A systematic review and meta-analysis of diagnostic performance and physicians’ perceptions of artificial intelligence (AI)-assisted CT diagnostic technology for the classification of pulmonary nodules
Introduction
Lung cancer was the second most commonly diagnosed cancer and the leading cause of cancer death in 2020, representing approximately one in 10 (11.4%) cancers diagnosed and one in 5 (18.0%) cancer deaths and accounting for an estimated 2.2 million new cancer cases and 1.8 million deaths (1). The five-year survival rate for lung cancer ranges from 70% for stage I to less than 5% for stage IV (2). A number of surgical and medical therapies can cure many cases of small localized tumors, but only 15% of patients with lung cancer are diagnosed at the localized stage (3). In addition, lung cancer treatment is costly, with approximately $9.6 billion being spent on this treatment in the United States each year (4).
Low-dose computed tomography (CT) has been widely used for lung cancer screening (5). It now offers the ability to detect small lesions (less than 10 mm in diameter) and has the potential to detect stage I tumors 4 to 6 times as frequently as conventional radiography (6). Many current guidelines for lung cancer screening recommend annual low-dose chest CT screening for high-risk individuals, for whom the benefit of low-dose chest CT screening outweighs its harms (7-9), and recommend lung cancer diagnosis is based on the size and attenuation characteristics of the nodule as well as the presence of lung cancer risk factors for small nodules and on the estimated probability of malignancy and the yield of additional testing for larger nodules (10,11).
However, with CT being used increasingly more often in healthy people and patients with suspected lung diseases, radiologists face a greatly increased workload regarding the assessment of pulmonary nodules [defined as a focal opacity of <3 cm in diameter (12)] in CT images. Moreover, one study showed that the sensitivity of radiologists in accurately identifying and classifying pulmonary nodules (as benign or malignant nodules) ranged from 30% to 97%, and the false positive rate was as high as 2.1 per scan (13). The differentiation of benign and malignant nodules is a challenging task and requires a combination of visual assessments and measurements. Different physicians may also have different interpretations.
Artificial intelligence (AI) holds great promise and has been used in clinical practice in recent years (14,15). One example is AI-assisted diagnostic technologies, which use computerized extraction and classification algorithms to identify and classify diseases. In general, AI-assisted diagnostic technology has four functions: preprocessing images (cleaning images and removing noise); segmenting the region of interest (ROI); extracting and selecting the most discriminative features; and classifying the disease according to the features (16). Machine learning algorithms (MLAs), such as artificial neural networks (ANNs) (17-20), support vector machines (SVMs) (21-24), deep belief networks (DBNs) (25-27), Bayesian networks (BNs) (28-30), convolutional neural networks (CNNs) (31-33), and decision trees (DTs) (34-37), are used in AI-assisted CT diagnostic technologies to detect and classify pulmonary nodules as benign or malignant.
AI-assisted diagnostic technology is being developed, explored and evaluated to support clinical diagnosis and treatment. It is used as a “second opinion” to assist in the clinical diagnostic process, and it may improve the quality and consistency of diagnoses; improve the accuracy of determining cancer susceptibility, recurrence and survival prediction; reduce the time required for diagnosis; prevent physical and psychological factors from influencing the diagnoses; and perhaps reduce hospital costs (38,39). Although AI-assisted diagnostic technologies have shown promise, no products related to AI-assisted CT diagnostic technologies for the classification of pulmonary nodules as benign or malignant have been approved by the National Medical Products Administration (NMPA) in China (40). Currently, few systematic reviews of the diagnostic performance of AI-assisted CT diagnostic technology for the classification of pulmonary nodules as benign or malignant have been conducted, no systematic review has been performed to compare the diagnostic performance of this technology with different MLAs, and the benefits and risks of this technology perceived by physicians remain unclear.
This study aimed to systematically review the diagnostic performance of AI-assisted CT diagnostic technology in the classification of pulmonary nodules as benign or malignant and to analyze physicians’ perceptions of its potential benefits and risks as well as their attitude toward its clinical application in China.
We present the following article in accordance with the PRISMA reporting checklist (available at https://dx.doi.org/10.21037/jtd-21-810).
Methods
Literature search and eligible studies
To conduct a systematic review, 6 electronic literature databases [PubMed, EMBASE (Ovid SP), the Cochrane Central Register of Controlled Trials (the Cochrane Library), the China National Knowledge Infrastructure (CNKI), the Wanfang Data Knowledge Service Platform (WANFANG data), and the China Biomedicine Database (Sinomed)] were searched for eligible studies from 2010 to 2019. The MeSH terms or keywords used for literature retrieval were as follows: (pulmonary nodule OR lung nodule OR pulmonary neoplasm OR pulmonary cancer OR lung cancer OR lung tumor OR lung neoplasm) AND (artificial intelligence OR AI OR computer-aided diagnosis OR computer-assisted diagnosis OR CAD OR deep learning OR neural network OR machine learning OR support vector machine OR decision tree) AND (computed tomography OR CT) AND (classification OR classifier OR classify OR diagnosis). The search strategy was designed according to different database searching standards by two reviewers. Additionally, a manual search of the literature was conducted to further identify eligible studies.
After the literature search, each study related to the diagnostic performance of AI-assisted CT diagnostic technology for the classification of pulmonary nodules as benign or malignant was screened to determine whether it met the inclusion and exclusion criteria. The inclusion criteria were as follows: (I) the purpose of the study was to assess the diagnostic performance of AI-assisted CT diagnostic technology in the classification of pulmonary nodules as benign or malignant; (II) at least one machine learning algorithm was used as a classifier; (III) a CT imaging modality was used; (IV) sufficient data were provided in or could be calculated from the articles, including the number of true positives (TPs), false positives (FPs), true negatives (TNs) and false negatives (FNs); (V) the full text of the article was available; and (VI) the article was published from 2010 to 2019. The exclusion criteria were as follows: (I) case reports, conference abstracts, reviews, posters, or other nonoriginal articles; (II) duplicate publications or studies with overlapping sample data; and (III) articles not written in Chinese or English.
Data extraction
The quality of the included studies was assessed using the second version of the quality assessment of diagnostic accuracy studies (QUADAS-2) scale (41). The quality score of each study was determined on the basis of 14 items from four domains (description, signaling questions, risk of bias and concern about applicability). The specific data extracted from each study were the first author’s name, year of publication, first author’s country, data source, gold standard, machine learning algorithm used, number of nodules, and data used to evaluate diagnostic performance (TP, FP, TN and FN). Two reviewers carefully rated the quality of all the included studies and extracted the data from these studies independently in accordance with the study protocol. Disagreements were resolved by discussion between the two reviewers.
Moreover, to develop AI-assisted CT diagnostic technology for the classification of pulmonary nodules as benign or malignant, data related to the texture features extracted from CT images are usually divided into a training dataset and a testing dataset. The training dataset is used to develop a model for pulmonary nodule classification, while the testing dataset is used to validate the model created using the training dataset. In our systematic review, the results obtained from testing datasets were used for the meta-analysis and meta-regression. Some studies did not specify the training dataset and testing dataset, and in this case, all samples were extracted (5 studies). If a study included more than one testing dataset to test the classifier, all results from the testing datasets were recorded and used for the analyses (3 studies). If a study evaluated the classification accuracy using the chi-square test at different confidence levels, only the result at 95% confidence level was recorded and used for the analyses (1 study on Bayes classifier). If a study used many MLTs to classify pulmonary nodules, all of them were mentioned and recorded in the data tables (6 studies).
Questionnaire survey
A cross-sectional questionnaire survey of physicians from 9 public tertiary hospitals in Shanghai, Hubei Province and Gansu Province in China was conducted from September to December 2019. These regions were selected to capture various socioeconomic statuses (high, middle and low) and geographic distributions (eastern, central and western) within China. Within each region, 3 public tertiary hospitals (1 general hospital and 2 specialty hospitals) were selected for the questionnaire survey.
Within each selected hospital, all physicians in the clinical departments and imaging departments related to the diagnosis and treatment of respiratory diseases and/or lung cancer (such as the departments of respiratory disease, thoracic surgery, oncology, and medical imaging) were invited to participate in the questionnaire survey. The survey of hospital physicians was conducted by anonymous, paper-based, self-administered questionnaires. The questionnaires were distributed and collected by hospital managers who were trained and served as coordinators of the study. In the training for the survey, the importance and aims of the study were provided.
The questionnaire included two sections: one section concerned the physician perceptions of AI-assisted CT diagnostic technology for the classification of pulmonary nodules as benign or malignant (such as its benefits and risks and their personal attitude toward its clinical application), and the other section concerned general information on the physicians (age, sex, educational attainment, department in which they worked, whether they were physicians or physician managers, and their practical experience with the technology) (Appendix 1). The physicians’ personal attitudes toward the clinical application of AI-assisted CT diagnostic technology for the classification of pulmonary nodules as benign or malignant were rated using a 5-point Likert scale (5= strongly supported, 4= somewhat supported, 3= neutral, 2= somewhat unsupported, 1= strongly unsupported). The percentage of physicians who gave scores equal to or greater than 4 regarding their attitude toward the clinical application of the technology was referred to as the support rate.
Data analysis
We analyzed the study characteristics, quality of the included studies, and characteristics of the surveyed physicians. The pooled sensitivity, specificity, positive likelihood ratio (PLR), negative likelihood ratio (NLR), diagnostic odds ratio (DOR), summary receiver operator characteristic (SROC) curve, and area under the SROC curve (AUC) with 95% confidence intervals (CIs) were calculated to assess the diagnostic performance of AI-assisted CT diagnostic technology for the classification of pulmonary nodules as benign or malignant. AUC values of 0.90–1.00 indicate excellent detection, 0.80-0.90 indicate good detection, 0.70–0.80 indicate fair detection, 0.60–0.70 indicate poor detection and 0.50–0.60 indicate failure (42).
Statistical methods
The Spearman correlation coefficients of the logarithm sensitivity and 1-specificity were calculated to detect the threshold effect (43). The Cochran-Q test and I2 were used to assess the no-threshold effect. If there was no significant heterogeneity caused by the no-threshold effect (P<0.05 and I2≤50%), a fixed-effects model was adopted; otherwise, a random-effects model was used (44, 45). In addition, Deek’s asymmetry test was used to assess publication bias.
A multilevel linear regression model (method = REML, weight = 1/variance of odds) was used for the meta-regression to explore the effects of the machine algorithms on the pooled DOR while controlling for the study random effects and other fixed effects (number of nodules and the first author’s country). Using the above multilevel linear regression model, the adjusted pooled DORs of AI-assisted CT diagnostic technologies for the classification of pulmonary nodules as benign or malignant were calculated and graphed, with the number of nodules and the first author’s country at the means.
In addition, chi-square tests were used to compare the perceptions of different groups of physicians toward AI-assisted CT diagnostic technologies for the classification of pulmonary nodules as benign or malignant. A univariate logistic model was used to analyze the association of physicians’ perceptions of the benefits and risks of AI-assisted CT diagnostic technology for the classification of pulmonary nodules as benign or malignant with their rate of support for its clinical application.
The data were analyzed by using Review Manager 5.3 (RevMan 5.3), Meta-Disc 1.4, Stata 12.0, and SAS 9.4. All P values were two-sided, and P<0.05 indicated statistical significance.
The study was conducted in accordance with the Declaration of Helsinki (as revised in 2013). The study was approved by the Institutional Review Board of the School of Public Health, Fudan University (IRB#2019-07-0767), and oral informed consent was obtained from all surveyed individuals because the survey was anonymous and carried no more than minimum risk. The systematic review in the study was not registered.
Results
Diagnostic performance
Study selection
From the 6 selected electronic literature databases, 1,859 studies were retrieved by search strategies. After the titles and abstracts were reviewed and duplicate publications were removed on the basis of the inclusion and exclusion criteria, 545 studies were selected for the next step. The full texts of the articles were read and screened based on the inclusion and exclusion criteria, and 2 additional studies were identified from the references of the articles. Finally, 28 studies related to AI-assisted CT diagnostic technology for the classification of pulmonary nodules as benign or malignant were included in the systematic review (Figure 1).
Study characteristics
Among the 28 studies on AI-assisted CT diagnostic technology for the classification of pulmonary nodules as benign or malignant, which included 5,727 pulmonary nodules, 25.00% of the studies were published in 2018; 71.43% of the first authors were from China; 25.00% used the gold standard of diagnoses from the LIDC-IDRI dataset; 42.86% used the gold standard of pathologic diagnoses or follow-up; and 32.14%, 25.00% and 10.71% of the data used for machine learning were from hospitals, the Lung Image Database Consortium of Image Database Resource Initiative (LIDC-IDRI) dataset (46) for both hospitals and the LIDC-IDRI dataset, respectively (Appendix 2).
In the development of AI-assisted CT diagnostic technology for the classification of pulmonary nodules as benign or malignant, SVMs, DBNs, DTs, CNNs, ANNs, BNs, Fuzzy C-means (FCMs) and other machine learning classification algorithms accounted for 29.41%, 15.69%, 13.73%, 9.80%, 5.88%, 3.92%, 3.92% and 17.65% of the total 51 times that classification algorithms were used in the 28 studies (more than one algorithm was used in some studies) (Appendix 2 and Appendix 3).
Original study results
The systematic review showed that AI-assisted CT diagnostic technology for the classification of pulmonary nodules as benign or malignant using different classification algorithms had sensitivity rates ranging from 52.00% to 100.00%, specificity rates ranging from 34.69% to 100.00%, and accuracy rates ranging from 56.00% to 100.00% (Appendix 3).
Quality assessment, heterogeneity and publication bias
Quality assessment of the 28 included studies showed that the majority of studies fulfilled the criteria of the reference standard. For example, the reference standard was likely to correctly classify the target condition. Almost all studies had an unclear risk of bias in patient selection and index tests because whether the samples were included randomly or consecutively was not explained, and a threshold was prespecified in the studies. With respect to flow and timing, 9 studies had a high risk of bias, because 7 of them did not adopt the same reference standard, while 2 of them did not include all samples in the testing datasets (Figure 2).
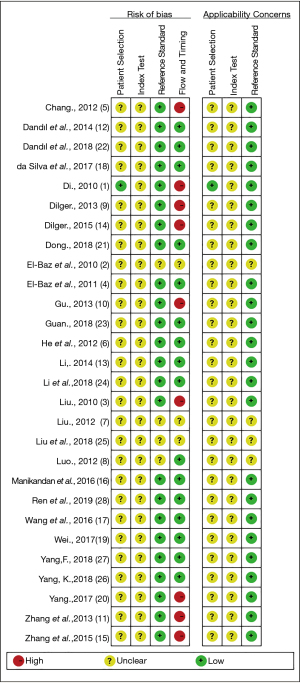
Spearman correlation analysis showed that the Spearman correlation coefficient between sensitivity and 1-specificity was −0.61 (P<0.05). The Cochrane-Q test showed that Q=79.54, I2=97.49% (95% CI: 95.82–99.15%), and P<0.01. These results indicated that there was no heterogeneity in the diagnostic test results caused by the threshold effect, but there was heterogeneity in the diagnostic test results caused by the no-threshold effect. Therefore, a random-effects model was used in our meta-analysis.
Deek’s asymmetry test showed that the coefficient of bias was −17.85 with P<0.05, which indicated that there was potential publication bias.
Pooled diagnostic performance
The meta-analysis weighted by the number of nodules showed that the pooled sensitivity, specificity, PLR and NLR of AI-assisted CT diagnostic technology for the classification of pulmonary nodules as benign or malignant were 0.90 (95% CI: 0.87–0.92), 0.89 (95% CI: 0.85–0.91), 7.95 (95% CI: 5.92–10.67), and 0.11 (95% CI: 0.09–0.15), respectively. The pooled DOR was 70.33 (95% CI: 41.39–119.51) (Table 1).
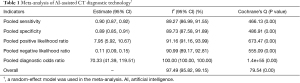
Full table
The AUC of AI-assisted CT diagnostic technology for the classification of pulmonary nodules as benign or malignant was 0.95 (95% CI: 0.93–0.97) (Figure 3).
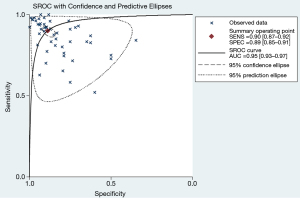
Meta-regression analysis
After a multilevel linear regression model was used to control for the study random effects and other fixed effects (number of nodules and countries), significant differences in the log values of the pooled DOR were detected between classification algorithms used in AI-assisted CT diagnostic technology for the classification of pulmonary nodules as benign or malignant. The log (pooled DOR) for DBN was not different from that for ANN, but was significantly higher than those for other types of classification algorithms. In addition, the model showed that the log (pooled DOR) in the studies of which the first authors were from China was nine times lower than that in studies with first authors from other countries (Table 2). The adjusted pooled DORs for DBN, CNN, DT, ANN, SVM, and other classifiers, as calculated using the above model, were 1318.57, 151.52, 127.46, 65.85, 54.96 and 60.95, respectively, and the adjusted pooled DORs in the groups in which the first authors were from China and from other countries were 47.71 and 372.56, respectively. All of the above adjusted pooled DORs were significantly higher than 1 (Figure S1).
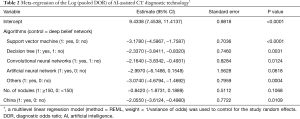
Full table
Physicians’ perceptions
Physician characteristics
Among 406 questionnaires sent to the selected physicians, 345 questionnaires were completed and returned. The response rate of the survey was 84.98%. Among the 345 physicians who responded to the survey, 32.46%, 38.84% and 28.70% were from Shanghai, Hubei Province and Gansu Province, respectively; 69.28% were from general hospitals; and 50.43% and 32.17% were from oncology and imaging departments, respectively. In total, 46.96%, 49.28%, 79.71% and 16.52% of the physicians were in the age group of 30–39 years, were male, had attained a master’s or PhD degree, and were physician managers in a clinical department, respectively. In addition, 20.87% of the surveyed physicians had practical experience using AI-assisted CT diagnostic technology for the classification of pulmonary nodules as benign or malignant (Appendix 4).
Perceptions of the benefits and risks
The study showed that 81.16% and 78.55% of the physicians perceived “reduced workload for radiologists” and “improved diagnostic efficiency”, respectively, as one of the top 3 benefits associated with AI-assisted CT diagnostic technology for the classification of pulmonary nodules as benign or malignant. Furthermore, 46.38% of the physicians perceived “high diagnostic accuracy” as one of the top 3 benefits. In addition, more physicians with practical experience in using AI-assisted CT diagnostic technology for the classification of pulmonary nodules as benign or malignant perceived “improved diagnostic efficiency” as one of the top 3 benefits (88.89%) compared to those without this experience (75.82%) (Table 3).
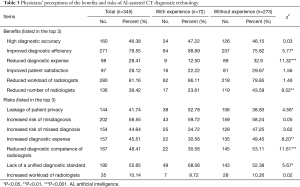
Full table
The study also showed that 58.55%, 55.65%, 48.41%, and 45.51% of the physicians perceived “increased risk of misdiagnosis”, “lack of unified diagnostic standard”, “reduced diagnostic competence of radiologists” and “increased diagnostic expense”, respectively, as one of the top 3 risks associated with AI-assisted CT diagnostic technology for the classification of pulmonary nodules as benign or malignant. A total of 41.74% of the physicians perceived “leakage of patient privacy” as one of the top 3 risks. In addition, more physicians with practical experience perceived “lack of unified diagnostic standard” and “leakage of patient privacy” as one of the top 3 risks compared to those without practical experience (68.06% vs. 52.38% and 52.78% vs. 38.83%, respectively), but fewer physicians with practical experience perceived “increased diagnostic expense” and “reduced diagnostic competence of radiologists” as one of the top 3 risks compared to those without practical experience (30.56% vs. 49.45% and 30.56% vs. 53.11%, respectively) (Table 3).
Attitude toward the clinical application of the technology and its influencing factors
The study revealed that the rate of physicians’ support for the clinical application of AI-assisted CT diagnostic technology for the classification of pulmonary nodules as benign or malignant was 73.62%. Physicians with practical experience had a significantly higher support rate than those without practical experience (87.50% vs. 69.96%, χ2=9.02, P<0.01), even after controlling for physician characteristics [age, sex, type of employee (physician vs. physician manager)] and the physicians’ perception of the benefits and risks associated with AI-assisted CT diagnostic technology for the classification of pulmonary nodules as benign or malignant (Table 4).
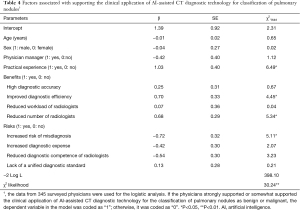
Full table
The univariate logistic model also showed that the physicians who perceived “high diagnostic accuracy” and “reduced number of radiologists” as one of the top 3 benefits had a higher support rate for the clinical application of the technology than did those who did not perceive these factors as a top benefit, while the physicians who perceived “increased risk of misdiagnosis” as one of the top 3 risks had a lower support rate than did those who did not perceive this factor as a top risk (Table 4).
Discussion
Good diagnostic performance
Our analyses showed that the quality of the included studies was generally acceptable and that the AUC of AI-assisted CT diagnostic technology for the classification of pulmonary nodules as benign or malignant was 0.95 (indicating a level of excellence). More specifically, the pooled sensitivity, specificity, PLR and NLR of AI-assisted CT diagnostic technology for the classification of pulmonary nodules as benign or malignant were 0.90, 0.89, 7.95, and 0.11, respectively, and the pooled DOR was 70.33. Our meta-analysis confirms that in the context of lung cancer diagnosis, AI-assisted CT diagnostic technology for the classification of pulmonary nodules as benign or malignant has good diagnostic performance, although the pooled specificity for pulmonary nodule diagnosis (0.89, 95% CI: 0.85–0.91) needs to be further increased by improving the feature extraction methods and reducing the FP rate (47,48).
The trade-off between sensitivity and specificity is crucial for diagnostic technology. When sensitivity and specificity have an inverse relationship, this indicates that a threshold effect is causing heterogeneity in the diagnostic test results (49). The ROC curve also presents the trade-off between sensitivity and specificity. A summary operating point with a small confidence region that is positioned in the upper left corner supports a desirable diagnostic performance of a technology (50). Our study found that no threshold effect was introduced in the meta-analysis and that the summary operating point had a relatively small confidence region (sensitivity: 0.87–0.92 and specificity: 0.85–0.91, respectively) and was positioned in the upper left corner (AUC =0.95). These findings support the good diagnostic performance of this technology.
In addition, the SVM, CNN, ANN and DBN algorithms were most commonly used for diagnosis classification in the included studies. This finding might be either because they have been recently developed and have accurate predictive performances (SVM, CNN and DBN) or because they have been used extensively for nearly 30 years (ANN) (51-53). In particular, the DBN algorithm has witnessed success and shown promising prospects in the classification of pulmonary nodules as benign or malignant in recent years (54). Our meta-regression showed that after the study random effects and other fixed effects were controlled for, the log value of the pooled DOR for DBN was higher than those for SVM, DT, CNN and other types of classification algorithms used in AI-assisted CT diagnostic technology for the classification of pulmonary nodules as benign or malignant.
The study also found that the log (pooled DOR) in the studies of which the first authors came from China was much lower than that in the studies with first authors from other countries. This may be caused by many factors, such as database resources, gold standards, image preprocessing, image segmentation, and nodule features extracted and selected from lung CT images.
The potential risk of study methodological bias and publication bias in the included studies may affect the validity of the results from the systematic review. Therefore, it is worth investigating the perceptions of physicians toward AI-assisted CT diagnostic technology for the classification of pulmonary nodules as benign or malignant, especially those of physicians with practical experience with this technology.
Highly perceived benefits of an improved diagnostic efficiency and reduced workload
Our study found that AI-assisted CT diagnostic technology for the classification of pulmonary nodules as benign or malignant was applied in many tertiary hospitals and used by a portion of relevant physicians. In 9 surveyed hospitals, 8 hospitals had applied this technology, and among 345 physicians who responded to the survey, 20.87% of them had practical experience using the technology. With the increasing demand for CT diagnosis of lung cancer, this technology is expected to be further developed and distributed in hospitals. Regarding the benefits of AI-assisted CT diagnostic technology for the classification of pulmonary nodules as benign or malignant, “reduced workload for radiologists” and “improved diagnostic efficiency” were highly perceived by 81.16% and 78.55% of the physicians, respectively, as one of the top 3 benefits, especially by those with practical experience. This finding is concordant with the general concept of AI-assisted diagnostic technology. This may be because AI excels at recognizing complex patterns in images and thus offers the opportunity to transform image interpretation from a purely qualitative and subjective task to one that is quantifiable and effortlessly reproducible (55). Furthermore, AI highlights and presents regions with suspicious imaging characteristics to the radiologists. These benefits could contribute to a relatively high rate of physician support for the clinical application of AI-assisted CT diagnostic technology for pulmonary nodules (73.62%), especially from physicians with practical experience (87.50%).
More concerns about diagnostic accuracy and patient privacy
CT, which is a commonly used diagnostic tool, provides a large amount of information about a patient’s health. However, correctly interpreting the information is a major challenge for physicians. AI-assisted CT diagnostic technology for the classification of pulmonary nodules as benign or malignant is expected to help physicians detect suspicious lesions that are easily missed and subsequently classify the lesions, thus improving the accuracy of diagnosis (56-58). Although our meta-analysis indicated that AI-assisted CT diagnostic technology for the classification of pulmonary nodules as benign or malignant has a good diagnostic performance, fewer than 50% of the physicians perceived “high diagnostic accuracy” as one of the top 3 benefits, and more than 50% of the physicians perceived “increased risk of misdiagnosis” as one of the top 3 risks of this technology. Other concerns about this technology included AI-assisted diagnostic standards, the effects on radiologists’ competence and diagnostic expenses, missed diagnoses, and patient privacy protection.
Physicians provide the diagnoses, and each CT image analyzed by a computer is verified by diagnostic physicians (59). Thus, AI-assisted CT diagnostic technology for the classification of pulmonary nodules as benign or malignant will not replace physicians in making a final diagnosis, and the effects of this technology on diagnostic accuracy and radiologists’ competence should not be excessively considered.
Nevertheless, it should be noted that physicians with practical experience had more concerns about unified diagnostic standards and patient privacy protection in the development of the technology than did those without practical experience (68.06% vs. 52.38% and 52.78% vs. 38.83%, respectively). Because large amounts of CT data are rarely curated in terms of labeling, annotation, segmentation, quality assurance, or fitness for the problem at hand (55), it is very difficult to establish a unified diagnostic standard. However, standardized benchmarking is of particular importance in the medical field, especially given the multitude of imaging modalities and anatomic sites, as well as acquisition standards and hardware (60). In addition, larger and publicly available databases are needed for improved validation (60). During the collection and use of patient data, measures to protect patient privacy and sensitive health information should be undertaken.
Factors affecting the rate of physician support for the clinical application of the technology
The overall rate of physician support for the clinical application of AI-assisted CT diagnostic technology for the classification of pulmonary nodules as benign or malignant was 73.62%. Physicians with practical experience had a significantly higher support rate than those without practical experience (87.50% vs. 69.96%), and the logistic model demonstrated a similar result. Moreover, the study showed that diagnostic accuracy (including misdiagnosis) and practical experience significantly influence physicians’ level of support for the clinical application of this technology. These findings indicate that AI-assisted CT diagnostic technology for the classification of pulmonary nodules as benign or malignant should be further improved to better meet physicians’ expectations.
To improve the diagnostic accuracy of AI-assisted CT diagnostic technology for the classification of pulmonary nodules as benign or malignant, extracting and selecting the most discriminative features (such as the size, shape, spatial complexity, intensity patterns, and a range of other “texture” features and “radiomics” of pulmonary nodules), developing large available datasets to capture a sufficiently broad disease spectrum and exploring better classification algorithms are the key issues and require cooperation among physicians, radiologists, and computer technicians (12).
Study limitations
Our study had some limitations. First, there was a risk of study methodological bias and publication bias in the included studies, which may affect the results from our systematic review. Second, the studies included in the meta-analysis were published from 2010 to 2019 and written in English or Chinese, and only one study from 2019 was included in this study because other identified studies from 2019 contained insufficient data for the analyses. These restrictions may have led to relevant studies being missed and not included in the systematic review. Third, the surveyed physicians were from public tertiary hospitals in China, and therefore, the findings from the survey have limited generalizability. Considering that public tertiary hospitals are the main medical setting in which CT scans for lung cancer are performed and clinical trials on the diagnostic performance of AI-assisted CT diagnostic technology for the classification of pulmonary nodules as benign or malignant are conducted in China, our findings from the representative survey of hospital physicians may reflect physicians’ perceptions of the technology to some extent.
Conclusions
AI-assisted CT diagnostic technologies for the classification of pulmonary nodules as benign or malignant have shown great promise and are under development. Our study confirms that in the context of lung cancer diagnosis, AI-assisted CT diagnostic technology for the classification of pulmonary nodules as benign or malignant has a good diagnostic performance (pooled DOR=70.33). The majority of physicians in China who were surveyed believed that this technology can improve diagnostic efficiency and reduce the workload for radiologists. Moreover, diagnostic accuracy (including the misdiagnosis rate) and practical experience were significantly associated with whether physicians supported the clinical application of this technology. Therefore, the specificity of this technology needs to be improved.
Acknowledgments
Funding: This work was supported by the National Center for Medical Service Administration, Beijing, China.
Footnote
Reporting Checklist: The authors have completed the PRISMA reporting checklist. Available at https://dx.doi.org/10.21037/jtd-21-810
Conflicts of Interest: All authors have completed the ICMJE uniform disclosure form (available at https://dx.doi.org/10.21037/jtd-21-810). All authors report grants from the National Center for Medical Service Administration, Beijing, P.R. China, during the conduct of the study.
Ethical Statement: The authors are accountable for all aspects of the work in ensuring that questions related to the accuracy and integrity of any part of the work are appropriately investigated and resolved. The study was conducted in accordance with the Declaration of Helsinki (as revised in 2013). The study was approved by the ISB of the School of Public Health, Fudan University (IRB# 2019-07-0767), and oral informed consent was obtained from all surveyed individuals because the survey was anonymous and carried no more than minimum risk.
Open Access Statement: This is an Open Access article distributed in accordance with the Creative Commons Attribution-NonCommercial-NoDerivs 4.0 International License (CC BY-NC-ND 4.0), which permits the non-commercial replication and distribution of the article with the strict proviso that no changes or edits are made and the original work is properly cited (including links to both the formal publication through the relevant DOI and the license). See: https://creativecommons.org/licenses/by-nc-nd/4.0/.
References
- Sung H, Ferlay J, Siegel RL, et al. Global Cancer Statistics 2020: GLOBOCAN Estimates of Incidence and Mortality Worldwide for 36 Cancers in 185 Countries. CA Cancer J Clin 2021;71:209-49. [Crossref] [PubMed]
- Amir GJ, Lehmann HP. After Detection: The Improved Accuracy of Lung Cancer Assessment Using Radiologic Computer-aided Diagnosis. Acad Radiol 2016;23:186-91. [Crossref] [PubMed]
- Gadgeel SM, Ramalingam SS, Kalemkerian GP. Treatment of lung cancer. Radiol Clin North Am 2012;50:961-74. [Crossref] [PubMed]
- U.S. Preventive Services Task Force. Lung cancer screening: recommendation statement. Ann Intern Med 2004;140:738-9. [Crossref] [PubMed]
- ur Rehman MZ, Javaid M, Shah SIA, et al. An appraisal of nodules detection techniques for lung cancer in CT images. Biomed Signal Process Control 2018;41:140-51.
- Henschke CI, McCauley DI, Yankelevitz DF, et al. Early Lung Cancer Action Project: overall design and findings from baseline screening. Lancet 1999;354:99-105. [Crossref] [PubMed]
- Mazzone PJ, Silvestri GA, Patel S, et al. Screening for Lung Cancer: CHEST Guideline and Expert Panel Report. Chest 2018;153:954-85. [Crossref] [PubMed]
- Moyer VAU.S. Preventive Services Task Force. Screening for lung cancer: U.S. Preventive Services Task Force recommendation statement. Ann Intern Med 2014;160:330-8. [Crossref] [PubMed]
- National Comprehensive Cancer Network. Available online: https://www.nccn.org/professionals/physician_gls/pdf/lung_screening.pdf. Accessed April 13, 2020.
- Gould MK, Donington J, Lynch WR, et al. Evaluation of individuals with pulmonary nodules: when is it lung cancer? Diagnosis and management of lung cancer, 3rd ed: American College of Chest Physicians evidence-based clinical practice guidelines. Chest 2013;143:e93S-e120S.
- Baldwin DR, Callister MEGuideline Development Group. The British Thoracic Society guidelines on the investigation and management of pulmonary nodules. Thorax 2015;70:794-8. [Crossref] [PubMed]
- Ather S, Kadir T, Gleeson F. Artificial intelligence and radiomics in pulmonary nodule management: current status and future applications. Clin Radiol 2020;75:13-9. [Crossref] [PubMed]
- Rubin GD. Lung nodule and cancer detection in computed tomography screening. J Thorac Imaging 2015;30:130-8. [Crossref] [PubMed]
- De Fauw J, Ledsam JR, Romera-Paredes B, et al. Clinically applicable deep learning for diagnosis and referral in retinal disease. Nat Med 2018;24:1342-50. [Crossref] [PubMed]
- Ravizza S, Huschto T, Adamov A, et al. Predicting the early risk of chronic kidney disease in patients with diabetes using real-world data. Nat Med 2019;25:57-9. [Crossref] [PubMed]
- Yassin NIR, Omran S, El Houby EMF, et al. Machine learning techniques for breast cancer computer aided diagnosis using different image modalities: A systematic review. Comput Methods Programs Biomed 2018;156:25-45. [Crossref] [PubMed]
- Li G, Kim H, Tan JK, et al. Semantic characteristics prediction of pulmonary nodule using Artificial Neural Networks. Annu Int Conf IEEE Eng Med Biol Soc 2013;2013:5465-8. [PubMed]
- Xie Y, Zhang J, Liu S, et al. Lung Nodule Classification by Jointly Using Visual Descriptors and Deep Features. In: Müller H. et al. (eds) Medical Computer Vision and Bayesian and Graphical Models for Biomedical Imaging. BAMBI 2016, MCV 2016. Lecture Notes in Computer Science, vol 10081. Springer, Cham 2017.
- Lo SCB, Hsu LY, Freedman MT, et al. Classification of lung nodules in diagnostic CT: an approach based on 3D vascular features, nodule density distribution, and shape features. Proceedings of SPIE 2003;5032:183-9. [Crossref]
- Tajbakhsh N, Suzuki K. Comparing two classes of end-to-end machine-learning models in lung nodule detection and classification: MTANNs vs. CNNs. Pattern Recognition 2016;63:476-86. [Crossref]
- Rendon-Gonzalez E, Ponomaryov V. Automatic lung nodule segmentation and classification in CT images based on SVM. Paper presented at 9th International Kharkiv Symposium on Physics and Engineering of Microwaves, Millimeter and Submillimeter Waves, MSMW 2016, 1-4.
- Wang J, Liu X, Dong D, et al. Prediction of malignant and benign of lung tumor using a quantitative radiomic method. Annu Int Conf IEEE Eng Med Biol Soc 2016;2016:1272-5. [Crossref] [PubMed]
- Dhara AK, Mukhopadhyay S, Dutta A, et al. A Combination of Shape and Texture Features for Classification of Pulmonary Nodules in Lung CT Images. J Digit Imaging 2016;29:466-75. [Crossref] [PubMed]
- Li X, Shen L, Luo S. A Solitary Feature-Based Lung Nodule Detection Approach for Chest X-Ray Radiographs. IEEE J Biomed Health Inform 2018;22:516-24. [Crossref] [PubMed]
- Hinton GE, Osindero S, Teh YW. A fast learning algorithm for deep belief nets. Neural Comput 2006;18:1527-54. [Crossref] [PubMed]
- Priya MMMA, Jawhar DSJ, Geisa DJM. Optimal Deep Belief Network with Opposition based Pity Beetle Algorithm for Lung Cancer Classification: A DBNOPBA Approach. Comput Methods Programs Biomed 2021;199:105902 [Crossref] [PubMed]
- Ampavathi A, Saradhi TV. Multi disease-prediction framework using hybrid deep learning: an optimal prediction model. Comput Methods Biomech Biomed Engin 2021; Epub ahead of print. [Crossref] [PubMed]
- Nishio M, Nishizawa M, Sugiyama O, et al. Computer-aided diagnosis of lung nodule using gradient tree boosting and Bayesian optimization. PLoS One 2018;13:e0195875 [Crossref] [PubMed]
- Mukherjee J, Chakrabarti A, Shaikh SH, et al. Automatic detection and classification of solitary pulmonary nodules from lung CT images. Emerging Applications of Information Technology 2014. doi:
10.1109/EAIT.2014.64 .10.1109/EAIT.2014.64 - Kawagishi M, Kubo T, Sakamoto R, et al. Automatic inference model construction for computer-aided diagnosis of lung nodule: Explanation adequacy, inference accuracy, and experts' knowledge. PLoS One 2018;13:e0207661 [Crossref] [PubMed]
- Shen W, Zhou M, Yang F, et al. Multi-crop convolutional neural networks for lung nodule malignancy suspiciousness classification. Pattern Recognition 2017;61:663-73. [Crossref]
- Hussein S, Cao K, Song Q, Bagci U. Risk Stratification of Lung Nodules Using 3D CNN-Based Multi-task Learning. In: Niethammer M. et al. (eds) Information Processing in Medical Imaging. IPMI 2017. Lecture Notes in Computer Science, vol 10265. Springer, Cham.
- Liu S, Xie Y, Jirapatnakul A, et al. Pulmonary nodule classification in lung cancer screening with three-dimensional convolutional neural networks. J Med Imaging (Bellingham) 2017;4:041308 [Crossref] [PubMed]
- Wu W, Hu H, Gong J, et al. Malignant-benign classification of pulmonary nodules based on random forest aided by clustering analysis. Phys Med Biol 2019;64:035017 [Crossref] [PubMed]
- Aggarwal P, Vig R, Sardana HK. Patient-wise versus nodule-wise classification of annotated pulmonary nodules using pathologically confirmed cases. JCP 2013;8:2245-55. [Crossref]
- Mao K, Deng Z. Lung nodule image classification based on ensemble machine learning. Journal of Medical Imaging and Health Informatics 2016;6:1679-85. [Crossref]
- Kumar D, Wong A, Clausi DA. Lung nodule classification using deep features in CT images. In: Proceedings of the 2015 12th Conference on Computer and Robot Vision (CRV) 2015: 133-8.
- Li H, Wang Y, Liu KJ, et al. Computerized radiographic mass detection--part II: Decision support by featured database visualization and modular neural networks. IEEE Trans Med Imaging 2001;20:302-13. [Crossref] [PubMed]
- Cruz JA, Wishart DS. Applications of machine learning in cancer prediction and prognosis. Cancer Inform 2007;2:59-77. [PubMed]
- Zhou J, Li H, Yang Y. Elements for ethical review of artificial intelligence medical device. Medicine and Philosophy 2020;41:35-39, 56.
- Whiting PF, Rutjes AW, Westwood ME, et al. QUADAS-2: a revised tool for the quality assessment of diagnostic accuracy studies. Ann Intern Med 2011;155:529-36. [Crossref] [PubMed]
- Bradley AP. The use of the area under the ROC curve in the evaluation of machine learning algorithms. Pattern Recognition 1997;30:1145-59. [Crossref]
- Zamora J, Abraira V, Muriel A, et al. Meta-DiSc: a software for meta-analysis of test accuracy data. BMC Med Res Methodol 2006;6:31. [Crossref] [PubMed]
- Egger M, Davey Smith G, Schneider M, et al. Bias in meta-analysis detected by a simple, graphical test. BMJ 1997;315:629-34. [Crossref] [PubMed]
- Begg CB, Mazumdar M. Operating characteristics of a rank correlation test for publication bias. Biometrics 1994;50:1088-101. [Crossref] [PubMed]
- Armato SG 3rd, McLennan G, Bidaut L, et al. The Lung Image Database Consortium (LIDC) and Image Database Resource Initiative (IDRI): a completed reference database of lung nodules on CT scans. Med Phys 2011;38:915-31. [Crossref] [PubMed]
- Morris MA, Saboury B, Burkett B, et al. Reinventing Radiology: Big Data and the Future of Medical Imaging. J Thorac Imaging 2018;33:4-16. [Crossref] [PubMed]
- Valente IR, Cortez PC, Neto EC, et al. Automatic 3D pulmonary nodule detection in CT images: A survey. Comput Methods Programs Biomed 2016;124:91-107. [Crossref] [PubMed]
- Reitsma JB, Glas AS, Rutjes AW, et al. Bivariate analysis of sensitivity and specificity produces informative summary measures in diagnostic reviews. J Clin Epidemiol 2005;58:982-90. [Crossref] [PubMed]
- Jeong E, Park J, Lee J. Diagnostic Test Accuracy of the 4AT for Delirium Detection: A Systematic Review and Meta-Analysis. Int J Environ Res Public Health 2020;17:7515. [Crossref] [PubMed]
- Simes RJ. Treatment selection for cancer patients: application of statistical decision theory to the treatment of advanced ovarian cancer. J Chronic Dis 1985;38:171-86. [Crossref] [PubMed]
- Mastouri R, Khlifa N, Neji H, et al. A bilinear convolutional neural network for lung nodules classification on CT images. Int J Comput Assist Radiol Surg 2021;16:91-101. [Crossref] [PubMed]
- Kourou K, Exarchos TP, Exarchos KP, et al. Machine learning applications in cancer prognosis and prediction. Comput Struct Biotechnol J 2014;13:8-17. [Crossref] [PubMed]
- Sun W, Zheng B, Qian W. Automatic feature learning using multichannel ROI based on deep structured algorithms for computerized lung cancer diagnosis. Comput Biol Med 2017;89:530-9. [Crossref] [PubMed]
- Bi WL, Hosny A, Schabath MB, et al. Artificial intelligence in cancer imaging: Clinical challenges and applications. CA Cancer J Clin 2019;69:127-57. [Crossref] [PubMed]
- van Ginneken B, Armato SG 3rd, de Hoop B, et al. Comparing and combining algorithms for computer-aided detection of pulmonary nodules in computed tomography scans: The ANODE09 study. Med Image Anal 2010;14:707-22. [Crossref] [PubMed]
- Doi K. Diagnostic imaging over the last 50 years: research and development in medical imaging science and technology. Phys Med Biol 2006;51:R5-27. [Crossref] [PubMed]
- Giger ML, Chan HP, Boone J. Anniversary paper: History and status of CAD and quantitative image analysis: the role of Medical Physics and AAPM. Med Phys 2008;35:5799-820. [Crossref] [PubMed]
- Prabhakar B, Shende P, Augustine S. Current trends and emerging diagnostic techniques for lung cancer. Biomed Pharmacother 2018;106:1586-99. [Crossref] [PubMed]
- Shiraishi J, Katsuragawa S, Ikezoe J, et al. Development of a digital image database for chest radiographs with and without a lung nodule: receiver operating characteristic analysis of radiologists' detection of pulmonary nodules. AJR Am J Roentgenol 2000;174:71-4. [Crossref] [PubMed]