CHMP4C regulates lung squamous carcinogenesis and progression through cell cycle pathway
Introduction
Lung cancer is one of the most common diagnosed cancer in the world, which detrimentally affect the quality of patients’ life (1). There are newly diagnosed about 1.8 and 1.6 million deaths occurred in the worldwide each year (2). Broadly, there are two major types of lung cancers: small cell lung cancer (SCLC) and non-small cell lung cancer (NSCLC). At present, NSCLC is defined histopathologically in the clinic into four broad categories: lung squamous cell carcinoma (LUSC), lung adenocarcinoma (LUAD), large cell carcinoma and undifferentiated NSCLC (3). LUSC is the most common type of NSCLC, which causes 400,000 deaths worldwide each year (4). For LUSC cases in TNM stage II, the 5-year survival rate is 40%, while the survival rate for LUSC cases of pathological TNM stage IV is <5% (5). Currently, thorough clinical examination and histological analysis of suspected sites are performed in diagnosing LUSC, but early diagnosis is difficult to performed for limited sensitivity and specificity (6). Meanwhile, expensive treatment options also remain ineffective sometimes due to poor prognosis (7). Effective diagnostic and prognostic biomarkers of LUSC are urgent to find (8).
Dysregulation of tumor cell cycle is usually caused by changes in oncogenes which indirectly affect cell cycle or cell cycle regulation (9). The cell cycle plays a significant role in the development of tumor, and abnormalities in cell cycle regulation lead to uncontrolled cell proliferation, making it an attractive therapeutic target for cancer therapy (10). Current studies have shown that an increasing number of cell cycle-related genes/proteins have become targets for cancer therapy and early diagnosis. For example, Cyclin D1 is related to the development and progression of cancers, which can cause poorer overall survival when expressing high (11). MuvB can mediate the suppression of cycle transcription activation or silent phase in ovarian cancer at different time points in cell cycle, which can be used in cancer therapy after targeting (12).
We present the following article in accordance with the TREND reporting checklist (available at https://dx.doi.org/10.21037/jtd-21-583).
Methods
Data acquisition
Clinical features and RNA-seq expression data with LUSC were collected from the TCGA database (https://portal.gdc.cancer.gov/), including 60,498 genes. The eligible LUSC database GSE19188 was searched in the Gene Expression Omnibus (GEO) database (http://www.ncbi.nlm.nih.gov/geo/), which has a total of 54,676 probes and 22,835 genes. And the original files (.CEL files) and platform files were downloaded. Raw RNA sequencing dataset was normalized via DESeq package in the R platform.
Patient selection
Patients who underwent surgical resection of primary LUSC at The Third Affiliated Hospital of Qiqihar Medical College, Qiqihar, Heilongjiang Province, China between Dec 2019 and Feb 2021 were selected for this study. Tumor and non-tumor specimens (located >2 cm from the tumor margin) obtained from the enrolled patients were analyzed by qPCR and WB for CHMP4C. All patients did not receive chemotherapy or radiotherapy prior to surgery. Pathological diagnosis was made by 2 pathologists based on depth of infiltration, degree of differentiation, lymph node metastasis, microvascular invasion, and nerve invasion. The study was conducted in accordance with the Declaration of Helsinki (as revised in 2013). The Third Affiliated Hospital of Qiqihar Medical College institutional review board approved the study protocol (2019LL-23) and individual consent for this retrospective analysis was waived.
Analysis on up-regulated differentially expressed genes (DEGs)
DEGs between patients with LUSC and controls in TCGA database and GEO database were identified with DESeq package in R software. In addition, P<1e−4 was considered as the cutoff value for screening DEGs. The Cox univariate survival analysis data from the TCGA database, the upregulated DEGs from the TCGA database and the GEO database, and the genes associated with the cell cycle pathway from the c2 Molecular Signatures database were used to intersect to obtain the overlapping regions as in the Venn diagram.
Receiver operating characteristic (ROC) curve and logistic regression analysis
Referring to the RNA-sequence data and clinicopathological characteristics of samples in two groups, the LUSC tumor category and the adjacent normal one were obtained by screening out the relevant documents (.count files) and clinical information related to LUSC from TCGA database and the GEO database. The data before log2 conversion were normalized and processed in R software. The sensitivity and specificity of biomarker for LUSC diagnosis were evaluated with ROC curve, which was performed on the analysis by evaluating the accuracy of the biomarker expression in predicting the prognosis of LUSC performed by “survival” package in the R software, and the area under the curve (AUC) could also be investigated with the software “medcalc”. For the further assessment on the efficacy of genes in diagnosing LUSC, five-fold cross-validation was performed with logistic regression in TCGA database (13). The logistic regression model was established with “scikit-learn” package in Python software (version 3.6, https://www.python.org) (14). Precision was introduced together with recall, accuracy and F1-score to evaluate this classification model. The precision of a classifier was calculated as the number of true positives divided by the number that we predicted to be positive. High precision indicated high accuracy of LUSC predicted by the biomarker. Recall was defined as true positives’ number divided by actual positives’ number. Accuracy referred to the proportion of samples with correctly predicted outcomes. Because of the interaction between precision and recall and their values that could not be ideal and large, we took the F1-score as a comprehensive evaluation index, which was the harmonic average of precision and recall.
Cell culture and transient transfection
Human LUSC cell lines NCI-H596, SK-MES-1, LK2, H520, SW900, LUDLU1 and human normal lung cell line CCD-8L were gained from ATCC (Beijing Beina Chuanglian Biotechnology Institute), cultured in F12 and DMEM containing 10% fetal bovine serum (Gibco, Carlsbad, CA, USA), respectively. Both the cell lines were stored in a humidified incubator at 37 °C with 5% CO2. Negative Control (NC) and CHMP4C siRNAs (Sigma-Aldrich, USA) was transfected into SK-MES-1 cells as the manufacturer's protocol suggested with Lipofectamine 2000 (Invitrogen, Carlsbad, CA, USA). Target sequences for siRNAs were 5'-CCUCUGGCAUUAGAAUUAUTT-3'(si-NC), 5'-CCUGCGUCUCUACAACUA U-3' (si-CHMP4C).
RNA extraction and qRT-PCR
Total RNA was extracted from LUSC-related cells with Trizol Reagent (Invitrogen, Carlsbad, CA, USA), which was reversely transcribed with RT reagent Kit gDNA Eraser (TaKaRa). And then, cDNA expression levels were detected by SYBR-Green (TaKaRa) and qRT-PCR analysis with GAPDH as internal reference. The primers were: GAPDH, Forward (F): 5'-TGTGTCCGTCGTGGATCTGA-3', Reverse(R): 5'-CCTGCTTCACCACCTTCTTGA-3'; hCHMP4C, Forward (F): 5'-AGACTGAGGAGATGCTGGGCAA-3', Reverse (R): 5'-TAGTGCCTGTAATGCAGCTCGC-3'. PCR amplification was carried out in a formula of three Wells. All experiments were repeated three times and genes’ relative expression levels were studied with 2−ΔΔCt.
Western blot
The concentration of total proteins extracted with lysis buffer including protease inhibitors was measured by BCA protein assay kit (Thermo Scientific Pierce). PVDF membranes (Millipore, Bedford, MA, USA) with proteins isolated on SDS-PAGE gels were blocked by 5% nonfat dry milk and incubated with primary antibodies at 4 °C overnight. Western blot was performed with an ECL assay reagent (Millipore) according to the manufacturer’s protocol. Antibodies used were: CHMP4C (Abcam, Cambridge, UK, ab168205), CCNA2 (Abcam, Cambridge, UK, ab181591), CDK2 (Abcam, Cambridge, UK, ab32147), SKP1 (Abcam, Cambridge, UK, ab80586), GAPDH (Santa Cruz Biotechnology, sc-47724).
Cell cycle analysis
The cells were harvested, fixed with 70% ethanol at 4 °C overnight, and stained with RNase A with Propidium Iodide (Sigma-Aldrich, USA). Cell cycle distribution was determined by flow cytometry with ModFit software, with Blank control, si-NC, and si-CHMP4C groups established, as well as green emission.
Statistical analysis
The Kaplan-Meier analysis for overall survival was proceeded according to gene’s cut-off level set at the median value with the “survival” package in R software. Univariate and multivariable analyses were performed with the “survival” package in R software, with a Cox proportional hazard regression model established. Patients were grouped according to the expression of targeted genes, and the correlation with the clinicopathological characteristics of TCGA was studied by χ2 test. Besides, the linear relationship between two groups was determined through Pearson correlation analysis. Each experiment was repeated three times or more and all data were presented as mean ± standard deviation (SD). Statistical significance was described as follows: n.s, not significant; *P≤0.05; **P≤0.01; ***P≤0.001.
Results
Identification of the unique DEGs related to cell cycle in LUSC
As shown in the volcano plots, gene expression profiles from TCGA LUSC database (498 patients with LUSC and 50 controls) identified 17,360 differential expressed genes with 9,027 up-regulated genes and 8,333 down-regulated genes in samples of LUSC compared with normal control tissues (Figure 1A). 11,344 differential expressed genes were ascertained in GSE19188 database (27 patients with LUSC and 65 control ones), comprising 5,992 up-regulated genes and 5,352 down-regulated genes in LUSC (Figure 1B). The differential genes from the TCGA database and GSE19188 database were combined to obtain 4,162 common DEGs, gene expression profiles from paired normal control and tumor samples in TCGA database confirmed that these 4,162 genes were overexpressed in samples of LUSC (Figure 1C). Referring to the cut-off criteria, 9,027 up-regulated genes from the TCGA database, 5,992 up-regulated genes from the GSE19188 database, 1,873 genes from the TCGA database with the Cox univariate survival rate analysis, and 1,383 genes associated with cell cycle pathways from c2 Molecular Signatures database gene sets were intersected to obtain the unique gene CHMP4C (Figure 1D).
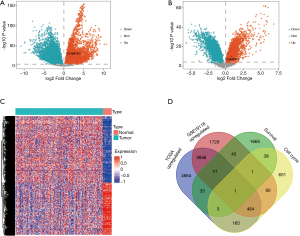
CHMP4C is a biomarker for diagnosis of LUSC
To confirm the expression of CHMP4C in LUSC, we collected 30 patients aged 27 to 85 years old (at the mean age of 62.8 years old), who underwent primary LUSC surgical excision were selected for comparison of tumor and non-tumor specimens (located at tumor margins >2 cm). Then, the CHMP4C protein expression was detected by Western blot, and qRT-PCR analysis was conducted to determine the mRNA levels of CHMP4C. Western blot showed that CHMP4C was overexpressed in patients with LUSC (Figure 2A), with the findings similar to the mRNA expression in LUSC tissues (Figure 2B). The CHMP4C in LUSC tumor tissues expressed relatively higher than paracancerous normal tissues (P<0.001). To sum up, these data suggested CHMP4C was up-regulated in LUSC.
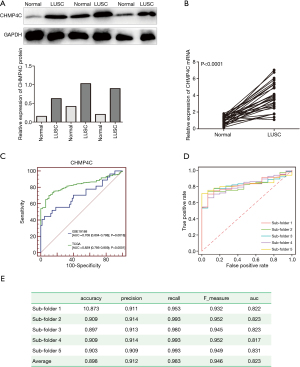
In order to evaluate the sensitivity and specificity of CHMP4C in diagnosing LUSC, ROC curves of CHMP4C from TCGA database and GSE19188 database were shown (Figure 2C), indicating that the average AUC was 0.829 and 0.708, respectively. Then, logical regression was modeled to further evaluate effectiveness of CHMP4C in diagnosing LUSC. In the ROC curve of CHMP4C derived from the regression model (Figure 2D), the average AUC was 0.823, and the average values of accuracy, precision, recall, and F1-score were 0.898, 0.912, 0.983, and 0.946, respectively (Figure 2E). These results implied that the CHMP4C could be used to distinguish well between LUSC samples and normal controls.
CHMP4C is an independent predictor of prognosis in LUSC patients
To test whether CHMP4C was a better biomarker of survival rate, we performed the univariate Cox analysis, and then found that among many clinical indicators, only CHMP4C expression could well predict the prognosis of patients and was a potential target for prognosis prediction (P=0.04, Table 1). Then the relationship between the expression of CHMP4C gene and clinical characteristics was evaluated using chi-square test. The statistical results in Table S1 demonstrated that the expression of CHMP4C was related to tumor T stage (P=0.018). Based on clinical variables of patients with LUSC, Kaplan-Meier analysis with data in TCGA revealed significant differences in survival rate among patients with different CHMP4C expression. Those data with high CHMP4C expression often associated with worse survival rate (P=0.002) (Figure 3A). Further we created a nomogram that the overall survival was predicted for patients at 1, 3, and 5 years (Figure 3B). Good accuracy was obtained by nomogram calibration curves for 1-year survival rate, and 3-year survival rate (Figure 3C,3D).
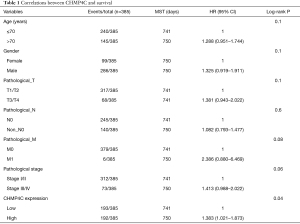
Full table
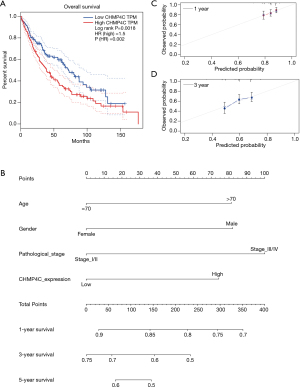
Genes positively associated to CHMP4C are enriched in cell cycle
For the further exploration of the potential biological function of CHMP4C in LUSC, correlation analysis was made to assess the relationship between the most significantly different genes which included the cell cycle pathway genes in the two groups of high and low CHMP4C expression, it was found that CHMP4C was positively correlated with 14 genes related to cell cycle pathway: CCNE2 (CyclinE2), SKP1 (S-phase kinase associated protein 1), DCTN2 (Dynactin subunit 2), NSMCE2 (NSE2 homolog), ORC4 (Origin recognition complex subunit 4), PPPICB (Protein phosphatase 1 catalytic subunit beta), PRKAG1 (Protein kinase AMP-activated non-catalytic subunit gamma 1), REEP3 (Receptor accessory protein 3), RHOC (Ras homolog family member C), SKIL (SKI like proto-oncogene), TOM1L1 (Target of myb1 like 1 membrane trafficking protein), UBE2B (Ubiquitin conjugating enzyme E2 B), YAF2 (YY1 associated factor 2), ZFYVE19 (Zinc finger FYVE-type containing 19) in 485 samples of LUSC from TCGA database (Figure 4). According to the results, we deduced CHMP4C which could regulate mitotic cell cycle progression in LUSC with an important role during tumorigenesis.
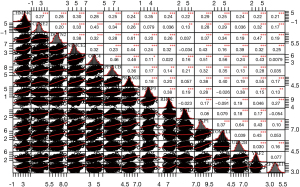
CHMP4C controls LUSC cell cycle in vitro
To verify the effect of CHMP4C on cell cycle, we detected the expression of CHMP4C in six lung squamous carcinoma cell lines (NCI-H596, SK-MES-1, LK2, H520, SW900, LUDLU1) and human normal lung cell line CCD-8L. By using qRT-PCR and Western Blot, we found that the protein (Figure 5A) and mRNA (Figure 5B) levels of CHMP4C in each LUSC cell line increased, compared with normal lung cell line CCD-8L, with significant differences (P<0.05). BrdU cell proliferation assay after knockdown of CHMP4C in LK3, LUDLU1, and SK-MES-1 cells revealed that the cell proliferation rate of si-CHMP4C was significantly reduced in all three cell lines, compared with si-NC and Blank (Figure S1A-S1C). Because the cell proliferation inhibition rate of LK3, and LUDLU1 was not as significant compared with SK-MES-1 cells after CHMP4C knockdown, SK-MES-1 cells were selected for subsequent experiments. Then CHMP4C was knocked down in SK-MES-1 cell lines. Firstly, we examined the silencing effect of CHMP4C by qRT-PCR and Western blot, showing that both protein and mRNA expression of CHMP4C were significantly reduced after transfection with si-CHMP4C (Figure 5C,5D), with significant differences (P<0.001). Cell cycle was analyzed with flow cytometry, the SK-MES-1 cells with CHMP4C suppression were observed to arrest in S phase as shown in (Figure 5E). The cell cycle is a sophisticated regulation process, which is mainly controlled by different cyclin-dependent kinases (CDKs) and their functional cyclin partners. CDK2 is an important G1/S transition regulator, and its activation allows G1/S phase transition. CyclinA2 is a highly conserved protein that is involved in control of the transition of the cell cycle from the G1/S phase to the G2/M phase, and cell mitosis. S phase kinase-associated protein (Skp1) is a highly conserved ubiquitous eukaryotic protein belonging to the SCF complex. SCF complexes are involved in the regulated ubiquitination of specific protein substrates, which targets them for degradation by the proteosome, many specific SCF substrates have been identified including regulators of cell cycle progression and development. Skp1 is essential for cell cycle progression at the G1/S transitions. To investigate the underlying molecular effects of the reduced cell cycle progression upon CHMP4C knockdown, the expression of cell cycle-related genes, SKP1, cyclinA2 and CDK2, was examined at the protein level by western blot analysis (Figure 5F). We quantified the proteins levels of SKP1, cyclinA2 and CDK2, which are known to play a critical role in cell cycle regulation, and the experimental results showed that CHMP4C knockdown significantly increased SKP1 protein level (Figure 5G, P<0.001), while significantly decreased cyclinA2 and CDK2 protein expression (Figure 5H,I, P<0.001). The above experimental results proved that CHMP4C was involved in controlling cell cycle progression.
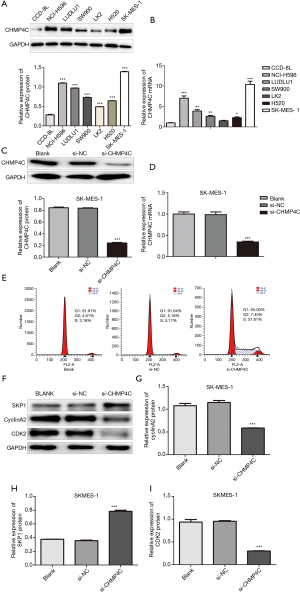
Discussion
CHMP4C belongs to the charged multivesicular body protein (CHMP) family. There are 10 proteins in the CHMP family namely CHMP1A, CHMP1B, CHMP2A, CHMP2B, CHMP3, CHMP4A, CHMP4B, CHMP4C, CHMP5, CHMP6 (15), which are components of ESCRT-III (Endosomal sorting complex required for transport III), mediating membrane division at the end of cytoplasmic division. Cytokinetic shedding promotes daughter cells’ irreversible separation, which requires the endosomal cell sorting complex for the translocation (ESCRT) machinery tightly regulated by charged multivesicular body protein 4C (CHMP4C), an ESCRT-III subunit involved in the abscission checkpoint (NoCut) in response to mitotic issues. A recent study found that CHMP4C has played crucial roles in cell cycle control (16), and it can detect shedding time through aurora b-directed phosphorylation, coordinate intermediate resolution, and prevent the accumulation of DNA damage during cell division (17). The CHMP4C gene identified in this study has been previously proved to be up-regulated in cervical cancer tissues, promoting proliferation, migration and invasion of cells (18), silencing CHMP4C strengthens the sensitivity of lung cancer cells to radiation exposure by delaying the S phase in cell cycle of lung cancer (17). CHMP4C protects human by promoting the localization of the spindle checkpoint complex to unlinked attachment sites cells from chromosome missegregation (19). Overall, although CHMP4C is involved in segregating checkpoints, how it plays a role in lung squamous carcinoma formation remains unclear.
This study focused on potential cancer genes with diagnostic and prognostic value. By cross-analysis of 9,027 up-regulated genes in the TCGA database, 5,992 up-regulated genes in the GSE19188 database, 1,873 Cox univariate survival rate analysis genes in the TCGA database, and 1,383 cell cycle pathway-related genes in the c2 Molecular Signatures Database gene sets, we found that the only gene with proven diagnostic value for LUSC was CHMP4C. This gene is a potential marker with high sensitivity and specificity. Further validation of the gene expression in the TCGA database and clinical samples demonstrated that CHMP4C overexpressed in tumor tissue compared to normal lung tissue, especially in patients with low stromal score, low immune score, low estimation score, and high tumor purity (Figure S2). To further explore the role of CHMP4C in LUSC, we analyzed the overall survival of patients with LUSC in TCGA database by Kaplan-Meier Plot and found that high CHMP4C expression was in line with poorer overall survival of patients with LUSC. We also investigated the biological function of CHMP4C in LUSC cells and validated in an independent cohort that increased CHMP4C expression predicted poor prognosis in patients with LUSC.
Cancer is characterized by abnormal cell cycle control gene activity, leading to uncontrolled cell proliferation (20). The aberrant function of cell cycle regulators can cause that cell proliferation is uncontrolled, making them an attractive therapeutic targets for cancer treatment. In fact, the survival rate of many cancers depends only on these proteins and several specific inhibitors used in the clinic. Though the ubiquitin-proteasome system is in charge of regular quality control of cell cycle proteins during cell cycle progression, increasing evidence clearly indicates that there is a close interaction between cell cycle regulation and selective autophagy, which is an important mechanism for maintaining homeostasis in vivo. Several cell cycle-related inhibitors have shown antitumor activity in cancer studies, such as HM90822B which inhibits IPAs (21), Dinaciclib which inhibits CDK1 activity (22), and PD 0332991 which is an inhibitor of cyclin D kinase 4/6 (23). A preliminary oncogenic role of CHMP4C in the development of LUSC was shown in our study. Depletion of CHMP4C expression was observed to lead to cellular S-phase arrest and inhibition of LUSC cell proliferation. The interaction between CHMP4C and cell cycle-related molecules underlies cell cycle arrest, we found that CHMP4C was associated with CCNE2, REEP3, SKP1, TOMIL1, DCTN2, NSMCE2, ORC4 and PPPICB, which were positively correlated.
Our study creatively integrated samples of LUSC from GEO and TCGA databases to speculate the potential biological function and clinicopathological significance of CHMP4C detected by GSEA in LUSC. CHMP4C was found to be overexpressed in LUSC, and its reduced expression led to abnormal cell cycle transition in LUSC. Therefore, inhibition of CHMP4C may be an effective way to prevent and treat LUSC.
Acknowledgments
Funding: This work was supported by the special fund of basic scientific research expenses for colleges and universities in Heilongjiang province [2019-KYYWF-1219].
Footnote
Reporting Checklist: The authors have completed the TREND reporting checklist. Available at https://dx.doi.org/10.21037/jtd-21-583
Data Sharing Statement: Available at https://dx.doi.org/10.21037/jtd-21-583
Conflicts of Interest: All authors have completed the ICMJE uniform disclosure form (available at https://dx.doi.org/10.21037/jtd-21-583). The authors have no conflicts of interest to declare.
Ethical Statement: The authors are accountable for all aspects of the work in ensuring that questions related to the accuracy or integrity of any part of the work are appropriately investigated and resolved. The study was conducted in accordance with the Declaration of Helsinki (as revised in 2013). The Third Affiliated Hospital of Qiqihar Medical College institutional review board approved the study protocol (2019LL-23) and individual consent for this retrospective analysis was waived.
Open Access Statement: This is an Open Access article distributed in accordance with the Creative Commons Attribution-NonCommercial-NoDerivs 4.0 International License (CC BY-NC-ND 4.0), which permits the non-commercial replication and distribution of the article with the strict proviso that no changes or edits are made and the original work is properly cited (including links to both the formal publication through the relevant DOI and the license). See: https://creativecommons.org/licenses/by-nc-nd/4.0/.
References
- Van Der Weijst L, Lievens Y, Schrauwen W, et al. Health-Related Quality of Life in Advanced Non-small Cell Lung Cancer: A Methodological Appraisal Based on a Systematic Literature Review. Front Oncol 2019;9:715. [Crossref] [PubMed]
- Bray F, Ferlay J, Soerjomataram I, et al. Global cancer statistics 2018: GLOBOCAN estimates of incidence and mortality worldwide for 36 cancers in 185 countries. CA Cancer J Clin 2018;68:394-424. [Crossref] [PubMed]
- Rami-Porta R, Bolejack V, Crowley J, et al. IASLC Staging and Prognostic Factors Committee, Advisory Boards and Participating Institutions. The IASLC Lung Cancer Staging Project: Proposals for the Revisions of the T Descriptors in the Forthcoming Eighth Edition of the TNM Classification for Lung Cancer. J Thorac Oncol 2015;10:990-1003.
- Siegel RL, Miller KD, Jemal A. Cancer statistics, 2019. CA Cancer J Clin 2019;69:7-34. [Crossref] [PubMed]
- Choi HS, Jeong BK, Jeong H, et al. Application of the new 8th TNM staging system for non-small cell lung cancer: treated with curative concurrent chemoradiotherapy. Radiat Oncol 2017;12:122. [Crossref] [PubMed]
- Testa U, Castelli G, Pelosi E. Lung Cancers: Molecular Characterization, Clonal Heterogeneity and Evolution, and Cancer Stem Cells. Cancers (Basel) 2018;10:248. [Crossref] [PubMed]
- Lemjabbar-Alaoui H, Hassan OU, Yang YW, et al. Lung cancer: Biology and treatment options. Biochim Biophys Acta 2015;1856:189-210. [PubMed]
- Wang C, Tan S, Liu WR, et al. RNA-Seq profiling of circular RNA in human lung adenocarcinoma and squamous cell carcinoma. Mol Cancer 2019;18:134. [Crossref] [PubMed]
- Zheng K, He Z, Kitazato K, et al. Selective Autophagy Regulates Cell Cycle in Cancer Therapy. Theranostics 2019;9:104-25. [Crossref] [PubMed]
- Li CW, Chen BS. Investigating core genetic-and-epigenetic cell cycle networks for stemness and carcinogenic mechanisms, and cancer drug design using big database mining and genome-wide next-generation sequencing data. Cell Cycle 2016;15:2593-607. [Crossref] [PubMed]
- VanArsdale T, Boshoff C, Arndt KT, et al. Molecular Pathways: Targeting the Cyclin D-CDK4/6 Axis for Cancer Treatment. Clin Cancer Res 2015;21:2905-10. [Crossref] [PubMed]
- Iness AN, Litovchick L, Muv B. A Key to Cell Cycle Control in Ovarian Cancer. Front Oncol 2018;8:223. [Crossref] [PubMed]
- Thoresen M. Logistic regression – applied and applicable. Tidsskr Nor Laegeforen 2017;137. [PubMed]
- Ambler JM, Mulaudzi S, Mulder N. GenGraph: a python module for the simple generation and manipulation of genome graphs. BMC Bioinformatics 2019;20:519. [Crossref] [PubMed]
- Tsang HT, Connell JW, Brown SE, et al. A systematic analysis of human CHMP protein interactions: additional MIT domain-containing proteins bind to multiple components of the human ESCRT III complex. Genomics 2006;88:333-46. [Crossref] [PubMed]
- Li K, Liu J, Tian M, et al. CHMP4C Disruption Sensitizes the Human Lung Cancer Cells to Irradiation. Int J Mol Sci 2015;17:18. [Crossref] [PubMed]
- Carlton JG, Caballe A, Agromayor M, et al. ESCRT-III governs the Aurora B-mediated abscission checkpoint through CHMP4C. Science 2012;336:220-5. [Crossref] [PubMed]
- Lin SL, Wang M, Cao QQ, et al. Chromatin modified protein 4C (CHMP4C) facilitates the malignant development of cervical cancer cells. FEBS Open Bio 2020;10:1295-303. [Crossref] [PubMed]
- Petsalaki E, Zachos G. CHMP4C: A novel regulator of the mitotic spindle checkpoint. Mol Cell Oncol 2018;5:e1445944 [Crossref] [PubMed]
- Otto T, Sicinski P. Cell cycle proteins as promising targets in cancer therapy. Nat Rev Cancer 2017;17:93-115. [Crossref] [PubMed]
- Lee SH, Lee JY, Jung CL, et al. A novel antagonist to the inhibitors of apoptosis (IAPs) potentiates cell death in EGFR-overexpressing non-small-cell lung cancer cells. Cell Death Dis 2014;5:e1477 [Crossref] [PubMed]
- Danilov AV, Hu S, Orr B, et al. Dinaciclib Induces Anaphase Catastrophe in Lung Cancer Cells via Inhibition of Cyclin-Dependent Kinases 1 and 2. Mol Cancer Ther 2016;15:2758-66. [Crossref] [PubMed]
- Liu M, Xu S, Wang Y, et al. PD 0332991, a selective cyclin D kinase 4/6 inhibitor, sensitizes lung cancer cells to treatment with epidermal growth factor receptor tyrosine kinase inhibitors. Oncotarget 2016;7:84951-64. [Crossref] [PubMed]