Construction, validation and, visualization of a web-based nomogram for predicting the overall survival and cancer-specific survival of leiomyosarcoma patients with lung metastasis
Introduction
Leiomyosarcoma (LMS) is a malignant mesenchymal-derived tumor that originates from smooth muscle cells, and accounts for 12% of soft tissue sarcomas (1,2). LMS often involves the uterus, retroperitoneal space, and soft tissue (3). The 5- and 10-year overall survival (OS) rates for LMS patients have been reported to be 64% and 46%, respectively (4,5). It has also been reported that about 20% of LMS patients will eventually develop lung metastasis (6). The prognosis of LMS patients with lung metastasis has been recognized to be poor. Thus, the survival and prognosis evaluation of LMS patients with lung metastasis is an important topic in LMS research.
The Tumor Node Metastasis (TNM) classification and staging system is generally considered to be an important tool in the diagnosis and treatment of LMS patients (7). In addition, existing research has shown that the prognosis of LMS patients varies depending on the metastatic organs (8). However, due to the different clinical and pathological characteristics of primary lesions, prognoses often differ, even when they involve the same organ (8). Thus, for patients with distant metastasis, the TNM stage cannot be effectively used to select appropriate clinical treatment options and predict a patient’s prognosis.
The nomogram has been widely used to predict the prognoses of patients with a variety of cancers, and has shown high accuracy (9,10). Further, web-based nomograms can be used to illustrate prognoses with intuitive graphics (11). Ethun and Lu built nomograms to predict the prognosis of limb LMS and uterine LMS (12,13). However, to date, no web-based nomogram has been developed to predict OS and cancer-specific survival (CSS) in LMS patients with lung metastasis. Consequently, this study sought to construct a web-based nomogram to predict the prognosis of LMS patients with lung metastasis. We present the following article in accordance with the TRIPOD reporting checklist (available at http://dx.doi.org/10.21037/jtd-21-598).
Methods
Patients
Patients were identified in the Surveillance, Epidemiology, and End Results (SEER) database. Specifically, all patients with LMS (ICD-O-3 histologic type: 8890, 8891, 8893, and 8896) were identified. To be eligible to be included in this study, patients had to meet the following inclusive criteria: (I) have a pathological diagnosis of LMS; (II) be aged more than 18 years; (III) have been diagnosed from 2010 to 2016 according to the term “year of diagnosis;” (IV) have lung metastasis; and (V) have completed the follow-up information without loss of data. Conversely, patients were excluded from the study if: (I) important details were missing from their data, including details about grade, TNM stage, tumor size, radiotherapy, chemotherapy, organ metastasis, and/or marital status; and/or (II) details of their follow-up status were missing. The study was conducted in accordance with the Declaration of Helsinki (as revised in 2013). The flow chart for patient selection criteria is shown in Figure 1. As public and anonymous data were used, according to the ethics guidelines, neither informed consent nor approval of an ethics committee was required.
Data element
Data about the following variables were extracted: age (≤55, 56–74 and ≥75 years), sex (female and male), race [White, Black, and Other (American Indian/Alaska Native, Asian/Pacific Islander)], grade (low and high), T stage (T1–T2 and T3–T4), N stage (N0 and N1), surgery (no or yes), radiotherapy (no or yes), chemotherapy (no or yes), tumor size (≤140 and >140 mm), tumor site (uterus, soft tissue and retroperitoneum), metastatic sites, including bone, brain, and liver, marital status (no or yes), and insurance (no or yes). An X-tile analysis provides the best age and tumor size cut-off point, and changes continuous variables into categorical variables (14). OS was defined as the time from diagnosis to death due to any cause. CSS was defined as the time from diagnosis to death because of the cancer. OS and CSS were designated as the 2 endpoints of the study.
Statistical analysis
Eligible LMS patients with lung metastasis were randomly assigned to the training set (70%), and the remaining patients were assigned to the validation set (30%). A univariate Cox regression analysis was conducted to analyze possible risk factors, and factors with a P value less than 0.1 in the univariate Cox regression analysis were included in the multivariate Cox analysis. Factors with a P value less than 0.05 in the multivariate Cox analysis were regarded as independent predictive factors of OS and CSS. Based on the independent predictive factors, the nomograms of OS and CSS for 1, 2 and 3 years were established by the “rms” package in R software. Receiver operating characteristic (ROC) curves and calibration curves were produced to evaluate the accuracy of the nomograms. Finally, all of the patients were divided into high- and low-risk groups using the “survival” and “survminer” package, and a Kaplan-Meier survival analysis with a log-rank test was performed to verify the prognostic value of nomogram. Finally, 2 web-based nomograms were further prepared based on the nomograms using the “Dynnom” package.
Results
Population information
A total of 228 patients were included in the OS nomogram construction, and randomly divided into the training set (n=160) and the validation set (n=68). One hundred and eighty-three patients were enrolled in the CSS nomogram construction, and randomly divided into the training set (n=129) and the validation set (n=54). The optimal critical ages for OS and CSS were 55 and 75 years old, respectively, and the optimal critical value for tumor size was 140 mm. Relevant patient data are set out in Table 1. Of the patients, 53 were male (23.25%) and 175 were female (76.75%). In relation to age, 93 patients (40.79%) were aged under 55 years, 111 patients (48.68%) were aged between 56 and 75 years, and 24 patients (10.63%) were older than 76 years. In relation to race, most of the patients were White [n=157 (65.86%)], followed by Black [n=48 (21.05%)] and Other [n=23 (10.09%)]. In relation to the tumor characteristics, 201 patients (88.16%) were in grade III–IV, 203 were in stages T1–T2 (89.04%), and 202 were in stage N0 (88.60%). Of the LMS patients with multiple metastases, 43 patients (18.86%) had liver metastasis on the basis of lung metastasis, 34 patients (14.91%) had bone metastasis on the basis of lung metastasis, and 8 patients (3.51%) had brain metastasis on the basis of lung metastasis. 161 patients (70.61%) underwent surgery, 160 patients (70.18%) underwent chemotherapy, and 54 patients (23.68%) underwent radiotherapy.
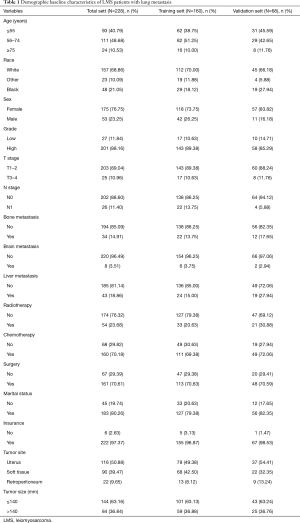
Full table
Prognostic factors of OS and CSS
In the univariate Cox analysis, the following variables were correlated with OS: age, race, grade, T stage, bone metastasis, surgery, chemotherapy, marital status, tumor site, and tumor size. In the multivariate Cox analysis, only the following variables were identified as independent risk factors of OS: an age ≥75 years, T3–T4 stage, bone metastasis, undergoing surgery, undergoing chemotherapy, marital status is married, a tumor site in soft tissue, a tumor site in retroperitoneum, and a tumor size >140 mm. Detailed information about OS is set out in Table 2. In the univariate Cox analysis, the following variables were correlated with OS: age, race, grade, T stage, bone metastasis, surgery, chemotherapy, marital status, tumor site, and tumor size. In the multivariate Cox analysis, only the following variables were identified as independent risk factors of CSS: an age ≥75 years, bone metastasis, undergoing surgery, undergoing chemotherapy, a tumor site in soft tissue, a tumor site in retroperitoneum, and a tumor size >140 mm. Detailed information about CSS is set out in Table 3.
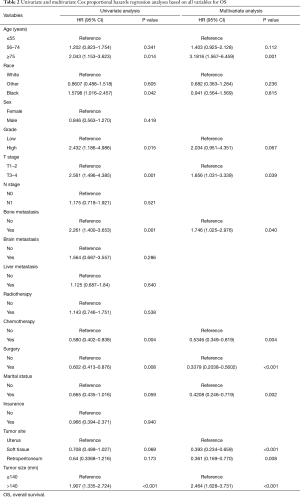
Full table
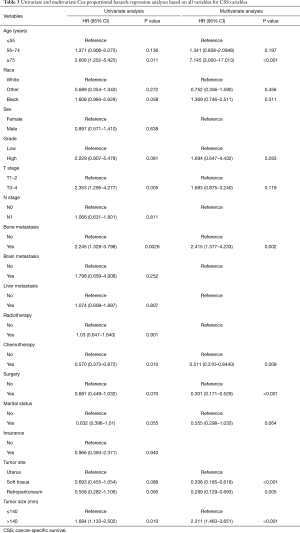
Full table
Prognostic nomogram
Based on the results of the multivariate Cox analysis, 2 prognostic nomograms were established that included all the significant independent factors of OS (see Figure 2A) and CSS (see Figure 2B). Using these nomograms, the 1-, 2-, 3-year survival probability of each patient can be predicted by adding up the specific numerical value of each predictive variable.
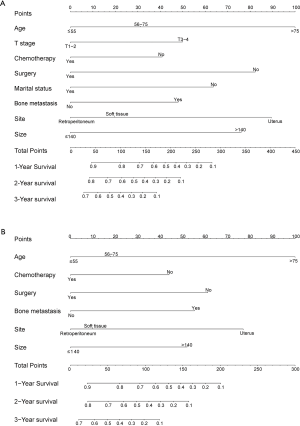
Verification of nomogram in the training set
The ROC shows that the areas under the curve (AUCs; see Figure 3A,B,C) of the nomogram for predicting 1-, 2-, and 3-year OS were 0.790, 0.839, and 0.841, respectively. The AUCs (see Figure 3D,E,F) of the nomogram for predicting 1-, 2-, and 3-year CSS were 0.789, 0.777, and 0.854, respectively. The time dependence curves of OS (see Figure 3G) and CSS (see Figure 3H) performed well, and the AUCs of the OS and CSS independent prognostic factors were significantly lower than those of the nomogram, indicating that the prediction accuracy of the OS and CSS nomograms at 1, 2 and 3 years was better than separate clinicopathological features (see Figures 3A,B,C,D,E,F). The calibration curves for OS (see Figure 4A,B,C) and CSS (see Figure 4D,E,F) showed the best agreement between the observations and the predictions of the survival probabilities.
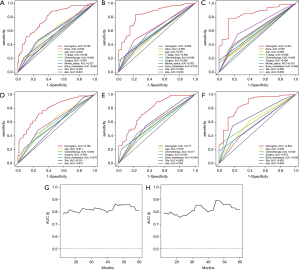
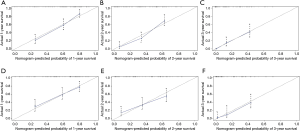
Verification of nomogram in the validation set
The ROC shows that the AUCs (see Figure 5A,B,C) of the nomogram for predicting 1-, 2-, and 3-year OS were 0.738, 0.760, and 0.785, respectively. The AUCs (see Figure 5D,E,F) of the nomogram for predicting 1-, 2-, and 3-year CSS were 0.741, 0.691, and 0.704, respectively. The time dependence curves of OS (see Figure 5G) and CSS (see Figure 5H) performed well, and the AUCs of the OS and CSS independent prognostic factors were significantly lower than those of the OS and CSS nomograms at 1, 2, and 3 years (see Figure 5A,B,C,D,E,F). The calibration curves for OS (see Figure 6A,B,C) and CSS (see Figure 6D,E,F) showed optimal consistency between the predicted and observed survival probabilities.
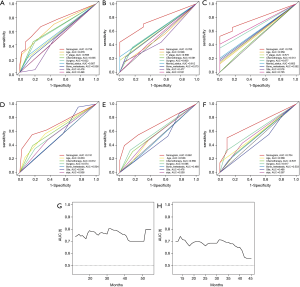
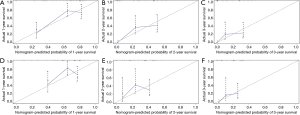
Risk classification systems for OS and CSS
High-risk and low-risk groups were defined based on the best cut-off scores of 0.6517, 0.9075, 0.9728, and 1.3887 in the OS training set, the CSS training set, the OS validation set, and the CSS validation set, respectively. The Kaplan-Meier survival analysis showed that both OS and CSS in the training set and the validation set differed between the 2 groups [P<0.001 (log-rank test); see Figure 7].
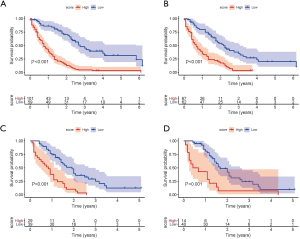
The web-based nomogram
We further visualized the nomogram and created web versions for OS (https://wenn23.shinyapps.io/lmslmosapp/) and CSS (https://wenn23.shinyapps.io/lmslmcssapp/). The survival curve and the probability of the LMS patients with lung metastasis can be displayed by selecting the corresponding clinical features and follow-up time on the left side of the web interface (see Figure 8A). For example, the OS of a LMS patient with lung metastasis whose basic information includes that the patient is 50 years old, has uterine LMS, is married, is in T2 stage, has a tumor 40 mm in size, has not undergone surgery, has not undergone chemotherapy, and has no bone metastasis can be predicted. Figure 8B shows the patient’s survival curve. The 1-year (see Figure 8C, black), 2-year (see Figure 8C, blue), 3-year (see Figure 8C, red) survival probability and 95% confidence interval (CI) can also be seen. Additionally, a specific numerical summary was produced to increase prediction accuracy (see Figure 8D). The CSS of a LMS patient with lung metastasis can be predicted in the same way.
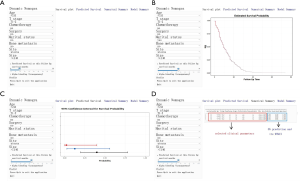
Discussion
LMS is considered an aggressive soft tissue sarcoma (15). Previous research has shown that 30% of LMS patients are prone to distant organ metastasis, and the most frequently occurring metastatic organ is the lung (16-18). LMS unresectable, lung malfunction, multiple metastases may lead to a worse prognosis for LMS patients with lung metastasis (19,20). There is evidence that the median survival time of LMS patients with lung metastasis is 15 months (16-18). Thus, precise forecasts of the survival rates of LMS patients with lung metastasis is essential if clinicians are to make effective medical decisions. The nomogram is a visualization tool for oncology-related prediction that can help clinicians to make individualized survival predictions and offer personalized treatments (21,22). However, previously, no nomogram for predicting OS and CSS in LMS patients with lung metastasis existed. Thus, we developed 2 web-based nomograms to predict OS and CSS in LMS patients with lung metastasis. Each of the developed nomograms, supported by a calibration curve and a ROC curve, predicted survival rates well. Previously, Xue analyzed the prognosis of soft tissue LMS patients using nomograms (3). However, the present study was the first to develop 2 web-based nomograms for LMS patients with lung metastasis to predict OS and CSS. The web-based nomogram can produce a target patient's prognosis by entering independent prognostic factors with single mouse click. Compared to traditional nomogram that can only predict the prognosis for certain time periods, such as one-, two-, and three-years, web-based nomogram can accurately predict the prognosis for patients at any time in the smallest unit of months. Therefore, our web-based nomograms are more accurately grouped to better capture heterogeneity between patients. Further, our comprehensive prediction model is more sensitive than separate clinicopathological features, such as, age, chemotherapy, and surgery. Additionally, a patient’s survival curve can be displayed on the web page by selecting the corresponding parameters.
In the present study, age, T stage, bone metastasis, surgery, chemotherapy, marital status, tumor size, and tumor site were found to be correlated with OS. While age, bone metastasis, surgery, chemotherapy, tumor size, and tumor site were found to be correlated with CSS. Gronchi and Krikelis's study concluded that an increase in age was related to a decrease in the survival rates of LMS patients (23,24). Similarly, our study found that patients aged over 55 and 75 years old had worse OS and CSS rates. In relation to malignant tumors, larger and higher grade tumors often indicate that the tumor is more difficult to remove completely (25). Our study further showed that tumors larger than 140 mm were associated with poor prognoses.
Our nomograms also showed the prognostic effects of different treatment regimens. Appropriate surgery can affect the prognosis of LMS patients with lung metastasis [P value (OS, CSS) <0.01]. Chemotherapy was also found to be a prognostic factor (P value (OS, CSS) <0.05), but radiotherapy was not [P value (OS) =0.538, P value (CSS) =0.901]. Consistent with other research (26), our nomograms predicted higher survival rates in patients who had undergone chemotherapy and surgery. However, risk scores for different treatments are not recommended as a direct basis for treatment selection, as clinical treatments need to be determined based on a variety of factors, including factors related to a patient’s imaging results and condition.
Lamm showed that uterine LMS had a worse prognosis than none-uterine LMS (4). Similarly, we found the patients whose primary site was in the uterus had a worse prognosis than primary site was in soft tissue and retroperitoneum. Previous studies have shown that the reason for the poor prognosis of uterine LMS is related to its late diagnosis, and negative clinical features (27,28). Notably, marital status was also related to the prognosis of LMS patients with lung metastasis, such that unmarried patients had a worse prognosis than married patients. This variable was not examined in previous studies. In our opinion, malignant tumor patients obtain psychological and financial support from their families after marriage, which has survival benefits (29). Previous studies have confirmed that lymph node metastasis (LNM) often results in a poor prognosis for LMS patients (30,31). Our study emphasized that LNM is not a prognostic factor for LMS patients with lung metastasis. The high T grade indicates the poor prognosis. Our study also found that patients’ prognoses were worse when multiple metastases occurred.
In addition to our nomogram not being 100% accurate, our research also had a number of limitations. First, in order to minimize the statistical bias, we adopted strict inclusion and exclusion criteria, and the selected cases were randomly included into training cohort and validation cohort. However, as a retrospective study, statistical bias cannot be avoided. Second, due to the irresistible absence of variables from the SEER database, we only included patients who had been diagnosed with LMS from 2010 to 2016. We believe that a longer time range and larger sample size could help to improve the practicability and accuracy of our model. Third, due to the insufficient number of end events among patients with other histological types in the SEER database, all LMS patients in this study had an ICD-O-3 histological type of 8890. Finally, due to limitations in collecting variables and data, the nomograms only provide a relative reference for clinicians.
Conclusions
We developed, validated, and visualized 2 web-based nomograms (OS: https://wenn23.shinyapps.io/lmslmosapp/) (CSS: https://wenn23.shinyapps.io/lmslmcssapp/) for LMS patient with lung metastasis. Despite some defects, these nomograms have reference value for clinicians and patients. In conclusion, the web-based nomograms showed that the prognosis of LMS patients with lung metastasis correlated with a worse prognosis if the patients were older in age, had a primary tumor larger than 140 mm, had a primary tumor located in the uterus, had bone metastasis, and had not undergone surgery or chemotherapy.
Acknowledgments
We are very grateful for the contributions of the SEER database, and the 18 registration agencies that provide information on cancer research. We would also like to thank all of our colleagues who were involved in this study.
Funding: None.
Footnote
Reporting Checklist: The authors have completed the TRIPOD reporting checklist. Available at http://dx.doi.org/10.21037/jtd-21-598
Conflicts of Interest: All authors have completed the ICMJE uniform disclosure form (available at http://dx.doi.org/10.21037/jtd-21-598). The authors have no conflicts of interest to declare.
Ethical Statement: The authors are accountable for all aspects of the work in ensuring that questions related to the accuracy or integrity of any part of the work are appropriately investigated and resolved. The study was conducted in accordance with the Declaration of Helsinki (as revised in 2013). As public and anonymous data were used, according to the ethics guidelines, neither informed consent nor approval of an ethics committee was required.
Open Access Statement: This is an Open Access article distributed in accordance with the Creative Commons Attribution-NonCommercial-NoDerivs 4.0 International License (CC BY-NC-ND 4.0), which permits the non-commercial replication and distribution of the article with the strict proviso that no changes or edits are made and the original work is properly cited (including links to both the formal publication through the relevant DOI and the license). See: https://creativecommons.org/licenses/by-nc-nd/4.0/.
Reference
- Gamboa AC, Gronchi A, Cardona K. Soft-tissue sarcoma in adults: An update on the current state of histiotype-specific management in an era of personalized medicine. CA Cancer J Clin 2020;70:200-29. [Crossref] [PubMed]
- Puri A, Gulia A. Management of extremity soft tissue sarcomas. Indian J Orthop 2011;45:301-6. [Crossref] [PubMed]
- Xue M, Chen G, Dai J, et al. Development and Validation of a Prognostic Nomogram for Extremity Soft Tissue Leiomyosarcoma. Front Oncol 2019;9:346. [Crossref] [PubMed]
- Lamm W, Natter C, Schur S, et al. Distinctive outcome in patients with non-uterine and uterine leiomyosarcoma. BMC Cancer 2014;14:981. [Crossref] [PubMed]
- Svarvar C, Böhling T, Berlin O, et al. Clinical course of nonvisceral soft tissue leiomyosarcoma in 225 patients from the Scandinavian Sarcoma Group. Cancer 2007;109:282-91. [Crossref] [PubMed]
- Okamoto M, Matsuoka M, Soma T, et al. Metastases of soft tissue sarcoma to the liver: A Historical Cohort Study from a Hospital-based Cancer Registry. Cancer Med 2020;9:6159-65. [Crossref] [PubMed]
- Pasquali S, Gronchi A. Neoadjuvant chemotherapy in soft tissue sarcomas: latest evidence and clinical implications. Ther Adv Med Oncol 2017;9:415-29. [Crossref] [PubMed]
- Riihimäki M, Hemminki A, Fallah M, et al. Metastatic sites and survival in lung cancer. Lung Cancer 2014;86:78-84. [Crossref] [PubMed]
- Yan B, Su BB, Bai DS, et al. A practical nomogram and risk stratification system predicting the cancer-specific survival for patients with early hepatocellular carcinoma. Cancer Med 2021;10:496-506. [Crossref] [PubMed]
- Yan L, Deng W, Guan L, Xu H. Nomogram forecasting 3-, 5-, and 8-year overall survival and cancer-specific survival of gingival squamous cell carcinoma. Cancer Med 2020;9:8266-74. [Crossref] [PubMed]
- Zheng X, Huang R, Liu G, et al. Development and verification of a predictive nomogram to evaluate the risk of complicating ventricular tachyarrhythmia after acute myocardial infarction during hospitalization: A retrospective analysis. Am J Emerg Med 2020; Epub ahead of print. [Crossref] [PubMed]
- Ethun CG, Lopez-Aguiar AG, Switchenko JM, et al. The Prognostic Value of Lymphovascular Invasion in Truncal and Extremity Soft Tissue Sarcomas: An Analysis from the National Cancer Database. Ann Surg Oncol 2019;26:4723-9. [Crossref] [PubMed]
- Lu YJ, Wang H, Fang LY, et al. A nomogram for predicting overall survival in patients with uterine leiomyosarcoma: a SEER population-based study. Future Oncol 2020;16:573-84. [Crossref] [PubMed]
- Camp RL, Dolled-Filhart M, Rimm DL. X-tile: a new bio-informatics tool for biomarker assessment and outcome-based cut-point optimization. Clin Cancer Res 2004;10:7252-9. [Crossref] [PubMed]
- Massi D, Beltrami G, Mela MM, et al. Prognostic factors in soft tissue leiomyosarcoma of the extremities: a retrospective analysis of 42 cases. Eur J Surg Oncol 2004;30:565-72. [Crossref] [PubMed]
- Tsuyoshi H, Yoshida Y. Molecular biomarkers for uterine leiomyosarcoma and endometrial stromal sarcoma. Cancer Sci 2018;109:1743-52. [Crossref] [PubMed]
- D'Angelo E, Prat J. Uterine sarcomas: a review. Gynecol Oncol 2010;116:131-9. [Crossref] [PubMed]
- Arend RC, Toboni MD, Montgomery AM, et al. Systemic Treatment of Metastatic/Recurrent Uterine Leiomyosarcoma: A Changing Paradigm. Oncologist 2018;23:1533-45. [Crossref] [PubMed]
- Hoven-Gondrie ML, Bastiaannet E, Ho VK, van Leeuwen BL, Liefers GJ, Hoekstra HJ, Suurmeijer AJ. Worse Survival in Elderly Patients with Extremity Soft-Tissue Sarcoma. Ann Surg Oncol 2016;23:2577-85. [Crossref] [PubMed]
- Di F, He C, Pu G, Zhang C. Support Vector Machine for Lung Adenocarcinoma Staging Through Variant Pathways. G3 (Bethesda) 2020;10:2423-34. [Crossref] [PubMed]
- Zhu Y, Fang X, Wang L, et al. A Predictive Nomogram for Early Death of Metastatic Gastric Cancer: A Retrospective Study in the SEER Database and China. J Cancer 2020;11:5527-35. [Crossref] [PubMed]
- Xu Y, Xu G, Wu H, et al. The Nomogram for Early Death in Patients with Bone and Soft Tissue Tumors. J Cancer 2020;11:5359-70. [Crossref] [PubMed]
- Meng Y, Yang Y, Zhang Y, et al. Construction and Validation of Nomograms for Predicting the Prognosis of Uterine Leiomyosarcoma: A Population-Based Study. Med Sci Monit 2020;26:e922739 [Crossref] [PubMed]
- Hoven-Gondrie ML, Bastiaannet E, Ho VK, et al. Worse Survival in Elderly Patients with Extremity Soft-Tissue Sarcoma. Ann Surg Oncol 2016;23:2577-85. [Crossref] [PubMed]
- Toulmonde M, Le Cesne A, Mendiboure J, et al. Long-term recurrence of soft tissue sarcomas: prognostic factors and implications for prolonged follow-up. Cancer 2014;120:3003-6. [Crossref] [PubMed]
- Dagan R, Indelicato DJ, McGee L, et al. The significance of a marginal excision after preoperative radiation therapy for soft tissue sarcoma of the extremity. Cancer 2012;118:3199-207. [Crossref] [PubMed]
- Major FJ, Blessing JA, Silverberg SG, et al. Prognostic factors in early-stage uterine sarcoma. A Gynecologic Oncology Group study. Cancer 1993;71:1702-9. [Crossref] [PubMed]
- Seagle BL, Sobecki-Rausch J, Strohl AE, et al. Prognosis and treatment of uterine leiomyosarcoma: A National Cancer Database study. Gynecol Oncol 2017;145:61-70. [Crossref] [PubMed]
- Merrill RM, Johnson E. Benefits of marriage on relative and conditional relative cancer survival differ between males and females in the USA. J Cancer Surviv 2017;11:578-89. [Crossref] [PubMed]
- Machida H, Nathenson MJ, Takiuchi T, et al. Significance of lymph node metastasis on survival of women with uterine adenosarcoma. Gynecol Oncol 2017;144:524-30. [Crossref] [PubMed]
- Keung EZ, Chiang YJ, Voss RK, et al. Defining the incidence and clinical significance of lymph node metastasis in soft tissue sarcoma. Eur J Surg Oncol 2018;44:170-7. [Crossref] [PubMed]