The role of clinical signs and spirometry in the diagnosis of obstructive airway diseases: a systematic analysis adapted to general practice settings
Introduction
Chronic obstructive pulmonary disease (COPD) and asthma, are common disorders (1,2) that are often diagnosed and treated within general practitioner (GP) or family practice settings, in which the scope of diagnostic possibilities is limited compared to specialists. The aim to maximize diagnostic efficiency leads to the question, which information is essential, and which is secondary. Obstructive airway diseases are often diagnosed via clinical history and signs, as well as lung function data derived from spirometry in that seems to be available to some extent under GP conditions (3). In contrast, specialists’ recommendations for the diagnosis of obstructive airway diseases (1,2,4) may include functional information that is not generally available under GP conditions. This could lead to heterogeneous diagnostic approaches with non-optimal and non-comparable results.
Thus, structured algorithms adapted to GP conditions could be helpful in raising the quality of diagnostic practice (4,5). An attractive option is to derive such algorithms directly from data and to quantify their performance under realistic conditions. This has been done previously by a number of investigators addressing the diagnosis of asthma or COPD or both [e.g., (6-9)]. Similar algorithms have been used for phenotyping and prognosis (10,11). These studies provided encouraging and interesting results but most of them did not pay attention to the limited scope of methods in the great majority of GP settings. The present study explicitly aimed to account for these limitations by using a minimal set of diagnostic variables.
For this, we used a large data set recruited in a pulmonological practice (12-14), taking advantage of the specific expertise and procedures providing reliable diagnoses for reference. In the present study, we focused on questions related to clinical history and symptoms, either taken alone, or in combination with spirometry. For analysis, advanced statistical procedures such as decision trees (15) and Bayesian probabilistic networks (16) were used, with the final aim to arrive at transparent, easily conceivable diagnostic algorithms that may be simple enough to be applied in clinical practice.
Methods
Study population
The analysis was based on data from a diagnostic study on capnovolumetry performed in a pulmonary outpatient clinic in Augsburg, Germany, from February 2018 to April 2018. This study had the aim to assess the diagnostic value of capnovolumetry in the detection of airway obstruction. These results have been published (12-14) and are not part of the present evaluation. Subjects were consecutively enrolled if the inclusion criteria of age ≥18 years and the ability to understand the German language were met. Out of 1,400 subjects recruited, only those with complete measurements and without bronchial provocation challenge or bronchodilator testing were included in order to avoid possible interferences with the diagnostic questions and spirometric results. This resulted in a final set of participants having either the diagnosis of COPD, or asthma, or other respiratory diseases (such as restrictive disorders, pneumonia or other infections, pleural diseases, lung tumor, bronchiectasis), or no respiratory disease (control).
Diagnoses as taken from subjects’ files were based on all previously obtained functional and clinical information including previous bronchial provocation and bronchodilator tests. In 114 subjects, an obstructive airway disease was newly diagnosed, but the questions of the present study were not explicitly used and the diagnosis was established by the physician independently. The study had been performed in accordance with the Declaration of Helsinki (as revised in 2013) and had been approved by the Ethical Committee of the Medical Faculty of the Technical University of Munich (522/17 S). All subjects gave their written, informed consent. The study is registered under DRKS00013935 at German Clinical Trials Register (DRKS), where its protocol can be accessed. Date of registration 01/03/2018.
Assessments
These included a clinical part (questions) and a functional part (spirometry). Spirometric measurements followed established criteria (17,18). The basic measure was forced expiratory volume in 1 s (FEV1), which is known to be most robust among lung function measures. Additionally, we evaluated forced vital capacity (FVC), the ratio FEV1/FVC, mean expiratory flow rates at 25%, 50% and 75% of vital capacity (MEF25, MEF50, MEF75), and maximal mid-expiratory flow rate (MMEF). In order to reveal the maximum information, we included all these measures, although the reliability of some of them that critically depend on the quality of spirometry might differ between GP and specialist conditions. To account for the dependence of lung function parameters on anthropometric characteristics, z scores were used based on established reference values [Global Lung Function Initiative (GLI), European Community for Steel and Coal (ECSC)] (19,20).
Regarding clinical history, signs and symptoms, we used seven questions regarding dyspnea upon mild or strong exertion, cough, phlegm, wheezing in the last 12 months, and smoking status (current, ex-smoker) selected from a larger set of questions (14) on the basis that they were answered by all or nearly all subjects, resulting in a low percentage of missing data. In addition, the question for self-diagnosed allergic rhinitis was included, but not in all analyses due to the relatively high percentage of missing values. Thus, the maximum number of questions was eight.
Statistical analysis
Median values and quartiles are given for description. To understand the structure of the data, binary logistic regression analyses were performed comparing COPD with control, asthma with control, and COPD with asthma. We only used these binary comparisons, as comparisons between three or more diagnostic groups resulted in complicated and non-robust models. The results of the logistic regression analyses are not presented in detail, as they only served for the pre-selection of relevant variables in the more advanced approaches.
Path analysis
Path analysis, a special case of structural equation modelling, is valuable for the understanding of networks of relationships (13,21-24), especially through its capability to describe direct and indirect relationships between variables. The goodness of fit was measured by the comparative fit index (CFI), with a required value of >0.95. We took this measure as primary criterion, as in large data sets the common χ2 statistics can be oversensitive. To account for the dependence on anthropometric characteristics and eliminate these as explicit items, all variables were adjusted for age, sex and BMI. Moreover, z-scores of lung function data were used based on the predicted values of the GLI (19). The model comprised data from asthma and COPD, whereby these two groups were treated as two layers of the same basic structure. This allowed to identify relationships that were significant in one of the two groups but not the other, while keeping all other relationships. The path analysis resulted in a statistically robust, consistent and physiological meaningful network that served as initial version of the Bayesian network (see below).
Classification and decision trees
The decision trees provided algorithms for diagnosis, in contrast to the path analysis. As we aimed at simple, intuitively understandable results, we computed single trees and did not follow the alternative approach of Random Forests (14), since an ensemble of trees cannot be simply visualized which was one of the aims of the present study. Separate trees were constructed for COPD vs. control, asthma vs. control, COPD vs. asthma, and COPD vs. asthma vs. control. Additionally, trees were obtained for the differentiation of asthma or COPD versus their respective complementary groups, comprising all participants not having either asthma or COPD, respectively. All anamnestic questions and all measures of spirometry (without pre-defined cut-off values) were offered to the search algorithm, in addition age, sex and BMI. The final trees were those that were robust to tenfold cross-validation.
Bayesian probabilistic networks
Associations between clinical or functional variables and diagnoses can also be described by probabilistic networks as shown in previous studies [e.g., (25-27)]. These networks bear some similarity to path analysis models, but the relationships are formulated as conditional probabilities instead of regression coefficients (28). The results were depicted as network of nodes representing binary alternatives of diagnostic criteria, and one node comprising the three diagnoses COPD, asthma and control. We used only categorical variables to keep the networks understandable. Thus, FEV1, which turned out to be the only relevant, robust lung function measure in the network, was categorized according to its lower limit of normal (LLN, lower 5-percentile) (19). The basic structure was taken from the path analysis result, and additional dependencies were added to achieve an optimal fit, which was quantified via the Bayesian information criterion (BIC). To illustrate the diagnostic use, those values of relevant variables were identified that resulted in the maximum probability for each of the three diagnoses.
For path analysis, AMOS version 25 (IBM Corp., Armonk, NY, USA) and the generalized least squares estimation criterion were used. For decision trees, we employed the CHAID method as implemented in SPSS (15), including Bonferroni correction and 10-fold cross-validation. The Bayesian network was constructed with GeNIe Modeler (Version 2.5.R4, BayesFusion, LLC, Pittsburgh, USA). All other statistical analyses were performed with SPSS (Version 25, IBM Corp., Armonk, NY, USA), and the level of significance was assumed at P<0.05.
Results
Study population
Overall, 1,287 subjects fulfilled the inclusion criteria (Figure 1). Among them, 433 subjects had a diagnosis of asthma, 260 of COPD (227 GOLD 1 to 4, 33 others) (2), 230 of other respiratory diseases, and 364 subjects did not show a respiratory disease. There were 34 subjects with COPD and the comorbidity asthma, who were assigned to the COPD group, as this disease dominated the functional alterations. Subjects’ characteristics stratified according to diagnostic groups are shown in Table 1. As indicated, groups differed significantly from each other in all measures except body mass index (BMI).
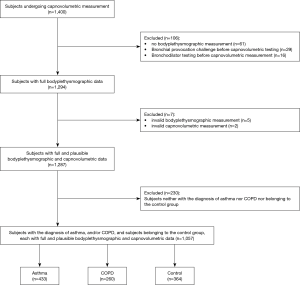
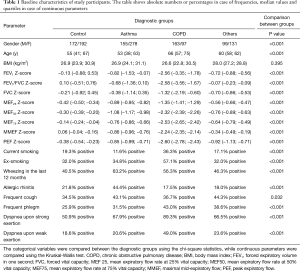
Full table
Path analysis for asthma and COPD
As the results of logistic regression analyses (see Supplementary) were not well suited for understanding, we focused on other analytical approaches. Among spirometric parameters, only FEV1 could be integrated into the path analysis network of clinical signs and symptoms in a consistent and robust manner. When replacing FEV1 by MMEF or by MEF50, these parameters turned out to be inferior to FEV1 as indicated by lower CFI values. The path analysis model incorporating FEV1 and clinical signs and symptoms is shown in Figure 2. It comprised asthma and COPD in a two-group design, assuming the same structure for both, but with the possibility that some relationships were significant in only one of the two groups. The overall χ2 statistics was 49.59 with 36 degrees of freedom (P=0.065), the CFI 0.978, indicating an acceptable fit. The differences between asthma and COPD were related to the symptom of wheezing. In asthma, wheezing was significantly linked to FEV1 and dyspnea at strong exertion, whereas this was not the case in COPD, underlining the minor role of this symptom in this disease.
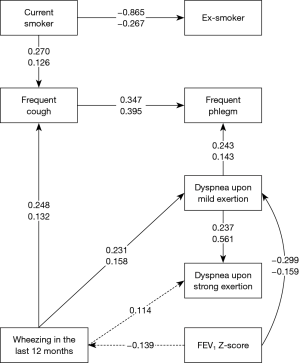
Decision trees
For each of the comparisons listed in the methods section, decision trees were developed. In order to facilitate their use, variables were not adjusted for age, sex and BMI. Instead, these characteristics were included explicitly and offered to the search algorithm together with the other variables. To illustrate the major results, we show the two trees referring to either the comparison of asthma vs. COPD vs. control (Figure 3), or that of asthma vs. COPD (Figure 4).
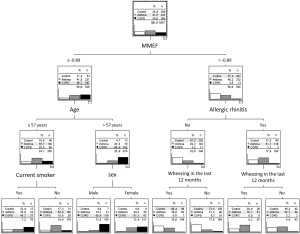
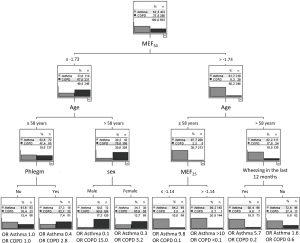
Asthma vs. COPD vs. control
In the first tree, the relevant variables occurring as nodes were MMEF, age, allergic rhinitis, current smoking, sex, and wheezing (Figure 3). As can be seen, some combinations of values along the tree were associated with major alterations in the distribution over diagnoses compared to baseline (root node), while other combinations were not associated with such alterations and thus barely informative. When having a high MMEF, no allergic rhinitis and no wheezing, the likelihood of being without respiratory disease was increased from 34.4% to 73.5%. When having high MMEF, allergic rhinitis and wheezing, the likelihood for asthma was maximized (70.1% vs. 41.0%), whereas the likelihood for COPD was maximized (80.4% vs. 24.6%) when having low MMEF, high age and being male. Conversely, the diagnostic gain was very low when, e.g., subjects had a low MMEF and low age and were smokers. These observations underlined the capability of the trees to indicate both informative and non-informative combinations of values. The overall diagnostic accuracy in the tree was 64.7%. Separate trees for asthma vs. control and COPD vs. control are shown in the Figures S1,S2.
Asthma vs. COPD
The second decision tree shown (Figure 4) referred to the distinction between asthma and COPD, implicitly assuming that an obstructive airway disease is present. The important variables under this condition were MEF50, MEF25, age, sex, phlegm and wheezing. The combination of low MEF50, high age and male sex was most indicative of COPD (90.0% vs. 37.5%), while asthma was most likely when MEF50 and MEF25 were relatively high and age was low (99.3% vs. 62.5%). Non-informative was the combination of low MEF50, low age and absence of phlegm, amongst other combinations. The overall diagnostic accuracy in this tree was 82.3%. Further decision trees are presented in the supplement.
All decision trees showed the phenomenon that specific combinations of values were informative for diagnosis, while others did not carry relevant information. The informative and non-informative combinations could always be easily recognized. This variation in performance renders it questionable, whether overall measures such as specificity and sensitivity are adequate, and we therefore do not present these values. In the analysis of asthma we did not differentiate between allergic and non-allergic asthma, as a proper differential diagnosis probably needs additional information not commonly available to a GP. Decision trees were essentially unchanged if the forced expiratory flow rates were substituted by FEV1, and there were only minor losses in predictive accuracy from this substitution indicating that FEV1 was almost as informative as the flow rates.
Probabilistic networks
The probabilistic network contained only one node comprising the distribution over the diagnoses of COPD, asthma and control, in contrast to the decision trees for which the distribution appeared in each final node (leaf). If the variables attained specific values in an individual subject, changes in the likelihood of diagnoses resulted via the network of conditional probabilities. When choosing values for the nodes directly linked to the diagnostic node, the influence of all nodes mediated through these nodes was screened, thus, not all nodes were directly relevant for the diagnostic node. We described the diagnostic power of the network by selecting values for the directly relevant nodes that maximized the likelihood for each of the diagnoses.
Figure 5 shows the diagnostic network comprising clinical signs and symptoms without lung function. The diagnostic node was directly linked to dyspnea at mild exertion, wheezing, ex-smoker status and current smoking. The probability for COPD achieved its highest gain (67% vs. 27%) when dyspnea at mild exertion, current smoking and wheezing were present. The probability for asthma was highest (69% vs. 42%) when no dyspnea at mild exertion, no current smoking but wheezing applied. The probability for control was highest (50% vs. 31%) when there was no dyspnea at mild exertion, no current smoking and no wheezing. The BIC score of this model was −3,826.9.
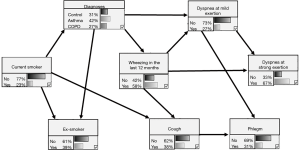
The analogous network with inclusion of FEV1 is shown in Figure 6. The node containing the three diagnoses was linked to current smoking and ex-smoking status, as well as FEV1. The former links to wheezing and dyspnea at mild exertion were now mediated via FEV1, underlining the importance of lung function. The probability for COPD was maximized for low FEV1 in combination with current smoking (81% vs. 27%), while that for asthma was maximal for low FEV1 in combination with being a non-smoker (66% vs. 42%), and that for control subjects was maximal for high FEV1 in combination with being a non-smoker (45% vs. 31%). These results were in line with those of the decision trees, showing that the diagnosis of COPD could be achieved with higher accuracy than the other diagnoses. The BIC score of the model was −3,845.3.
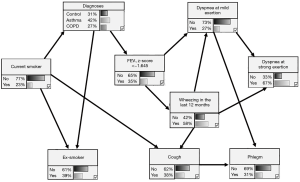
Discussion
In the present study, we evaluated the role of anamnestic questions in the diagnosis of asthma and COPD, with or without inclusion of spirometric lung function. By using a small number of simple questions comprising eight items on clinical history and symptoms, and eventually spirometry, the set-up resembled the situation encountered in general practice, where resources are strongly limited. Based on modern statistical approaches, we finally aimed at comprehensive algorithms that might be easily transferred to clinical practice. COPD was most easily recognized, whereas stable asthma turned out to be more difficult. In most comparisons, smoking history was relevant, moreover wheezing and dyspnea upon exertion, whereas phlegm and cough were of minor importance. The role of lung function parameters depended on the specific diagnostic comparison but among them FEV1 appeared to be most robust. All decision models required at least some anamnestic information, thereby underlining the clinical intuition that spirometric lung function alone is never sufficient for diagnosis. Our results may be useful to improve the quality and comparability of diagnostic procedures in GP settings.
Among respiratory diseases, obstructive disorders represent a considerable proportion of diagnoses. Although their therapy bears similarities, e.g., in the use of bronchodilators, there are also differences, e.g., in the prescription of inhaled corticosteroids. Moreover, their clinical course and prevention measures are different. Thus, a diagnosis of asthma versus COPD has practical consequences but it requires a minimum of information not always available to a GP. It would be helpful to make a diagnosis with high reliability, wherever possible, but also to identify individuals with functional or clinical patterns requiring the involvement of a specialist. Another problem is that spirometry is not available in all general practices and, if performed, is not guaranteed to satisfy established quality criteria (29). To simulate the worst case, we therefore examined the diagnostic potential of anamnestic data without spirometry. In our previous studies (12,13), lung function data had only been used to decide on the presence of airway obstruction, while anamnestic questions were analysed only in relation to capnovolumetry (14), which is neither widely available nor equivalent to spirometry (13).
For the purpose of our analysis, the reliability of diagnoses had to be guaranteed, and this was achieved by including subjects from a specialists’ practice. In more than 90% of subjects, the diagnoses had been established prior to inclusion, furthermore the answers to the questions that we used were never given to the physician. By this approach, we aimed to mimic the situation of a subject newly diagnosed under GP conditions. The differential diagnoses included comparisons with the control group showing no signs of respiratory disease, as well as comparisons with the complement of asthma or COPD including subjects who might have obstructive or other airway disorders. The results confirmed the expectation that diagnostic reliability was maximal with the control group and lower with the complementary, inhomogeneous groups. This difference underlined the importance of prior information to get the diagnostic process as targeted as possible.
Among the spirometric measures, FEV1 was the only robust and consistent parameter in both the path analysis and the probabilistic model, although not in the decision trees which, however, covered a range of diagnostic comparisons. This is an argument in favour of FEV1 as primary measure from spirometry. Another argument is that among spirometric measures, FEV1 is less dependent on a potentially compromised (29,30) quality of spirometric measurements than expiratory flow rates. Whether a lower quality of spirometry would affect its value relative to the questions, might be addressed in trials performed under GP conditions.
Algorithms for the optimization of diagnostic processes and the identification of exacerbation risk in asthma or COPD have been proposed in a number of previous studies, using a variety of statistical approaches such as logistic regression, decision trees including Random Forest, support vector machines and neural networks (6-11,31,32). These algorithms incorporated anamnestic questions and spirometric parameters, similar to our study, but also data such as the bronchodilator response that is not routinely assessed in GP conditions. Our study has similarities to that by Metting and co-workers (6) who developed a decision tree from a large data set, and to that by Spathis and co-workers who developed a Random Forest ensemble of trees for the diagnosis of asthma and COPD (7). Compared to previous studies, our goal was (I) to limit the required information as much as possible and (II) to focus on algorithms that, as far as possible, could be intuitively captured irrespective of the fact that they might have been found with complicated mathematics.
This was the reason why we opted for single decision trees that can be used without computer, although these may be improved by an ensemble of trees (Random Forest) being more reliable than a single tree (14). The price payed is the lack of visualization and usability as the ensemble can only be evaluated with a computer. Moreover, in our previous study the difference between the results of a single tree and the ensemble were minor (14). The trees and the probabilistic network involved the a priori distributions over diagnoses, which may differ from practice to practice. The implementation of a priori probabilities would provide a way to adapt the algorithm to local conditions. It has to be mentioned, however, that the Bayesian networks, although easily depicted, can only be efficiently evaluated via computer, which might be easily achieved by an App. The path analysis was not utilized for the purpose of diagnosis, but only to understand the relationship between questions and lung function including differences between COPD and asthma, which indeed occurred for the associations with wheezing.
Limitations
The study aimed at a potential application in GP settings but the data was obtained in a specialist practice in order to ensure the validity of diagnoses and assessments, and the spirometry might have been of higher quality than many measurements performed in general practices (29,30). It is important to note that pulmonary outpatient clinics in Germany are organized as private practices of specialists in primary care that can be visited by patients without referral. Therefore, we believe that patients recruited in general practice settings would not differ much from our study population which has relatively good lung function values (see Table 1). Nevertheless, the results should be validated in a general practice setting. Moreover, subjects were treated with respiratory medication, thereby reducing the burden from symptoms and functional deteriorations. Thus, in newly diagnosed subjects diagnostic accuracy might well be higher than observed in the present study, especially for asthma, for which the likelihood to be detected by the algorithms proposed by us might be reduced. In case of COPD, functional alterations and clinical signs are known to be less variable, thus the likelihood that these subjects are correctly diagnosed may not have been much affected by treatment. The diagnostic accuracy might also have been affected by the fact that the 34 patients with asthma and COPD were attributed to COPD in order to have unique diagnoses. This, however, was only a minority of patients and the proper diagnosis of concomitant asthma and COPD cannot be reasonably expected from a simple tools which we used. Using separate trees for asthma vs. control and COPD vs. control and their combination, we tried to identify these patients but this was not possible with sufficient specificity (see Supplementary). Another limitation is that the study is a secondary analysis of data addressing the diagnostic value of a novel lung function method (capnovolumetry). It is, however, an independent analysis and does not refer to the previous findings. Irrespective of this, its results should be validated using both different cohorts and methods. The strength of the study is the high quality of assessments and the large sample size.
Conclusions
We propose simple and at the same time statistically validated approaches for the diagnosis of asthma and COPD, whereby the required information was compact enough in view of the inevitable limitations of diagnostic tools under GP conditions. For this purpose decision trees and probabilistic networks were developed. Smoking history, age, dyspnea upon exertion and wheezing turned out as primary diagnostic determinants, resulting in a considerable diagnostic gain when used either alone or in combination with FEV1. Overall, the diagnosis of COPD was more accurate than that of stable asthma. These findings might be helpful as a starting point for standardized diagnostic algorithms that may be easily understood by the user and also valuable for the training of physicians.
Acknowledgments
We are grateful to Drs. Hellmann, Wehgartner-Winkler, Faderl, Dankelmann, Vitiello and Schlimok for the opportunity to perform the study in their practice. We also thank the technical personnel, especially Therese Kauf, Marion Saladin and Franziska Schön, for making the study possible through logistic and organizational support. In addition, we appreciate the willingness of all participants to participate in the study.
Funding: The Institute of General Practice and Health Services Research (Munich, Germany) received a grant from Ganshorn Medizin Electronic GmbH (Niederlauer, Germany) during the conduct of the study.
Footnote
Data Sharing Statement: Available at http://dx.doi.org/10.21037/jtd-20-3539
Peer Review File: Available at http://dx.doi.org/10.21037/jtd-20-3539
Conflicts of Interest: All authors have completed the ICMJE uniform disclosure form (available at http://dx.doi.org/10.21037/jtd-20-3539). A.S., the director of the Institute of General Practice and Health Services Research (Munich, Germany), received a grant from Ganshorn Medizin Electronic GmbH (Niederlauer, Germany) during the conduct of the study. The funders did not play any role in the design of the study, data evaluation and interpretation of the results. The other authors have no conflicts of interest to declare.
Ethical Statement: The authors are accountable for all aspects of the work in ensuring that questions related to the accuracy or integrity of any part of the work are appropriately investigated and resolved. The study had been performed in accordance with the Declaration of Helsinki (as revised in 2013) and had been approved by the Ethical Committee of the Medical Faculty of the Technical University of Munich (522/17 S). All subjects gave their written, informed consent.
Open Access Statement: This is an Open Access article distributed in accordance with the Creative Commons Attribution-NonCommercial-NoDerivs 4.0 International License (CC BY-NC-ND 4.0), which permits the non-commercial replication and distribution of the article with the strict proviso that no changes or edits are made and the original work is properly cited (including links to both the formal publication through the relevant DOI and the license). See: https://creativecommons.org/licenses/by-nc-nd/4.0/.
References
- Global Initiative for Asthma. Global Strategy for Asthma Management and Prevention, 2020. Available online: www.ginasthma.org
- Singh D, Agusti A, Anzueto A, et al. Global strategy for the diagnosis, management, and prevention of chronic obstructive lung disease: the GOLD science committee report 2019. Eur Respir J 2019;53:1900164 [Crossref] [PubMed]
- Derom E, Van Weel C, Liistro G, et al. Primary care spirometry. Eur Respir J 2008;31:197-203. [Crossref] [PubMed]
- Graber M, Gordon R, Franklin N. Reducing diagnostic errors in medicine: what's the goal? Acad Med 2002;77:981-92. [Crossref] [PubMed]
- McDonald KM, Matesic B, Contopoulos-Ioannidis DG, et al. Patient safety strategies targeted at diagnostic errors: a systematic review. Ann Intern Med 2013;158:381-9. [Crossref] [PubMed]
- Metting EI, Dekhuijzen PR, van Heijst E, et al. Development of a diagnostic decision tree for obstructive pulmonary diseases based on real-life data. ERJ Open Res 2016;2:00077-2015 [Crossref] [PubMed]
- Spathis D, Vlamos P. Diagnosing asthma and chronic obstructive pulmonary disease with machine learning. Health Informatics J 2019;25:811-27. [Crossref] [PubMed]
- Pescatore AM, Dogaru CM, Duembgen L, et al. A simple asthma prediction tool for preschool children with wheeze or cough. J Allergy Clin Immunol 2014;133:111-8.e1-13.
- Badnjevic A, Cifrek M, Koruga D, et al. Neuro-fuzzy classification of asthma and chronic obstructive pulmonary disease. BMC Med Inform Decis Mak 2015;15:S1. [Crossref] [PubMed]
- Mohktar MS, Redmond SJ, Antoniades NC, et al. Predicting the risk of exacerbation in patients with chronic obstructive pulmonary disease using home telehealth measurement data. Artif Intell Med 2015;63:51-9. [Crossref] [PubMed]
- Prosperi MC, Marinho S, Simpson A, et al. Predicting phenotypes of asthma and eczema with machine learning. BMC Med Genomics 2014;7:S7. [Crossref] [PubMed]
- Kellerer C, Jankrift N, Jorres RA, et al. Diagnostic accuracy of capnovolumetry for the identification of airway obstruction - results of a diagnostic study in ambulatory care. Respir Res 2019;20:92. [Crossref] [PubMed]
- Kellerer C, Schneider A, Klütsch K, et al. Correspondence between Capnovolumetric and Conventional Lung Function Parameters in the Diagnosis of Obstructive Airway Diseases. Respiration 2020;99:389-97. [Crossref] [PubMed]
- Kellerer C, Klütsch K, Husemann K, et al. Capnovolumetry in combination with clinical history for the diagnosis of asthma and COPD. NPJ Prim Care Respir Med 2020;30:32. [Crossref] [PubMed]
- Song YY, Ying L. Decision tree methods: applications for classification and prediction. Shanghai Arch Psychiatry 2015;27:130-5. [PubMed]
- Scutari M, Denis JB. Bayesian networks: with examples in R. CRC press; 2014.
- Criée C-P, Baur X, Berdel D, et al. Leitlinie zur Spirometrie. Pneumologie 2015;69:147-64. [Crossref] [PubMed]
- Graham BL, Steenbruggen I, Miller MR, et al. Standardization of spirometry 2019 update. An official American thoracic society and European respiratory society technical statement. Am J Respir Crit Care Med 2019;200:e70-e88. [Crossref] [PubMed]
- Quanjer PH, Stanojevic S, Cole TJ, et al. Multi-ethnic reference values for spirometry for the 3–95-yr age range: the global lung function 2012 equations. Eur Respir J 2012;40:1324-43. [Crossref] [PubMed]
- Quanjer PH, Tammeling G, Cotes J, et al. Lung volumes and forced ventilatory flows. Eur Respir J 1993;6:5-40. [Crossref] [PubMed]
- Kahnert K, Alter P, Welte T, et al. Uric acid, lung function, physical capacity and exacerbation frequency in patients with COPD: a multi-dimensional approach. Respir Res 2018;19:110. [Crossref] [PubMed]
- Kahnert K, Lucke T, Huber RM, et al. Relationship of hyperlipidemia to comorbidities and lung function in COPD: results of the COSYCONET cohort. PLoS One 2017;12:e0177501 [Crossref] [PubMed]
- Alter P, Watz H, Kahnert K, et al. Airway obstruction and lung hyperinflation in COPD are linked to an impaired left ventricular diastolic filling. Respir Med 2018;137:14-22. [Crossref] [PubMed]
- Alter P, Watz H, Kahnert K, et al. Effects of airway obstruction and hyperinflation on electrocardiographic axes in COPD. Respir Res 2019;20:61. [Crossref] [PubMed]
- Agrahari R, Foroushani A, Docking TR, et al. Applications of Bayesian network models in predicting types of hematological malignancies. Sci Rep 2018;8:6951. [Crossref] [PubMed]
- Park E, Chang H-j, Nam HS. A Bayesian Network Model for Predicting Post-stroke Outcomes With Available Risk Factors. Front Neurol 2018;9:699. [Crossref] [PubMed]
- Sa-ngamuang C, Haddawy P, Luvira V, et al. Accuracy of dengue clinical diagnosis with and without NS1 antigen rapid test: Comparison between human and Bayesian network model decision. PLoS Negl Trop Dis 2018;12:e0006573 [Crossref] [PubMed]
- Pearl J. Bayesian networks. 2011.
- Schneider A, Gindner L, Tilemann L, et al. Diagnostic accuracy of spirometry in primary care. BMC Pulm Med 2009;9:31. [Crossref] [PubMed]
- Akhtar R, Wilson A. A comparison of spirometry in general practice and a pulmonary function laboratory. Prim Care Respir J 2005;14:215-20. [Crossref] [PubMed]
- Prasad B, Prasad PK, Sagar Y, editors. A comparative study of machine learning algorithms as expert systems in medical diagnosis (Asthma). International Conference on Computer Science and Information Technology. Springer 2011;570-6.
- Dexheimer JW, Brown LE, Leegon J, et al. Comparing decision support methodologies for identifying asthma exacerbations. Stud Health Technol Inform 2007;129:880-4. [PubMed]