Value of radiomics model based on multi-parametric magnetic resonance imaging in predicting epidermal growth factor receptor mutation status in patients with lung adenocarcinoma
Introduction
Lung adenocarcinoma is the most common histological type of lung cancer. The discovery of epidermal growth factor receptor (EGFR) mutations has revolutionized lung adenocarcinoma treatment (1,2). EGFR tyrosine kinase inhibitors can target specific mutations within the EGFR gene and improve EGFR-mutant lung adenocarcinoma patients' outcomes. Lung cancer patients with EGFR mutations have longer progression-free survival compared to those without mutations (3,4).
Mutational sequencing of biopsies has become the gold standard for EGFR mutation detection. However, biopsy testing is not available to all lung cancer patients because of technical difficulties or tumor heterogeneity (5,6). Besides, studies have suggested that biopsy testing may increase the risk of cancer metastasis (7). Furthermore, repeated tumor sampling, difficulty accessing tissue samples, poor DNA quality (8), and relatively high costs can limit the use of mutational sequencing (8).
Over the last decade, biomedical engineers have been working on developing non-invasive and convenient methods to predict EGFR mutation status. As a non-invasive, radiation-free technique, magnetic resonance imaging (MRI) has attracted increasing attention for evaluating lung cancer. Both morphological and functional sequences are effective in the assessment of different pulmonary lesions (9-11). Recently, pulmonary nodule characterization using MR has been recommended for clinical use (12,13).
Over recent years, radiomics has been widely used in the early diagnosis, efficacy evaluation, and prognosis prediction of tumors. Previous studies indicated that radiomics has great clinical potential and is expected to become a new biomarker with satisfactory predictive performance (14-17). Currently, radiomics research for predicting lung cancer gene mutations has been mainly based on CT or PET/CT images (18-20), while only a few studies used the MRI. Several attempts were made using advanced diffusion models, such as intravoxel incoherent motion or diffusion kurtosis imaging, along with histogram analysis to predict EGFR mutation status in stage IIIA–IV lung adenocarcinoma (21). However, compared with conventional diffusion-weighted imaging (DWI), these advanced diffusion models lack a unified standard for b values, especially when used in the lung (10). DWI is more convenient for clinical applications. Also, histogram as the first-order feature ignores the spatial relationship between pixels.
In this study, multiparametric MR-based radiomics, extracting texture features from both functional and morphological images, was applied to develop and validate a radiomics signature to predict EGFR mutation status in preoperative lung adenocarcinoma.
We present the following article in accordance with the STROBE reporting checklist (available at http://dx.doi.org/10.21037/jtd-20-3358).
Methods
Patients
The study conformed to the provisions of the Declaration of Helsinki (as revised in 2013). Ethical approval is not required for this study as it is based on information collected as part of routine clinical practice. Informed consent was waived because of the retrospective design. We retrospectively analyzed data from patients who met the following criteria from November 2015 to September 2019. Inclusion criteria were: (I) solid pulmonary lesions detected by CT with a maximum diameter ≥10 mm, and patients who did not receive radiotherapy or chemotherapy; (II) patients had no contraindications related to MRI examination and could cooperate to complete the routine scanning of chest MRI; (III) after the completion of the MRI examination, lung adenocarcinoma was pathologically confirmed by surgical resection, puncture, or fiberoptic bronchoscopy biopsy; (IV) all patients were tested for EGFR gene mutations; (V) all patients had complete clinical data. A total of 92 patients who met the above criteria were included in the study.
Training and test groups were generated by the R software with the function “createDataPartition” in the “caret” package, and all patients were randomly divided into training and test groups in a ratio of 7:3. There were 65 cases in the training group (39 cases of EGFR mutant type, 26 cases of wild type) and 27 cases in the test group (12 cases of EGFR mutant type, 15 cases of wild type).
MRI acquisition
All patients received the same T2 weighted imaging (T2WI) and echo-planar imaging/DWI (EPI-DWI) sequence scanning (Achieva, Philips Healthcare, Best, The Netherlands). A sense-XL-torso body coil was used. Sensitivity encoding imaging (SENSE) technology was applied during the scanning. MRI sequences included coronal T2WI, transverse T2WI, and EPI-DWI. The conventional sequence was scanned under respiratory triggering. DWI was performed during free-breathing. The scanning range was from the thoracic inlet to the lower margin of the diaphragm. The images were exported in DICOM format. The scanning parameters were as follows: transverse T2WI: T2WI/turbo spin-echo (TSE), repetition time (TR)/echo time (TE) (ms) =992/80, number of signal averages (NSA) =1, field of view (FOV) =430 mm × 350 mm, matrix 640×640, thickness/gap (mm) =5.0/0.5, scanning time: 23 seconds; transverse EPI-DWI: TR/TE (ms) =1,306/54, NSA =3, FOV =430 mm×336 mm, matrix 144×109, thickness/gap (mm) =5.0/0.5, scanning time: 1 minute and 5 seconds, B value selected 0, 20, 40, 200, 800 s/mm2.
EGFR gene mutation test
EGFR gene mutation tests were performed within 2 weeks after MR examination. Histological specimens obtained by surgical resection or puncture using the Applied Biosystems (ABI7500) real-time PCR machine were used to analyze the EGFR gene mutation, using a Human EGFR Gene Mutation Detection Kit (Xiamen Aide Biological Co., Ltd.). If exon mutation was detected, the tumor was identified as an EGFR mutation; otherwise, the tumor was identified as EGFR wild type. The researchers did not know the predicted results of radiomics while testing.
Lesion segmentation
Using the open-source software ITK-SNAP (ITK-snap v.3.6.0, http://www.itksnap.org) to assess the T2WI, DWI, and apparent diffusion coefficient (ADC) images, we manually delineated and segmented all the layers of the lesion, layer by layer, placing a region of interest (ROI) along the contour of the lesion, including the entire tumor, and excluding the visible air area (Figure 1). The ROI of lesions was manually determined by two radiologists with 3 and 8 years of experience, with unanimous agreement. Radiologists were blind to grouping and genetic test results.
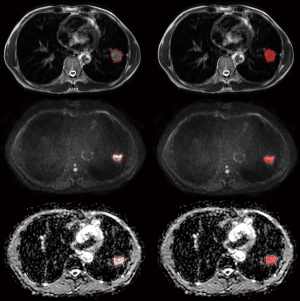
Image preprocessing
The original images of all cases and the segmented tumor ROI file (.NII format) were imported into the AK software of GE (Artificial Intelligence Kit). The following parameters were selected in the image preprocessing module: for MR model resampling, X-spacing, Y-spacing, and Z-spacing were set to 1.000 mm; for intensity standardization, the parameters were gray-level discretization: 0.0, desired maximum: 255.0; the discretization time period was set to gray discretization: 64 (Figure 2).
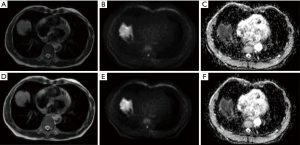
Extraction of features
The preprocessed images and corresponding ROIs were imported into AK analysis software. Six categories of feature parameters, including histogram, form factor, gray-level co-occurrence matrix (GLCM), Haralick features, gray-level run-length matrix (GLRLM), and gray-level size zone matrix (GLSZM) were selected for feature extraction. A total of 396 image features were extracted from each sequence of each case, including 42 histogram features, 9 morphological features, 144 GLCM features, 10 Haralick features, 180 GLRLM features, and 11 GLSZM features.
Radiomics feature selection and radiomics model building
Three MR sequence images (ADC, DWI, T2WI) from 92 patients were included, and 396 features were extracted from each sequence of each patient. R software (version 3.0.1, http://www.Rproject.org) was used for the statistical analysis of characteristic parameters for all load corresponding packages or functions; the corresponding model was established, and statistical tests were performed. Prediction models based on radiomics feature parameters included the ADC model, DWI model, T2WI model, multi-sequence model, and nomogram.
Using the Spearman correlation coefficients generated by correlation analysis to eliminate features above 0.9 characteristic parameters, we used the least absolute shrinkage and selection operator (LASSO) algorithm, logistic regression, and 10-fold cross-validation to build radiomics models. Further forecasting may ultimately select characteristic parameters to establish the image of omics labels. The final features were selected in each model, and a corresponding radiomics score (Radscore) for each patient was calculated. The Radscore obtained by the multisequence model was used to construct the nomogram. The area under the receiver operating characteristic (ROC) curve (AUC) was used to evaluate the radiomics prediction model’s diagnostic efficacy in the preoperative prediction of EGFR mutation status in lung adenocarcinoma to calculate the accuracy, sensitivity, specificity, and accuracy. The DeLong test was used to compare the AUCs of five models in the training group and the validation group. P<0.05 was considered statistically significant. Decision curve analysis (DCA) was used to evaluate each model's benefits in different threshold probabilities.
A significant clinical predictor was obtained through univariate analysis of the relationship between each variable and EGFR mutation in lung adenocarcinoma. Then, a combined model including radiomics labels and clinical predictors was established through multifactor logistic regression, and a nomogram based on radiomics was constructed. A calibration curve was used to evaluate the predictive ability of the nomogram. The total scores of 92 patients with EGFR mutations were calculated according to the nomogram and divided patients into high-risk and low-risk groups. The chi-square test was used to compare the number of patients between the high/low-risk groups and the actual EGFR mutation/wild type groups to determine the clinical effect of the nomogram.
Statistical analysis
The clinical data and MRI features of 92 patients were analyzed by SPSS Statistics 25.0 software, and P<0.05 was considered statistically significant. For the comparison of demographic characteristics, normality and homogeneity of variance tests were performed according to age, maximum tumor diameter, levels of carcinoembryonic antigen (CEA), carbohydrate antigen 125 (CA125), and carbohydrate antigen 153 (CA153), and ADC values of patients in the wild type group, the training group and the verification group. The differences between the two groups were compared by independent sample t-test or Mann-Whitney U test. The chi-square test was used to compare sex, smoking history, tumor stage, lobules, burr signs, and chest cavity signs between the wild-type group and the mutant group.
Results
Clinical characteristics
The patients’ clinical characteristics are summarized in Tables 1,2. Among 92 patients with lung adenocarcinoma, 53 were males and 39 were females, aged 27 to 79 years old (57.65±10.90). There were 51 cases with EGFR mutation and 41 cases with EGFR wild type. With reference to clinical staging, 34 cases were stage I, 14 cases were stage II, 20 cases were stage III, and 24 cases were stage IV. There were 28 patients with a smoking history and 64 patients without a smoking history. The CEA level ranged from 0.27 to 3,101 ng/mL, with an average value of 106.06±412.86 ng/mL; CA125 ranged from 2.57 to 2,014 U/mL, with an average value of 86.57±248.13 U/mL; CA153 ranged from 4.57 to 181.60 U/mL, with an average value of 27.32±30.80 U/mL. Considering the MRI morphological features of 92 patients, 27 patients had the tumor in the right upper lung, 10 patients had the tumor in the right middle lung, 18 patients had the tumor in the right lower lung, 24 patients had the tumor in the left upper lung, and 13 patients in the left lower lung. The maximum tumor diameter range was 1.02–8.93 cm, with an average of 3.90±1.99 cm. There were 53 cases without lobular signs, 39 cases with lobular signs, 67 cases without spicule signs, 25 cases with spicule signs, 68 cases without pleural indentation signs, and 24 cases with pleural indentation signs. ADC values ranged from 603 to 2,335 mm2/s, with an average value of 1,171.59±286.67 mm2/s. There were statistically significant differences in sex, smoking status, and ADC values between the EGFR mutant and wild type groups (P<0.001, P<0.001, P=0.04, respectively).
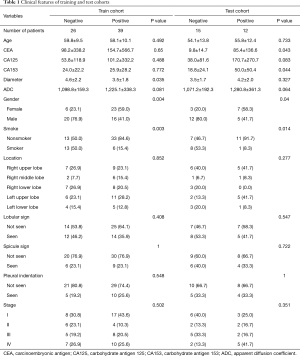
Full table
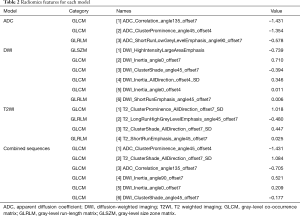
Full table
Model performance
There were 65 cases in the training group, including 39 cases with EGFR mutation and 26 cases with EGFR wild type; there were significant differences in sex and smoking status in the training group (P=0.004, P=0.003, respectively). There were 27 patients in the test group, including 12 patients with EGFR mutations and 15 patients without EGFR mutations; there were significant differences in sex and smoking status of the patients with and without mutations in the test group (P=0.04, P=0.014, respectively). Sex and smoking status were screened as clinical factors for predicting EGFR mutation status in lung adenocarcinoma. After screening the features extracted from ADC, DWI, T2WI, and combined sequences, 3, 6, 4, and 6 radiomics features remained, respectively. Among the three single-sequence models, the ADC model has the best prediction performance. The AUCs of the training group and the test group in the ADC model were 0.873 (95% CI: 0.785 to 0.961) and 0.805 (95% CI: 0.610 to 1.000), respectively. Compared to the ADC model, the prediction efficiency of the DWI model and T2WI model was relatively lower, with AUCs equaling 0.784 (95% CI: 0.674 to 0.893) and 0.722 (95% CI: 0.519 to 0.924) in the training group and 0.692 (95% CI: 0.563 to 0.821) and 0.655 (95% CI: 0.438 to 0.872) in the test group. However, compared with the single-sequence model, the multi-sequence model achieved even better performance; the AUC in the training and test group was 0.888 (95% CI: 0.808 to 0.968) and 0.838 (95% CI: 0.685 to 0.992), respectively (Figure 3). The AUC of the nomogram (Figure 4) with sex, smoking status, and radiomics signatures in the training group was 0.925 (95% CI: 0.855 to 0.994), with a sensitivity of 84.6%, a specificity of 96.2%, and an accuracy of 89.2%. The AUC of the test group was 0.727 (95% CI: 0.531 to 0.924), while the sensitivity was 66.7%, the specificity was 73.3%, and the accuracy was 70.4%. The calibration curve for the probability of EGFR mutation in the training and the validation group demonstrated good agreement between prediction and observation (Figure 5).
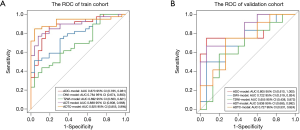
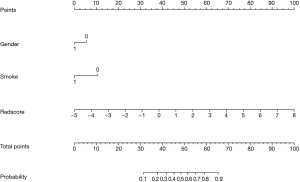
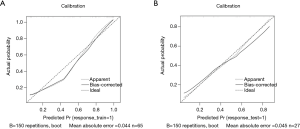
Discussion
To the best of our knowledge, this is the first study that predicted EGFR mutation in patients with lung adenocarcinoma using MR radiomics. We found that the ADC and DWI models were slightly superior to T2WI models in predicting the risk of EGFR gene mutations in lung adenocarcinoma. The prediction performance of the multi-sequence radiomics model was further improved compared to that of the single-sequence model. The nomogram based on MRI radiomics signature and clinical factors could be used as a quantitative tool; however, it did not outperform the radiomics-only model.
In this study, we analyzed the relationship between traditional imaging signs and EGFR mutation. We found no significant correlation between imaging signs (including tumor location, maximum diameter, lobular sign, spicule sign, pleural indentation sign) and EGFR mutation status. Some studies reporting on the relationship between the traditional CT imaging signs of lung cancer and EGFR mutation also reported inconsistent results (22,23). On the one hand, these discrepancies may be caused by different patient inclusion criteria, sample size, and ethnic difference; on the other hand, the evaluation of image signs is subjective, and doctors with different professional background may have different understanding of the same imaging sign. Therefore, we believe that it is very challenging to determine EGFR mutation status merely from imaging signs.
It has been reported that radiomics features can predict the mutation status of EGFR (19,20). However, these radiomics studies on the prediction of lung cancer gene mutations are mainly based on CT or PET/CT images. As a promising radiation-free biomarker, ADC performs well in tumor detection and characterization, treatment response assessment, and tumor staging, and could also reflect biological characteristics (24-26). In our study, the radiomics method was applied to multi-parametric MR images to extract and integrate the texture features of both biological and anatomical information. The combination of multiple MRI sequences, such as T2WI and DWI, has been proven to provide complementary information for tumor characterization (27). According to our results, the predictive efficacy of the ADC and DWI models was better than that of the T2WI model, possibly because the dense area of tumor cells has a high signal on DWI, while the sparse area has a low signal. At the same time, ADC images basically have inverse patterns. Such image contrast may better reflect the heterogeneity inside the tumor than T2W images. Compared with the single-sequence model, the combined model has a slightly better predictive efficiency, which is consistent with a previous MR radiomics study (28) on the classification of pulmonary diseases. This suggests that the radiomics features extracted from each sequence contain complementary information needed for EGFR status prediction, thus providing more valuable information.
Our results showed that the EGFR mutation in lung adenocarcinoma was significantly more common in women and nonsmokers than in other patients, which is consistent with previous studies (29,30). The combination of sex and smoking status, two clinical factors, with radiomics constituted the nomogram. However, we found that the nomogram had a lower predictive performance than the radiomics-only model. One possible explanation much lower contribution of clinical factors in the current nomogram compared to the contribution of radiomics features.
In this study, 3, 6, and 4 features were selected from ADC, DWI, and T2WI, respectively, to form radiomics models, which were mainly derived from GLCM, GLRLM, and GLSZM. These three types of features all belong to the high-order texture features, which reflect image uniformity and heterogeneity, and cannot be perceived by the naked eye. This indicated that texture features associated with image uniformity and heterogeneity were correlated with EGFR mutation status in lung adenocarcinoma. Yang et al. (31) reported that radiomic features combined with clinicopathological factors could reflect genetic differences and predict EGFR mutation type. Moreover, Zhang et al. (18) suggested that PET/CT-based texture features (from GLCM, GLZLM, and so on) have a good performance in predicting EGFR mutation. We found that adenocarcinoma with EGFR mutation was more heterogeneous than those with EGFR wild lung cancer, which is in line with the studies mentioned above.
Recent advances in MR technology are expanding clinical opportunities for MR application in the lungs, and the lack of radiation makes it an ideal model for pediatric examinations, pregnant women, and patients needing continuous and longitudinal follow-up. Pulmonary nodule characterization using MR is recommended for clinical use (12,13). Therefore, the potential clinical significance of our research is the following: (I) it provides a relatively accurate, convenient, and non-invasive method for predicting EGFR mutation, which is suitable for patients sensitive to radiation or with contraindications to biopsies; (II) patients requiring serial follow-up do not have to worry about radiation, and the mutation status of EGFR can be dynamically observed.
There are some limitations in the present study. First, the fact that this was a single-center design and relatively small sample size may affect the generalization ability of the model. In the future, multicenter experiments with a larger sample size are needed. Second, lesion segmentation was not automatic and thus could be susceptible to potential human error. Lastly, deep learning models should be developed and compared with different modeling approaches to achieve optimal predictive properties.
In conclusion, our study showed that MR radiomics models could reveal different imaging phenotypes for EGFR mutations. Our results provide a basis for the use of MR radiomics for the accurate prediction of gene mutations, which may also be used as a reference for personalized, targeted therapy of lung cancer.
Acknowledgments
We thank MedSci for providing language editing services.
Funding: This work is supported by the National Natural Science Foundation of China (81601457), the Foundation of Guangzhou Municipal Science and Technology Bureau (202102010253), Guangdong Demonstration Base for Joint Training of Graduate Students (20201), and Open Project Fund of the Sixth Affiliated Hospital of Guangzhou Medical University (2020-11-370).
Footnote
Reporting Checklist: The authors have completed the STARD reporting checklist. Available at http://dx.doi.org/10.21037/jtd-20-3358
Data Sharing Statement: Available at http://dx.doi.org/10.21037/jtd-20-3358
Conflicts of Interest: All authors have completed the ICMJE uniform disclosure form (available at http://dx.doi.org/10.21037/jtd-20-3358). The authors have no conflicts of interest to declare.
Ethical Statement: The authors are accountable for all aspects of the work in ensuring that questions related to the accuracy or integrity of any part of the work are appropriately investigated and resolved. The study conformed to the provisions of the Declaration of Helsinki (as revised in 2013). Ethical approval is not required for this study as it is based on information collected as part of routine clinical practice. Informed consent was waived because of the retrospective design.
Open Access Statement: This is an Open Access article distributed in accordance with the Creative Commons Attribution-NonCommercial-NoDerivs 4.0 International License (CC BY-NC-ND 4.0), which permits the non-commercial replication and distribution of the article with the strict proviso that no changes or edits are made and the original work is properly cited (including links to both the formal publication through the relevant DOI and the license). See: https://creativecommons.org/licenses/by-nc-nd/4.0/.
References
- Sequist LV, Yang JC, Yamamoto N, et al. Phase III study of afatinib or cisplatin plus pemetrexed in patients with metastatic lung adenocarcinoma with EGFR mutations. J Clin Oncol 2013;31:3327-34. [Crossref] [PubMed]
- Maemondo M, Inoue A, Kobayashi K, et al. Gefitinib or chemotherapy for non-small-cell lung cancer with mutated EGFR. N Engl J Med 2010;362:2380-8. [Crossref] [PubMed]
- Li T, Kung HJ, Mack PC, et al. Genotyping and genomic profiling of non-small-cell lung cancer: implications for current and future therapies. J Clin Oncol 2013;31:1039-49. [Crossref] [PubMed]
- Zhou C, Wu YL, Chen G, et al. Erlotinib versus chemotherapy as first-line treatment for patients with advanced EGFR mutation-positive non-small-cell lung cancer (OPTIMAL, CTONG-0802): a multicentre, open-label, randomised, phase 3 study. Lancet Oncol 2011;12:735-42. [Crossref] [PubMed]
- Sacher AG, Dahlberg SE, Heng J, et al. Association between younger age and targetable genomic alterations and prognosis in non-small-cell lung cancer. JAMA Oncol 2016;2:313-20. [Crossref] [PubMed]
- Itakura H, Achrol AS, Mitchell LA, et al. Magnetic resonance image features identify glioblastoma phenotypic subtypes with distinct molecular pathway activities. Sci Transl Med 2015;7:303ra138 [Crossref] [PubMed]
- Loughran CF, Keeling CR. Seeding of tumour cells following breast biopsy: a literature review. Br J Radiol 2011;84:869-74. [Crossref] [PubMed]
- Rios Velazquez E, Parmar C, Liu Y, et al. Somatic mutations drive distinct imaging phenotypes in lung cancer. Cancer Res 2017;77:3922-30. [Crossref] [PubMed]
- Meier-Schroers M, Homsi R, Schild HH, et al. Lung cancer screening with MRI: characterization of nodules with different non-enhanced MRI sequences. Acta Radiol 2019;60:168-76. [Crossref] [PubMed]
- Wan Q, Deng YS, Lei Q, et al. Differentiating between malignant and benign solid solitary pulmonary lesions: are intravoxel incoherent motion and diffusion kurtosis imaging superior to conventional diffusion-weighted imaging? Eur Radiol 2019;29:1607-15. [Crossref] [PubMed]
- Brea TP, Ravina AR, Villamor JMC, et al. Use of magnetic resonance imaging for N-staging in patients with non-small cell lung cancer. A systematic review. Arch Bronconeumol 2019;55:9-16. [PubMed]
- Schiebler ML, Parraga G, Gefter WB, et al. Synopsis from expanding applications of pulmonary MRI in the clinical evaluation of lung disorders: Fleischner Society Position Paper. Chest 2021;159:492-5. [Crossref] [PubMed]
- Hatabu H, Ohno Y, Gefter WB, et al. Expanding applications of pulmonary MRI in the clinical evaluation of lung disorders: Fleischner Society Position Paper. Radiology 2020;297:286-301. [Crossref] [PubMed]
- Sun Y, Reynolds HM, Parameswaran B, et al. Multiparametric MRI and radiomics in prostate cancer: a review. Australas Phys Eng Sci Med 2019;42:3-25. [Crossref] [PubMed]
- Li Z, Yu L, Wang X, et al. Diagnostic performance of mammographic texture analysis in the differential diagnosis of benign and malignant breast tumors. Clin Breast Cancer 2018;18:e621-7. [Crossref] [PubMed]
- Wu W, Parmar C, Grossmann P, et al. Exploratory study to identify radiomics classifiers for lung cancer histology. Front Oncol 2016;6:71. [Crossref] [PubMed]
- Islam A, Reza SM, Iftekharuddin KM. Multifractal texture estimation for detection and segmentation of brain tumors. IEEE Trans Biomed Eng 2013;60:3204-15. [Crossref] [PubMed]
- Zhang J, Zhao X, Zhao Y, et al. Value of pre-therapy 18F-FDG PET/CT radiomics in predicting EGFR mutation status in patients with non-small cell lung cancer. Eur J Nucl Med Mol Imaging 2020;47:1137-46. [Crossref] [PubMed]
- Li S, Ding C, Zhang H, et al. Radiomics for the prediction of EGFR mutation subtypes in non-small cell lung cancer. Med Phys 2019;46:4545-52. [Crossref] [PubMed]
- Jia TY, Xiong JF, Li XY, et al. Identifying EGFR mutations in lung adenocarcinoma by noninvasive imaging using radiomics features and random forest modeling. Eur Radiol 2019;29:4742-50. [Crossref] [PubMed]
- Yuan M, Pu XH, Xu XQ, et al. Lung adenocarcinoma: assessment of epidermal growth factor receptor mutation status based on extended models of diffusion-weighted image. J Magn Reson Imaging 2017;46:281-9. [Crossref] [PubMed]
- Rizzo S, Petrella F, Buscarino V, et al. CT radiogenomic characterization of EGFR, K-RAS, and ALK mutations in non-small cell lung cancer. Eur Radiol 2016;26:32-42. [Crossref] [PubMed]
- Ko KH, Hsu HH, Huang TW, et al. Value of 18F-FDG uptake on PET/CT and CEA level to predict epidermal growth factor receptor mutations in pulmonary adenocarcinoma. Eur J Nucl Med Mol Imaging 2014;41:1889-97. [Crossref] [PubMed]
- Çakmak V, Ufuk F, Karabulut N. Diffusion-weighted MRI of pulmonary lesions: comparison of apparent diffusion coefficient and lesion-to-spinal cord signal intensity ratio in lesion characterization. J Magn Reson Imaging 2017;45:845-54. [Crossref] [PubMed]
- Koyama H, Ohno Y, Seki S, et al. Value of diffusion-weighted MR imaging using various parameters for assessment and characterization of solitary pulmonary nodules. Eur J Radiol 2015;84:509-15. [Crossref] [PubMed]
- Koşucu P, Tekinbaş C, Erol M, et al. Mediastinal lymph nodes: assessment with diffusion-weighted MR imaging. J Magn Reson Imaging 2009;30:292-7. [Crossref] [PubMed]
- Malayeri AA, El Khouli RH, Zaheer A, et al. Principles and applications of diffusion-weighted imaging in cancer detection, staging, and treatment follow-up. Radiographics 2011;31:1773-91. [Crossref] [PubMed]
- Wang X, Wan Q, Chen H, et al. Classification of pulmonary lesion based on multiparametric MRI: utility of radiomics and comparison of machine learning methods. Eur Radiol 2020;30:4595-605. [Crossref] [PubMed]
- Chen Y, Yang Y, Ma L, et al. Prediction of EGFR mutations by conventional CT-features in advanced pulmonary adenocarcinoma. Eur J Radiol 2019;112:44-51. [Crossref] [PubMed]
- Girard N, Sima CS, Jackman DM, et al. Nomogram to predict the presence of EGFR activating mutation in lung adenocarcinoma. Eur Respir J 2012;39:366-72. [Crossref] [PubMed]
- Yang B, Ji HS, Zhou CS, et al. 18F-fluorodeoxyglucose positron emission tomography/computed tomography-based radiomic features for prediction of epidermal growth factor receptor mutation status and prognosis in patients with lung adenocarcinoma. Transl Lung Cancer Res 2020;9:563-74. [Crossref] [PubMed]