODE to screen for the central node of the network. Genes corresponding to the central node were considered core genes.
Validation and survival analysis of core genes
GEPIA (http://gepia.cancer-pku.cn/) is an online website tool based on the TCGA and GTEX databases (6) and was used to further verify the core genes. The Kaplan-Meier plotter is an online deposit of the survival analysis data of EGA, TCGA, and GEO (Affymetrix microarrays only) databases. In this study, the Kaplan-Meier plotter was used to perform survival analysis for the core genes.
Statistical analysis
Differentially expressed genes with an adjusted P value <0.05 and |logFC| >2 were considered statistically significant by using t-test. The Kaplan-Meier plotter was used to perform survival analysis for the core genes. Differences with P<0.05 were considered statistically significant.
Results
Identification of DEGs in lung adenocarcinoma
The three data sets consisted of 109 pairs of lung adenocarcinoma and matched paracancerous normal lung tissues. The GEO2R analysis showed 108 up-regulated genes and 198 down-regulated genes in GSE7670, 145 up-regulated genes and 329 down-regulated genes in GSE27262, and 31 up-regulated genes and 156 down-regulated genes in GSE32863. A Venn diagram was created according to the different gene expression patterns of the three data sets, and 83 common differentially expressed genes were finally obtained (Figure 1). Compared with paracancerous tissues, there were 16 up-regulated genes (logFC >2) and 67 down-regulated genes (logFC <−2) in lung adenocarcinoma tissues (Table 1 and Figure 1).
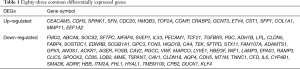
Full table
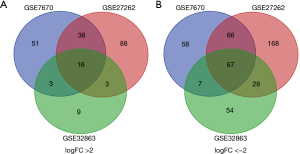
GO and KEGG pathway analysis
The GO enrichment analysis of 83 differentially expressed genes on the DAVID website showed differentially expressed genes mainly participated in the following processes: position regulation of transcription from RNA polymer II promoter, cell adhesion, angiogenesis, and position regulation of gene expression of the BP; extracellular exosome, plasma membrane, extracellular space, and extracellular region of the CC; calcium ion binding, heparin binding, protein kinase binding, and cytokine activity of MF (Table 2).

Full table
KEGG analysis showed that the differentially expressed genes were mainly enriched in six signaling pathways, including the PI3K-Akt signaling pathway, malaria, focal adhesion, ECM-receptor interaction, cell adhesion molecules (CAMs), and leukocyte transendothelial migration (Table 3).
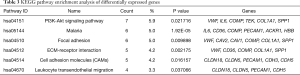
Full table
Construction of PPI network and screening of core genes
Eighty-three differentially expressed genes were imported into the STRING database to construct the PPI network, and 60 differentially expressed genes were incorporated into the PPI network complex (Figure 2A). Cytotype MCODE software further identified 11 core genes (Figure 2B) in the PPI network complex, three of which were up-regulated (COMP, SPP1, COL1A1) and eight of which were down-regulated (CDH5, CAV1, CLDN5, LYVE1, IL6, VWF, TEK, PECAM1).
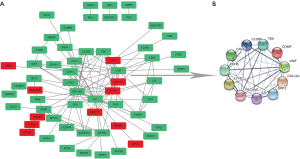
Validation of core genes with GEPIA tumor database
Query of the GEPIA tumor database identified that COMP, SPP1, and COL1A1 were significantly higher in lung adenocarcinoma than in normal lung tissues (Figure 3A,B,C), while CDH5, CAV1, CLDN5, LYVE1, IL6, VWF, TEK, and PECAM1 were significantly lower in lung adenocarcinoma (Figure 3D,E,F,G,H,I,J,K). These results were consistent with the previous GEO2R analysis.
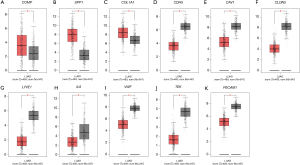
Relationship between core genes and patient prognosis
Survival analysis performed using the Kaplan-Meier plotter showed that the expression of 11 core genes (COMP, SPP1, COL1A1, CDH5, CAV1, CLDN5, LYVE1, IL6, VWF, TEK, PECAM1) was related to lung adenocarcinoma prognosis (P<0.05) (Figure 4A,B,C,D,E,F,G,H,I,J,K). Patients with a high expression of SPP1, COL1A1, LYVE1, and IL6, or, a low expression of COMP, CDH5, CAV1, CLDN5, VWF, TEK, and PECAM1, had a worse prognosis (Figure 4A,B,C,D,E,F,G,H,I,J,K).
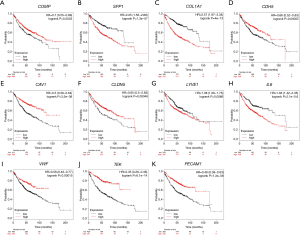
KEGG pathway analysis of core genes
The KEGG pathway enrichment of 11 core genes was reanalyzed using DAVID software. This showed that six genes (COMP, SPP1, COL1A1, IL6, VWF, TEK) were significantly enriched in the PI3K-Akt signaling pathway (P=1.62E-06, Table 4 and Figure 5).
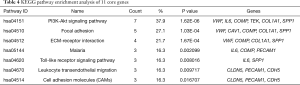
Full table
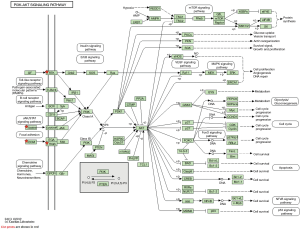
Discussion
In this study, three gene expression profiles (GSE7670, GSE27262, GSE32863) containing 109 pairs of lung adenocarcinoma and matched paracancerous normal lung tissues were analyzed. This revealed 83 common differential expression genes including 16 up-regulated and 67 down-regulated genes. The GO analysis showed that the differentially expressed genes were mainly involved in position regulation of transcription from RNA polymer II promoter, extracellular exosome, calcium ion binding, etc. KEGG analysis showed that differentially expressed genes were mainly enriched in the PI3K-Akt signaling pathway, malaria, etc. These data indicate that tumor pathogenesis is a complex biologic process driven by specific genes and epigenetic changes. Abnormal regulation of multiple genes can promote the occurrence and development of lung adenocarcinoma through different mechanisms.
To further screen core genes, a PPI network was constructed using the STRING database, visualized by Cytotype, and screened by MCODE plug-in. Finally, 11 core genes were identified, including three up-regulated genes (COMP, SPP1, and COL1A1) and eight down-regulated genes (CDH5, CAV1, CLDN5, LYVE1, IL6, VWF, TEK, and PECAM1). Among these, SPP1, CDH5, and VWF have been reported in other literature (7). These core genes were verified by the GEPIA tumor database. Survival analysis results obtained using the Kaplan-Meier plotter online website tool showed that expression of the 11 core genes was significantly related to lung adenocarcinoma prognosis: patients with a high expression of SPP1, COL1A1, LYVE1, and IL6, or, a low expression of COMP, CDH5, CAV1, CLDN5, VWF, TEK, and PECAM1 had a worse prognosis. Many cancers have specific diagnostic and prognostic markers. For example, alpha-fetoprotein (AFP) is a marker for liver cancer (8), while prostate-specific antigen (PSA) is a marker for prostate cancer (9). However, lung cancer lacks specific diagnostic and prognostic markers. Among 11 core genes, three were up-regulated (COMP, SPP1, COL1A1) and survival analysis showed that patients with a high expression of SPP1 and COL1A1 had a worse prognosis. Previous studies reported that the GRGDS sequence of SPP1 is related to cell adhesion and promotes tumor cell metastasis and invasion (10), which may explain why the prognosis of patients with high expressions of SPP1 is worse. COL1A1 is an important strand of the type I collagen triple helix, which is closely related to tumors and acts as a carcinogenic gene to promote tumor cell growth (11-13). Mori et al. found that COL1A1 plays an important role in differentiation and metastasis of human bladder cancer (14). Liu et al. confirmed that COL1A1 mediates breast cancer metastasis and is a potential therapeutic target for breast cancer (15). In addition, COL1A1 is an important marker of hepatocarcinogenesis and development (16). However, there are few studies concerning the role of COL1A1 in lung cancer. Further study on the role of SPP1 and COL1A1 is needed to confirm them as specific biomarkers for the diagnosis and prognosis judgment of lung adenocarcinoma.
The PI3K-Akt signaling pathway is an important signal pathway in the human body. This signaling pathway controls cellular BP of tumor development through a phosphorylation cascade of downstream proteins that affect cell proliferation, apoptosis, cell cycle regulation, and angiogenesis. Imbalance of the PI3K-Akt pathway plays an important role in the development of NSCLC as the PI3K-Akt pathway is activated in about 90% of NSCLC cell lines (17). Mutation of EGFR, PTEN deletion or mutation, and PI3K mutation or amplification can all activate the PI3K-Akt pathway, promote NSCLC tumor cell proliferation and migration, and lead to tumor progression (18). In this study, we re-analyzed the enrichment of the KEGG pathway of 11 core genes using DAVID software to show that six genes (COMP, SPP1, COL1A1, IL6, VWF, TEK) were significantly enriched in the PI3K Akt-signaling pathway. The results demonstrate that the occurrence and development of lung adenocarcinoma is closely related to the PI3K-Akt signaling pathway. Further study of the role of PI3K-Akt signaling in lung adenocarcinoma will provide new directions for understanding its mechanisms and promote the development of therapeutic targets.
With rapid developments in high-throughput technology, many experimental data related to lung adenocarcinoma have been obtained. In this study, existing public database and analysis tools were used to integrate multiple groups of lung adenocarcinoma data and conduct unified processing. We identified specific genes and pathways related to the prognosis of lung adenocarcinoma, which will be helpful for the diagnosis, treatment, prognosis judgement, and in-depth study of the mechanisms of this deadly disease in the future. The results also provide valuable information for future research and the development of new drugs. Nonetheless, this study has some limitations. Firstly, the experimental verification of core genes is lacking, and we plan to carry this out immediately. Secondly, the sample size was small and only included three data sets. Our own clinical data should be further supplemented to verify the findings in this study. Finally, only 720 lung adenocarcinoma samples were included in the survival analysis. Further experiments are needed to examine the specific molecular mechanisms of lung adenocarcinoma.
Acknowledgments
Funding: This research was funded by the National Natural Science Foundation of China (grant number: 31700690), and the Natural Science Foundation of Zhejiang Province, China (grant number: LQ18H180002).
Footnote
Reporting Checklist: The authors have completed the MDAR reporting checklist. Available at http://dx.doi.org/10.21037/jtd-20-3453
Conflicts of Interest: All authors have completed the ICMJE uniform disclosure form (available at http://dx.doi.org/10.21037/jtd-20-3453). The authors have no conflicts of interest to declare.
Ethical Statement: The authors are accountable for all aspects of the work in ensuring that questions related to the accuracy or integrity of any part of the work are appropriately investigated and resolved. The study was conducted in accordance with the Declaration of Helsinki (as revised in 2013).
Open Access Statement: This is an Open Access article distributed in accordance with the Creative Commons Attribution-NonCommercial-NoDerivs 4.0 International License (CC BY-NC-ND 4.0), which permits the non-commercial replication and distribution of the article with the strict proviso that no changes or edits are made and the original work is properly cited (including links to both the formal publication through the relevant DOI and the license). See: https://creativecommons.org/licenses/by-nc-nd/4.0/.
References
- Bray F, Ferlay J, Soerjomataram I, et al. Global cancer statistics 2018: GLOBOCAN estimates of incidence and mortality worldwide for 36 cancers in 185 countries. CA Cancer J Clin 2018;68:394-424. [Crossref] [PubMed]
- Chiba R, Morikawa N, Sera K, et al. Elemental and mutational analysis of lung tissue in lung adenocarcinoma patients. Transl Lung Cancer Res 2019;8:S224-34. [Crossref] [PubMed]
- Lu T, Yang X, Huang Y, et al. Trends in the incidence, treatment, and survival of patients with lung cancer in the last four decades. Cancer Manag Res 2019;11:943-53. [Crossref] [PubMed]
- Wu X, Li X, Fu Q, et al. AKR1B1 promotes basal-like breast cancer progression by a positive feedback loop that activates the EMT program. J Exp Med 2017;214:1065-79. [Crossref] [PubMed]
- Szklarczyk D, Morris JH, Cook H, et al. The STRING database in 2017: quality-controlled protein-protein association networks, made broadly accessible. Nucleic Acids Res 2017;45:D362-68. [Crossref] [PubMed]
- Tang Z, Li C, Kang B, et al. GEPIA: a web server for cancer and normal gene expression profiling and interactive analyses. Nucleic Acids Res 2017;45:W98-102. [Crossref] [PubMed]
- Piao J, Sun J, Yang Y, et al. Target gene screening and evaluation of prognostic values in non-small cell lung cancers by bioinformatics analysis. Gene 2018;647:306-11. [Crossref] [PubMed]
- Kanwal F, Singal AG. Surveillance for Hepatocellular Carcinoma: Current Best Practice and Future Direction. Gastroenterology 2019;157:54-64. [Crossref] [PubMed]
- Fenton JJ, Weyrich MS, Durbin S, et al. Prostate-Specific Antigen-Based Screening for Prostate Cancer: Evidence Report and Systematic Review for the US Preventive Services Task Force. JAMA 2018;319:1914-31. [Crossref] [PubMed]
- Oldberg A, Franzen A, Heinegard D. Cloning and sequence analysis of rat bone sialoprotein (osteopontin) cDNA reveals an Arg-Gly-Asp cell-binding sequence. Proc Natl Acad Sci U S A 1986;83:8819-23. [Crossref] [PubMed]
- Oleksiewicz U, Liloglou T, Tasopoulou KM, et al. COL1A1, PRPF40A, and UCP2 correlate with hypoxia markers in non-small cell lung cancer. J Cancer Res Clin Oncol 2017;143:1133-41. [Crossref] [PubMed]
- Li J, Ding Y, Li A. Identification of COL1A1 and COL1A2 as candidate prognostic factors in gastric cancer. World J Surg Oncol 2016;14:297. [Crossref] [PubMed]
- Zhang Z, Wang Y, Zhang J, et al. COL1A1 promotes metastasis in colorectal cancer by regulating the WNT/PCP pathway. Mol Med Rep 2018;17:5037-42. [Crossref] [PubMed]
- Mori K, Enokida H, Kagara I, et al. CpG hypermethylation of collagen type I alpha 2 contributes to proliferation and migration activity of human bladder cancer. Int J Oncol 2009;34:1593-602. [PubMed]
- Liu J, Shen JX, Wu HT, et al. Collagen 1A1 (COL1A1) promotes metastasis of breast cancer and is a potential therapeutic target. Discov Med 2018;25:211-23. [PubMed]
- Ma HP, Chang HL, Bamodu OA, et al. Collagen 1A1 (COL1A1) Is a Reliable Biomarker and Putative Therapeutic Target for Hepatocellular Carcinogenesis and Metastasis. Cancers (Basel) 2019;11:786. [Crossref] [PubMed]
- Zhao R, Chen M, Jiang Z, et al. Platycodin-D Induced Autophagy in Non-Small Cell Lung Cancer Cells via PI3K/Akt/mTOR and MAPK Signaling Pathways. J Cancer 2015;6:623-31. [Crossref] [PubMed]
- Li X, Wu C, Chen N, et al. PI3K/Akt/mTOR signaling pathway and targeted therapy for glioblastoma. Oncotarget 2016;7:33440-50. [Crossref] [PubMed]
(English Language Editor: B. Draper)