Identification of genes associated with sudden cardiac death: a network- and pathway-based approach
Introduction
Sudden cardiac death (SCD) can either be caused by cardiac-related or unknown factors, both of which ultimately lead to a sudden, nonviolent, natural death, occurring within 1 hour of the onset of acute symptoms (1,2). In Western countries, SCD is one of the most common causes of death. At present, approximately 250,000–350,000 people die of SCD every year in America, accounting for 63% of all deaths due to heart disease (3). The majority of these events occur in older adults, and are often concurrent with coronary heart disease (4,5). Children, teenagers, and young adults—but not infants—are the other major groups that suffer from SCD (6-8). In China, SCD results in more than 544,000 deaths each year (9). Over the past decades, there have been some progressive declines in other heart diseases due to major advances in treatment and preventive measures; in contrast, the SCD rate has only seen a slight reduction. Although a few factors, including atherosclerotic cardiovascular disease (CVD), may account for a large proportion risk for SCD, the pathogenesis and especially the molecular mechanisms underlying SCD are still not fully understood. Therefore, in the last decade, in order to allow for an early diagnosis and prevention of SCD for a significant percentage of young individuals, scientists have paid considerable attention to the molecular analysis of cardiac channelopathies and cardiomyopathies (10). Numerous studies have helped us to understand the pathological and molecular mechanisms of SCD. For example, it was found that the ATP-binding cassette B1 (ABCB1) gene encodes P-glycoprotein, which plays a vital role in digoxin bioavailability. The researchers found that, in users who had all three loci mutations of the ABCB1 gene, digoxin was much more highly related to SCD than in people who had no or only 1 T allele (11). Several other genes that are associated with SCD also have been found, such as genes encoding calcium-handling regulating proteins (RyR2, CASQ2, ATP2A2, NOS1AP, TRDN and CALM1) (12-17), long QT syndrome (KCNJ5, KCNJ2, KCNE2, AKAP9, SNTA1, and AKAP9), idiopathic ventricular fibrillation (DPP6 and KCNJ8), dilated cardiomyopathy (DCM) (NEBL), and hypertrophic cardiomyopathy (HCM) (NEXN) (18,19).
In our study, we comprehensively collected the SCD-related genes from published genetic association studies. Then, to identify the significant functional themes within these genetic factors, we performed biological enrichment analysis; to analyze the interactions among the enriched biochemical pathways, we conducted pathway crosstalk analysis. In order to further study the pathogenesis of the SCD in a more specific manner, an SCD-specific molecular network was constructed and evaluated within the frame of a human protein–protein interaction network. Two SCD-specific protein networks were inferred: one based on the Search Tool for the Retrieval of Interacting Genes/Proteins (STRING) database via the Markov cluster algorithm, and the other based on Pathway Commons via the Steiner minimal tree algorithm. This research should give us valuable insights into the molecular mechanisms of SCD, and are likely to yield potential biomarkers for useful diagnostic and therapeutic strategies in SCD prevention.
Methods
Collection of SCD-associated genes
The study was conducted in accordance with the Declaration of Helsinki (as revised in 2013). We searched SCD-associated candidate genes by retrieving the human genetic association studies deposited in the PubMed (https://www.ncbi.nlm.nih.gov/pubmed/) and Gene-Cloud Biotechnology Information (GCBI) databases (https://www.gcbi.com.cn). We searched for reports linked to SCD with the following terms: (sudden cardiac death [MeSH]) and (genotype [MeSH] or alleles [MeSH]) or (polymorphism [MeSH]). By December 31, 2017, a total of 2,076 publications were retrieved from PubMed. Furthermore, we searched the publications that clearly mentioned 1 or more genes significantly related to SCD in the GCBI database, and 1,094 publications were retrieved. We reviewed all the abstracts of these publications, and chose the genetic studies of SCD. To remove the false-positive findings, the publications reporting negative or insignificant association were excluded. To ensure the conclusions were supported by the content, we reviewed the full articles of the remaining publications. From these studies, genes that were reported to be significantly related to SCD were chosen for our study. In some studies, several genes were reported have high associations with SCD, while the actual effects were found to be moderate; however, based on our inclusion criteria of gene collection, these genes were included in our study. Moreover, genes from genome-wide association studies (GWAS) that had a demonstrated genetic association with SCD at a significant genome-wide level or that were frequently mentioned in the original publications were included. Finally, we collected 257 genes that were related to SCD, and referred to these as the SCD-related gene set (SCDgset), for further study. To check the mRNA expression of each gene in normal human heart, three different databases: Genotype-Tissue Expression (GTEx) database (https://gtexportal.org/home/topExpressedGenePage), BioGPS (http://biogps.org/#goto=welcome) and Serial Analysis of Gene Expression (SAGE, https://www.genecards.org/) were applied.
Functional enrichment analysis of genes related to SCD
To analyze the biological themes of the SCD-related genes, we used the functional annotation tool DAVID (Database for Annotation, Visualization and Integrated Discovery; https://david.ncifcrf.gov/summary.jsp) with the Kyoto Encyclopedia of Genes and Genomes (KEGG) database (https://www.kegg.jp/) and overrepresentation analysis (ORA), functional class scoring (FCS), and pathway topology (PT)-based approaches. DAVID is able to organize and condense a wide range of heterogeneous annotation content, such as Gene Ontology (GO) terms, protein domains, pathways etc., into term or gene classes (20,21). However, this database is updated relatively slowly. To gain insight into the underlying biology of differentially expressed genes and proteins, pathway analysis should be the first choice. For obtaining the most recent data and pathway enrichment, we combined the data from KEGG with that from DAVID and analyzed the enrichment by ORA, FCS, and PT-based approaches that provided us better specificity, sensitivity, and relevance in pathway analysis (22,23). It has been reported that the higher hierarchical level of GO terms in the tree structure is, the more explicit the biological function demonstrated (24). Therefore, only the leaf GO terms of biological processes with a false discovery rate (FDR) value less than 0.05 were kept as the remarkably enriched terms. We extracted all the pathways when 1 or more genes overlapped with candidate genes, and Fisher’s exact test for P value assignment was used to denote the significant overlap between the pathway and the input genes. The pathways with an FDR value less than 0.05 and which contained at least 5 genes were considered to be significantly enriched. Since there were numerous of pathways involved in cancer, we excluded these pathways.
Analysis of pathway crosstalk
To investigate interlinks and interactions of the enriched pathways, we further performed pathway crosstalk analysis. For the measurement of the overlap between any given pathway pair, we calculated the overlap coefficient (OC) () and the Jaccard coefficient (JC) () by the corresponding formulas, in which A and B are the gene lists contained in the two examined pathways. To build the pathway crosstalk, we executed the following procedure:
- We only chose the pathways with an FDR value less than 0.05 and which contained more than five candidate genes that would give us sufficient biological information;
- We counted the common candidate genes of each pathway pair which had more than two overlapped genes. Furthermore, the pathway crosstalk needed to match with KEGG;
- We calculated the overlap in every pathway pair and ranked them according to their JC and OC values;
- We constructed pathway crosstalk via Cytoscape software (25).
Establishment of the human protein-protein interaction network
To deduce the interaction and correlation between the SCD-related genes, we constructed a relatively comprehensive and dependable human interactome and inferred and analyzed the potential topological characteristics of the SCDgset molecular network. By pooling and curating the nonredundant physical interaction data from the STRING database (26), we selected the interactions with a score more than 0.6 and which were approved with reported experimental data or database annotation. Then, to cluster the interaction network and recover clusters of associated interactions, Markov cluster algorithm, which is an algorithm for clustering graph flow simulation, was used at an inflation value of 3.0 (27). Finally, we established a relatively full-scale human physical interactome, which contained 196 genes and 653 edges.
Construction of the SCD-specific network
A subnetwork specific to certain diseases could guide us in clarifying the interaction mechanisms of the disease-related molecules. A network parsimony principle in the context of biological processes has been previously proposed (28), and the Steiner minimal tree algorithm meets this principle (29). We extracted the SCD-specific potential pathological network from Pathway Commons (http://www.pathwaycommons.org) (30), which currently contains data from nine databases with over 1,400 pathways and 687,000 interactions, including biochemical reactions, complex assembly, transport and catalysis events, and physical interactions involving proteins, DNA, RNA, small molecules, and complexes. We used the minimum spanning tree-based approximation (KB) method to search for the Steiner tree from the SteinerNet package (31). Here, we mainly focused on the human database and built the directed networks based on analyzing genes that the regulate state change of the SCDgset, expression of the SCDgset, transport of the SCDgset, and phosphorylation of the SCDgset, which yielded 17,901 genes/proteins and 385,553 interactions.
More specifically, 255 genes associated with SCD were input into the Pathway Commons. Except for C1ORF185, which had no annotation, all the genes in the SCDgset turned out to be involved in the interaction networks (undirected networks). During the process of building the Steiner minimal tree, the reciprocal degree of each node was used as its weight to ease the effect of the genes with extremely high interactions in the network structure. The Erdos-Renyi model in R software “igraph” package was used to obtain the nonrandomness of the constructed network. We then generated 1,000 random networks with the same number of vertices and interactions as the SCD-specific network (32). Next, we calculated the arithmetic average values of the shortest-path distance and clustering coefficient. We evaluated the significance level of nonrandomness according to the number of random networks with an average shortest-path distance (ND) less than that of the SCD-specific networks, and the number of random networks with an average clustering coefficient (NC) more than the observed clustering coefficient. Lastly, the empirical P values using ND/1,000 and NC/1,000 were separately calculated.
Statistical analysis
Functional enrichment of KEGG pathways and GO terms were done based on Fisher’s exact test which is performed by David online system. FDR in DAVID requests adaptive linear step-up adjusted P values for approximate control of the FDR. FDR <0.05 was considered statistically significant.
Results
Identification of SCD-related genes
We reviewed publications on the genetic association studies related to SCD by searching the PubMed and GCBI databases. Only those reported genes that were significantly associated with the SCD were collected, and we discarded those reported as having a negative or insignificant association by the authors of the original literature. In total, from 1,094 publications, we selected 257 genes that were reported to be associated with SCD, and the detailed list of all genes (referred to as the SCDgset) is provided in online table (available at https://cdn.amegroups.cn/static/public/jtd-21-361-1.pdf). Besides, we searched three different databases (GTEx, BioGPS and SAGE) to check all these gene expression patterns. Unsurprisingly, all genes are expressed in normal human heart.
Among these genes, there were 15 genes related to potassium sodium-activated channel (HERG, KCNA5, KCND2, KCND3, KCNE1, KCNE2, KCNE3, KCNE5, KCNJ11, KCNJ2, KCNJ5, KCNJ8, KCNQ1, KCNT1, and KCTD1), six genes encoding sodium voltage-gated channel alpha subunit (SCN10A, SCN1B, SCN2B, SCN3B, SCN4B, and SCN5A), three genes encoding genes that were involved in calcium voltage-gated channel (CACNA1C, CACNA2D1, and CACNB2), four genes belonging to the solute carrier family (SLC12A9, SLC19A2, SLC25A26, and SLC4A4), two transforming growth factor beta genes (TGFB2 and TGFB3), two transforming growth factor beta receptor genes (TGFBR1 and TGFBR2), and three fibroblast growth factors (FGF12, FGF13, and FGF23). A further four tropomyosin family members were collected in the SCDgset (TPM1, TPM2, TPM3, and TPM4). Apparently, the significant SCD-related genes had a diversity of functions but were mostly reported to be involved in cardiac function.
Biological function enrichment analysis of the SCDgset
One more specific biological function array of these SCD-related genes was analyzed by the DAVID website, the results of which are provided in online table (available at https://cdn.amegroups.cn/static/public/jtd-21-361-2.pdf). Among the 383 GO terms overrepresented in the SCDgset, we unsurprisingly found 67 biological functions clearly related to heart diseases, including cardiac muscle contraction, heart development, muscle contraction, cardiac conduction, regulation of heart rate by cardiac conduction, regulation of heart rate, muscle filament sliding, action potential of myocardial cells participating in contraction, regulation of cardiac muscle contraction, histomorphogenesis of ventricular muscle, heart contraction, regulation of atrial cardiac muscle cell membrane depolarization, and regulation of cardiac conduction. Obviously, any of these biological dysfunctions could lead to cardiac diseases, including SCD. In this study, we also found results containing several biological functions that could lead to noncardiac causes of SCD, such as positive/negative regulation of RNA polymerase II promoter transcription and neurologic disorders (negative regulation of neuronal apoptosis, neuron fate commitment, regulation of calcium ion-dependent exocytosis of neurotransmitter, neuron projection morphogenesis, and neurotransmitter receptor metabolic process). Moreover, regulation of cell proliferation, cell adhesion, and apoptotic process were highly enriched in GO terms, while 13 and 22 GO terms related to cell membrane ion channels and transporters were enriched in the results, respectively. As one previous study reported, the cardiac action potential is made possible by active and passive processes that maintain highly regulated electrochemical gradients for sodium, potassium, and calcium ions through cell membrane ion channels and transporters (33). These results demonstrated that the collected candidate genes were relatively dependable for our further study.
Analysis of pathway enrichment in the SCSgset
In order to acquire useful information concerning the pathogenic molecular mechanism underlying SCD, it was necessary to identify the biochemical pathways enriched in the candidate genes. Pathway enrichment of the SCDgset was conducted through using KEGG and DAVID, and 47 significant enrichment pathways for SCD were identified (Table 1). Among these, HCM (ranked first in Table 1), DCM (ranked second in Table 1), and adrenergic signaling in cardiomyocytes (ranked third in Table 1) were highly enriched in the SCDgset. Consistent with previous studies (34,35), HCM is a leading cause of SCD in young adults, and DCM is another major cause of SCD (36,37). Arrhythmogenic right ventricular cardiomyopathy (ARVC) is a genetic form of cardiomyopathy which is usually transmitted with an autosomal dominant pattern, and it accounts for 11–22% of cases of SCD in the young athlete population (38). In this study, we found that ARVC was enriched in the SCDgset at the fourth position. Furthermore, various signaling pathways that are involved in cell metabolism and cell behavior were highly enriched in our results, including calcium signaling pathway, oxytocin signaling pathway, cyclic GMP (cGMP)-protein kinase G (PKG) signaling pathway, cyclic AMP (cAMP) signaling pathway, HIF-1 signaling pathway (responding to hypoxic stress), mitogen-activated protein kinase (MAPK) signaling pathway, and others. Moreover, pathways in cancer, proteoglycans in cancer, pancreatic cancer, and AGE-RAGE signaling pathway in diabetic complications were highly enriched, which is consistent with previous studies which suggest these pathways have important functions in SCD (39,40).
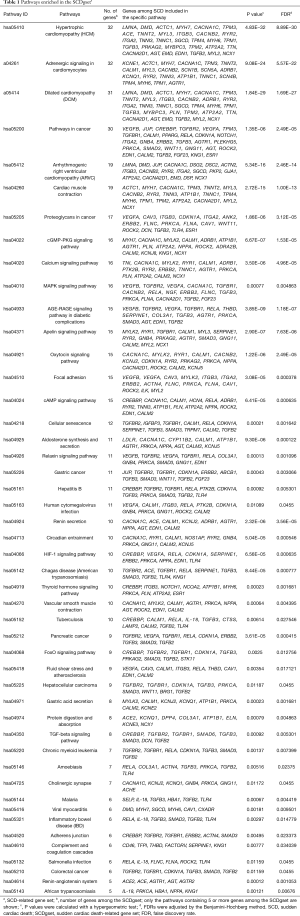
Full table
Crosstalk among significantly enriched pathways
Besides identifying lists of significantly enriched pathways, we also conducted pathway analysis among the 47 enriched pathways to explore the correlations between the pathways and understand how they interact with each other. There were 53 pathways including 5 or more members in the SCDgset. If two pathways shared a proportion in the SCDgset, we assumed that there was a degree of crosstalk between them (41). Only if one pathway shared at least two genes with 1 or more other pathway(s), we considered it to meet the criterion for crosstalk analysis, and 34 pathways met this criterion. To construct the pathway crosstalk network, we used all the pathway pairs (edges). Based on the average scores of the JC and OC, we calculated the overlap significance of each pathway pair.
We roughly divided the pathways into two major modules according to their crosstalk. Each module has more interactions than other pathways, and all the genes from one module might participate in the same/similar biological process (Figure 1). One module primarily is connected by calcium signaling pathway while the other is related to MAPK signaling pathway. GWAS studies have determined that calcium signaling pathway and MAPK signaling pathway are the common pathways between type 2 diabetes (T2D) and coronary artery disease (CAD) (42). The two modules were not independent; instead, the two main pathways were connected directly with each other and indirectly via a few other pathways.
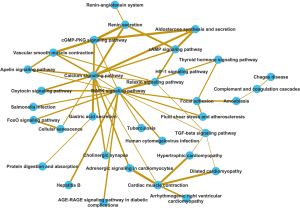
In order to unravel the possible pathological protein network for the SCDgset, 1 subnetwork for SCD from the STRING database via the Markov cluster algorithm was built. The protein network of SCD comprised 196 nodes and 653 edges (interactions; Figure 2). In Figure 2, the proteins labeled in red mainly play important roles in the regulation of heart contraction and ion transport. For example, most of the proteins, such as KCNE1, KCNE1L, KCNE2, KCNE3, and KCNJ5, are involved in the potassium sodium-activated channel, the proteins that belong to the sodium voltage-gated channel alpha subunit, SCN10A, SCN1B, SCN2B, SCN3B, SCN4B, and SCN5A, and the members of the calcium voltage-gated channel, CACNA1C, CACNA2D1, and CACNB2, are all circled in the red part. SCN5A-encoded cardiac sodium channel is the basis of cardiac excitability, and glycerol phosphate dehydrogenase 1-like protein (GPD1L) can be found on the two endpoints of 1 line, meaning they can interact with each other to induce certain functions. It has been reported that dysfunction of sodium current (INa) may lead to fatal ventricular arrhythmia in maladies, including long QT syndrome, sudden infant death syndrome (SIDS), and Brugada syndrome (BrS), and that mutations in GPD1L are also related to SIDS, BrS, and a decrease in INa. Valdivia et al. clarified the relationship between GPD1L and SCN5A: PKC-dependent phosphorylation of SCN5A leads to the connection of redox state and cardiac excitability through GPD1L (43). Here, we depicted the relationship between GPD1L and SCN5A via a protein network in a more intuitive way. The light green-labeled proteins mostly act in the modulation of muscle structure development (Figure 2). Several sarcomeric proteins, such as alpha myosin heavy chain, play crucial roles in cardiac development. According to the reports, mutations in these genes result in congenital heart defects (CHDs), which occur in approximately 1 in 145 live births. Therefore, identifying these potentially pathogenic genes is vital. Tropomyosin 1 (TPM1), an essential sarcomeric component, has multiple roles in the developing heart and in the formation of CHDs (44). Consistent with the previous study, our results showed that TPM1, and other TPM family members, TPM2, TPM3 and TPM4, were all in the light green circle suggesting their roles in cardiac development. Interestingly, we found that junctophilin-2 (JPH2) directly interacts with the proteins (red circles). JPH2 contributes to the construction of skeletal muscle triad junctions and is integral to proper intracellular Ca2+ signaling in cardiac myocytes via its involvement in ryanodine receptor–mediated calcium ion release. Jiang et al. suggested that JPH2 directly or indirectly interacts with Cai-handling proteins, Cav1.2 and KCNQ1 (45). In our results, we demonstrated that the interaction between JPH2 and KCNQ1 is indirect, because ANK2 or/and SCN5A is/are the bridge(s) that connect these two proteins. The remaining proteins outside of the red or light green circles are involved in various other pathways.
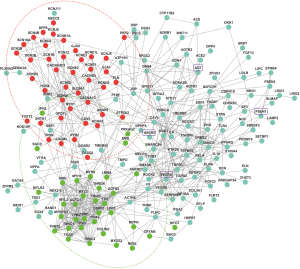
We also extracted the specific network for SCD from Pathway Commons via the Steiner minimal tree algorithm. The SCD protein network comprised 272 edges (interactions) and 273 nodes (Figure 3). To examine the nonrandomness of the extracted network, we created 1,000 random networks by using the Erdos-Renyi model. The average clustering coefficient and shortest-path distance were compared with the corresponding values of the SCD-specific network. The mean shortest-path distance of these random subnetworks was 5.23, while that of the SCD-related network was 3.30. The random networks had an average clustering coefficient (NC) of 0.008, while the SCD distinctive network had an NC of 0. Therefore, we extracted a nonrandom SCD-specific network.
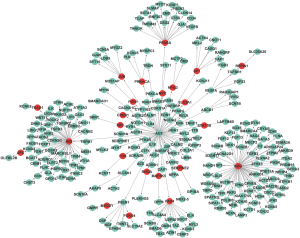
Except for C1ORF185, which had no annotation, all genes in the SCDgset were inside of the SCD-specific network. They accounted for 93.0% of the 273 genes in the network, suggesting a very high coverage rate of the SCDgset in the subnetwork. In the SCD-specific molecular network, we found 23 genes that were enrolled outside of the SCDgset (Table 2). Interestingly, there were 3 big clusters in our directed SCD-specific network (Figure 3). Angiotensinogen (AGT), a gene in the SCDgset, was the jumping-off point of all 3 of these clusters and interacted with noggin (NOG) to extend 1 cluster and with insulin (INS) to spread the third cluster. Surprisingly, NOG and INS were not included in the SCDgset. It is known that AGT plays crucial roles in heart diseases, such as hypertrophy of cardiac myocytes and coronary heart disease (46-48). The bone morphogenetic protein antagonist, NOG, has been shown to be a regulator in mammalian cardiac development in several previous studies (49,50), while INS has been shown to be related to cardiovascular events (51,52). However, no research has demonstrated that AGT can directly interact with NOG or INS. Considering the close interaction between other intermediate genes and known SCD-related genes, they may also participate in the pathological process of disease phenotypes. Interestingly, a number of the genes that occupy the jumping-off points of small clusters, such as presenilin 1 (PSEN1), ATP-binding cassette subfamily B member 1 (ABCB1), and vascular endothelial growth factor A (VEGFA), have been shown to be related to SCD (11,53,54). Although no reports have suggested that any of these 23 genes directly participate in the pathophysiological process of SCD, genes interacting with them or their family have been indicated to have functions in such processes. These include paired box 4 (PAX4), a node in the SCD-specific network that is not part of the SCDgset, was reported as an additional promising candidate of circadian transcriptional regulator, and Asian-specific missense variant in PAX4 associated with T2D in Chinese individuals. However, the genes linking to it were all included in the SCDgset and have been shown relate to heart diseases. Therefore, among these 23 genes, there are several that may figure prominently in SCD susceptibility and may be considered as novel targets for further study.
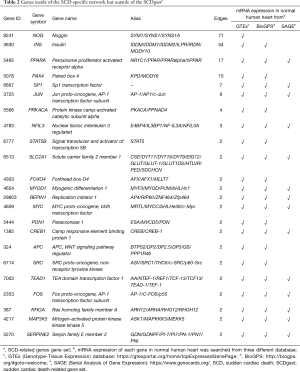
Full table
Discussion
In the past decades, substantial progress has been made to clarify the molecular mechanisms underlying SCD. With the development of high-throughput technology, the elements related to this disease have been identified on a much larger scale. GWAS, in particular, represents a significant step forward in investigating biologic pathways of disease causation and towards identifying a huge number of genes that are involved in SCD (10,37). Even though the numbers of reports regarding the genes and proteins potentially involved in this disease are growing, a thorough understanding of the molecular biological process related to SCD pathogenesis is still far from complete. Therefore, a systematic analysis of SCD-related genes is needed to decode the potential pathogenesis of SCD. In the current study, we collected genetically SCD-associated genes, and explored the interaction of these genes via a pathway-based and network-based analytical framework, with SCD-related biochemical processes and their interactions also being analyzed.
Although some knowledge concerning the factors involving SCD have been obtained from the candidate gene(s)-based genetic association and biochemical studies, our comprehensive analysis on SCD-related has significant advantages. First, we implemented an extensive compilation and curation of genetically SCD-associated human genes, which provided us a valuable gene data source for further study. Moreover, since many diseases are not caused by a single gene and instead are the result of multiple genes working together, each gene can be said to only play a small to medium role in the emergence of disease (4). Considering this, we retrieved genes jointly showing significant genetic association with SCD to select a high coverage of related genes. Furthermore, by paying attention to the biological correlation of genes, pathway and network analysis can resist the effect of false-positive gene results more effectively and may offer a more comprehensive glimpse into the pathological mechanisms of SCD.
The results of biological function enrichment analysis demonstrated that genes in the SCDgset participated in various biological processes and that the dysfunction of these genes might lead to heart diseases. For instance, terms that were directly related to cardiac functions, such as cardiac muscle contraction, heart development, regulation of heart rate, regulation of heart rate by cardiac conduction, and cardiac conduction, were highly enriched in SCD-related genes, implying these activities in the pathologic process of SCD are significant; furthermore, all of these terms were concurrent with the priori biological findings for SCD (4,18,39). Of further note, the GO biological processes that might lead to noncardiac causes of SCD, such as response to hypoxia, neurologic disorders, and terms related to cell membrane ion channels and transporters, were also enriched in the results. It was reported that central nervous system disorders can directly (or indirectly via cardiac interaction) result in SCD (41,55). Surprisingly, the gene numbers related to the terms like transcriptional regulation of RNA polymerase II promoter were in the top rank, while 31 genes functioned as positive regulators and 28 genes functioned as negative regulators. However, few previous reports have examined the relationship between transcriptional regulation of RNA polymerase II promoter and SCD, therefore, the current findings may give us new insights into the mechanisms and causes of SCD.
Pathway analysis showed that cardiac -related pathways were enriched in the SCDgset, and included HCM, DCM, adrenergic signaling in cardiomyocytes, ARVC, and cardiac muscle contraction (Table 1), which further highlights the dysfunctions of cardiac pathway as the key precipitators of SCD (35,37,38). Meanwhile, cGMP-PKG pathway was found to be enriched in the SCDgset at a high rank, which is consistent with its indispensable role in the pathogenesis and pathological development of SCD. The cGMP-PKG pathway plays a major role in the regulation of cardiac function, and is involved in heart failure, cardiac hypertrophy, and ischemic cardiomyopathy (56,57). The next highest-ranking pathway was calcium signaling pathway; interestingly, previous studies have demonstrated that crosstalk occurs between Ca2+ and cGMP signaling during cardiac hypertrophy (58). Calcium directly mediates cellular depolarization, diastolic current flow, early ischemic arrhythmias, and calcium homeostasis dysfunction to induce various cardiac diseases (59,60). MAPKs are prominent players that have been the focus of extensive investigations in the past decades. As MAPKs are essential to cardiac development, cardiac hypertrophy, ischemia /reperfusion injury, and pathological remodeling, they have been considered to be viable targets in therapeutic development (61). Our study showed that the MAPK pathway was one of the enriched pathways of SCD-related genes. However, within the pathway, the 4 best characterized MAPK subfamilies, ERK1/2, JNK, p38, and ERK5 were not included (Table 1), which might give clues that these genes participated SCD via noncanonical MAPK pathways. Consequently, these results indicated that the molecular mechanisms underlying SCD are fairly complex and require further study.
Much more interestingly, we identified two main modules via by pathway crosstalk analysis. One module was mostly composed of pathways related to calcium modulation and the other module was related to MAPK pathway. As previous studies have shown, calcium signaling and MAPK signaling are the furthest upstream nodes in heart development, and they are involved in induction of cardiac mesoderm, outflow tract formation, trabeculation, cardiac cushion formation, and valve formation. Furthermore, we observed that calcium signaling pathway and MAPK pathway were interconnected and shared most of the hormone-related pathways that were enriched in the SCDgset (i.e., apelin signaling pathway, oxytocin signaling pathway, and FoxO signaling pathway), suggesting that the two pathways share the same molecular mechanisms that regulate SCD. However, as it pertains to the MAPK module, it showed a greater degree and strength of crosstalk. The module emphasizes the notion that there might be a connection between virus infection pathway (e.g., tuberculosis, human cytomegalovirus infection, hepatitis B) and SCD via MAPK signaling pathway. Due to the limited number of relevant studies attesting to the close relationship between virus infection and SCD, our study may offer only preliminary evidence for the regulatory aspects of SCD, and this requires further examination. Hence, by combining the analytical results from biochemical pathway and pathway crosstalk with the a priori biological knowledge base, the major pathways associated with SCD can be identified.
Further, we inferred the SCD-specific protein network on the basis of the human reference interactome network. It is worth noting that 23 novel genes from the SCDgset that also appear in the human interaction group were ones credibly reported to be related to SCD. Interestingly, in the SCD-specific protein network, NOG has 71 edges that interact with the greatest number of proteins, and INS has 54 interactions, which is far more than that of other protein-protein interaction networks. By interacting with AGT and NOG, INS became the jumping-off point of two large clusters. Moreover, PPARA is a famous gene in studying the mechanisms of overweight or obese patients, and is potentially one of the causes of SCD (62). Even though we did not collect PPARA in the SCDgest, pathway crosstalk analysis identified it as a potential target gene in SCD. As for PAX4, it showed no definitive linkage to SCD. However, our results found it to be one of the important elements in the SCDgset because it interacted with 15 SCD-related proteins. SP1 and JUN mainly acted by means of MAPK pathway to contribute to the emergence of SCD (63,64).
We further extracted the specific protein network for SCD through two methods (Markov cluster algorithm and Steiner minimal tree algorithm) with different databases (STRING database and Pathway Commons), and the subnetworks also showed different patterns. In the first approach, the genes had different roles in SCD that could easily be identified; the genes labeled in red dots are known as regulators in heart contraction and ion transport, while the light green genes are modulators in muscle structure development (Figure 2). AGT was identified as the most central point in Figure 3, and it links to all other small clusters characterized by NOG, INS, PPARA, PSEN1, PAX4, SP1, ABCB1, and VEGFA. Surprisingly, even though AGT, PSEN1, ABCB1, and VEGFA were included in the SCDgset and were the jumping-off points to their corresponding clusters, they were just as normal as other factors in the subnetwork in Figure 2. However, other jumping-off points, such as NOG, INS, PAX4, PPARA, JUN, SP1 and PPKACA were not included in the SCDgset but do play important roles in the SCD-specific protein network, and reports do indicate that they are directly linked with SCD (46-48,62-64). Here, we strongly suggest that further effort should be put into their functional study. According to the results, our predictive approach could not only produce an important predictive subnetwork of the SCDgset for SCD but could potentially help detect related genes.
In current study, we compiled SCD-associated genes from selective literature deposited in the PubMed and GCBI databases and adopted a systems biology framework to conduct a comprehensive and systematic biological function- and network-based analysis of SCD. We focused only on those genes showing a positive association with SCD and that were proven with sufficiently solid evidence by original authors. Via integrating the analysis results from GO, pathway, and pathway crosstalk, we demonstrated that the basic cardiac-related pathways, including HCM, DCM, adrenergic signaling in cardiomyocytes, ARVC, and cardiac muscle contraction were enriched in the SCDgset. Furthermore, calcium signaling pathway and MAPK pathway were enriched in the SCDgset and were interrelated. Moreover, the SCD-specific pathological molecular network indicated some potential genes associated with SCD (NOG, INS, PAX4, PPARA, JUN, SP1, and PPKACA) which should be noted for further study. To confirm the association of these novel genes with any inherited cardiac disease, we will check the expression patterns in both mRNA and protein levels, build the overexpressing or/and knock-out mutants in cell and animal levels to perform functional study, and the molecular mechanisms will also be studied.
Therefore, our systematic and comprehensive exploration of the SCD-associated genes may not only offer broad insights into understanding the contribution of genetic factors and other related environmental factors in the pathogenesis of SCD but can also aid in exploring the molecular mechanisms underlying SCD. The SCDgset should provide an informative source and be considered a useful dataset for SCD inquiry. Finally, several potential biomarkers were identified that may be valuable for the prevention of SCD.
Acknowledgments
Funding: This study was supported by the Nantong Science and Technology Bureau (No. JC2018016, MS12019012, and MS12020056), the National Natural Science Foundation of China (No. 81701127), the Natural Science Foundation of Jiangsu Province (No. BK20170446), and the University of Nantong.
Footnote
Conflicts of Interest: All authors have completed the ICMJE uniform disclosure form (available at http://dx.doi.org/10.21037/jtd-21-361). The authors have no conflicts of interest to declare.
Ethical Statement: The authors are accountable for all aspects of the work in ensuring that questions related to the accuracy or integrity of any part of the work are appropriately investigated and resolved. The study was conducted in accordance with the Declaration of Helsinki (as revised in 2013).
Open Access Statement: This is an Open Access article distributed in accordance with the Creative Commons Attribution-NonCommercial-NoDerivs 4.0 International License (CC BY-NC-ND 4.0), which permits the non-commercial replication and distribution of the article with the strict proviso that no changes or edits are made and the original work is properly cited (including links to both the formal publication through the relevant DOI and the license). See: https://creativecommons.org/licenses/by-nc-nd/4.0/.
References
- World Health Organization. International classification of diseases (ICD-10). 10th Revision. 1992.
- Priori SG, Wilde AA, Horie M, et al. HRS/EHRA/APHRS expert consensus statement on the diagnosis and management of patients with inherited primary arrhythmia syndromes: document endorsed by HRS, EHRA, and APHRS in May 2013 and by ACCF, AHA, PACES, and AEPC in June 2013. Heart rhythm 2013;10:1932-63. [Crossref] [PubMed]
- Benjamin EJ, Blaha MJ, Chiuve SE, et al. Heart disease and stroke statistics-2017 update: a report from the American heart association. Circulation 2017;135:e146-603. [Crossref] [PubMed]
- Roberts WC. Sudden cardiac death: a diversity of causes with focus on atherosclerotic coronary artery disease. Am J Cardiol 1990;65:13B-19B. [Crossref] [PubMed]
- Zheng ZJ, Croft JB, Giles WH, et al. Sudden cardiac death in the United States, 1989 to 1998. Circulation 2001;104:2158-63. [Crossref] [PubMed]
- Puranik R, Chow CK, Duflou JA, et al. Sudden death in the young. Heart Rhythm 2005;2:1277-82. [Crossref] [PubMed]
- Papadakis M, Sharma S, Cox S, et al. The magnitude of sudden cardiac death in the young: a death certificate-based review in England and Wales. Europace 2009;11:1353-8. [Crossref] [PubMed]
- Wong LC, Behr ER. Sudden unexplained death in infants and children: the role of undiagnosed inherited cardiac conditions. Europace 2014;16:1706-13. [Crossref] [PubMed]
- Hua W, Zhang LF, Wu YF, et al. Incidence of sudden cardiac death in China: analysis of 4 regional populations. J Am Coll Cardiol 2009;54:1110-8. [Crossref] [PubMed]
- Brion M, Blanco-Verea A, Sobrino B, et al. Next generation sequencing challenges in the analysis of cardiac sudden death due to arrhythmogenic disorders. Electrophoresis 2014;35:3111-6. [Crossref] [PubMed]
- Niemeijer MN, van den Berg ME, Deckers JW, et al. ABCB1 gene variants, digoxin and risk of sudden cardiac death in a general population. Heart 2015;101:1973-9. [Crossref] [PubMed]
- Ran Y, Chen J, Li N, et al. Common RyR2 variants associate with ventricular arrhythmias and sudden cardiac death in chronic heart failure. Clin Sci (Lond) 2010;119:215-23. [Crossref] [PubMed]
- Westaway SK, Reinier K, Huertas-Vazquez A, et al. Common variants in CASQ2, GPD1L, and NOS1AP are significantly associated with risk of sudden death in patients with coronary artery disease. Circ Cardiovasc Genet 2011;4:397-402. [Crossref] [PubMed]
- Arking DE, Pfeufer A, Post W, et al. A common genetic variant in the NOS1 regulator NOS1AP modulates cardiac repolarization. Nat Genet 2006;38:644-51. [Crossref] [PubMed]
- Francia P, Adduci C, Ricotta A, et al. Common genetic variants in selected Ca2+ signaling genes and the risk of appropriate ICD interventions in patients with heart failure. J Interv Card Electrophysiol 2013;38:169-77. [Crossref] [PubMed]
- Liu X, Pei J, Hou C, et al. A common NOS1AP genetic polymorphism, rs12567209 G>A, is associated with sudden cardiac death in patients with chronic heart failure in the Chinese Han population. J Card Fail 2014;20:244-51. [Crossref] [PubMed]
- Liu Z, Liu X, Yu H, et al. Common variants in TRDN and CALM1 are associated with risk of sudden cardiac death in chronic heart failure patients in Chinese Han population. PloS One 2015;10:e0132459 [Crossref] [PubMed]
- Chopra N, Knollmann BC. Genetics of sudden cardiac death syndromes. Curr Opin Cardiol 2011;26:196-203. [Crossref] [PubMed]
- Liu W, Deng J, Ding W, et al. Decreased KCNE2 Expression Participates in the Development of Cardiac Hypertrophy by Regulation of Calcineurin-NFAT (Nuclear Factor of Activated T Cells) and Mitogen-Activated Protein Kinase Pathways. Circ Heart Fail 2017;10:e003960 Retraction in: Circ Heart Fail 2017 Nov;10(11):e000024. doi: 10.1161/HHF.0000000000000024. [Crossref] [PubMed]
- Huang DW, Sherman BT, Tan Q, et al. The DAVID gene functional classification tool: a novel biological module-centric algorithm to functionally analyze large gene lists. Genome Biol 2007;8:R183. [Crossref] [PubMed]
- Huang DW, Sherman BT, Tan Q, et al. DAVID bioinformatics resources: expanded annotation database and novel algorithms to better extract biology from large gene lists. Nucleic Acids Res 2007;35:W169-75 [Crossref] [PubMed]
- Khatri P, Sirota M, Butte AJ. Ten years of pathway analysis: current approaches and outstanding challenges. PLoS Comput Biol 2012;8:e1002375 [Crossref] [PubMed]
- Pavlidis P, Qin J, Arango V, et al. Using the gene ontology for microarray data mining: a comparison of methods and application to age effects in human prefrontal cortex. Neurochem Res 2004;29:1213-22. [Crossref] [PubMed]
- Jain S, Bader GD. An improved method for scoring protein-protein interactions using semantic similarity within the gene ontology. BMC Bioinformatics 2010;11:562. [Crossref] [PubMed]
- Shannon P, Markiel A, Ozier O, et al. Cytoscape: a software environment for integrated models of biomolecular interaction networks. Genome Res 2003;13:2498-504. [Crossref] [PubMed]
- Szklarczyk D, Gable AL, Lyon D, et al. STRING v11: protein-protein association networks with increased coverage, supporting functional discovery in genome-wide experimental datasets. Nucleic Acids Res 2019;47:D607-13. [Crossref] [PubMed]
- van Dongen S. Graph clustering by flow simulation. PhD thesis. The Netherlands: University of Utrecht, 2000.
- Barabási AL, Gulbahce N, Loscalzo J. Network medicine: a network-based approach to human disease. Nat Rev Genet 2011;12:56-68. [Crossref] [PubMed]
- Klein P, Ravi R. A nearly best-possible approximation algorithm for node-weighted Steiner trees. J Algorithms 1995;19:104-15. [Crossref]
- Cerami EG, Gross BE, Demir E, et al. Pathway Commons, a web resource for biological pathway data. Nucleic Acids Res 2011;39:D685-90. [Crossref] [PubMed]
- Hwang FK, Richards DS, Winter P. The Steiner Tree Problem. 1st edition. Elsevier Kruskal-Based Heuristic Section 414, 1992.
- Erdös P, Rényi A. On the evolution of random graphs. Publication of The Mathematical Institute of the Hungarian Academy of Sciences 1960;5:17-61.
- Liu C, Zhao Q, Su T, et al. Postmortem molecular analysis of KCNQ1, KCNH2, KCNE1 and KCNE2 genes in sudden unexplained nocturnal death syndrome in the Chinese Han population. Forensic Sci Int 2013;231:82-7. [Crossref] [PubMed]
- O'Mahony C, Jichi F, Pavlou M, et al. A novel clinical risk prediction model for sudden cardiac death in hypertrophic cardiomyopathy (HCM risk-SCD). Eur Heart J 2014;35:2010-20. [Crossref] [PubMed]
- Vriesendorp PA, Schinkel AF, Liebregts M, et al. Validation of the 2014 European Society of Cardiology guidelines risk prediction model for the primary prevention of sudden cardiac death in hypertrophic cardiomyopathy. Circ Arrhythm Electrophysiol 2015;8:829-35. [Crossref] [PubMed]
- Pahl E, Sleeper LA, Canter CE, et al. Incidence of and risk factors for sudden cardiac death in children with dilated cardiomyopathy: a report from the Pediatric Cardiomyopathy Registry. J Am Coll Cardiol 2012;59:607-15. [Crossref] [PubMed]
- O'Mahony C, Akhtar MM, Anastasiou Z, et al. Effectiveness of the 2014 European Society of Cardiology guideline on sudden cardiac death in hypertrophic cardiomyopathy: a systematic review and meta-analysis. Heart 2019;105:623-31. [PubMed]
- Romero J, Mejia-Lopez E, Manrique C, et al. Arrhythmogenic Right Ventricular Cardiomyopathy (ARVC/D): A systematic literature review. Clin Med Insights Cardiol 2013;7:97-114. [Crossref] [PubMed]
- Yeung CY, Lam KS, Li SW, et al. Sudden cardiac death after myocardial infarction in type 2 diabetic patients with no residual myocardial ischemia. Diabetes Care 2012;35:2564-9. [Crossref] [PubMed]
- Ackerman M, Atkins DL, Triedman JK. Sudden cardiac death in the young. Circulation 2016;133:1006-26. [Crossref] [PubMed]
- Jia P, Kao CF, Kuo PH, et al. A comprehensive network and pathway analysis of candidate genes in major depressive disorder. BMC Syst Biol 2011;5:S12. [Crossref] [PubMed]
- Dong C, Tang L, Liu Z, et al. Landscape of the relationship between type 2 diabetes and coronary heart disease through an integrated gene network analysis. Gene 2014;539:30-6. [Crossref] [PubMed]
- Valdivia CR, Ueda K, Ackerman MJ, et al. GPD1L links redox state to cardiac excitability by PKC-dependent phosphorylation of the sodium channel SCN5A. Am J Physiol Heart Circ Physiol 2009;297:H1446-52. [Crossref] [PubMed]
- England J, Granados-Riveron J, Polo-Parada L, et al. Tropomyosin 1: Multiple roles in the developing heart and in the formation of congenital heart defects. J Mol Cell Cardiol 2017;106:1-13. [Crossref] [PubMed]
- Jiang M, Zhang M, Howren M, et al. JPH-2 interacts with Cai-handling proteins and ion channels in dyads: Contribution to premature ventricular contraction-induced cardiomyopathy. Heart Rhythm 2016;13:743-52. [Crossref] [PubMed]
- Sadoshima J, Xu Y, Slayter HS, et al. Autocrine release of angiotensin II mediates stretch-induced hypertrophy of cardiac myocytes in vitro. Cell 1993;75:977-84. [Crossref] [PubMed]
- Fatini C, Abbate R, Pepe G, et al. Searching for a better assessment of the individual coronary risk profile. The role of angiotensin-converting enzyme, angiotensin II type 1 receptor and angiotensinogen gene polymorphisms. Eur Heart J 2000;21:633-8. [Crossref] [PubMed]
- Rodríguez-Pérez JC, Rodríguez-Esparragón F, Hernández-Perera O, et al. Association of angiotensinogen M235T and A(-6)G gene polymorphisms with coronary heart disease with independence of essential hypertension: the PROCAGENE study. Prospective Cardiac Gene. J AM Coll Cardiol 2001;37:1536-42. [Crossref] [PubMed]
- Yuasa S, Itabashi Y, Koshimizu U, et al. Transient inhibition of BMP signaling by Noggin induces cardiomyocyte differentiation of mouse embryonic stem cells. Nat Biotechnol 2005;23:607-11. [Crossref] [PubMed]
- Choi M, Stottmann RW, Yang YP, et al. The bone morphogenetic protein antagonist noggin regulates mammalian cardiac morphogenesis. Circ Res 2007;100:220-8. [Crossref] [PubMed]
- Klein S, Fontana L, Young VL, et al. Absence of an effect of liposuction on insulin action and risk factors for coronary heart disease. New Engl J Med 2004;350:2549-57. [Crossref] [PubMed]
- Gerstein HC, Bosch J, Dagenais GR, et al. Basal insulin and cardiovascular and other outcomes in dysglycemia. New Engl J Med 2012;367:319-28. [Crossref] [PubMed]
- Song XW, Yuan QN, Tang Y, et al. Conditionally targeted deletion of PSEN1 leads to diastolic heart dysfunction. J Cell Physiol 2018;233:1548-57. [Crossref] [PubMed]
- Marneros AG. Effects of chronically increased VEGF-A on the aging heart. FASEB J 2018;32:1550-65. [Crossref] [PubMed]
- Finsterer J, Wahbi K. CNS-disease affecting the heart: brain-heart disorders. J Neurol Sci 2014;345:8-14. [Crossref] [PubMed]
- Vila-Petroff MG, Younes A, Egan J, et al. Activation of distinct cAMP-dependent and cGMP-dependent pathways by nitric oxide in cardiac myocytes. Circ Res 1999;84:1020-31. [Crossref] [PubMed]
- Zhang M, Kass DA. Phosphodiesterases and cardiac cGMP: evolving roles and controversies. Trends Pharmacol Sci 2011;32:360-5. [Crossref] [PubMed]
- Miller CL, Oikawa M, Cai Y, et al. Role of Ca2+/calmodulin-stimulated cyclic nucleotide phosphodiesterase 1 in mediating cardiomyocyte hypertrophy. Circ Res 2009;105:956-64. [Crossref] [PubMed]
- McMurray JJ, Smith GL. Calcium handling in the failing heart and SUMO--weighing the evidence. New Engl J Med 2011;365:1738-9. [Crossref] [PubMed]
- Clusin WT, Buchbinder M, Harrison DC. Calcium overload, "injury" current, and early ischaemic cardiac arrhythmias--a direct connection. Lancet 1983;1:272-4. [Crossref] [PubMed]
- Rose BA, Force T, Wang Y. Mitogen-activated protein kinase signaling in the heart: angels versus demons in a heart-breaking tale. Physiol Rev 2010;90:1507-46. [Crossref] [PubMed]
- Bohula EA, Wiviott SD, McGuire DK, et al. Cardiovascular safety of Lorcaserin in overweight or obese patients. New Engl J Med 2018;379:1107-17. [Crossref] [PubMed]
- Du S, Li Z, Xie X, et al. IL-17 stimulates the expression of CCL2 in cardiac myocytes via Act1/TRAF6/p38MAPK-dependent AP-1 activation. Scand J Immunol 2020;91:e12840 [Crossref] [PubMed]
- Nguyên-Trân VT, Kubalak SW, Minamisawa S, et al. A novel genetic pathway for sudden cardiac death via defects in the transition between ventricular and conduction system cell lineages. Cell 2000;102:671-82. [Crossref] [PubMed]