Inflammatory parameters and pulmonary biomarkers in smokers with and without chronic obstructive pulmonary disease (COPD)
Introduction
Chronic obstructive pulmonary disease (COPD) is the fourth leading cause of death worldwide and its burden is increasing due to continued exposure to its risk factors and population aging (1-4). Even though the development of COPD is multifactorial, tobacco smoking remains its most important risk factor worldwide (4-9) to the extent that the term COPD is sometimes used as a synonym for smoking-induced lung disease (5).
Yet, for still unclear reasons not every smoker develops COPD, with percentages of smokers who develop clinically significant airway obstruction (AO) and COPD ranging from 15–30% (8) up to 50% and higher (6,7). Some researchers have suggested defining smokers with chronic respiratory symptoms without AO (6,7) as smoking-induced airway diseases (10,11) or even using a new concept of common smoke-related pulmonary disease (CSPD) with an initial stage without AO (12). Previous studies have investigated among other explanations the molecular mechanisms of augmented inflammation caused by smoking (13).
The potential roles of different biomarkers in association with COPD progression, exacerbation and mortality have already been studied, including cellular biomarkers [i.e., circulating white blood cells (WBCs)] and blood protein biomarkers [i.e., C-reactive protein (CRP), fibrinogen, interleukins, Clara cell secretory protein 16 (CC16), surfactant protein D (SP-D], and blood protease enzymes [i.e., matrix metallopeptidase 9 (MMP9)] (14-17). As COPD is a complex disorder no single biomarker can be used to assess it (16) but rather a model has been proposed using multiple classes of proteins such as acute phase proteins and signaling proteins playing a role in systemic inflammation, pneumoproteins involved in impaired lung homeostasis, and protease enzymes involved in oxidant injury (18).
Smoking itself may result in systemic inflammation, but the level of inflammation appears to be higher in patients with COPD (19). The inflammome is one suggested approach to distinguish the systemic inflammatory pattern in COPD patients from that in smokers with normal lung function (20). It has been previously shown in the ECLIPSE study that some biomarkers [WBCs, CRP, interleukin (IL)-6, and fibrinogen] were significantly higher in patients with COPD and increased with the degree of AO, whereas others [IL-8 and tumor necrosis factor-α (TNF-α)] were higher in smokers without COPD suggesting these could be primary markers of smoking but not of COPD (21). Additionally, COPD patients with persistent systemic inflammation in the ECLIPSE study had higher mortality and exacerbation rates than those without inflammation (21). The roles of the predominantly lung-derived biomarkers (so-called “pneumoproteins”) such as SP-D (a protein secreted mainly by type II pneumocytes and Clara cells) and CC16 (also called club cell protein) in smokers with and without COPD have also been investigated (14,19,22,23). Another suggested model of tobacco smoking-induced immune response is a network where proinflammatory and anti-inflammatory regulatory pathways interlink with each other through molecular mediators (24). However, many biomarkers in these models are not specific to COPD and smoking, and require validation in appropriately designed studies prior to routine use in clinical practice. In summary, the current data on hypothetical pathophysiological pathways to explain why some smokers develop COPD while others do not, are fragmented and incomplete thereby and further research is needed in this area.
The aim of our study is to investigate differences in serum inflammatory profiles and circulating serum pneumoproteins between smokers with and without confirmed COPD in the RESPECT (“The RESearch on the PrEvalence and diagnosis of COPD and its Tobacco-related etiology”) study. This study of adults 35–70 years old in two northwestern cities in Russia has investigated the prevalence of AO, identified risk factors and assessed the diagnostic value of respiratory symptoms for AO as well as followed-up with a comprehensive diagnostic assessment by a pulmonologist the participants with AO at baseline to identify those with a clinical diagnosis of COPD (24-27).
Methods
Study design and population
RESPECT is a population-based study that has been conducted as a collaboration between the Université Catholique de Louvain (Belgium), North-Western State Medical University (named for I.I. Mechnikov, St. Petersburg, Russia) and Northern State Medical University, Arkhangelsk (Russia). The study aimed to improve understanding of the epidemiology of COPD in northwestern Russia. We published the design of the RESPECT elsewhere (25). In total, we invited 15 primary care centers in two northwestern Russian cities (St. Petersburg and Arkhangelsk) to participate in the RESPECT study, and recruited fifteen investigators (10 from St. Petersburg and 5 from Arkhangelsk). The participating sites recruited a population-based random sample of at least 200 non-institutionalized adults, 35–70 years old, and living in a well-defined administrative area. Questionnaires were administered by research investigators regarding the participants’ background characteristics, including sociodemographic data, smoking status, occupational exposures, and respiratory symptoms. We invited all participants to undergo a spirometry test. Baseline characteristics of the total RESPECT population were published elsewhere (26).
The comparative study invited patients aged 35–70 years with a clinical diagnosis of COPD after a comprehensive assessment and a smoking history of more than 10 pack-years(cases, n=38) and 38 participants with the same smoking history without AO (controls) to participate. The inclusion criteria for cases was as follows: (I) fixed AO defined as a post-bronchodilator (post-BD) fixed ratio of 0.7 [a ratio of the forced expiratory volume in 1 second to the forced vital capacity (FEV1/FVC) <0.7] and/or a FEV1/FVC ratio below the fifth percentile of a large healthy reference group [the statistically defined lower limit of normal (LLN) using the Global Lung Initiative (GLI) 2012 equations (28),FEV1/FVC < GLI-LLN] and (II) a COPD diagnosis based on the Global Initiative for Chronic Obstructive Lung Disease (GOLD) (1) criteria and confirmed by a pulmonologist after a comprehensive clinical examination. The inclusion criteria for the controls were as follows: (I) a post-BD FEV1/FVC >0.7 and FEV1/FVC > GLI-LLN; (II) no history of asthma (absence of symptoms); and (III) no usage of bronchodilators. Cases and controls were matched for age and smoking history.
To obtain “reference values” for biomarkers, a group of healthy non-smokers (n=35) aged 35–70 years from the studied population and the RESPECT’ investigators was composed (Table S1). The healthy non-smokers, smokers without AO and the participants with COPD were matched for age. The inclusion criteria for the group of non-smokers were: (I) a post-BD FEV1/FVC >0.7 and FEV1/FVC > GLI-LLN; (II) no history of asthma (absence of symptoms and no usage of bronchodilators); and (III) no smoking history; (IV) no other chronic diseases. Exclusion criteria were: the presence of a respiratory disorder other than COPD (for cases) and the presence any other significant inflammatory disease (for cases and controls)
Enrollment was between June 8, 2012, and December 17, 2013 for the baseline study, and May 16, 2013, and May 13, 2015 we examined those who participated in the comparison study.
The study was conducted in accordance with the Declaration of Helsinki (as revised in 2013). Local medical ethics review boards (North-West State Medical University, named for I.I. Mechnikov, protocol N 11 from 07/12/2011, and Northern State Medical University, protocol N 01/1-12 from 11/01/2012) approved the study protocol. All participants signed a written informed consent form.
Background characteristics and other variables
Background characteristics included the sex, age, and socioeconomic status. Smoking status was categorized as “never smoked”, “ex-smoker” (persons who had quit smoking ≥6 months prior), or “current smoker”. Former and current smokers reported the age at which they began to smoke, how many years they had been smoking and how many cigarettes they smoked per day (in pack years). One pack-yearof smoking indicated that an individual smoked one pack of cigarettes (20 cigarettes) daily for 1 year. We used the American Thoracic Society (ATS) 1978 Adult Questionnaire (ATS-DLD-78) to assess exposure to occupational hazards (29). Participants reported if they had exposure to any of the following for 1 year or more: dusty job, a job with exposure to gas or chemical fumes or a job involving the use of protective equipment. Information was systematically collected regarding any personal or family history of obstructive airway disease (asthma, chronic bronchitis, emphysema, and chronic cough), allergic diseases, or tuberculosis and the presence of co-morbidities [cardiovascular diseases (CVDs) and others]. At enrollment we assessed if persons had a history of hospitalization, treatment [including BD, inhaled corticosteroid (ICS), statins and others] or an exacerbation frequency of obstructive disease.
The comprehensive standardized assessment protocol used by the pulmonologist included a physical examination with lung and heart auscultation, measurement of the height, weight, body mass index (BMI), waist circumference, pulse, respiratory rate, and blood pressure, skin and edema assessments, and a new spirometry with bronchodilator test. Patients were asked about the presence of chronic respiratory symptoms, including chronic cough, sputum production (defined as lasting longer than 3 months), and dyspnea (30).
Spirometry
Spirometry required the use of a portable turbine Micro Spirometer (MIR Spirobank, Rome, Italy) and a personal computer equipped with a program to facilitate the uploading of data to a central database. In a previous paper we reported the accuracy of the spirometry measurements by trained investigators (31). All investigators could participate in a three-week distance-learning course on spirometry with a daylong face-to-face training session (SpiroCourse) (32). All investigators completed the course successfully and agreed to receive continuous quality feedback on the tests performed. Winspiro Pro software (MIR) compared the measured values with those in reference tables and automatically calculated the reproducibility of the performed spirometry in accordance with the European Respiratory Society (ERS) guidelines. During both the baseline assessment and follow-up study, post-BD spirometry used 400 µg of salbutamol or 160 µg of ipratropium bromide (for patients older than 60 years of age or with comorbid CVD). We used ATS/ERS quality criteria to assess the acceptability and repeatability of results (33). Spirogram evaluations were conducted by two independent experts and classified into 4 categories (ATS1: all ATS/ERS criteria, including reproducibility, were fulfilled; ATS2: all criteria except for the duration of expiration >6 seconds were fulfilled; ATS3: the test was “usable” for the interpretation of the peak expiratory flow and FEV1, and the spirograms displayed good starts and no coughs during the 1st second of the maneuver; and ATS4: none of the ATS/ERS criteria were fulfilled, and the spirograms were not “usable”). For inclusion in the study, we considered spirograms classified as ATS1 or ATS2 were considered to be of acceptable quality. We calculated predictive values of the spirometry parameters based on the GLI 2012 reference values using the GLI 2012 Data Conversion software (34). AO was defined using FEV1/FVC <0.7 (fixed cut-off) and/or FEV1/FVC < GLI-LLN as the cut-off values.
Laboratory testing
Blood samples were collected from each patient after fasting (between 7:00 AM and 10:30 AM) and immediately stored in a refrigerated container prior to delivery to the central laboratory (<3 h after blood collection). Plasma [ethylenediaminetetraacetic acid (EDTA) or heparin] or serum samples were obtained after centrifugation. The samples were separated into aliquots (n=4–6 depending on the volume obtained) and immediately stored frozen at −80 °C for subsequent analysis at the central lab in St. Petersburg. The analytical process was organized to avoid several freeze-thaw cycles.
A hemogram, including red blood cell (RBC), WBC and leukocyte counts, was immediately performed at 1 of 2 laboratories (St. Petersburg and Arkhangelsk).
The following laboratory tests were performed for all cases and controls: blood counts (WBCs and RBCs), hemoglobin, cholesterol, triglycerides, creatinine, glucose, aspartate transaminase (AST), alanine transaminase (ALT), 25-hydroxyvitamin D, α1-antitripsin and NTpro-brain natriuretic peptide (NTproBNP).
Several biomarkers [including high-sensitivity CRP (hs-CRP), IL-4, IL-6, IL-8, IL-10, TNF-α, CC16, SP-D, MMP9, and tissue inhibitor of metalloproteinases (TIMP)] were quantified in smokers with COPD, smokers without AO and healthy non-smokers. A rationale for the various selected biomarkers is provided in Table S2.
A large panel of biomarkers was analyzed using the enzyme-linked immunosorbent assay (ELISA) “sandwich” method in the Alisei Q.S. SEAC analyzer (Italy). All measurements were performed in the laboratory of the North-West Federal Medical University (named for I.I. Mechnikov, St. Petersburg, Russia).
The list of the biomarkers analyzed together with their sensitivities is reported in Table 1. To obtain “reference values” for all of the abovementioned biomarkers, blood samples from 35 healthy non-smokers with normal spirometry and without any obstructive lung disease were analyzed with the same kits and in the same laboratory (Table 2).
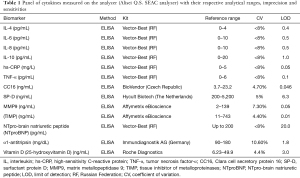
Full table
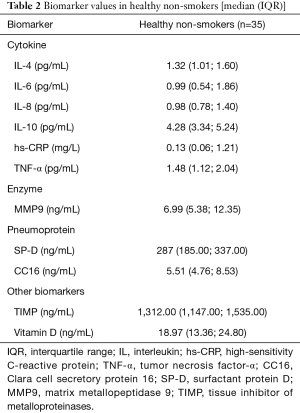
Full table
Statistical analysis
Descriptive statistics were calculated for all variables. Continuous variables are presented as the mean ± standard deviation (SD), and categorical variables are presented as numbers with frequencies. Laboratory data were presented as the median, interquartile range (IQR) and logarithmic scale values. The baseline variables were compared between the two groups with a one-sample t-test, and Pearson’s χ2 test was performed for the categorical variables. Inflammatory parameters and pulmonary biomarkers were compared between the two groups consecutively (smokers with COPD and without AO) with the non-parametric Mann-Whitney U test. The percentage of smokers with COPD and without AO that exceeded the 90th percentile of the values of the healthy non-smokers was presented in radar plots.
To determine the prevalence of elevated cytokines, pulmonary pneumoproteins and other biomarkers, values exceeding the 90th percentile in the healthy non-smokers were considered abnormal. A model for the cigarette smoking-induced immune response for selected biomarkers was presented as a network layout, with each node corresponding to one of the six biomarkers, and its size was proportional to the prevalence of abnormal values (the 90th percentile of the healthy nonsmokers) for that particular biomarker in that particular group of subjects. Two nodes are linked if more than 1% of subjects in the network share abnormal values of these two biomarkers, its width being proportional to that proportion.
Subsequently all participants (cases and controls) were divided into the following groups: smokers with systemic inflammation (i.e., with 2 or more elevated biomarker levels in the upper quartile, including participants with COPD and without AO n=19) and smokers without systemic inflammation (i.e., with no elevated biomarkers, also including participants with COPD and without AO n=36). To verify the pattern of systemic inflammation, smokers with 3 or more elevated biomarker levels in the upper quartile (n=11) were additionally compared to smokers with no elevated biomarkers (n=36).
Smokers with a high cytokines profile (defined as in upper quartile for at least 2 (or 3) biomarkers) were considered as “inflamed” smokers. Smokers with 2+ (or 3+) elevated biomarker levels (“inflamed”) and smokers with no elevated biomarkers (non-inflamed) were compared between the two groups with the one-sample t-test for baseline variables and Pearson’s χ2 test for categorical variables. Logistic regression was used to investigate factors contributing to systemic inflammation and high pneumoprotein levels in the whole group of smokers.
A group of heavy smokers (those who smoked more than 40 pack/years including participants with COPD and without AO) was identified.
Statistical significance was set at P<0.05 (a two-tailed probability value). SPSS version 20.0 (SPSS Inc., Chicago, IL, USA) was used for the statistical analyses.
Results
Demographic and clinical data
Of the 2,388 individuals who performed a spirometry test with satisfactory quality in the baseline part of the RESPECT study, 278 demonstrated post-BD AO based on the fixed or GLI-LLN cut-offs, and 2,110 did not (Figure 1).Current or ex-smokers with a ≥10 pack/years smoking history (95 with AO and 106 without AO) were included in the diagnostic study with follow-up spirometry and examined by the pulmonologist. Based on the comprehensive standardized assessment protocol, 38 individuals with AO were diagnosed with COPD (cases), and 38 individuals without AO were diagnosed with no objective evidence of obstructive lung disease (controls) (Figure 1). Those 38 cases and 38 controls (altogether 76 individuals) were included in the comparative study. The mean interval between the baseline and the second spirometry was 14.5±4.8 months (range from 4 to 27 months).
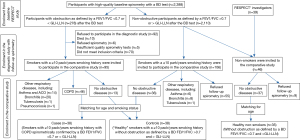
The mean age of the smoker participants was 55.9 years, and 71.1% were male. The mean smoking history was 33.2 pack/years. The baseline characteristics of the smokers with COPD and without AO based on the clinical and spirometric criteria are presented in Table 3. No significant differences were found between the cases and controls in their educational levels, socioeconomic statuses and indoor exposure (wood/coal stove use more than 7 times/week) (Table 3). Smokers without AO had a higher FEV1/FVC ratio and had less CVDs than the patients with COPD.
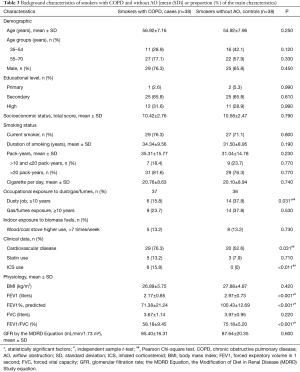
Full table
Cross-sectional analysis of systemic inflammation
Figure 2 shows a box plot of the inflammatory biomarkers (log scale) measured during the follow-up spirometry in the two groups of subjects studied, and Table 4 shows median (IQR) values of all biomarkers studied. Despite variability within each group (Figure 2) and relatively small absolute differences between groups (Table 4), on average the WBC counts, CRP and TNF-α levels were significantly higher in the patients with COPD than in the smokers with normal lung function (Figure 2, Table 4). The median α1-antitripsin level was also significantly higher in the case group. No difference in MMP9 and TIMP levels between two groups was found.
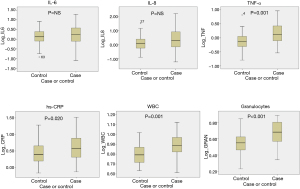
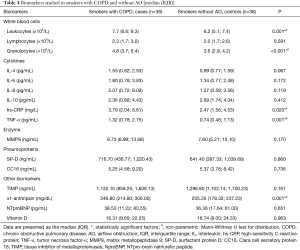
Full table
Figure 3 presents the percentages of the smokers with COPD and without AO in both groups who exceeded the 90th percentiles of the values of the healthy non-smokers for cytokines (Figure 3A), pulmonary pneumoproteins and other biomarkers (Figure 3B). These percentages were significantly higher for IL-4 and IL-8 in the case group. No difference in any pneumoproteins was found between the cases and controls. The highest percentages of persons in both groups that exceeded the 90th percentiles of the values of the healthy non-smokers were observed for IL-8 (and IL-4 for cases) and SP-D among the cytokines and pneumoproteins, respectively. A significant difference in MMP9 levels was observed between non-smokers and smokers with COPD.
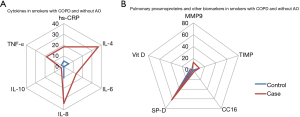
Figure 4 presents a network model for the cigarette smoking-induced immune response for selected biomarkers in the two groups of participants. Each node of the network corresponds to 1 of the 6 cytokines, and its size is proportional to the prevalence of abnormal values (the 90th percentile of the healthy nonsmokers) for that particular biomarker in that particular group of subjects. Two nodes are linked if more than 1% of subjects in the network share abnormal values of these two biomarkers, its width being proportional to that proportion. In the smokers with COPD, the network is substantially developed (more and thicker lines), with all nodes increasing in size compared with those of the smokers with normal lung function and healthy non-smokers (Figure 4). The inflammatory (such as IL-6, IL-8, TNF-α) and anti-inflammatory (such as IL-4, IL-10) pathways are interlinked to each other through molecular mediators (such as IL-6 and IL-10).
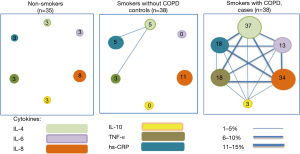
Smoking and systemic inflammation
Participants (cases and controls) were subsequently divided into two groups: smokers with systemic inflammation (with 2 or more elevated biomarker levels in the upper quartile, n=19) and smokers without systemic inflammation (with no elevated biomarkers, n=36). To verify the pattern of systemic inflammation, smokers with 3 or more elevated inflammatory biomarker levels in the upper quartile (n=11) were compared to smokers with no elevated biomarkers (n=36).
Table 5 compares the baseline demographics, clinical and functional characteristics and risk factors (smoking and occupational and environmental exposures) of the inflamed [among IL-6, IL-8, hs-CRP and TNF-α; (I) with 2+ elevated biomarker levels and (II) with 3+ elevated biomarker levels] and non-inflamed smokers.
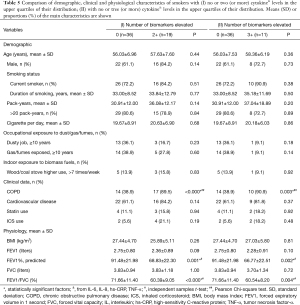
Full table
Out of all smokers, 57.9% with normal spirometry and 36.8% with COPD did not demonstrate any elevated biomarker levels (“non-inflamed” smokers). Systemic inflammation (“inflamed” smokers) based on 2+ elevated biomarker levels was identified in 44.7% of the patients with COPD and 5.3% of the patients without AO, whereas systemic inflammation based on 3+ elevated levels was identified in 26.3% of the patients with AO in 2.6% of the patients with normal spirometry.
No difference in age, gender, and smoking history, environmental and occupational exposure was found between the non-inflamed and the inflamed smokers (with either 2+ or 3+ elevated biomarkers levels). The prevalence of CVD and the proportions who used ICS and statins were similar in both groups. The FEV1/FVC ratio was lower in the patients with systemic inflammation.
Table 6 shows a summary of the logistic regression analysis of the high inflammatory profile [defined as the upper quartile for at least 2 (I) or 3 (II) biomarkers among IL-6, IL-8, TNF-α and CRP]. Gender, history of CVD, and use of ICS or statin were not associated with the presence of systemic inflammation. After adjustment for age, gender, smoking exposure (current smokers), only spirometrically confirmed and clinically proven COPD was associated with systemic inflammation [for 2+ biomarkers odds ratio (OR) 11.42, 95% confidence interval (CI): 2.13–58.84; for 3+ biomarkers OR 15.64, 95% CI: 1.65–148.26].
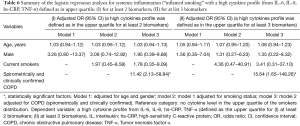
Full table
No association between lung function expressed as FEV1/FVC and high pneumoprotein (SP-D or CC16) levels (defined as a value in the upper quartile) was found. After adjustment for age, sex and renal function, a high CC16 level (defined as a value in the upper quartile) was not associated with COPD (OR 1.33, 95% CI: 0.40–4.36).
Additionally, a comparison of the demographic, clinical and physiological characteristics of heavy smokers (>40 pack-years) and other smokers is presented in Table S3. Heavy smokers (those who smoked more than 40 pack/years)were mostly male, but no difference was found in their occupational or environmental exposures compared to those who smoked less than 40 pack/years. Heavy smokers had similar cardiovascular comorbidities, statin and ICS usage compared to those who smoked less. Moreover, heavy smokers had a similar percentage of persons with COPD and “inflamed” smokers [with ≥2 (or 3) elevated cytokine levels] than those who smoke less. It is worth being mentioned that 40.9% of heavy smokers did not any show elevated biomarker levels. Concerning single biomarkers, hs-CRP, IL-6, and SP-D levels were significantly higher in heavy smokers than in those who smoked less (Table S3); α1-antitripsin levels were also significantly higher in the group of heavy smokers.
Discussion
Main findings
In a sample of adults in Northwestern Russia we found that smokers with COPD have a different systemic inflammatory network pattern than smokers with normal lung function, but no differences in pneumoproteins levels. Additionally, more than one-third of smokers with COPD in our study had no abnormal biomarkers, while a subgroup of smokers (of which most had COPD) had elevated inflammatory biomarker levels (“inflamed” smokers) and worse lung function impairment.
Differences in inflammatory profiles between smokers with and without COPD
In our study, the WBCs, hs-CRP and TNF-α levels were significantly higher for the smokers with COPD than smokers without AO (Figure 3, Table 4), similar to what was observed in the ECLIPSE study (25). We found no significant difference in MMP9 levels between smokers with COPD and those without AO, but there were significantly higher levels of MMP9 in smokers with COPD compared to healthy non-smokers. Compared to smokers without AO, the network of systemic inflammation (with anti-inflammatory/regulatory pathways) in smokers with COPD was substantially more developed (Figure 4).
The “inflammome” is a complex network of multiple cell types and mediators that has been used to identify difference between systemic inflammatory biomarkers in smokers with and without COPD (21). Several studies have supported the idea of a “persistent systemic inflammation” COPD phenotype (35-38). Elevated levels of different circulating biomarkers such as CRP and fibrinogen have been reported in patients with tobacco- and/or biomass smoke-induced COPD (37,38).
In our study, no significant difference was found between smokers with and without AO in regard to indoor exposure (wood/coal stove use more than 7 times/week), but there were more participants with occupational hazards (dust or gas/chemical fume exposure for more than 10 years) among smokers without AO (Table 3). Yet for the total RESPECT population, AO was significantly associated with tobacco exposure, but not with occupational or indoor pollution (26). Smokers with COPD in our study reported more CVDs than smokers without AO despite similar statin use histories, NTproBNP levels and BMIs (Table 3). As in the ECLIPSE study, we observed that smokers with COPD (cases) used ICS significantly more often than those without AO but compared to the ECLIPSE study patients with COPD in our RESPECT study used ICS less frequently (15.8% vs. 71% in the ECLIPSE study). This is probably due to the different degrees of disease severity of the participants in the two studies.
Similar to the ECLIPSE study (25), we found no differences in occupational and environmental exposure between the “inflamed” smokers (with 2+ elevated biomarker levels) and the “non-inflamed” smokers. In contrast to the ECLIPSE study, we also found no difference in age, sex and smoking history between “inflamed” and “non-inflamed” smokers. Cardiovascular comorbidities, statin and ICS usage were also similar between these two groups, but the “inflamed” smokers had worse lung function (characterized by AO) (Table 5). Compared to the ECLIPSE study, we observed that “inflamed” smokers had more cardiovascular comorbidities, which corresponds to a high prevalence of and mortality due to CVDs in Russia (39,40). In our analysis, spirometry-based and clinically proven COPD was associated with higher risk for chronic inflammation, whereas age, sex and current smoking were not (Table 6, I).Meanwhile, in the ECLIPSE study age, BMI, current smoking, and airflow limitation were associated with persistent inflammation in smokers with COPD (21). This difference can be explained by the fact that the ECLIPSE study investigated systemic inflammation in patients with COPD, while in the RESPECT study we evaluated systemic inflammation in smokers (including those with COPD and those without AO). Even though both studies concern smokers with a smoking history of more than 10 pack-years, the focus of the ECLIPSE study was on the “inflamed” and “non-inflamed” patients with COPD, while in our study we compared smokers with COPD and those without AO, as well as “inflamed” and “non-inflamed” smokers (both including participants with COPD and those without AO). Additionally, we also compared heavy smokers (those who smoked more than 40 pack-years including participants with COPD and without AO) with those who smoked less than 40 pack-years.
Similar to the ECLIPSE study, we found that approximately one-third of patients with COPD did not have any abnormal biomarkers (21). In addition, we found that heavy smokers had similar COPD and “inflammation” profiles (with at least 2 elevated cytokine levels) with those who smoked less, and more than of one-third of heavy smokers did not demonstrate any elevated inflammatory biomarker levels (“non-inflamed” heavy smokers). Concerning single biomarkers, hs-CRP, IL-6 and SP-D levels, as well as α1-antitripsin level, were significantly higher in heavy smokers (Table S3).
Recently, a new concept of “individual natural histories of COPD” has been proposed in which COPD is no longer defined as a single disease but as a syndrome (41,42). Based on all the above we can hypothesize that each smoker and by extension each patient with COPD, has his/her own natural history of a smoking-induced disease. Thereby, a smoker with normal spirometry may become a COPD patient, and vice versa, mild COPD patients may become non-obstructive. We have already reported findings of this diagnostic instability (reversal of COPD diagnosis) in the RESPECT study (27).
Differences in pneumoprotein levels between smokers with and without COPD
The second aim of our study was to explore the levels of circulating serum pneumoproteins in smokers with COPD and those without AO. We found no difference for levels of any pneumoprotein among smokers with COPD and those without AO. In both groups, SP-D had the highest percentage of participants that exceed the 90th percentile of the healthy non-smokers values among the pneumoproteins. In the ECLIPSE study, higher serum SP-D levels were reported among COPD patients than among current and ex-smokers without AO and the largest difference in the serum SP-D levels was observed between non-smokers and current/former smokers leading to the conclusion that SP-D is a powerful biomarker for smoking (23,43). In our study, serum SP-D levels were significantly higher for smokers with normal spirometry (without AO) and smokers with COPD compared to non-smokers. Higher serum SP-D levels were demonstrated among heavy smokers than in those who smoked less, despite a similar number of participants with COPD.
The clinical relevance and utility of circulating SP-D and CC16 as diagnostic, prognostic, responsive biomarkers of COPD (either single biomarker or in combination with inflammatory biomarkers) has already been discussed (17,18,43). However, whether SP-D and CC16 levels can differentiate COPD from smoking or other respiratory diseases is uncertain.
The data regarding the association between circulating SP-D and lung function are contradictory. Circulating SP-D has been proposed to define smokers with low initial lung function who are most at risk for developing COPD, and therefore SP-D measurements can be used complimentary to spirometry for the early detection of COPD (44). SP-D is a useful COPD marker within specified prognostic subgroups and in multi-marker analyses (43). Although the SP-D levels increase strongly in response to smoking, high circulating SP-D levels appear to be related to disease outcomes even after adjustment for smoking (43). In our study, no association between circulating SP-D and lung function was found in smokers with a smoking history of more than 10 pack/years. This finding can be at least partially explained by the small number of observations.
Polymorphisms in CC16 (a protein secreted by non-ciliary bronchiolar cells) and local deficiency as well as a diminished number of club cells (which can be identified as a “smoking gun” candidate) have been marked as COPD features (44-48). The significance of lowered serum CC16 levels in patients with COPD remains unclear. In the ECLIPSE studies it was found that median serum levels of CC-16 were significantly lower in individuals with COPD than in smokers with normal lung function, and that CC-16 levels were also lower in smoking controls than in non-smokers and that otherwise serum CC16 levels did not correlate with lung function or reversible AO in current smokers with COPD (22). However, a low vitamin D level was related to emphysema, the 6MWD, airway reactivity, and the CC16 level (49). The authors concluded that CC16 was a useful biomarker for COPD but could not be applied to screen populations or as a substitute for lung function testing to identify reversible AO, and when used should be corrected for age, sex, smoking status and lung function (22).
In our study, we found no significant difference in CC16 and vitamin D levels between smokers with COPD and smokers without AO and high CC16 levels were not associated either with FEV1/FVC or with COPD.
Multiple biomarkers (combination of inflammatory biomarkers and pneumoproteins)
Recently the role of combinations of multiple biomarkers has been reported (14,16,49). The ECLIPSE and COPDGene studies both indicated that combinations of multiple biomarkers were much more strongly predictive of AO [CC16, soluble receptor for advanced glycation end products (sRAGE), fibrinogen, CRP and SP-D], emphysema (SP-D, CRP, sRAGE and fibrinogen), and mortality (SP-D, CRP and fibrinogen) than any single biomarker, although the amount of variance explained by the multiple biomarkers was lower than that of clinical variables (44,49). We did not investigate these combinations of multiple biomarkers in our study and this may be an area of future research.
Strengths and limitations
Our study has several strengths and limitations. The RESPECT study is a prospective population-based study of adults aged 35–70 years. A large number of persons were screened based on symptoms, risk factors and pre- and post-BD spirometry. Patients at risk for COPD were reassessed using a standardized diagnostic work-up protocol. The final diagnosis of COPD was based on a comprehensive assessment, which included a detailed history, rigorous clinical examination by experienced pulmonologists and repeated spirometry with a BD test. A major strength of the study is that both cases and controls come from the same original population (the case-control study was nested in the cohort study). Both cases and controls were matched for age and smoking history, and healthy non-smokers were matched for age as well.
Our study has some potential limitations. The biology of the inflammatory response is complex, and we studied only a limited panel of biomarkers. Nevertheless, the biomarkers chosen correspond to those investigated by the majority of previous studies, and some of them are often and easily measured in routine clinical practice.
Higher tobacco consumption has been shown to be associated with higher systemic inflammation both genetically and observationally (50). In our study, we reported cross-sectional data for inflammatory and pulmonary biomarkers, but no longitudinal follow-up observations or genotype studies of high systemic inflammation or tobacco consumption.
Conclusions
In a sample of adults in Northwestern Russia, we found in smokers with COPD a systemic inflammatory network pattern that is different from that of smokers with normal lung function. We also observed that systemic inflammation is not present in all smokers with COPD, while some smokers without AO have systemic inflammation. From this perspective, smoking itself could be seen as a disease and studied accordingly. Additionally, we identified a subgroup of smokers (of which most have COPD) with elevated inflammatory biomarker levels (“inflamed” smokers) and worse lung function impairment who could be a target for specific therapeutic interventions beside smoking cessation.
COPD includes several different clinical and pathophysiologic phenotypes. In the future biomarkers that predict responsiveness to particular treatments might guide specific targeted therapeutic interventions. More studies are needed to clarify if early anti-inflammatory therapies aiming prevention of further decline of lung function are effective and safe in some specific subgroups of COPD patients and non-obstructed smokers with systemic inflammation.
Acknowledgments
The authors thank all investigators from St. Petersburg and Arkhangelsk who made this study possible, Yulia Zhilinkova and Yekaterina Bul’ina who performed all of the laboratory testing.
Funding: The RESPECT study was supported by an unconditional grant from AstraZeneca International to the Fondation Louvain, the support unit of the Université Catholique de Loiuvain, which is charged with developing the educational and research projects of the university by collecting gifts from corporations, foundations and alumni. Laboratory testing was supported by an unconditional grant from AstraZeneca Russia.
Footnote
Data Sharing Statement: Available at https://dx.doi.org/10.21037/jtd-20-1580
Conflicts of Interest: All authors have completed the ICMJE uniform disclosure form (available at https://dx.doi.org/10.21037/jtd-20-1580). The authors have no conflicts of interest to declare.
Ethical Statement: The authors are accountable for all aspects of the work in ensuring that questions related to the accuracy or integrity of any part of the work are appropriately investigated and resolved. The study was conducted in accordance with the Declaration of Helsinki (as revised in 2013). Local medical ethics review boards (North-West State Medical University, named for I.I. Mechnikov, protocol N 11 from 07/12/2011, and Northern State Medical University, protocol N 01/1-12 from 11/01/2012) approved the study protocol. All participants signed a written informed consent form. The clinical trial registration is: NCT02307799.
Open Access Statement: This is an Open Access article distributed in accordance with the Creative Commons Attribution-NonCommercial-NoDerivs 4.0 International License (CC BY-NC-ND 4.0), which permits the non-commercial replication and distribution of the article with the strict proviso that no changes or edits are made and the original work is properly cited (including links to both the formal publication through the relevant DOI and the license). See: https://creativecommons.org/licenses/by-nc-nd/4.0/.
References
- Global Initiative for Chronic Obstructive Lung Disease. Global strategy for the diagnosis, management and prevention of chronic obstructive pulmonary disease; 2018. Available online: http://goldcopd.org
- . GBD 2016 Causes of Death Collaborators. Global, regional, and national age-sex specific mortality for 264 causes of death, 1980-2016: a systematic analysis for the Global Burden of Disease Study 2016. Lancet 2017;390:1151-210. [Crossref] [PubMed]
- World Health Organization. Global burden of disease website; 2017. Available online: http://www.who.int/gho/mortality_burden_disease/causes_death/top_10/en/
- Thun MJ, Carter BD, Feskanich D, et al. 50-year trends in smoking-related mortality in the United States. N Engl J Med 2013;368:351-64. [Crossref] [PubMed]
- Salvi S. Tobacco smoking and environmental risk factors for chronic obstructive pulmonary disease. Clin Chest Med 2014;35:17-27. [Crossref] [PubMed]
- Montes de Oca M, Zabert G, Moreno D, Laucho-Contreras ME, et al. Smoke, Biomass Exposure, and COPD Risk in the Primary Care Setting: The PUMA Study. Respir Care 2017;62:1058-66. [Crossref] [PubMed]
- Lundbäck B, Lindberg A, Lindström M, et al. Not 15 but 50% of smokers develop COPD?--Report from the Obstructive Lung Disease in Northern Sweden Studies. Respir Med 2003;97:115-22. [Crossref] [PubMed]
- Bartal M. COPD and tobacco smoke. Monaldi Arch Chest Dis 2005;63:213-25. [Crossref] [PubMed]
- ERS White Book 2015. European lung white book. Available online: http://www.erswhitebook.org/
- Fabbri LM. Smoking, Not COPD, as the Disease. N Engl J Med 2016;374:1885-6. [Crossref] [PubMed]
- Thomson NC. Asthma and smoking-induced airway disease without spirometric COPD. Eur Respir J 2017;49:1602061 [Crossref] [PubMed]
- Baddini-Martinez J, de Pádua AI. Chronic obstructive pulmonary disease: time to discuss new concepts. Lancet 2016;388:2740-1. [Crossref] [PubMed]
- Barnes PJ. Inflammatory mechanisms in patients with chronic obstructive pulmonary disease. J Allergy Clin Immunol 2016;138:16-27. [Crossref] [PubMed]
- Faner R, Tal-Singer R, Riley JH, et al. Lessons from ECLIPSE: a review of COPD biomarkers. Thorax 2014;69:666-72. [Crossref] [PubMed]
- Pinto-Plata V, Toso J, Lee K, et al. Profiling serum biomarkers in patients with COPD: associations with clinical parameters. Thorax 2007;62:595-601. [Crossref] [PubMed]
- Cazzola M, Puxeddu E, Ora J, et al. Evolving Concepts in Chronic Obstructive Pulmonary Disease Blood-Based Biomarkers. Mol Diagn Ther 2019;23:603-14. [Crossref] [PubMed]
- Stockley RA, Halpin DMG, Celli BR, et al. Chronic Obstructive Pulmonary Disease Biomarkers and Their Interpretation. Am J Respir Crit Care Med 2019;199:1195-204. [Crossref] [PubMed]
- Moon JY, Leitao Filho FS, Shahangian K, et al. Blood and sputum protein biomarkers for chronic obstructive pulmonary disease (COPD). Expert Rev Proteomics 2018;15:923-35. [Crossref] [PubMed]
- Barnes PJ. Cellular and molecular mechanisms of chronic obstructive pulmonary disease. Clin Chest Med 2014;35:71-86. [Crossref] [PubMed]
- American Association of Immunologists. The definition of the inflammome. An AAI recommendation for the NIH “Roadmap for Medical Research” FY 2011. Am Assoc Immunol Newsletter 2008;7:7-8.
- Agustí A, Edwards LD, Rennard SI, et al. Persistent systemic inflammation is associated with poor clinical outcomes in COPD: a novel phenotype. PLoS One 2012;7:e37483 [Crossref] [PubMed]
- Lomas DA, Silverman EK, Edwards LD, et al. Evaluation of serum CC-16 as a biomarker for COPD in the ECLIPSE cohort. Thorax 2008;63:1058-63. [Crossref] [PubMed]
- Lomas DA, Silverman EK, Edwards LD, et al. Serum surfactant protein D is steroid sensitive and associated with exacerbations of COPD. Eur Respir J 2009;34:95-102. [Crossref] [PubMed]
- Pan Z, Yu H, Liao JL. Probing Cellular and Molecular Mechanisms of Cigarette Smoke-Induced Immune Response in the Progression of Chronic Obstructive Pulmonary Disease Using Multiscale Network Modeling. PLoS One 2016;11:e0163192 [Crossref] [PubMed]
- Andreeva E, Pokhaznikova M, Lebedev A, et al. The RESPECT study: RESearch on the PrEvalence and the diagnosis of COPD and its Tobacco-related etiology: a study protocol. BMC Public Health 2015;15:831. [Crossref] [PubMed]
- Andreeva E, Pokhaznikova M, Lebedev A, et al. The Prevalence of Chronic Obstructive Pulmonary Disease by the Global Lung Initiative Equations in North-Western Russia. Respiration 2016;91:43-55. [Crossref] [PubMed]
- Andreeva E, Pokhaznikova M, Lebedev A, et al. Spirometry is not enough to diagnose COPD in epidemiological studies: a follow-up study. NPJ Prim Care Respir Med 2017;27:62. [Crossref] [PubMed]
- Quanjer PH, Stanojevic S, Cole TJ, et al. Multi-ethnic reference values for spirometry for the 3-95-yr age range: the global lung function 2012 equations. Eur Respir J 2012;40:1324-43. [Crossref] [PubMed]
- Ferris BG. Epidemiology Standardization Project (American Thoracic Society). Am Rev Respir Dis 1978;118:1-120. [PubMed]
- Bridevaux PO, Probst-Hensch NM, Schindler C, et al. Prevalence of airflow obstruction in smokers and never-smokers in Switzerland. Eur Respir J 2010;36:1259-69. [Crossref] [PubMed]
- Degryse J, Buffels J, Van Dijck Y, et al. Accuracy of office spirometry performed by trained primary-care physicians using the MIR Spirobank hand-held spirometer. Respiration 2012;83:543-52. [Crossref] [PubMed]
- Pokhaznikova MA, Kuznetsova OY, Andreeva EA, et al. Experience of development of distance learning course of spirometry in training of general practitioners. Russian Family Doctor 2012;16:39-44. [Crossref]
- Miller MR, Crapo R, Hankinson J, et al. General considerations for lung function testing. Eur Respir J 2005;26:153-61. [Crossref] [PubMed]
- Global Lung Function Initiative. GLI-2012 desktop software for individual calculations. Available online: http://www.ers-education.org/guidelines/global-lung-function-initiative/tools/gli-2012-desktop-software-for-individual-calculations.aspx
- Singh S, Verma SK, Kumar S, et al. Correlation of severity of chronic obstructive pulmonary disease with potential biomarkers. Immunol Lett 2018;196:1-10. [Crossref] [PubMed]
- Celli BR, Locantore N, Yates J, et al. Inflammatory biomarkers improve clinical prediction of mortality in chronic obstructive pulmonary disease. Am J Respir Crit Care Med 2012;185:1065-72. [Crossref] [PubMed]
- Golpe R, Martín-Robles I, Sanjuán-López P, et al. Differences in systemic inflammation between cigarette and biomass smoke-induced COPD. Int J Chron Obstruct Pulmon Dis 2017;12:2639-46. [Crossref] [PubMed]
- Olloquequi J, Jaime S, Parra V, et al. Comparative analysis of COPD associated with tobacco smoking, biomass smoke exposure or both. Respir Res 2018;19:13. [Crossref] [PubMed]
- Shalnova SA, Konradi AO, Karpov YA, et al. Cardiovascular mortality in 12 Russian Federation regions– participants of the “Cardiovascular Disease Epidemiology in Russian Regions” study. Russ J Cardiol 2012;97:6-11.
- Sidorenkov O, Nilssen O, Nieboer E, et al. Premature cardiovascular mortality and alcohol consumption before death in Arkhangelsk, Russia: an analysis of a consecutive series of forensic autopsies. Int J Epidemiol 2011;40:1519-29. [Crossref] [PubMed]
- Agustí A, Celli B. Natural history of COPD: gaps and opportunities. ERJ Open Res 2017;3:00117-2017. [Crossref] [PubMed]
- Celli BR, Agustí A. COPD: time to improve its taxonomy? ERJ Open Res 2018;4:00132-2017. [Crossref] [PubMed]
- Sorensen GL. Surfactant Protein D in Respiratory and Non-Respiratory Diseases. Front Med (Lausanne) 2018;5:18. [Crossref] [PubMed]
- Johansson SL, Tan Q, Holst R, et al. Surfactant protein D is a candidate biomarker for subclinical tobacco smoke-induced lung damage. Am J Physiol Lung Cell Mol Physiol 2014;306:L887-95. [Crossref] [PubMed]
- Knabe L, Fort A, Chanez P, et al. Club cells and CC16: another "smoking gun"? (With potential bullets against COPD). Eur Respir J 2015;45:1519-20. [Crossref] [PubMed]
- Kim DK, Cho MH, Hersh CP, et al. Genome-wide association analysis of blood biomarkers in chronic obstructive pulmonary disease. Am J Respir Crit Care Med 2012;186:1238-47. [Crossref] [PubMed]
- Bourdin A, Kotsimbos T, Nguyen K, et al. Non-invasive assessment of small airway remodelling in smokers. COPD 2010;7:102-10. [Crossref] [PubMed]
- Laucho-Contreras ME, Polverino F, Gupta K, et al. Protective role for club cell secretory protein-16 (CC16) in the development of COPD. Eur Respir J 2015;45:1544-56. [Crossref] [PubMed]
- Zemans RL, Jacobson S, Keene J, et al. Multiple biomarkers predict disease severity, progression and mortality in COPD. Respir Res 2017;18:117. [Crossref] [PubMed]
- Çolak Y, Afzal S, Lange P, et al. Smoking, Systemic Inflammation, and Airflow Limitation: A Mendelian Randomization Analysis of 98 085 Individuals From the General Population. Nicotine Tob Res 2019;21:1036-44. [Crossref] [PubMed]