Weighted miRNA co-expression network reveals potential roles of apoptosis related pathways and crucial genes in thoracic aortic aneurysm
Introduction
Thoracic aortic aneurysm (TAA) is a potentially life-threatening disease with an incidence of approximately 10 in 100,000 persons per year (1). Most patients with TAA are asymptomatic, and the major risk associated with TAA is aneurysm rupture, which is associated with high mortality (2). To date, surgical interventions, especially endovascular repairs, are the first-line procedure for treating TAA. However, such surgical procedures are often complicated (3). Thus, it is critically important to investigate the underlying molecular mechanisms of TAA and to identify potential targets for TAA treatment. The pathogenesis of TAA is complex and includes the apoptosis of vascular smooth muscle cells (VSMCs), chronic inflammation, and oxidative stress (4-6). Over the past decade, a number of studies have demonstrated that genetic factors play an important role in the initiation and progression of TAA (7-9). Based on a genome-wide analysis, the genes associated with pathologies of TAA, such as those associated with VSMC contractility, have been discovered (10).
Through transcriptome, miRNAs have been explored in TAA patients or mouse models. In a recent study, a miRNA microarray assay identified 232 differentially expressed miRNAs (DEMs), and miR-574-5p was confirmed and validated as a possible therapeutic target in serum, mouse, and cellular experiments (11). Another study using tissue and plasma samples with NanoString nCounter technology also revealed 3 miRNAs that might serve as biomarkers for ascending TAA patients (12). However, it is insufficient to focus on the differential expression status of miRNAs between TAA and control groups, as the potential interactions between the miRNAs and the crucial miRNAs that might mediate TAA pathogenesis also need to be explored. To address this, several methods have been developed to focus on genes with similar expression patterns that may participate in a specific pathogenesis. Of these methods, the most widely used is the weighted correlation network analysis (WGCNA) (13). WGCNA can be used to construct a weighted co-expression network for genes, detect gene modules, associate gene modules with clinical traits, and mine crucial genes that could potentially mediate important biological processes. Furthermore, WGCNA can also be applied to construct co-expression networks based on noncoding RNA, such as miRNA (14,15). The WGCNA has been used to construct gene co-expression networks and identify crucial genes mediating abdominal aortic aneurysm (AAA) based on mRNA expression data (16). In TAA, a miRNA-target derived from the Kyoto Encyclopedia of Genes and Genomes (KEGG) pathway network examined the role of Krüppel-like factor 4 in the pathogenesis of TAA (17). In a recently published study, WGCNA was conducted on mRNA expression data of AAA, TAA, and intracranial aneurysm. The results of this study identified conserved gene modules between different types of aneurysms. This study then mined hub genes, built miRNA-hub gene network using miRNet, and identified several crucial genes and miRNAs based on this network (18). However, to date, very few studies have directly constructed a weighted miRNA co-expression network by undertaking WGCNA in TAA.
In the present study, we constructed a co-expression work based on a miRNA data set of TAA (GSE110527). The DEMs were mapped into the whole miRNA co-expression network of TAA, and a DEM co-expression network was constructed. Crucial miRNAs were mined based on the DEM co-expression network. Target genes were predicted for crucial miRNAs, and were compared with differentially expressed genes (DEGs) of an mRNA data set of TAA (GSE26155). Overlapping target genes were identified as important target genes. Another independent mRNA data set (GSE9106) was used to validate these genes; the successfully validated genes were considered as crucial genes. Finally, a miRNA risk score was also calculated by performing least absolute shrinkage and selection operator (LASSO) regression to show the potential clinical significance of miRNA in a DEM co-expression network.
We present the following article in accordance with the MDAR checklist (available at http://dx.doi.org/10.21037/jtd-20-3601).
Methods
Medical ethics
The study was conducted in accordance with the Declaration of Helsinki (as revised in 2013). The raw data sets were obtained from the Gene Expression Omnibus (GEO) database (http://www.ncbi.nlm.nih.gov/geo/; GSE110527, GSE26155, and GSE9106). In this study, no human trials or animal experiments were conducted.
Data sets and data preprocessing
Three GEO data sets (GSE110527, GSE26155, and GSE9106) were included in our study. GSE110527 is a miRNA microarray data set containing 19 TAA and 19 normal thoracic aorta samples, and was used in the construction of miRNA co-expression network. GSE26155 and GSE9106 are 2 mRNA microarray data sets containing aortic samples and plasma samples, respectively. These 2 data sets were used for crucial mRNA mining. The series matrix files were downloaded from the GEO database, and all the data sets were converted into log2-transformed format before being subjected to further analysis. For the mRNA microarray data sets, the microarray platform data tables were also downloaded to annotate probe IDs in the series matrix files with a gene symbol.
DEM and DEG screening
The “limma” R package was used to conduct a DEM analysis for GSE110527, and a DEG analysis for GSE26155 and GSE9106. For the DEM analysis, a threshold of |log2(fold-change)|>1 and an adjusted P value of <0.01 were chosen. For the DEG analysis, a threshold of adjusted P<0.05 was chosen. To explore the biological processes and pathways in which these DEMs were involved, the DEMs were subjected to FunRich v3.1.3 for a functional enrichment analysis. For the DEGs, the “clusterProfiler” R package was used to conduct a functional enrichment analysis.
miRNA co-expression network construction
The “WGCNA” R package was used to create a miRNA co-expression network for the TAA samples. The miRNAs with the highest 25% variance were included in further analysis. A Pearson’s correlation matrix was calculated and transformed into an adjacency matrix using the following formula: amn = |cmn|β (where amn represents adjacency between miRNA m and miRNA n, cmn represents Pearson’s correlation coefficient between miRNA m and miRNA n, and β represents the soft threshold). The adjacency matrix stored the information of the whole network. To identify miRNA modules, a topological overlap matrix (TOM) was built based on the adjacency matrix, and a dynamic tree-cut algorithm was used to allocate miRNAs to different modules. The minimal module size was set at 100, and a threshold of 0.25 was selected to merge similar miRNA modules. FunRich was also used to conduct a functional enrichment analysis of the miRNAs of each module.
DEM co-expression network construction and crucial miRNA mining
We mapped DEMs into the whole co-expression network of all samples in Cytoscape v3.7.0 and created a DEM co-expression network. Molecular Complex Detection (MCODE), a plugin in Cytoscape that detects densely connected regions in a network, was applied to the DEM co-expression network. The most significant MCODE cluster was chosen. We considered miRNAs with top-10 degree in this cluster as crucial miRNAs.
Target prediction and crucial mRNA mining
To obtain an appropriate number of target genes for further mining and to minimize the false-positive rate, the miRTarbase database (http://mirtarbase.cuhk.edu.cn/php/index.php), a literature-based miRNA-target interactions prediction tool, was employed to predict the target genes of crucial miRNAs. The biological processes and pathways for these genes were also shown using a “clusterProfiler” package. After the prediction of the target genes, the overlap between these target genes and DEGs in GSE26155 were used to obtain the important target genes. Finally, another independent database was used to validate these important target genes; the successfully validated target genes were considered as crucial genes.
LASSO regression and the calculation of a miRNA risk score
The stability of the miRNAs raises the possibility of their being used as disease biomarkers (19). To further evaluate the potential diagnostic value of miRNAs, we adopted a machine-learning approach whereby we undertook a LASSO regression to calculate a miRNA risk score. Important variables with nonzero coefficients that can accurately predict disease status can be screened by a LASSO regression. The “glmnet()” function in the “glmnet” R package was used to conduct a LASSO regression to select which miRNAs would be the most useful in the prediction of TAA. To ensure the robustness of the selected variables, a 10-fold cross-validation was performed using the “cv.glmnet()” function. Based on the minimal 1-standard error (SE) criterion, the optimal λ was chosen to obtain the coefficients for the variables. A miRNA risk score was calculated through a linear combination of selected variables that were weighted by their corresponding coefficients. A receiver operating characteristics (ROC) analysis was undertaken to determine the diagnostic value of this miRNA risk score.
Statistical analysis
Data preprocessing, the DEM and DEG analyses, the WGCNA analysis, the functional enrichment analysis of the DEGs and the target genes, the LASSO regression and a cross-validation were conducted in R v.3.6.2 (The R Foundation for Statistical Computing). The details of these bioinformatic analyses are described in corresponding subsections, and codes for these analyses are available in the Supplementary Data. The ROC analysis was conducted using SPSS 25.0 (IBM Corp). A P value of <0.05 was considered significant.
Results
Flowchart of the whole study
Figure 1 shows a flowchart of our study. After data downloading and preprocessing, we conducted DEM, DEG, and WGCNA analyses. We mapped DEMs into the whole miRNA co-expression network of TAA and created a DEM co-expression network. MCODE was then used to detect the most significant cluster, and miRNAs with the top-10 degree were considered as crucial miRNAs. The miRTarbase database was used to find literature-based target genes of crucial miRNAs. Any genes that overlapped between the target genes and DEGs of GSE26155 were identified as important target genes. Another independent data set (GSE9106) was employed to validate these important target genes, and the successfully validated genes were considered as crucial genes. Finally, a LASSO regression was used to calculate a miRNA risk score to show the potential diagnostic value of miRNAs in the most significant MCODE cluster.
Screening of DEMs and DEGs
Using the threshold of |log2(fold-change)|>1 and adjusted P value of <0.01, a total of 165 DEMs were screened. Of these, 77 were up-regulated and 88 were down-regulated (Figure 2A,B). Table 1 shows DEMs with top-10 |log2(fold-change)| and the most significant genes and pathways interacting with DEMs. Figure 2C,D and Table S1 show the biological processes and pathways of all DEMs. For GSE26155 and GSE9160, a total of 1,965 and 2,368 DEGs were screened, respectively (Figure 3 and https://cdn.amegroups.cn/static/public/jtd-20-3601-1.xlsx). Figure S1 and https://cdn.amegroups.cn/static/public/jtd-20-3601-2.xlsx show the results of the functional enrichment analysis for the DEGs. Pathways related to apoptosis, such as the tumor necrosis factor (TNF)-related apoptosis-inducing ligand (TRAIL) signaling pathway, appeared in the top-6 significant pathways.
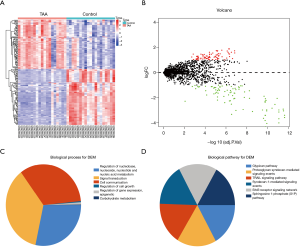
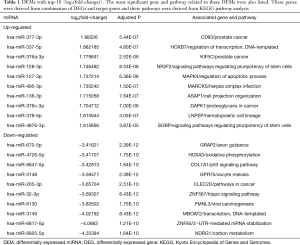
Full table
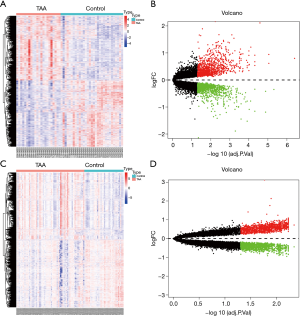
Construction of miRNA co-expression network
No outliers were excluded and all samples were involved in the network construction (Figure S2A). Using a cutoff of R2=0.9, a soft threshold of β=5 was chosen (Figure S2B,S2C). As the histogram and linear plot show, the network that we constructed met the requirement of a scale-free topology with an R2 of 0.91 (Figure S2D,S2E). The miRNA expression modules were then detected based on the TOM, and a total of 4 modules were obtained (Figure S2F). A functional enrichment analysis was conducted for the miRNAs in each module (Figure S3).
Mining of crucial miRNAs
DEMs were mapped into the whole co-expression network of all samples, and a DEM co-expression network was created (Figure S4). MCODE was employed to identify densely connected network regions, and the most significant MCODE cluster with 159 nodes and 12,388 edges was included in further analysis (Figure 4A). miRNAs with the top-10 degree in the MCODE cluster were considered as crucial miRNAs (Figure 4B).
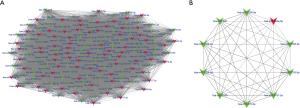
Target prediction and mining of crucial genes
The target genes of crucial miRNAs were predicted using the miRTarbase database (https://cdn.amegroups.cn/static/public/jtd-20-3601-3.xlsx), and a total of 994 target genes were predicted. Figure 5 and Table S2 show the Gene Ontology (GO) and KEGG pathway enrichment analyses of these genes. The regulation of the apoptotic signaling pathway (ASP) was the GO term with the highest count number. These genes were then compared with the DEGs of GSE26155, and 113 overlapping genes were identified (Figure 6A). These overlapping genes were validated using the DEGs of GSE9160, and 5 crucial genes were identified (Figure 6B and Table 2).
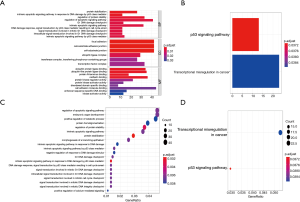
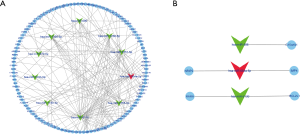
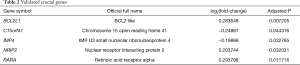
Full table
Calculating a miRNA risk score to predict TAA
A total of 11 miRNAs with a nonzero coefficient were screened by the LASSO regression (Figure S5A,S5B). Table 3 shows the areas under the curves (AUCs) of these 11 miRNAs. Notably, most of these miRNAs (8 of 11) had AUCs higher than 0.9. The risk score was calculated through a linear combination of the 11 miRNAs weighted by their coefficients (Table S3). An ROC analysis showed the potential diagnostic value of the miRNA risk score with an AUC of 1 (SE =6.73E-9, P=1.37E-7; Figure S5C).

Full table
Summary of main findings
In the present study, a DEM analysis was first conducted, and a total of 165 DEMs were screened. A miRNA co-expression network of TAA was then constructed and combined with DEMs to obtain a DEM co-expression network. MCODE was applied to detect densely connected regions in the DEM co-expression network, and miRNAs with the top-10 degree in the most significant MCODE cluster were selected as crucial miRNAs. A total of 994 target genes for crucial miRNAs were predicted, and 113 overlapping genes were identified after comparing target genes with the DEGs of GSE26155. Next, these genes were validated using another independent data set (GSE9106), and 5 crucial genes were identified. Finally, a miRNA risk score was constructed by a LASSO regression based on miRNAs in the MCODE cluster.
Discussion
The functional enrichment analysis showed that DEMs participate in multiple biological processes. The TRAIL signaling pathway was among the top-6 significant biological pathways for DEMs. The TRAIL signaling pathway is thought to be vital in the induction of cell apoptosis (20). Gonçalves et al. (21) showed that TRAIL activation induced the apoptosis of VSMCs in vitro. Consistent with previous studies, the findings of the present study suggest that TRAIL-induced apoptosis might play a role in TAA pathogenesis (22). Apoptosis-related pathways were also found in the functional enrichment analysis of target genes. The regulation of ASP is an enriched pathway with the highest count number. ASP can be divided into two categories: intrinsic and extrinsic ASP. Several pathways associated with intrinsic ASP were also found in the functional enrichment analysis of target genes. Intrinsic ASP could be induced by DNA damage, and p53 is the central effector molecule in DNA damage response (23). Previous research has shown that DNA damage-induced agents, such as chemotherapeutic drugs and irradiation, could cause apoptotic death through a p53-dependent pathway (24). Zhang et al. found that p53-dependent VSMC senescence plays a role in the development of TAA (25), and several studies have documented p53-dependent VSMC apoptosis in AAA (26,27). The second category of ASP is extrinsic ASP. The TRAIL pathway, which was shown in the biological pathway analysis of DEMs in our study, can initiate the extrinsic ASP through the formation of the death-inducing signaling complex, followed by the activation of effector caspases (28,29). The above-mentioned results emphasize the importance of gaining a comprehensive understanding of apoptosis in TAA pathogenesis.
By further screening important target genes and using an independent data set to validate these genes, 5 crucial genes were identified. Of these genes, the expression of B-cell lymphoma 2 like 1 (BCL2L1), retinoic acid receptor alpha (RARα), and nuclear receptor interacting protein 2 (NRIP2) were higher in the TAA group, and the expressions of the other 2 genes were lower with limited studies. The protein encoded by BCL2L1 belongs to the BCL2 protein family. Genes with varying degrees of homology to BCL2 have been identified that code for both antiapoptotic and proapoptotic proteins. As a result of alternative splicing, BCL2L1 has two isoforms (i.e., Bcl-xl and Bcl-xs). These two isoforms have opposite effects in regulating apoptosis. Bcl-xl is the longer isoform with antiapoptotic activity, while Bcl-xs is the shorter isoform with proapoptotic activity (30). The overexpression of Bcl-xs causes the mitochondrial activation of a caspase cascade through the release of cytochrome c, and antagonizes the antiapoptotic effects of Bcl-xl (31,32). Bcl-xs might play an important role in vascular diseases. Okura et al. found that platelet-derived growth factor (PDGF)-BB induced apoptosis of VSMCs in vitro, and caused a decrease in Bcl-xl and an increase in Bcl-xs (33). In an in vivo experiment, Bcl-xs was found to be up-regulated in balloon-injured carotid arteries in a rat model. These findings suggest that Bcl-xs might be a crucial mediator of VSMC apoptosis. Although Durdu et al. found that BCL2L1 was down-regulated in TAA compared with control group in immunohistochemical staining with a borderline P value (P=0.49) (31,32,34), the role of Bcl-xs in apoptosis still provided us with a new perspective to understand TAA pathogenesis.
RARα is a nuclear RAR that has been implicated in crucial biological processes, such as development, differentiation, and apoptosis (35). Fusion protein PML/RARα inhibits the differentiation and apoptosis of myeloid precursor cells, causing acute promyelocytic leukemia (APL) (36). Retinoids represent a promising treatment for APL. Indeed, Altucci et al. revealed that the all-trans retinoic acid, one of the RARα agonist, induced apoptosis in NB4 cells. Additionally, the activation of RARα has also been shown to induce the expression of TRAIL and thus cause apoptosis (37). Our findings that RARα was up-regulated in the TAA group and TRAIL signaling pathways in the DEM functional enrichment analysis suggest that RARα-induced apoptosis might also play a role in TAA pathogenesis.
NRIP2 belongs to the aspartic protease family, and can bind directly to the C-terminal ligand binding domain of mouse retinoic acid receptor β (RORβ) to inhibit the transcriptional activity of RORβ (38,39). Wen et al. found that NRIP2 could up-regulate the Wnt signaling pathway in colorectal cancer by targeting RORβ (40). Activated Wnt signaling pathway was observed in both TAA and AAA (37,41,42). It is not clear whether the Wnt pathway participates in TAA pathogenesis through apoptosis; however, studies have shown that the Wnt signaling pathway might be involved in aneurysm pathogenesis by inducing inflammation, up-regulating MMPs, and promoting endothelial cell senescence. It could be inferred that NRIP2 might participate in TAA pathogenesis by up-regulating the Wnt signaling pathway and possibly participate apoptosis.
The pathway analyses and the identification of the crucial genes showed that apoptosis might play an important role in TAA pathogenesis, with VSMCs also being implicated in this disease. VSMCs and elastic fibers are the main components of tunica media, and maintain the tensile strength and elasticity of the aorta (43). Additionally, as Shanahan summarized, VSMCs also serve as central mediators in vascular wall inflammation through crosstalk with endothelial cells and immune cells. VSMC apoptosis not only contributes to the destabilization of the vascular wall, but also enhances inflammation and oxidative stress and shifts the vascular microenvironment toward a proteolytic state. The roles of miRNA in VSMCs have been subjected to more investigation in the murine aneurysmal model. Notably, Woo et al. explored how the interactions between 30b-5p miRNA and MBNL1 mRNA participate in human coronary atherosclerosis by mediating VSMC differentiation (44) and similar studies should seek to test the role of VSMCs in human TAA.
Finally, we made a miRNA risk score system for TAA for which we obtained a quite good AUC value. Over the past decade, miRNAs have been accepted as potential diagnostic markers or prognostic biomarkers for diseases of different organs. For example, a recent study developed a novel prognostic signature that excellently predicted the 1- and 3-year survival rate of patients with glioma (45). Another study on pancreatic cancer established a risk score system using 7 miRNAs to predict overall survival and recurrence-free survival, which showed reliable performance with a high C-index (46). To the best of our knowledge, this study is the first to report a miRNA risk score system in TAA.
In the present study, we constructed a miRNA co-expression network in TAA for the first time, and identified crucial miRNAs based on a DEM co-expression network. The target genes of these crucial miRNAs were predicted using a literature-based miRNA-target interaction prediction tool (i.e., the miRTarbase database). Important target genes were identified as the overlapping genes of the target genes and DEGs in a TAA mRNA microarray data set. Then we validated these important target genes using another independent data set; the successfully validated genes were considered as crucial genes.
However, it should be noted that this study had a number of limitations. First, the 3 data sets used had some discrepancies in TAA localization and clinical causes. Detailed grouping information about these discrepancies was not provided, and we cannot conduct a subgroup analysis to examine the effect of the heterogeneity. Second, we made a compromise to choose GSE9106, which was based on plasma samples, as our validation set, as there were no other available or appropriate data sets using aortic tissue that could be used for validation (47). Third, to simultaneously obtain an appropriate number of target genes for further mining and minimize the false-positive rate, we only used one a single target gene prediction tool, although it’s literature-based. In addition, due to the lack of detailed clinical information in the GEO database we could not associate miRNA modules with clinical traits. Finally, while our study found that apoptosis might potentially play an important role in TAA pathogenesis based on miRNA co-expression network, other epigenetic mechanisms, such as epigenetic modulations in VSMCs by histone deacetylases, are also potential contributors to TAA pathogenesis and require further exploration (48).
Conclusions
We performed a WGCNA analysis to construct a weighted miRNA co-expression network, predicted target genes of crucial miRNAs, identified crucial genes, and finally calculated a miRNA risk score. Heterogeneity regarding TAA localization and clinical causes exists; however, our study still has implications for translational application, as TAAs share common pathophysiological features, such as the loss of VSMCs, extracellular matrix degradation, and proteoglycan deposition (43,49,50). Our study revealed that pathways and genes associated with apoptosis might play an important role in TAA pathogenesis, and medications targeting apoptosis might be promising therapies to slow TAA progression. Future in vitro and in vivo experimental studies need to be conducted to further validate our findings and investigate the mechanistic details of these crucial miRNAs and genes.
Acknowledgments
We thank English Language Editors L. Huleatt and J. Gray for their polishing of our manuscript.
Funding: This work was supported by the Natural Science Foundation of China (No. 81770481 and No. 51890894) and CIFMS 2017-I2M-1-008)/Chinese Academy of Medical Sciences, innovation Fund for Medical Sciences (CIFMS 2017-I2M-1-008). The funders had no role in the design of the study, data collection and analysis, decision to publish, or preparation of the manuscript.
Footnote
Reporting Checklist: The authors have completed the MDAR checklist. Available at http://dx.doi.org/10.21037/jtd-20-3601
Peer Review File: Available at http://dx.doi.org/10.21037/jtd-20-3601
Conflicts of Interest: All authors have completed the ICMJE uniform disclosure form (available at http://dx.doi.org/10.21037/jtd-20-3601). The authors have no conflicts of interests to declare.
Ethical Statement: The authors are accountable for all aspects of the work in ensuring that questions related to the accuracy or integrity of any part of the work are appropriately investigated and resolved. The study was conducted in accordance with the Declaration of Helsinki (as revised in 2013).
Open Access Statement: This is an Open Access article distributed in accordance with the Creative Commons Attribution-NonCommercial-NoDerivs 4.0 International License (CC BY-NC-ND 4.0), which permits the non-commercial replication and distribution of the article with the strict proviso that no changes or edits are made and the original work is properly cited (including links to both the formal publication through the relevant DOI and the license). See: https://creativecommons.org/licenses/by-nc-nd/4.0/.
References
- Elefteriades JA, Sang A, Kuzmik G, et al. Guilt by association: paradigm for detecting a silent killer (thoracic aortic aneurysm). Open Heart 2015;2:e000169 [Crossref] [PubMed]
- Erbel R, Aboyans V, Boileau C, et al. 2014 ESC Guidelines on the diagnosis and treatment of aortic diseases: Document covering acute and chronic aortic diseases of the thoracic and abdominal aorta of the adult. The Task Force for the Diagnosis and Treatment of Aortic Diseases of the European Society of Cardiology (ESC). Eur Heart J 2014;35:2873-926. [Crossref] [PubMed]
- Elefteriades JA, Farkas EA. Thoracic aortic aneurysm clinically pertinent controversies and uncertainties. J Am Coll Cardiol 2010;55:841-57. [Crossref] [PubMed]
- Nataatmadja M, West M, West J, et al. Abnormal extracellular matrix protein transport associated with increased apoptosis of vascular smooth muscle cells in marfan syndrome and bicuspid aortic valve thoracic aortic aneurysm. Circulation 2003;108:II329-34. [Crossref] [PubMed]
- He R, Guo DC, Sun W, et al. Characterization of the inflammatory cells in ascending thoracic aortic aneurysms in patients with Marfan syndrome, familial thoracic aortic aneurysms, and sporadic aneurysms. J Thorac Cardiovasc Surg 2008;136:922-9, 929.e1.
- Yang HH, van Breemen C, Chung AW. Vasomotor dysfunction in the thoracic aorta of Marfan syndrome is associated with accumulation of oxidative stress. Vascul Pharmacol 2010;52:37-45. [Crossref] [PubMed]
- Vapnik JS, Kim JB, Isselbacher EM, et al. Characteristics and Outcomes of Ascending Versus Descending Thoracic Aortic Aneurysms. Am J Cardiol 2016;117:1683-90. [Crossref] [PubMed]
- Ostberg NP, Zafar MA, Ziganshin BA, et al. The Genetics of Thoracic Aortic Aneurysms and Dissection: A Clinical Perspective. Biomolecules 2020;10:182. [Crossref] [PubMed]
- Li Y, Gao S, Han Y, et al. Variants of Focal Adhesion Scaffold Genes Cause Thoracic Aortic Aneurysm. Circ Res 2021;128:8-23. [Crossref] [PubMed]
- Prakash SK, LeMaire SA, Guo DC, et al. Rare copy number variants disrupt genes regulating vascular smooth muscle cell adhesion and contractility in sporadic thoracic aortic aneurysms and dissections. Am J Hum Genet 2010;87:743-56. [Crossref] [PubMed]
- Boileau A, Lino Cardenas CL, Courtois A, et al. MiR-574-5p: A Circulating Marker of Thoracic Aortic Aneurysm. Int J Mol Sci 2019;20:3924. [Crossref] [PubMed]
- Moushi A, Pillar N, Keravnou A, et al. MicroRNAs in ascending thoracic aortic aneurysms. Biosci Rep 2020;40:BSR20200218 [Crossref] [PubMed]
- Kakati T, Bhattacharyya DK, Barah P, et al. Comparison of Methods for Differential Co-expression Analysis for Disease Biomarker Prediction. Comput Biol Med 2019;113:103380 [Crossref] [PubMed]
- Langfelder P, Horvath S. WGCNA: an R package for weighted correlation network analysis. BMC Bioinformatics 2008;9:559. [Crossref] [PubMed]
- Ma X, Tao R, Li L, et al. Identification of a 5-microRNA signature and hub miRNA-mRNA interactions associated with pancreatic cancer. Oncol Rep 2019;41:292-300. [PubMed]
- Chen S, Yang D, Lei C, et al. Identification of crucial genes in abdominal aortic aneurysm by WGCNA. PeerJ 2019;7:e7873 [Crossref] [PubMed]
- Gasiulė S, Stankevičius V, Patamsytė V, et al. Tissue-Specific miRNAs Regulate the Development of Thoracic Aortic Aneurysm: The Emerging Role of KLF4 Network. J Clin Med 2019;8:1609. [Crossref] [PubMed]
- Bi S, Liu R, He L, et al. Bioinformatics analysis of common key genes and pathways of intracranial, abdominal, and thoracic aneurysms. BMC Cardiovasc Disord 2021;21:14. [Crossref] [PubMed]
- Santovito D, Weber C. Zooming in on microRNAs for refining cardiovascular risk prediction in secondary prevention. Eur Heart J 2017;38:524-8. [PubMed]
- Micheau O. Regulation of TNF-Related Apoptosis-Inducing Ligand Signaling by Glycosylation. Int J Mol Sci 2018;19:715. [Crossref] [PubMed]
- Gonçalves I, Singh P, Tengryd C, et al. sTRAIL-R2 (Soluble TNF [Tumor Necrosis Factor]-Related Apoptosis-Inducing Ligand Receptor 2) a Marker of Plaque Cell Apoptosis and Cardiovascular Events. Stroke 2019;50:1989-96. [Crossref] [PubMed]
- Huang B, Lu S, Lai H, et al. LncRNA LOXL1-AS is up-regulated in thoracic aortic aneurysm and regulated proliferation and apoptosis of aortic smooth muscle cells. Biosci Rep 2019;39:BSR20191649 [Crossref] [PubMed]
- Mohammadzadeh A, Mirza-Aghazadeh-Attari M, Hallaj S, et al. Crosstalk between P53 and DNA damage response in ageing. DNA Repair (Amst) 2019;80:8-15. [Crossref] [PubMed]
- Coutts AS, La Thangue NB. The p53 response: emerging levels of co-factor complexity. Biochem Biophys Res Commun 2005;331:778-85. [Crossref] [PubMed]
- Zhang WM, Liu Y, Li TT, et al. Sustained activation of ADP/P2ry12 signaling induces SMC senescence contributing to thoracic aortic aneurysm/dissection. J Mol Cell Cardiol 2016;99:76-86. [Crossref] [PubMed]
- Cao X, Cai Z, Liu J, et al. miRNA504 inhibits p53dependent vascular smooth muscle cell apoptosis and may prevent aneurysm formation. Mol Med Rep 2017;16:2570-8. [Crossref] [PubMed]
- Leeper NJ, Raiesdana A, Kojima Y, et al. Loss of CDKN2B promotes p53-dependent smooth muscle cell apoptosis and aneurysm formation. Arterioscler Thromb Vasc Biol 2013;33:e1-e10. [Crossref] [PubMed]
- Kischkel FC, Lawrence DA, Chuntharapai A, et al. Apo2L/TRAIL-dependent recruitment of endogenous FADD and caspase-8 to death receptors 4 and 5. Immunity 2000;12:611-20. [Crossref] [PubMed]
- Zhang L, Fang B. Mechanisms of resistance to TRAIL-induced apoptosis in cancer. Cancer Gene Ther 2005;12:228-37. [Crossref] [PubMed]
- Boise LH, González-García M, Postema CE, et al. bcl-x, a bcl-2-related gene that functions as a dominant regulator of apoptotic cell death. Cell 1993;74:597-608. [Crossref] [PubMed]
- Minn AJ, Boise LH, Thompson CB. Bcl-x(S) anatagonizes the protective effects of Bcl-x(L). J Biol Chem 1996;271:6306-12. [Crossref] [PubMed]
- Braun T, Dar S, Vorobiov D, et al. Expression of Bcl-x(S) in Xenopus oocytes induces BH3-dependent and caspase-dependent cytochrome c release and apoptosis. Mol Cancer Res 2003;1:186-94. [PubMed]
- Okura T, Igase M, Kitami Y, et al. Platelet-derived growth factor induces apoptosis in vascular smooth muscle cells: roles of the Bcl-2 family. Biochim Biophys Acta 1998;1403:245-53. [Crossref] [PubMed]
- Durdu S, Deniz GC, Balci D, et al. Apoptotic vascular smooth muscle cell depletion via BCL2 family of proteins in human ascending aortic aneurysm and dissection. Cardiovasc Ther 2012;30:308-16. [Crossref] [PubMed]
- Altucci L, Leibowitz MD, Ogilvie KM, et al. RAR and RXR modulation in cancer and metabolic disease. Nat Rev Drug Discov 2007;6:793-810. [Crossref] [PubMed]
- Grignani F, Ferrucci PF, Testa U, et al. The acute promyelocytic leukemia-specific PML-RAR alpha fusion protein inhibits differentiation and promotes survival of myeloid precursor cells. Cell 1993;74:423-31. [Crossref] [PubMed]
- Altucci L, Rossin A, Raffelsberger W, et al. Retinoic acid-induced apoptosis in leukemia cells is mediated by paracrine action of tumor-selective death ligand TRAIL. Nat Med 2001;7:680-6. [Crossref] [PubMed]
- Greiner EF, Kirfel J, Greschik H, et al. Differential ligand-dependent protein-protein interactions between nuclear receptors and a neuronal-specific cofactor. Proc Natl Acad Sci U S A 2000;97:7160-5. [Crossref] [PubMed]
- Gabriely G, Kama R, Gelin-Licht R, et al. Different domains of the UBL-UBA ubiquitin receptor, Ddi1/Vsm1, are involved in its multiple cellular roles. Mol Biol Cell 2008;19:3625-37. [Crossref] [PubMed]
- Wen Z, Pan T, Yang S, et al. Up-regulated NRIP2 in colorectal cancer initiating cells modulates the Wnt pathway by targeting RORbeta. Mol Cancer 2017;16:20. [Crossref] [PubMed]
- Kostina A, Bjork H, Ignatieva E, et al. Notch, BMP and WNT/β-catenin network is impaired in endothelial cells of the patients with thoracic aortic aneurysm. Atheroscler Suppl 2018;35:e6-e13. [Crossref] [PubMed]
- Krishna SM, Seto SW, Jose RJ, et al. Wnt Signaling Pathway Inhibitor Sclerostin Inhibits Angiotensin II-Induced Aortic Aneurysm and Atherosclerosis. Arterioscler Thromb Vasc Biol 2017;37:553-66. [Crossref] [PubMed]
- Michel JB. Biology of Vascular Wall Dilation and Rupture: Oxford, UK: Oxford University Press; 2017.
- Woo CC, Liu W, Lin XY, et al. The interaction between 30b-5p miRNA and MBNL1 mRNA is involved in vascular smooth muscle cell differentiation in patients with coronary atherosclerosis. Int J Mol Sci 2019;21:11. [Crossref] [PubMed]
- Ji B, Chen L, Cai Q, et al. Identification of an 8-miRNA signature as a potential prognostic biomarker for glioma. PeerJ 2020;8:e9943 [Crossref] [PubMed]
- Zhuang H, Ma Z, Huang K, et al. Establishment of a 7-miRNA-Based Risk Score System for Predicting Prognosis of Pancreatic Cancer. Pancreas 2020;49:655-62. [Crossref] [PubMed]
- Wang Y, Barbacioru CC, Shiffman D, et al. Gene expression signature in peripheral blood detects thoracic aortic aneurysm. PLoS One 2007;2:e1050 [Crossref] [PubMed]
- Gurung R, Choong AM, Woo CC, et al. Genetic and Epigenetic Mechanisms Underlying Vascular Smooth Muscle Cell Phenotypic Modulation in Abdominal Aortic Aneurysm. Int J Mol Sci 2020;21:6334. [Crossref] [PubMed]
- Goldfinger JZ, Halperin JL, Marin ML, et al. Thoracic aortic aneurysm and dissection. J Am Coll Cardiol 2014;64:1725-39. [Crossref] [PubMed]
- Zhang L, Wang HH. The genetics and pathogenesis of thoracic aortic aneurysm disorder and dissections. Clin Genet 2016;89:639-46. [Crossref] [PubMed]
(English Language Editors: L. Huleatt and J. Gray)