Initial rapidity of tumor growth as a prognostic factor for the therapeutic effect of immune-checkpoint inhibitors in patients with non-small cell lung cancer: evaluation for linear and non-linear correlation
Introduction
Lung cancer is the leading cause of cancer-related death, and its prevalence is increasing worldwide. The prevalence of tracheal, bronchus, and lung cancer increased by 28% from 2006 to 2016; 1.7 million patients died in 2016 (1). Lung cancer is frequently detected in an already advanced stage; therefore, most patients have no choice but chemotherapy as the primary therapy. Unfortunately, the prognosis of lung cancer patients with distant metastasis is devastating, and their median overall survival at our hospital was 9.5 months (2).
Docetaxel has hitherto been the standard second-line chemotherapy (3); however, CheckMate-017 and CheckMate-057 revealed that nivolumab, the first-released immune-checkpoint inhibitor (ICI), prolonged the overall survival compared with docetaxel (4,5). Afterward, pembrolizumab was approved as the first-line therapy against non-small cell lung cancer (NSCLC) with programmed death-ligand 1 (PD-L1) expression of 50% or higher (6). However, at present, ICIs in combination with cytotoxic chemotherapies have been approved as the first-line treatment for lung cancer regardless of PD-L1 expression (7,8).
Clinically useful predictors of ICI treatment efficacy are needed. Other biomarkers besides PD-L1 expression rate have also been proposed as candidates. Tumor mutation burden detected by next-generation sequencing has been reported to correlate with ICI treatment efficacy (9); however, its application is clinically limited, and a standard value remains to be set. Microsatellite instability is the first cross-organ biomarker approved by the U.S. Food and Drug Administration (10); however, its clinical efficacy has not been proven for NSCLC. The effectiveness of peripheral blood CD62Llow CD4+T-cells as indicators of therapeutic response to ICIs is examined experimentally (11). At present, more convenient predictors are still necessary. The baseline tumor diameter of more than 101 mm has been shown to predict poor prognosis (12). We anticipated that tumor growth rate, which represents a change of tumor diameter over a period of time, might relate to prognosis with ICI treatment.
In this study, to evaluate the tumor growth rate during the pre-treatment period as a predictor of ICIs efficacy, we scatterplotted initial rapidity of the tumor growth as a function of time to treatment failure (TTF) and then calculated non-linear and linear coefficients. We adopted the maximal information coefficient (MIC), which is a novel non-linear correlation coefficient (13), and the conventional Spearman’s rank correlation coefficient as estimators of linear correlation.
We present the following article in accordance with the STROBE reporting checklist (available at https://dx.doi.org/10.21037/jtd-21-774).
Methods
Patients
All NSCLC patients, who had undergone nivolumab, pembrolizumab, or atezolizumab therapy at Saitama Medical Center, Saitama Medical University from January 1, 2016 to December 31, 2018 were retrospectively enrolled. For measurement of the tumor growth rate during the pre-treatment period and the best response to ICIs, chest computed tomography (CT) needs to be performed at least twice before the start of ICI treatment, and at least once after the treatment. Patients who did not undergo these three chest CT examinations were excluded. Follow-up was continued until December 31, 2020.
Procedures
Clinical information was retrospectively collected from electronic medical records at Saitama Medical Center. We defined a tumor size as the sum of the diameters of the intrathoracic tumors and the lymph nodes on chest CT images, and an initial tumor size (ITS) was obtained at the first checkup in a previous hospital or clinic, or at Saitama Medical Center. We also defined the initial rapidity of tumor progression (IRP) as the calculated increase in the summed diameter from the time of the first CT scan (initial checkup) to the time of the second CT scan (just before initiation of treatment) divided by the number of days between the two scans. After intrathoracic tumor lesions were detected, lung lesions of 10 mm or more in a major axis and lymph nodes of 15 mm or more in a minor axis were defined as measurable lesions, and the top two largest lesions each in lung lesions and lymph nodes were measured for IRP calculation. For response evaluation, up to 5 lesions including other organ lesions were measured. A specialized physician in respiratory diseases and a data management assistant measured lesions according to the RECIST guideline version 1.1. TTF was defined as an interval from ICI treatment initiation to its withdrawal by an attending physician. We also accumulated their neutrophil counts, lymphocyte counts, the value of C-reactive protein (CRP), and the value of albumin on their first visit, and calculated the initial value of the neutrophil-lymphocyte ratio (NLR) and the CRP-albumin ratio. We examined the correlation of ITS, IRP, the initial NLR and the initial CRP-albumin ratio with TTF to verify the tumor growth rate as a predictor of ICI benefits.
Statistics
TTF was estimated by the Kaplan-Meier method, and the differences between groups were evaluated by the log-rank test using the EZR software program (Saitama Medical Center, Jichi Medical University, Saitama, Japan) (14), with the level of significance set at P<0.05. A Normal distribution of patients’ parameters was verified by the Shapiro-Wilk test, and scatter plots were drawn and analyzed to determine correlation. Three or more groups were compared with a non-parametric multiple test, the Kruskal-Wallis test. Spearman’s rank correlation coefficient and MIC were adopted as tools for use in distribution analysis on scatter plots with EZR. MIC was calculated using the tools implemented in Python 3.6.5 with the imported minepy library. Spearman’s rank correlation coefficient with P<0.05 was considered statistically significant, and the absolute value of their coefficients was interpreted as the follows: 0–0.3, no correlation; 0.3–0.5, weak correlation; 0.5–0.7, moderate correlation; 0.7–0.9, high correlation; and 0.9–1.0, very high correlation. MIC was evaluated according to interpretation for Spearman’s rank correlation coefficients.
Ethical statement
The study was conducted in accordance with the Declaration of Helsinki (as revised in 2013). The study was approved by the ethics board of Saitama Medical Center, Saitama Medical University (application number: 2179) and individual consent for this retrospective analysis was waived.
Results
A total of 73 patients underwent ICI treatment at Saitama Medical Center; however, 18 patients were excluded from this study because of insufficient chest CT information in 17 patients and a different diagnosis of mesothelioma in 1 patient (Figure 1A).
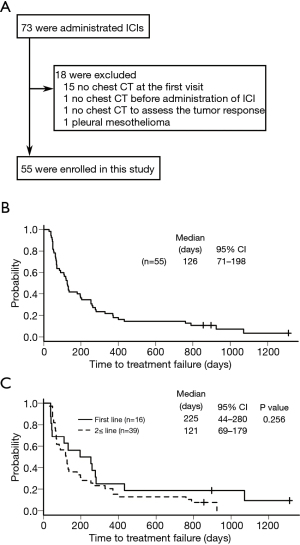
A total of 55 patients were included [47 men and 8 women, 44–88 years old (median age, 70 years old)]; 29 had pembrolizumab, 16 first-line treatment, and 13 second- or more-line treatment; 22 had nivolumab, and 4 had atezolizumab for second- or more-line treatment (Table 1). The best confirmed response to ICIs was: complete response (CR), 5 (9.1%); partial response (PR), 14 (25.5%); stable disease (SD), 27 (49.1%); and progressive disease (PD), 9 (16.4%).
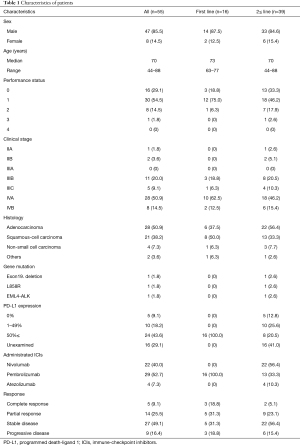
Full table
While four patients continued ICI treatments over the follow-up period, 51 patients were withdrawn from treatment due to the following: tumor progression, 34; pneumonia, 9; hypothyroidism, 2; liver function disorder, 1; hypophysitis, 1; peripheral neuropathy, 1; and skin rash, 1 (Table 2).
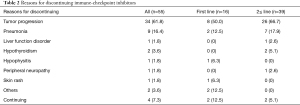
Full table
The median TTF of ICI treatment for all 55 patients was 126 days (range, 28–1,311 days) (Figure 1B). TTF for 16 patients with the first-line ICI was not significantly different from that for 39 patients with the second- or more-line ICI (P=0.256), while the former tended to be longer than the latter, with the median TTF, 225 days (38–1,311 days) and 121 days (28–925 days), respectively (Figure 1C).
The median interval between the first CT scan at the initial checkup and the second CT scan just before treatment was 44 days (range, 5–1,573 days), and the median IRP during the period was 0.14 mm/days (0.02–2.09 mm/days). Twenty-five patients had extrathoracic metastases before the first treatment, and the median sum of diameters in each metastatic site was the following: the brain (n=10), 18.75 mm (range, 3.7–38.4 mm); the bone (n=12), 26.15 mm (3.5–86.0 mm); the liver (n=2), 20.2 and 25.1 mm; the adrenal gland (n=4), 20.15 mm (17.4–39.4 mm); the extrathoracic lymph node (n=2), 10.2 mm and 34.3 mm; and the muscle (n=1), 8.4 mm. A patient with a 38.4-mm nodule in the cerebellum underwent tumor resection before ICI administration, and recurrence did not occur in the brain during the second-line pembrolizumab treatment. A patient with an 86-mm bulky mass in the ilium underwent radiation therapy, which apparently shrunk the bone mass, leading to osteosclerosis without fluorodeoxyglucose accumulation. While the patient received nivolumab treatment, recurrence did not occur in the bone.
We assumed that the correlation of IRP or ITS with TTF might not be linear; therefore, we examined the correlation of IRP and TTF using MICs, non-linear correlation coefficients reported for the first time by Reshef et al. in 2011 (13), and Spearman’s rank correlation coefficients (Table 3). In a linear function set, MIC is 1 and is the same as Pearson’s correlation coefficient; in a quadratic function, Pearson’s correlation coefficient was 0.499, and MIC was 1; and, in the inverse proportion correlation, Pearson’s correlation coefficient was −0.648, and MIC was 1, indicating that MIC is a measure of the strength of non-linear correlation (Figure 2A). Since the Gompertzian model originally showed that the tumor growth rate slows as the tumor size increases (15), IRP was thought to be negatively correlated with ITS. However, Spearman’s rank correlation coefficient between them was 0.393 (P=0.00296) indicating a weakly positive correlation (Figure 2B). The correlation of IRP to TTF was weak with MIC, 0.302, and negative linear, −0.347 (P=0.00938), with Spearman’s rank correlation coefficient (Figure 2C). On the other hand, ITS and TTF were not correlated using MIC, 0.259, and Spearman’s rank correlation coefficient, −0.228 (P=0.09430) (Figure 2D).
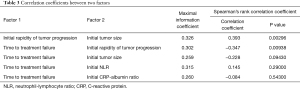
Full table
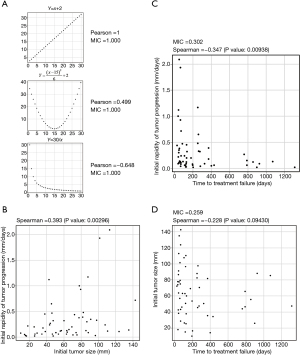
IRP was evaluated according to histology of lung cancer or PD-L1 expression. The median IRP in each histology of lung cancer is the following: 0.125 mm/days (range 0.02–0.93) in adenocarcinoma, 0.115 mm/days (0.04–1.17) in NSCLC, which was impossible to be determined as detailed types, 0.270 mm/days (0.03–2.09) in squamous cell carcinoma, and 0.15 mm/days and 0.09 mm/days in the other two types (P=0.393) (Figure 3A); therefore, IRP was not associated with histology of lung cancer. IRP and PD-L1 expression were also not correlated in 39 patients, in which PD-L1 expression had been measured; MIC, 0.234; and Spearman’s rank correlation coefficient, 0.111 (P=0.502) (Figure 3B).
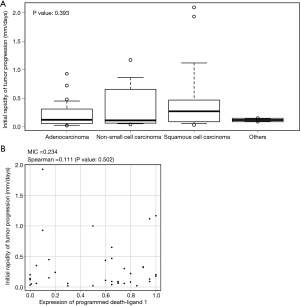
The relationship between IRP and the response to treatment after 3, 6, and 9 months after ICI administration was evaluated (Figure 4). The median IRP in each response to treatment were the following: CR, (n=3), 0.18 mm/days (range 0.02–0.20 mm/days); PR (n=16), 0.13 mm/days (0.03–1.17 mm/days); SD (n=18), 0.085 mm/days (0.02–0.45 mm/days); and discontinuation of ICI (n=15), 0.3 mm/days (0.09–2.09 mm/days) at three months after ICI administration (P=0.0217); CR (n=2), 0.11 mm/days (0.04–0.18 mm/days), PR (n=9), 0.32 mm/days (0.02–1.17 mm/days); SD (n=9), 0.08 mm/days (0.02–0.45 mm/days); PD (n=3), 0.09 mm/days (0.02–0.20 mm/days); and discontinuation of ICI (n=31), 0.22 mm/days (0.03–2.09 mm/days) at six months (P=0.24); and CR (n=3), 0.04 mm/days (0.02–0.18 mm/days); PR (n=5), 0.13 mm/days (0.05–0.39 mm/days); SD (n=5), 0.06 mm/days (0.02–0.12 mm/days); PD (n=4), 0.565 mm/days (0.33–1.17 mm/days); and discontinuation of ICI (n=36), 0.2 mm/days (0.02–2.09 mm/days) after nine months (P=0.00834). Due to lack of appropriate examination, 3, 1, and 2 patients were excluded from the evaluation at 3, 6, and 9 months, respectively.
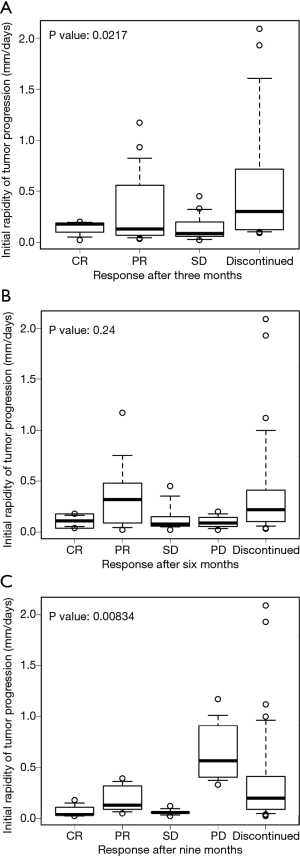
In addition, the initial value of NLR or the CRP-albumin ratio, which has already been reported as a prognostic factor of treatment with ICIs (16,17), was also examined. Although NLR has previously been reported as a negative predictor of ICI therapy, the initial NLR seemed to be positively correlated with TTF on the scatter plot, with MIC, 0.315; however, NLR did not linearly correlate with TTF using Spearman’s rank correlation coefficient, 0.145 (P=0.29000) (Figure 5A). The initial value of CRP-albumin ratio was not correlated with TTF using MIC, 0.260, and Spearman’s rank correlation coefficient, −0.084 (P=0.54300) (Figure 5B).
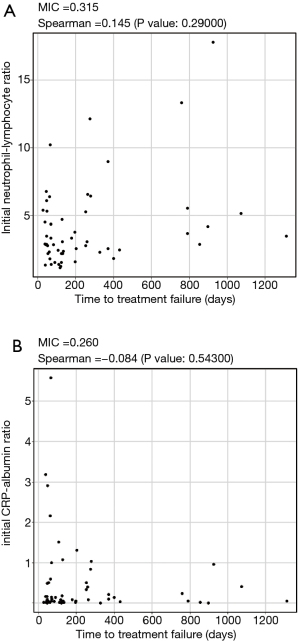
Excluding patients with discontinuation of ICIs due to adverse events or ongoing ICI treatment, the correlation between TTF and IRP, the initial NLR or the initial CRP-albumin ratio was not significant in only patients with discontinuation due to tumor progression (Figure 6). Because some patients with discontinuation of ICIs due to adverse events have longer TTF, excluding these patients in addition to patients with ongoing ICI treatment might omit patients who were able to have benefit by ICI treatment for TTF.
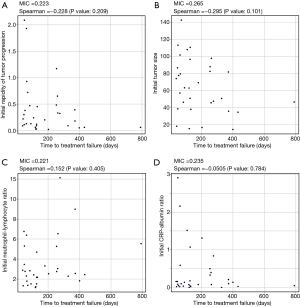
Discussion
In this research, we evaluated the tumor growth rate, IRP, as a prognostic factor of ICI treatment, comparing it with other previously reported prognostic factors: ITS, initial NLR, and the initial value of CRP-albumin ratio. Because some of these factors might have non-linear correlations with prognosis, we needed to use scatter plots rather than survival curves for estimation; however, at present, there is no standard non-linear correlation coefficient. The correlation of each factor with TTF was evaluated by drawing scatter plots, and MIC, a non-parametric statistic, followed by Spearman’s rank correlation coefficient was calculated. We adopted MIC, as described in the language Python or R, because it has been developed to show the distribution of non-linearly related variables on a scatter plot. Although, at present, no commonly used methods for calculating the correlation of clinical factors have been approved for non-linear distributions, MIC has successfully been applied to genome-wide association studies as a non-parametric statistic (18). In this investigation, MIC showed that there were correlations of the initial NLR, which is an already reported prognostic factor, and IRP with TTF, before we examined whether the correlations were linear.
The scatter plots showed NLR and TTF were positively correlated in ICIs-treated patients, although NLR has recently been reported to be negatively correlated with the effect of ICIs (16). This controversial result is attributed to the lack of accepted measures for evaluating each prognostic predictor of ICIs treatment. Katsurada et al. reported the ITS as a useful predictor (12), but tumor size and the rate of tumor growth are not necessarily linearly correlated, which indicates that time-sequential observation of tumor progression before ICI treatment might be useful. When we examined the rate of tumor growth before ICI treatment, and evaluated its correlation with TTF, MIC proved to be weak; furthermore, Spearman’s rank correlation coefficient supported this correlation more than the correlation with tumor size. In addition, even though previously-reported prognostic factors did not regress linearly on TTF, our study suggested that only the rate of tumor growth had negatively correlated with TTF in a linear manner.
From our results, ICI treatment of acutely progressive tumors is less beneficial; however, our investigation has the following limitations. First, this investigation was a small-scale retrospective study at a designated regional cancer treatment hospital in Japan; therefore, we will set up an independent cohort to validate the results. Secondly, because 4 of 55 patients continued ICI therapy after the end of the follow-up period, the TTF of these patients might blur the results. Since ICIs could give extremely long survival, excluding these patients would result in omitting long-term survivors treated with ICI; therefore, we included their data up to the end of the follow-up in the scatter plot. Thirdly, tumor size and response to ICIs were measured on two-dimensional CT scans according to the RECIST guideline. Three-dimensional measurement may improve the accuracy of the results. Fourthly, 40% of patients received nivolumab; therefore, our results may be affected by the first-released ICI.
The predictors of clinical efficacy of ICIs are yet to be determined owing to the lack of consistent results and differences in procedures between different researchers. Our investigation revealed the correlation between the rate of tumor growth and the therapeutic effect of ICIs using a novel non-parametric test, MIC, and Spearman’s rank correlation coefficient. The MIC score presented a weak correlation between the rate of tumor growth and TTF, and they regressed linearly. This result suggests that the effect of ICIs is less beneficial in tumors with an acutely progressive feature in their inherent properties.
Acknowledgments
Funding: None.
Footnote
Reporting Checklist: The authors have completed the STROBE reporting checklist. Available at https://dx.doi.org/10.21037/jtd-21-774
Data Sharing Statement: Available at https://dx.doi.org/10.21037/jtd-21-774
Peer Review File: Available at https://dx.doi.org/10.21037/jtd-21-774
Conflicts of Interest: All authors have completed the ICMJE uniform disclosure form (available at https://dx.doi.org/10.21037/jtd-21-774). Akihiko Gemma reports receiving honoraria from Bristol-Myers Squibb K.K, Chugai Pharmaceutical Co. Ltd., Daiichi Sankyo Co. Ltd., Kyorin Pharmaceutical Co., Ltd., AstraZeneka K.K., Ono Pharmaceutical Co. Ltd., Eisai Co. Ltd., Nippon Boehringer Ingelheim Co. Ltd., Pfizer Japan Inc. and TAIHO Pharmaceutical Co. Ltd. The other authors have no conflicts of interest to declare.
Ethical Statement: The authors are accountable for all aspects of the work in ensuring that questions related to the accuracy or integrity of any part of the work are appropriately investigated and resolved. The study was conducted in accordance with the Declaration of Helsinki (as revised in 2013). The study was approved by the ethics board of Saitama Medical Center, Saitama Medical University (application number: 2179) and individual consent for this retrospective analysis was waived.
Open Access Statement: This is an Open Access article distributed in accordance with the Creative Commons Attribution-NonCommercial-NoDerivs 4.0 International License (CC BY-NC-ND 4.0), which permits the non-commercial replication and distribution of the article with the strict proviso that no changes or edits are made and the original work is properly cited (including links to both the formal publication through the relevant DOI and the license). See: https://creativecommons.org/licenses/by-nc-nd/4.0/.
References
- Fitzmaurice C, Akinyemiju TF, Al Lami FH, et al. Global, regional, and national cancer incidence, mortality, years of life lost, years lived with disability, and disability-adjusted life-years for 29 cancer groups, 1990 to 2016: A systematic analysis for the global burden of disease study. JAMA Oncol 2018;4:1553-68. [Crossref] [PubMed]
- Sakai K, Kuramoto J, Kojima A, et al. Validation of prognostic impact of number of extrathoracic metastases according to the eighth TNM classification: A single-institution retrospective study in japan. Int J Clin Oncol 2019;24:1549-57. [Crossref] [PubMed]
- Shepherd FA, Dancey J, Ramlau R, et al. Prospective randomized trial of docetaxel versus best supportive care in patients with non-small-cell lung cancer previously treated with platinum-based chemotherapy. J Clin Oncol 2000;18:2095-103. [Crossref] [PubMed]
- Brahmer J, Reckamp KL, Baas P, et al. Nivolumab versus docetaxel in advanced squamous-cell non-small-cell lung cancer. N Engl J Med 2015;373:123-35. [Crossref] [PubMed]
- Borghaei H, Paz-Ares L, Horn L, et al. Nivolumab versus docetaxel in advanced nonsquamous non-small-cell lung cancer. N Engl J Med 2015;373:1627-39. [Crossref] [PubMed]
- Reck M, Rodriguez-Abreu D, Robinson AG, et al. Pembrolizumab versus chemotherapy for PD-L1-positive non-small-cell lung cancer. N Engl J Med 2016;375:1823-33. [Crossref] [PubMed]
- Gandhi L, Rodríguez-Abreu D, Gadgeel S, et al. Pembrolizumab plus chemotherapy in metastatic non-small-cell lung cancer. N Engl J Med 2018;378:2078-92. [Crossref] [PubMed]
- Paz-Ares L, Luft A, Vicente D, et al. Pembrolizumab plus chemotherapy for squamous non-small-cell lung cancer. N Engl J Med 2018;379:2040-51. [Crossref] [PubMed]
- Rizvi H, Sanchez-Vega F, La K, et al. Molecular determinants of response to anti-programmed cell death (PD)-1 and anti-programmed death-ligand 1 (PD-L1) blockade in patients with non-small-cell lung cancer profiled with targeted next-generation sequencing. J Clin Oncol 2018;36:633-41. [Crossref] [PubMed]
- Chang L, Chang M, Chang HM, et al. Microsatellite instability: A predictive biomarker for cancer immunotherapy. Appl Immunohistochem Mol Morphol 2018;26:e15-21. [Crossref] [PubMed]
- Kagamu H, Kitano S, Yamaguchi O, et al. CD4(+) T-cell immunity in the peripheral blood correlates with response to anti-PD-1 therapy. Cancer Immunol Res 2020;8:334-44. [Crossref] [PubMed]
- Katsurada M, Nagano T, Tachihara M, et al. Baseline tumor size as a predictive and prognostic factor of immune checkpoint inhibitor therapy for non-small cell lung cancer. Anticancer Res 2019;39:815-25. [Crossref] [PubMed]
- Reshef DN, Reshef YA, Finucane HK, et al. Detecting novel associations in large data sets. Science 2011;334:1518-24. [Crossref] [PubMed]
- Kanda Y. Investigation of the freely available easy-to-use software 'EZR' for medical statistics. Bone Marrow Transplant 2013;48:452-8. [Crossref] [PubMed]
- Norton L. A Gompertzian model of human breast cancer growth. Cancer Res 1988;48:7067-71. [PubMed]
- Bagley SJ, Kothari S, Aggarwal C, et al. Pretreatment neutrophil-to-lymphocyte ratio as a marker of outcomes in nivolumab-treated patients with advanced non-small-cell lung cancer. Lung Cancer 2017;106:1-7. [Crossref] [PubMed]
- Inoue T, Tamiya M, Tamiya A, et al. Analysis of early death in Japanese patients with advanced non-small-cell lung cancer treated with nivolumab. Clin Lung Cancer 2018;19:e171-6. [Crossref] [PubMed]
- Yang D, Liu H. Maximal information coefficient applied to differentially expressed genes identification: A feasibility study. Technol Health Care 2019;27:249-62. [Crossref] [PubMed]