A simple assessment of lung nodule location for reduction in unnecessary invasive procedures
Introduction
The National Lung Screening Trial (NLST) demonstrated that annual low dose computed tomography (LDCT) screening for lung cancer in high-risk individuals results in ~20% reduction in mortality. This reduction arises from the early detection of asymptomatic, but still treatable, early lung cancers. However, the majority of nodules detected by CT screening are not cancerous (1). In an effort to identify the few malignant nodules from among the many benign lesions, patients undergo unnecessary invasive procedures and surgical lung resections. The resulting morbidity and mortality generated by these potentially avoidable procedures is a significant public health burden.
To attempt to reduce morbidity associated with the high detection rate of benign lung lesions by LDCT screening, the American College of Radiology established the Lung-RADS system for nodule interpretation and management. The primary difference between Lung-RADS and the original NLST criteria is an increase in the diameter at which a nodule is considered to be “positive” (2). However, diameter alone is not entirely predictive of cancer and there is evidence that the false-positive rate remains high (3). To further assist clinicians, guidelines recommend the use of a probability calculator to determine the risk that an indeterminate nodule is cancer (4-9). However, performance of these models is variable and in fact, may not be better than a clinician’s judgement (10). There is thus an ongoing need for additional biomarkers to discriminate benign from malignant lung nodules.
The position of a lung cancer within the lung is not solely determined by stochastic probability. Multiple studies have demonstrated that lung cancers have a predilection for the upper lung regions (5,7,11-13). Particulate clearance, differences in blood vessel distribution, and concomitant inflammatory conditions such as emphysema and pulmonary fibrosis may all predispose a lung region to the development of cancer. However, lung nodule location has never been quantitatively assessed and applied for prediction. Additional spatial metrics of the nodule, such as proximal-distance from the central airways, also have not been rigorously assessed. Modern quantitative imaging techniques can evaluate both nodules and the surrounding lung (14-16) and may be applied to precisely determine the geospatial location of a nodule within the lung from a clinical CT scan. We thus sought to develop an entirely objective prediction model, based on a simple assessment of the geospatial location of a nodule, to improve discrimination of benign from malignant nodules.
We present the following article in accordance with the STARD reporting checklist (available at https://dx.doi.org/10.21037/jtd-20-3093).
Methods
Study oversight
The study was conducted in accordance with the Declaration of Helsinki (as revised in 2013). It was approved by the University of Vermont Institutional Review Board under a data use agreement through the Cancer Data Access System, to evaluate the National Lung Screening Trial data. The Detection of Early Lung Cancer Among Military Personnel (DECAMP) Consortium study was approved by the Human Research Protection Office of the Department of Defense and the individual site IRBs for every participating site. All subjects were approached for written informed consent to participate in the study in accordance with IRB regulations.
Study populations
We performed a secondary analysis of two prospectively collected cohorts: the NLST and DECAMP studies. Split set training and testing was performed in the NLST and the model deployed within DECAMP for replication. Details of the NLST have been published previously (NCT00047385) (1,17). The NLST defined an LDCT study as “positive” if a non-calcified nodule ≥4 mm in diameter was detected. All lung cancer endpoints were adjudicated. All single, solid, lung nodules between 4mm and 20mm were included.
The DECAMP study is comprised of 15 military, Veterans Affairs, and academic hospitals across the United States. The details of this study have been published previously (18). DECAMP-1 is a prospective study evaluating airway and blood-based molecular biomarkers for evaluation of an indeterminate nodule on CT (NCT01785342). This study enrolls patients aged 45 and older with an indeterminate pulmonary nodule 7–30 mm in diameter and at least a 20 pack-year smoking history. Patients are followed for up to two years to determine a benign or malignant diagnosis.
Quantitative CT analysis
All single lung nodules deemed “positive” by the original NLST criteria were included in the analysis. Following translation of CT image data, the location of the nodule was identified. Pure ground glass lesions were excluded from further analysis as these lesions have a distinct management algorithm (19,20). A reference fiducial point was placed on the main carina and defined as the origin for a Cartesian coordinate system. The coordinates for each indeterminate nodule were then determined and the distances in each perpendicular Cartesian axis were measured. Radial distance was defined as the Euclidian distance from the carina to the nodule. To account for differences in lung size between patients, the three Cartesian coordinates and the radial distance were normalized to the cube root of the lung volume.
Measurements of lung volumes was performed using the freely available Chest Imaging Platform (https://chestimagingplatform.org) using an automated segmentation of the lungs (21,22).
Statistical analysis
All statistical analyses were performed using STATA (Version 13, College Station, TX, USA). P values less than or equal to 0.05 were considered significant and all statistical tests were two-sided. We determined the appropriate number of NLST participants to include in the validation set (n=1,067) based on the sample size needed to achieve a 95% CI (±0.03).
Prediction models were constructed based on geospatial variables. Variables for inclusion in the model were evaluated using the receiver operating characteristic area-under-the curve (AUC), likelihood ratio test, and net reclassification index (23). Model calibration was tested via the Hosmer Lemeshow assessment and model specification assessed using the linktest. Sensitivity and specificity cutoffs were evaluated beginning from the inflection point of the receiver operating curve (24). Fagan’s nomogram was applied for calculation of posterior probability (25).
Results
Characteristics of the cohorts
Three thousand nine hundred twenty-four NLST CT scans were evaluated. One hundred forty-seven did not meet the original inclusion criteria or were incomplete/corrupt (Table S1). Seven percent of the remaining cases were not analyzable by the automated algorithms that performed lung or nodule segmentation. The final data set consisted of 3,483 participants. These were randomly divided into derivation and validation cohorts with proportions based on an a priori power calculation. Characteristics of the 2,444 participants in the derivation cohort and the 1,029 participants in the validation cohort are detailed in Table 1. In the DECAMP study there were 147 cases for which participants achieved a final adjudicated cancer status. All DECAMP CT scans completed automated analysis successfully. Characteristics listed in Table 1 were evaluated as predictors during model development.
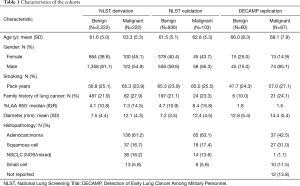
Full table
Model construction, validation, and replication
Similar to other cohorts, nodule diameter in the NLST was associated with a significant increase in risk of cancer among indeterminate nodules [OR 1.16 (1.13, 1.19), P<0.001] (7). The position of all indeterminate nodules is displayed in Figure 1. Cancer risk was not significantly associated with the lateral position (X axis) of an indeterminate nodule. Y (anterior-posterior) distance was a statistically significant predictor but had a very small effect [OR 1.00 (1.00, 1.01), P=0.008]. The Z distance (superior-inferior) was more strongly associated with a risk for malignancy [OR 1.02 (1.01, 1.02), P<0.001], implying a 2% increase in the risk of cancer per each additional millimeter above the carina in the superior-inferior axis (Figure 2). All other factors being equal, a nodule situated 10mm more superiorly in the lung would carry an additional 20% risk of malignancy. The radial distance from the carina to the nodule was also independently associated with a risk of malignancy [OR 0.96 (0.95, 0.97), P<0.001, Figure S1]. Radial distance and Z distance were therefore included with nodule diameter to form the Geospatial model. Inclusion of Y distance did not result in improvement in net reclassification (net reclassification improvement: P=0.11; AUC delta: z=−0.47, P=0.64) and therefore was not included in the final model.
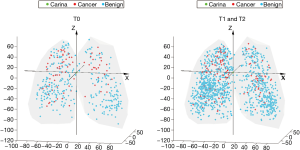
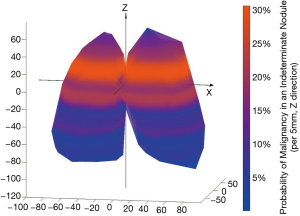
The final Geospatial model consisted of the radial distance from the carina to the cancer, the Z distance (the distance above or below the level of the carina), and the nodule diameter. In the derivation set, the Geospatial model, yielded an AUC of 0.83 and performed well in the strata of both prevalent (T0) and incident (T1/T2) nodules (Table S2). In the NLST validation, the Geospatial model produced an AUC of 0.85 (Table 2).

Full table
To assess performance in an external replication cohort, we tested the Geospatial model within the DECAMP cohort. The Geospatial model demonstrated an AUC of 0.75. Interestingly, the sensitivity and specificity curves, plotted against probability, were very similar between the two cohorts (Figure S2). This finding implies that a probability cut point to define sensitivity and specificity would be reproducible across diverse cohorts and thus may be clinically applicable.
Definition of the geospatial test
Based on the receiver operating characteristic curve, we defined a probability threshold of 0.03 for the Geospatial Model to create the Geospatial Test. A negative test thus implies a lower risk of cancer while a positive test implies a higher risk of cancer. In order to understand if the Geospatial test, as defined by this cutoff, performed well enough to be clinically useful we evaluated the performance of this test in strata of nodule diameters chosen to correspond with Lung-RADS criteria. The pre-test probability was determined by dividing the number of malignant nodules by the total number of indeterminate nodules, within each diameter category. The pre-test probability was then modified by the likelihood ratio (calculated from sensitivity and specificity) and Fagan’s nomogram used to define post-test probabilities of malignancy based on a positive or negative test. Among all size categories, a negative Geospatial Test resulted in a less than 2% post-test probability of malignancy (Table 3).
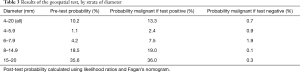
Full table
Applying the Geospatial Test resulted in correctly reclassifying 28.5% of benign nodules while incorrectly classifying a cancer as benign in only 1.8% of cases (Table 4).

Full table
Clinical utility of the geospatial test compared to the Brock model
We then sought to compare this performance to a modern standard for nodule assessment, the Brock model (7). Since emphysema and spiculation were not assessed in a standardized fashion we chose to use the parsimonious Brock model, which has been demonstrated to have similar performance in both the PanCan and NLST cohorts (26). In the NLST, the parsimonius Brock also outperformed both the Mayo and VA models. The parsimonious Brock model includes gender, nodule diameter, and location. The American College of Chest Physicians considers a low risk nodule to have a <5% risk of malignancy (4). We thus evaluated a 5% risk cutoff as determined by the Brock Model. In contrast to the Geospatial Test, the parsimonious Brock model resulted in correctly reclassifying 78% of nodules as benign, but with the untoward consequence of incorrectly classifying 21.5% of cancers as benign.
LungRADS is the modern standard for interpreting the results of lung cancer screening examinations. Thus, we also evaluated both the Geospatial and the parsimonious Brock models in combination with the LungRADS size definitions (no information about growth was available and thus only the size of the solid component was included). Limiting the analysis to nodules classified as LungRADS 3 or greater (≥6 mm), the Geospatial Test resulted in correctly reclassifying 19.7% of benign nodules while incorrectly classifying a cancer as benign in only 1.2% of cases (Table 5). In contrast, application of the Brock model resulted in correctly reclassifying 64.5% of benign nodules while incorrectly classifying a cancer as benign in 18.2% of cases. Limiting the analysis to LungRADS 4 or greater (diameter ≥8 mm), demonstrated that the Geospatial Test could still correctly reclassify many indeterminate nodules as benign (9.3%) with a low rate of misclassification for cancers (0.7%). The Brock applied to the same group of nodules resulted in correctly reclassifying 37% of nodules as benign but misclassifying 9.7% of the cancers as benign.

Full table
Among the 3,483 participants in the NLST data set, 318 underwent invasive procedures (percutaneous biopsy, bronchoscopy, mediastinoscopy, or surgery) for evaluation of cancers while 141 underwent invasive procedures for benign lesions, the latter group accounting for 29% of all invasive procedures. The average diameter of lesions for which an invasive procedure was performed was 12.6 mm (+/−6.6).
Applying the Geospatial test would result in reducing invasive procedures performed for benign lesions by 11.3% (16/141 cases) with a 1.3% (4/118 cases) rate of misclassification. In contrast, the Brock model applied to the same group of patients results in decreasing invasive procedures for benign lesion by 39.0% (55/141 cases) but misclassifying 21.1% of cancers (67/318) as benign.
Conclusions
In addition to the use of LungRADS, guidelines suggest that physicians estimate the probability of malignancy either based on their experience or with the use a nodule malignancy calculator (4). However, the models used to estimate the probability of nodular malignancy in these calculators have performed well in some settings but in others fare no better than a provider’s judgement (13,27). These models can also produce vastly different estimates of the probability of cancer. For example, a 9 mm spiculated nodule in the upper lobe of the lung in a 65-year-old man with a history of cigarette smoking would have either a 10% or a 37% risk of cancer depending on whether the Brock Model or Mayo Clinic Model were used. Perhaps more importantly, an intermediate probability result is difficult for both clinicians and patients to interpret.
These factors highlight the need to improve current nodule prediction models and motivated the design of the present study. In its treatise on biomarker development to improve lung cancer screening the American Thoracic Society identified distinct needs, one of which was “decreasing invasive procedures for patients with benign nodules without substantially delaying the diagnosis of cancer in patients with malignant nodules” (28). It is this goal which the Geospatial Test potentially addresses.
The Geospatial model quantitatively codifies the empiric relationship between lung nodule location and cancer risk noted in prior studies. For instance, the NELSON lung screening study reported a predilection for lung cancers to occur in the upper lobes and outer 1/3 of the lung (in axial section), although did not use any form of this information to discriminate benign vs. malignant nodules (29). In testing and replication sets, application of the Geospatial Test demonstrated a very high negative predictive value, implying a low risk of nodular malignancy. In contrast, a positive Geospatial test provides little additional diagnostic information. Practically speaking, demonstrating a reproducible probability cutoff across cohorts allowed us to create a test to identify lesions with a less than 2% risk of malignancy, potentially obviating the need for a complicated discussion of what is a “meaningful risk of cancer” between a patient and provider. However, it is important to note that the use of the Geospatial Test does not replace continuous probability calculators, particularly for those with a positive test, since it fails to provide further diagnostic information in this setting.
To assess the relative value of these tradeoffs, we also evaluated the potential effect of the Geospatial test on invasive procedures. This is the eventual, patient centered outcome. In the NLST data set, application of the Geospatial test would reclassify approximately 1 in every 4 indeterminate nodules as having a less than 2% risk of cancer. Even among LungRADS 3 or greater nodules, and similarly also in the group of individuals selected for invasive procedures, the Geospatial Test would reclassify 12–15% of indeterminate nodules into a low risk group. Perhaps more importantly, the rate of misclassification of cancers as benign lesions remains at ~1.0%. This was far superior to the Brock model which misclassified approximately 20% of cancers as benign. Both performance of the Geospatial Test and Brock Model were evaluated within the context of LungRAD. It is also important to note that most benign nodules for which an invasive procedure was performed were greater than 12mm, implying that application of LungRADS would not have prevented the majority of potentially avoidable procedures. Application of the Geospatial Test could alter management for approximately 1 in 4 of the nearly 2.25 million individuals estimated to have an indeterminate nodule detected by CT screening and result in significant health care cost savings (30).
There are several other aspects of our study that have important implications related to clinical applicability. We leveraged fully automated quantitative image analysis thereby removing subjective bias from the assessment. We also avoided the selection bias associated with case-control designs by including all available cases from both NLST and DECAMP. Indeed, the size of the NLST data set allowed us to evaluate model performance in both prevalent and incident nodules, and among strata of nodule diameter. In comparison to deep learning models, the anatomic CT measurements required to perform the Geospatial Test may be easily performed at nearly any existing radiology workstation or via free, open-source, HIPAA compliant software packages.
Nevertheless, our study has several important limitations. Surveillance CT scans were not collected as part of the NLST and thus nodule growth, a component of Lung-RADS, could not be assessed. Internal derivation and validation were performed in a CT screening cohort. External validation was assessed in the DECAMP cohort, which identified participants based on their need for an invasive evaluation of a nodule. Using disparate cohorts is a robust approach to validation, but limits our knowledge of the applicability of the Geospatial Test to cohorts of incidentally detected nodules. Furthermore, we chose to only include nodules up to 2 cm in diameter. The NLST data indicate that the risk of cancer is approximately 50% for indeterminate nodules between 2 and 3 cm. In most cases, this a priori probability necessitates invasive tissue sampling and not further risk assessment. We compared the Geospatial Test to the parsimonious Brock since radiographic emphysema was not uniformly captured in the NLST. However, the full Brock and the parsimonious Brock have similar performance characteristics (7). Finally, the test is not helpful for all individuals with a screen detected nodule since many individuals will have a positive Geospatial test, which provides little additional information about cancer risk. Individuals with a positive Geospatial Test may thus still benefit from application of a continuous probability calculator.
In summary, the data presented here highlight the importance of nodule location within the lung as a determinant of malignancy. The Geospatial Test performed well across multiple diverse cohorts and its application could potentially improve lung cancer screening performance for approximately 1 in 4 individuals with an indeterminate nodule.
Acknowledgments
Funding: NIH K23 HL133476 (CMK). The DECAMP study is supported by funds from the Department of Defense (W81XWH-11-2-0161), the National Cancer Institute (U01CA196408), and Johnson and Johnson Services, Inc. (JJSI).
Footnote
Reporting Checklist: The authors have completed the STARD reporting checklist. Available at https://dx.doi.org/10.21037/jtd-20-3093
Conflicts of Interest: All authors have completed the ICMJE uniform disclosure form (available at https://dx.doi.org/10.21037/jtd-20-3093). CMK reports grant funding from the NIH, consulting fees and clinical trial funding from Johnson and Johnson. CMK is a consultant and equity holder for Quantitative Imaging Solutions, and sits on the Board of Directors of the American Association of Bronchology and Interventional Pulmonology and the Prevention Committee of the Alliance for Oncology Clinical Trials. EB reports grant funding from the Department of Defense, Johnson and Johnson, and the National Cancer Institute. VM reports funding from the Damon Runyon Cancer Research Foundation and CAPES (Brazil). HM reports grant funding from Novartis, Janssen, and the Department of Defense. BT, AC, and ID, and Ruben SJ report consulting fees from Quantitative Imaging Services. CS reports Johnson and Johnson funding for DECAMP, and is employed by and owns stock in Johnson and Johnson. DA reports grant funding from American College of Radiology, Boston University, the NIH, and the Kaiser Foundation/PCORI, honoraria from the NIH, Department of Defense, International Symposium on Clinical Update in Respiratory Medicine, Cancer Research UC, American Lung Association, Japanese Society for CT screening, and grant support from the Early Detection Research Network, Molecular and Cellular Characterization of Screen-Detected Lesions Consortium, Department of Defense, International Lung Cancer Conference, Early Detection of Cancer Conference, and the Molecular and Cellular Characterization of Screen-Detected Lesions Consortium, support for meetings/travel from the American Institute for Medical and Biological Engineering, Cleveland Clinic, Specialized Programs of Research Excellence, and the International Association for the Study of Lung Cancer. JHB reports consulting fees from Johnson and Johnson. AS is an employee of Johnson and Johnson. GRW is an equity holder in Quantitative Imaging Solutions. RSJ reports grants from the NIH, a patent pending in lung cancer risk assessment, and is an equity holder of Quantitative Imaging Solutions. The authors have no other conflicts of interest to declare.
Ethical Statement: The authors are accountable for all aspects of the work in ensuring that questions related to the accuracy or integrity of any part of the work are appropriately investigated and resolved. The study was conducted in accordance with the Declaration of Helsinki (as revised in 2013). It was approved by the University of Vermont Institutional Review Board under a data use agreement through the Cancer Data Access System, to evaluate the National Lung Screening Trial data. The Detection of Early Lung Cancer Among Military Personnel (DECAMP) Consortium study was approved by the Human Research Protection Office of the Department of Defense and the individual site IRBs for every participating site. All subjects were approached for written informed consent to participate in the study in accordance with IRB regulations.
Open Access Statement: This is an Open Access article distributed in accordance with the Creative Commons Attribution-NonCommercial-NoDerivs 4.0 International License (CC BY-NC-ND 4.0), which permits the non-commercial replication and distribution of the article with the strict proviso that no changes or edits are made and the original work is properly cited (including links to both the formal publication through the relevant DOI and the license). See: https://creativecommons.org/licenses/by-nc-nd/4.0/.
References
- National Lung Screening Trial Research Team. Reduced lung-cancer mortality with low-dose computed tomographic screening. N Engl J Med 2011;365:395-409. [Crossref] [PubMed]
- Kazerooni EA, Armstrong MR, Amorosa JK, et al. ACR CT accreditation program and the lung cancer screening program designation. J Am Coll Radiol 2015;12:38-42. [Crossref] [PubMed]
- Pinsky PF, Gierada DS, Black W, et al. Performance of Lung-RADS in the National Lung Screening Trial: a retrospective assessment. Ann Intern Med 2015;162:485-491. [Crossref] [PubMed]
- Gould MK, Donington J, Lynch WR, et al. Evaluation of individuals with pulmonary nodules: when is it lung cancer? Diagnosis and management of lung cancer, 3rd ed: American College of Chest Physicians evidence-based clinical practice guidelines. Chest 2013;143:e93S-e120S.
- Swensen SJ, Silverstein MD, Ilstrup DM, et al. The probability of malignancy in solitary pulmonary nodules. Application to small radiologically indeterminate nodules. Arch Intern Med 1997;157:849-855. [Crossref] [PubMed]
- Gould MK, Ananth L, Barnett PG, et al. A clinical model to estimate the pretest probability of lung cancer in patients with solitary pulmonary nodules. Chest 2007;131:383-8. [Crossref] [PubMed]
- McWilliams A, Tammemagi MC, Mayo JR, et al. Probability of Cancer in Pulmonary Nodules Detected on First Screening CT. N Engl J Med 2013;369:910-9. [Crossref] [PubMed]
- Deppen SA, Blume JD, Aldrich MC, et al. Predicting lung cancer prior to surgical resection in patients with lung nodules. J Thorac Oncol 2014;9:1477-84. [Crossref] [PubMed]
- Li Y, Wang J. A mathematical model for predicting malignancy of solitary pulmonary nodules. World J Surg 2012;36:830-5. [Crossref] [PubMed]
- Hammer MM, Nachiappan AC, Barbosa EJM Jr. Limited Utility of Pulmonary Nodule Risk Calculators for Managing Large Nodules. Curr Probl Diagn Radiol 2018;47:23-7. [Crossref] [PubMed]
- Horeweg N, Aalst CM, van der , Thunnissen E, et al. Characteristics of lung cancers detected by computer tomography screening in the randomized NELSON trial. Am J Respir Crit Care Med 2013;187:848-54. [Crossref] [PubMed]
- Kinsey CM, Estepar RSJ, Zhao Y, et al. Invasive adenocarcinoma of the lung is associated with the upper lung regions. Lung Cancer 2014;84:145-50. [Crossref] [PubMed]
- Swensen SJ, Silverstein MD, Edell ES, et al. Solitary pulmonary nodules: clinical prediction model versus physicians. Mayo Clin Proc 1999;74:319-29. [Crossref] [PubMed]
- Washko GR, Hunninghake GM, Fernandez IE, et al. Lung volumes and emphysema in smokers with interstitial lung abnormalities. N Engl J Med 2011;364:897-906. [Crossref] [PubMed]
- Castaldi PJ, Estépar RSJ, Mendoza CS, et al. Distinct quantitative computed tomography emphysema patterns are associated with physiology and function in smokers. Am J Respir Crit Care Med 2013;188:1083-90. [Crossref] [PubMed]
- Aerts HJWL, Velazquez ER, Leijenaar RTH, et al. Decoding tumour phenotype by noninvasive imaging using a quantitative radiomics approach. Nat Commun 2014;5:4006. [Crossref] [PubMed]
- Aberle DR, DeMello S, Berg CD, et al. Results of the Two Incidence Screenings in the National Lung Screening Trial. N Engl J Med 2013;369:920-31. [Crossref] [PubMed]
- Billatos E, Duan F, Moses E, et al. Detection of early lung cancer among military personnel (DECAMP) consortium: study protocols. BMC Pulm Med 2019;19:59. [Crossref] [PubMed]
- Lee HY, Choi YL, Lee KS, et al. Pure Ground-Glass Opacity Neoplastic Lung Nodules: Histopathology, Imaging, and Management. AJR Am J Roentgenol 2014;202:W224-33 [Crossref] [PubMed]
- Yip R, Yankelevitz DF, Hu M, et al. Lung Cancer Deaths in the National Lung Screening Trial Attributed to Nonsolid Nodules. Radiology 2016;281:589-96. [Crossref] [PubMed]
- Yip SSF, Parmar C, Blezek D, et al. Application of the 3D slicer chest imaging platform segmentation algorithm for large lung nodule delineation. Ooijen PMA van, editor. PLoS One 2017;12:e0178944.
- Velazquez ER, Parmar C, Jermoumi M, et al. Volumetric CT-based segmentation of NSCLC using 3D-Slicer. Sci Rep 2013;3:3529. [Crossref] [PubMed]
- Leening MJG, Vedder MM, Witteman JCM, et al. Net reclassification improvement: computation, interpretation, and controversies: a literature review and clinician’s guide. Ann Intern Med 2014;160:122-31. [Crossref] [PubMed]
- Cancienne JM, Werner BC, Browne JA. Is There a Threshold Value of Hemoglobin A1c That Predicts Risk of Infection Following Primary Total Hip Arthroplasty? J Arthroplasty 2017;32:S236-240. [Crossref] [PubMed]
- Caraguel CGB, Vanderstichel R. The two-step Fagan’s nomogram: ad hoc interpretation of a diagnostic test result without calculation. Evid Based Med 2013;18:125-8. [Crossref] [PubMed]
- Nair VS, Sundaram V, Desai M, et al. Accuracy of Models to Identify Lung Nodule Cancer Risk in the National Lung Screening Trial. Am J Respir Crit Care Med 2018;197:1220-3. [Crossref] [PubMed]
- Folch EE, Bowling MR, Gildea TR, et al. Design of a prospective, multicenter, global, cohort study of electromagnetic navigation bronchoscopy. BMC Pulm Med 2016;16:60. [Crossref] [PubMed]
- Mazzone PJ, Sears CR, Arenberg DA, et al. Evaluating Molecular Biomarkers for the Early Detection of Lung Cancer: When Is a Biomarker Ready for Clinical Use? An Official American Thoracic Society Policy Statement. Am J Respir Crit Care Med 2017;196:e15-29. [Crossref] [PubMed]
- Horeweg N, van der Aalst C, Thunnissen E, et al. Characteristics of Lung Cancers Detected by Computer Tomography Screening in the Randomized NELSON Trial. Am J Resp Crit Care Med 2013;187:848-54. [Crossref] [PubMed]
- Cheung LC, Katki HA, Chaturvedi AK, et al. Preventing Lung Cancer Mortality by Computed Tomography Screening: The Effect of Risk-Based Versus U.S. Preventive Services Task Force Eligibility Criteria, 2005-2015. Ann Intern Med 2018;168:229-32. [Crossref] [PubMed]