Artificial intelligence in thoracic surgery: a narrative review
Introduction
Artificial intelligence (AI) can be defined as the science of computer algorithms able to perform tasks that imitate human cognitive functions and intelligence (1). AI-based technologies have recently experienced a widespread explosion across many disciplines, and their implementation in healthcare settings is accordingly growing (2).
The applications of AI systems in medicine seem currently endless, ranging from diagnosis generation and risk prediction, to therapy selection and outcome evaluation (3,4). The aim of these techniques is to extract relevant information from massive healthcare data and to assist clinical decision-making, thus reducing medical errors, and enhancing the quality and efficiency of care (5).
AI devices mainly fall into two major categories, natural language processing (NLP) methods and machine learning (ML) techniques, even if some overlapped features are common to both technologies (3). NLP methods can obtain useful information from unstructured data, such as clinical reports, operative notes, and discharge summaries, turning narrative texts into data that can be processed by computer programs (6). ML techniques construct analytical algorithms to iteratively analyze titanic amount of structured information, such as imaging and genetic data; they can extract meaningful patterns and create prediction models about input variables (7,8) (Figure 1). There are three subtypes of ML that have been applied to medicine: supervised learning, unsupervised learning, and reinforcement learning (RL) (Table 1).
Table 1
Algorithm | Definition |
---|---|
Supervised learning | Training a model from input variables and their corresponding labels, using a dataset that has to be labeled by humans |
Regression models | Simple models, can provide great insight into linear relationships |
Support vector machine | Fast and flexible, its goal is to find an optimal decision boundary between 2 or more classes that put the maximum margin between the 2 groups |
Random forest | A collection of short height data structures called random decision trees, that uses different combination of explanatory variables to predict the outcome of interest |
Naïve bayes | Family of simple “probabilistic classifiers” based on applying Bayes’ theorem with strong (naïve) independence assumptions between the features |
Deep learning | Neural network with many hidden layers, able to handle complex data with various structures to create a prediction. The commonly used deep learning algorithms in medicine include convolution neural network, recurrent neural network, deep belief network and deep neural network |
Unsupervised learning | Training a model to find hidden patterns in an unlabeled dataset. Principle component analysis and cluster analysis are the main methods used in healthcare |
Reinforcement learning | Group of algorithms that iteratively try different series of actions until the system is able to appropriately perform a reward function |
ML, machine learning.
A supervised ML algorithm uses a dataset that has to be labeled by humans, building a relationship between the input variables and the outcomes of interest, when the outcomes are known. Due to the proficiency to provide the best results, supervised techniques such as linear regression, logistic regression, Support Vector Machine (SVM), random forest, naïve Bayes, decision tree, and neural network (3,9), are frequently used in healthcare applications. Although considered a subcategory of supervised learning, deep learning should be mentioned as an independent part, due to its wide application in healthcare setting. This system is made of a series of inputs which go through multiple interconnected layers of neurons (neural networks), that recognize different features independently, and makes predictions on a large quantity of information, finally providing an output. The use of deep learning techniques in medicine have been especially effective if applied to images detection and classification.
In unsupervised ML algorithm, conversely, the only inputs are raw features, and the outcomes are unknown, therefore the method can be used to find hidden patterns in data without human feedback. Principle Component Analysis (PCA) and cluster analysis are the main methods of unsupervised learning successfully used in healthcare to discover new phenotypes of a multifactorial disease (10).
Finally, RL techniques are a family of algorithms that maximize return and are the core technique at the heart of robotic surgery. RL algorithms iteratively try different series of actions until a system can achieve an appropriate performance. They can be used to train a surgical robot to perform a series of actions promoting positive actions and discouraging negative ones, by means of a reward function (11).
The creation and application of a ML method depends on four crucial steps: the collection and preparation of data, the choice and training of the algorithm according to the objective to pursue, the implementation of the software, and the analysis and validation of the system for its proper use (Figure 2).

Despite the promising results of AI implementation in thoracic surgery, its widespread diffusion is far to be a common practice. The aim of this article is to review the current applications of AI to thoracic surgery, exploring the influence of AI-based technologies on each step of the clinical pathway, from diagnosis to the Operating Room (OR), including the legal and ethical aspects related to AI in healthcare (Figure 3).
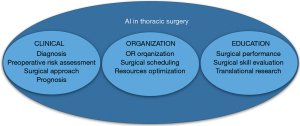
We present the following article in accordance with the Narrative Review reporting checklist (available at: https://dx.doi.org/10.21037/jtd-21-761).
Methods
We performed a narrative review of the literature on Scopus, PubMed and Cochrane databases. All the relevant studies published in the last ten years, until March 2021 were included. The research string comprised various combinations of “artificial intelligence”, “machine learning”, “lung cancer”, “esophageal cancer”, “pathology”, “risk assessment”, “thoracic surgery”, “robotics”, “deep learning”. Papers concerning children and animals, studies published after March 2021 or written in languages other than English were excluded. Articles of interest that had been cited by the articles identified in the initial search were also included (Figure 4).
Thoracic lesions management
Due to the weight of early detection of lung malignancies on the survival rates, pulmonary nodule management has been one of the main fields to be influenced by the implementation of AI-based technologies. In fact, the substantial variability reported among radiologists in the detection of lung nodules and the high false-positive rate in screening programs (12), bared the need of tools assisting the radiologist in nodule identification, measurement, risk-stratification, and monitoring.
Various Computer-Aided Diagnosis (CAD) techniques have been proposed in pulmonary nodule assessment with CT and chest radiography since last decades (13,14). Although CAD has revealed to improve detection and efficiency, its acceptance in routine clinical practice is prevented from the high number of false positives (15).
More recently, due to their ability to increase diagnostic accuracy (16,17), the introduction of deep learning techniques gained sparkling attention. The turning point was the publication of the paper titled “ImageNet Classification with Deep Convolutional Networks” by Krizhevsky et al. (18), who first used a multilayered convolutional computational model known as Convolutional Neural Network (CNN) to identify and classify more than one million of images to a level of accuracy never seen before. Even if the use of CNNs in this paper was not in a radiological setting, the manuscript by Krizhevsky et al. paved the way for a wide application of the technique. In fact, CNN is a form of neural networks that uses convolution filters to extract features from images (18) and seem able to detect patterns beyond human perception. In comparison to CAD technique, the innovation of CNNs is due to its capacity to learn from verified data, and to self-determine previously unknown features, thus maximizing classification with limited direct supervision (16). Thus, this architecture of feature extraction by convolutional layers proved to be appliable to image classification and segmentation (19-21). Since 2012, CNNs have proven to be a valid tool in radiologist’ hands in assisting pulmonary nodule detection, confirming its superior efficacy compared to both human perception and standard CAD techniques (22-25). Specifically, they show a reduction in the false-positive rate, therefore potentially enabling to prevent unnecessary follow-up (26,27). Moreover, recently, deep learning reported significative results in nodule segmentation and characterization, while only few preliminary studies are currently available about the application of these techniques on monitoring and follow-up of pulmonary lesions (28). Despite the high sensitivity of CNNs in detecting and discriminating pulmonary lesions, some limits have still to be overcome. The main advantage of CNNs is the ability to self-learn previously unknown features, but the learning capacity requires a huge amount of high-quality data; moreover, a substantial number of studies has been conducted on the same large database; so, to validate these techniques for routine clinical use, further work is needed (16,27).
Among other supervised ML algorithms, SVM, random forest, and decision tree are the most widely used in the field of diagnosis of thoracic disease (29). SVM has proven to be successful in enhancing the efficiency of diagnosis (30,31), while random forest has been able to correctly classify non-small cell lung cancer (NSCLC) (32) (Table 2).
Table 2
Author | Objective | Algorithm | Application | Main results |
---|---|---|---|---|
Nam JG, et al. | To develop and validate a DLAD for malignant pulmonary nodules on chest radiographs and to compare its performance with physicians including thoracic radiologists | Deep learning-based automatic detection algorithm | Outperformance of radiograph classification and nodule detection for malignant pulmonary nodules on chest radiographs | Radiograph classification performances of DLAD were a range of 0.92–0.99 (AUROC) and 0.831–0.924 (JAFROC FOM), respectively |
Li W, et al. | To design a deep convolutional neural networks method for nodule classification, with the advantage of autolearning representation and strong generalization ability | Deep convolutional neural networks | Pulmonary nodule recognition and classification | Results demonstrate the effectiveness of the proposed method in terms of sensitivity and overall accuracy and that it consistently outperforms the competing methods |
Nibali A, et al. | To improve the ability of CAD systems to predict the malignancy of nodules from cropped CT images of lung nodules | Deep residual networks | Pulmonary nodule malignancy classification | The system achieves the highest performance in terms of all metrics measured including sensitivity, specificity, precision, AUROC, and accuracy |
Eppenhof KAJ, et al. | To develop a deformable registration method based on a 3-D convolutional neural network, together with a framework for training such a network | Convolutional neural networks | Pulmonary CT registration | This approach results in an accurate and very fast deformable registration method, without a requirement for parameterization at test time or manually annotated data for training |
da Silva GLF, et al. | To proposes a methodology to reduce the number of false positives using a deep learning technique in conjunction with an evolutionary technique | Convolutional neural networks | Lung nodule false positive reduction on CT images | The methodology was tested on CT scans with the highest accuracy of 97.62%, sensitivity of 92.20%, specificity of 98.64%, and AUROC curve of 0.955 |
Naqi SM, et al. | To develop a multistage segmentation model to accurately extract nodules from lung CT images | Support vector machine | Lung nodule segmentation method | The classification is performed over GTFD feature vector, and the results show 99% accuracy, 98.6% sensitivity and 98.2% specificity with 3.4 false positives per scan |
Choi W, et al. | To develop a radiomics prediction model to improve pulmonary nodule classification in low-dose CT, and to compare the model with the Lung-RADS for early detection of lung cancer | Support vector machine | Improvement of pulmonary nodule classification in low‐dose CT | The model achieved an accuracy of 84.6%, which was 12.4% higher than Lung-RADS |
Bashir U, et al. | To compare the performance of random forest algorithms utilizing CT radiomics and/or semantic features in classifying NSCLC | Random forest | Non-invasive classification of non-small cell lung cancer | Non-invasive classification of NSCLC can be done accurately using random forest classification models based on well-known CT-derived descriptive features |
DLAD, deep learning-based automatic detection algorithm; CAD, computer-aided diagnosis; CT, computed tomography; AUROC, area under the receiver operating characteristic; GTFD, Geometric texture features descriptor; Lung-RADS, Lung CT Screening Reporting and Data System of the American College of Radiology; NSCLC, non-small cell lung cancer.
Current literature about AI application on the management of thoracic disease other than pulmonary nodules is to date limited. AI based technologies have been mainly applied in the early and accurate detection of esophageal malignant lesion (33-35), and in the classification of thymic epithelial tumours (36).
Preoperative evaluation and risk- assessment
In last decades, AI gained attention in the field of preoperative risk assessment, resulting in many ML algorithms to predict the risk of major complications and mortality after surgery (37,38).
Due to the high morbidity rates, it is of utmost importance to properly evaluate candidates for a thoracic surgical procedure, to assess their individual risk and prognosis (39). In this regard, AI-based technologies have shown promising results, providing an effective aid in the decision-making process, and in the achievement of overall integrated risk scores (40-45) (Table 3). Particularly, in 2002, Esteva et al. used four different probabilistic artificial neural network models to estimate post-operative prognosis after lung resection (41). Shortly after, Santos-García et al., similarly, evaluated the prediction of cardio-respiratory morbidity after pulmonary resection for NSCLC (42).
Table 3
Author | Objective | AI algorithm | Application | Main results |
---|---|---|---|---|
Esteva H, et al. | Assessment of surgical risk in patients undergoing pulmonary resection | Neural network | Prediction of postoperative outcomes in lung resections | NN can integrate results from multiple data predicting the individual outcome for patients, rather than assigning them to less-precise risk group categories |
Santos-Garcia G, et al. | To propose an ensemble model of ANNs to predict cardio-respiratory morbidity after pulmonary resection for NSCLC | Artificial neural network | Prediction of cardio-respiratory morbidity after pulmonary resection for NSCLC | In this series an ANN ensemble offered a high performance to predict postoperative cardio-respiratory morbidity |
Bolourani S, et al. | To identify risk factors for respiratory failure after pulmonary lobectomy | Random forest | Predicting of respiratory failure after pulmonary lobectomy | Two ML-based prediction models were generated and optimized. The first model, with high accuracy and specificity, is suited for performance evaluation, and the second model, with high sensitivity, is suited for clinical decision making |
Salati M, et al. | To verify if the application of an AI analysis could develop a model able to predict cardiopulmonary complications in patients submitted to lung resection | Extreme gradient boosting | Prediction of cardiopulmonary complications after lung resection | XGBOOST algorithm generated a model able to predict complications with an area under the curve of 0.75 |
Chang YJ, et al. | To construct a prediction model with seven supervised ML algorithms to predict whether patients could be weaned immediately after lung resection surgery | Multiple ML algorithms | Prediction of staged weaning from ventilator after lung resection surgery | The AI model with Naïve Bayes Classifier algorithm had the best testing result and was therefore used to develop an application to evaluate risk based on patients’ previous medical data, to assist anesthesiologists, and to predict patient outcomes in pre-anesthetic clinics |
ML, machine learning; NN, neural networks; ANNs, artificial neural networks; NSCLC, non-small cell lung cancer; AI, artificial intelligence.
More recently, Bolourani et al. identified risk factors for respiratory failure after lobectomy and introduced two machine learning-based techniques to predict respiratory failure for quality review and clinical decision-making settings (43). Encouraging results were obtained by Salati et al. who, by means of an innovative ML approach called XGBOOST, developed a model able to define the risk of cardiac and pulmonary complications in the early postoperative period for patients submitted to anatomic lung resection (44). Lastly, an AI prediction model with seven supervised ML algorithms was constructed to predict whether patients could be weaned immediately after lung resection surgery or if they could need a staged weaning with transfer to the intensive care unit (45).
Overall, ML algorithms proved to be effective in a tailored optimization of risk definition, increasing the efficacy of the pre-anesthetic evaluation, suggesting the proper therapeutic planning, and improving the communication with patients and family members.
Surgical performance and planning
To date, implementation of AI-based technologies in the OR is limited. Still, they have a promising future in increasing surgical precision and safety, supporting intraoperative decision-making, and predicting postoperative outcomes (46,47).
In the last two decades, surgical robotics has experienced a relentless growth. Minimally invasive surgery has been shown to decrease length of hospital stay and post-operative complications and is currently considered as a core issue of enhanced recovery programs.
However, even if it is commonly associated with AI, robotic-assisted surgery should not be considered an AI-based technology, and it requires full supervision by human surgeon. The range of robotically assisted thoracic procedures is widening and includes lobectomies, resection of mediastinal malignancy, and esophagectomies. The Da Vinci Robotic Surgical System (Intuitive Surgical Inc. Sunnyvale, CA, USA) is the most used platform. Robotic tele-manipulators provide three-dimensional (3D) and magnified visualization, and are equipped with flexible effector instruments, that have a wide freedom of motion, thus enhancing surgeon’s dexterity during the procedure (48). Nevertheless, the lack of tactile feedback might impair surgical outcomes, leading to suture breakage or failure. Dai et al. evaluated a biaxial haptic feedback system that effectively warns the user when the tension is approaching the suture’s failure point (49). Similarly, in 2016, Shademan et al. proved the feasibility of autonomous surgery, evaluating a supervised system compared to robotic, laparoscopic, and manual approach, when performing an intestinal anastomosis (50). Furthermore, ML models were used to develop and enhance contactless interfaces with gesture recognition, decreasing the risk of contamination during surgical procedures (51).
Also, the application of AI could prompt the progression of precision surgery and surgical training. ML algorithms have been proposed to accurately assess surgical skills, therefore providing a feedback during learning curves and periodic evaluations (52-54) (Table 4).
Table 4
Author | Objective | AI algorithm | Application | Main results |
---|---|---|---|---|
Dai Y, et al. | To develop and validate a novel grasper-integrated system with biaxial shear sensing and haptic feedback to warn the operator prior to anticipated suture breakage | Biaxial haptic feedback system | Improvement of outcomes related to knot tying tasks in robotic surgery | This system may improve outcomes related to knot tying tasks in robotic surgery and reduce instances of suture failure while not degrading the quality of knots produced |
Shademan A, et al. | To demonstrate in vivo supervised autonomous soft tissue surgery in an open surgical setting, enabled by a near-infrared fluorescent imaging system and an autonomous suturing algorithm | Smart Tissue Autonomous Robot | Feasibility of supervised autonomous robotic soft tissue surgery | The outcome of supervised autonomous procedures is superior to surgery performed by expert surgeons |
Cho Y, et al. | To enhance the accuracy of gesture recognition for contactless interfaces | Support vector machine classifier and Naïve Bayes classifier | Enhancement of the accuracy of gesture recognition | Overall accuracy of the five gestures was 99.58%±0.06%, and 98.74%±3.64% on a personal basis using SVM and Naïve Bayes classifiers |
Wang Z, et al. | To propose an analytical deep learning framework for skill assessment in surgical training | Convolutional neural network | Objective skill evaluation in robot-assisted surgery | The proposed learning model achieved competitive accuracies of 92.5%, 95.4%, and 91.3%, in the standard training tasks: suturing, needle-passing, and knot-tying |
Fard et al. | To build a classification framework to automatically evaluate the performance of surgeons with different levels of expertise | Multiple ML algorithms | Automated robot-assisted surgical skill evaluation | The proposed framework can classify surgeons’ expertise as novice or expert with an accuracy of 82.3% for knot tying and 89.9% for a suturing task |
Ershad M, et al. | To propose a sparse coding framework for automatic stylistic behavior recognition in short time intervals using only position data from the hands, wrist, elbow, and shoulder | Support vector machine | Evaluation of technical skills in robotic surgery | The proposed dictionary learning method can assess stylistic behavior performance in near real time using user joint position data with improved accuracy |
SVM, support vector machine.
Albeit its undisputed advantages, robotic surgery is associated with longer procedural times, and substantial costs (55,56), hence an accurate scheduling of surgical procedures is needed. AI algorithms proved to be a valuable tool to properly plan each procedure, improving the prediction of case duration, and the detection of surgeries with high risks of cancellation (57,58).
The problem of surgical room organization has become increasingly important in the last year; the current coronavirus disease pandemic challenged us to face a disruption of healthcare systems, with considerable consequences on surgery waiting lists and scheduling. In this context, it was hypothesized that ML models might have a substantial role in the optimization of operating rooms efficiency, allowing to save costs and maximize resources (59,60).
Pathology
Histopathological diagnosis remains a crucial step for the optimal therapeutic planning and prognosis prediction. The high variability among pathologists prompted to evaluate the application of AI to computational pathology. In 2016, Yu et al. successfully used ML methods for the prognostic prediction of lung adenocarcinoma and squamous cell carcinoma (SCC) patients (61). Comparable results were obtained by Coudray et al., who trained a CNN to distinguish between adenocarcinoma and SCC and to predict mutations from NSCLC histopathology (62). Neural networks algorithms were similarly employed to distinguish histologic patterns of lung adenocarcinoma (63,64), and, more recently, to successfully differentiating between lung carcinoma and non-neoplastic lesion (65) (Table 5).
Table 5
Reference | Objective | AI algorithm | Application | Main results |
---|---|---|---|---|
Yu KH, et al. | To improve the prognostic prediction of lung adenocarcinoma and squamous cell carcinoma patients through objective features distilled from histopathology images | Elastic net-Cox proportional hazards model | Prediction of the prognosis of lung cancer by automated pathology image features and thereby contribution to precision oncology | Automatically derived image features can predict the prognosis of lung cancer patients |
Coudray N, et al. | To train a deep convolutional neural network on whole-slide images obtained from The Cancer Genome Atlas to accurately and automatically classify them | Deep convolutional neural network | Detection of cancer subtype or gene mutations and mutation prediction from non-small cell lung cancer histopathology | Deep-learning models can assist pathologists in the detection of cancer subtype or gene mutations |
Wei JW, et al. | To propose a deep learning model that automatically classifies the histologic patterns of lung adenocarcinoma on surgical resection slides | Deep neural network | Improvement of classification of lung adenocarcinoma patterns | All evaluation metrics for the model and the three pathologists were within 95% confidence intervals of agreement |
Gertych A, et al. | To a pipeline equipped with a CNN to distinguish four growth patterns of pulmonary adenocarcinoma (acinar, micropapillary, solid, and cribriform) and separate tumor regions from non-tumor | Convolutional neural network | To assist pathologists in improving classification of lung adenocarcinoma patterns by automatically pre-screening and highlighting cancerous regions prior to review | The overall accuracy of distinguishing the tissue classes was 89.24% |
KanavatI F, et al. | To train a CNN, using transfer learning and weakly-supervised learning, to predict carcinoma in Whole Slide Images | Convolutional neural network | Development of software suites that could be adopted in routine pathological practices and potentially help reduce the burden on pathologists | Highly promising results for differentiating between lung carcinoma and non-neoplastic lesion |
CNN, Convolutional Neural Network.
Finally, some studied investigated the application of AI on CT scan and PET/CT to provide a pathological classification of lung carcinoma (66,67).
Prognosis
Some researchers recently explored the application of AI models and radiomics to predict therapy response and outcomes in lung malignancies (68,69).
Moreover, machine learning approaches provide encouraging results to predict the risk of recurrence of lung and esophageal adenocarcinoma (70,71).
Limits, legal and ethical issues
Despite the encouraging results of AI implementation in all fields of patient-care settings, before it can be applied to daily practice, several issues remain to be addressed.
The widespread application of AI has opened new debates about legislative issues and the protection of privacy. This aspect has become even more evident in the world of healthcare, where progress has to deal with the protection of personal and extremely sensitive information. For this reason, some scientific societies have developed specific guidelines on the subject (72,73). Nevertheless, the technology at the moment is spread so fast that the legislative paths are not always able to keep its rapidity. An example is the General Data Protection Regulation 2018 by the European Union. (EU-GDPR), which despite having been drawn up to meet new requirements, not explicitly insert the term “artificial intelligence” in the text (74). Furthermore, by not specifically addressing the subject of AI, often the indications provided are sometimes too stringent to allow adequate progress of these technologies and escape strategies are needed to be able to continue developing them (75). If on the one hand AI in medicine is offering many promises, on the other hand the rights of citizens must always be able to be considered; it is a very delicate balance between progress and privacy (76). A specific regulation of AI in healthcare will probably be necessary. Furthermore, a legislative expert should be also included in each multidisciplinary research group dealing with this topic in order to be in line with current legislation.
The efficacy, validation, and improvement of ML algorithms depend on the amount and supply of high-quality data (4). Data need to be available in a standard format and accurately labeled to be shared across centers, thus raising concerns about data protection, informed consent, and cybersecurity (77,78). Furthermore, data used for ML training models can be easily biased, hence the publication of consensus guidelines to assess the validation of AI-based technologies is needed (79). Still, the different geographic distribution of AI implementation remains a major ethical concern; a mindful effort should be made to ensure that all population can receive equal access to the benefits provided by ML models (11).
Finally, due to the huge influence that the implementation of AI algorithms can exert on clinical practice, a primary focus should be the involvement of physicians and researchers, who need to be adequately educated on these technologies, their proper use, and limitations (80). Recently, several prestigious Universities and Scientific Societies are offering specialized courses regarding the use of Big Data, AI and ML in healthcare. It will be essential that healthcare professionals reach a certain degree of digital literacy that will enable them to interface with these new technologies and to extrapolate the maximum use for their patients (81). This will allow for parallel growth and prevent the physician from succumbing to this technological revolution.
Conclusions
Technologies are becoming more and more present in health-care settings. In the perioperative medicine, ML algorithms implementation could prompt a multidisciplinary approach, particularly in preoperative assessment, risk stratification, and postoperative outcomes. AI along with the last innovations of the Health-Technology Assessment (HTA) and Telemedicine, will be the cornerstone of the future of perioperative medicine. Several applications in thoracic surgery have been described, both clinical, organizational, and educational. However, further validation studies are needed to understand the real impact of AI in this specific surgical context.
Acknowledgments
Funding: This review was completed as a part of the research fellowship of University of Parma MADA-MED (MAchine learning and big DAta in medicina perioperatoria), co-funded with resources of FSE (Fondo Sociale Europeo, delibera di G.R. 589/2019–Rif. PA 2019-11449/RER).
Footnote
Provenance and Peer Review: This article was commissioned by the Guest Editors (Jianxing He and Hengrui Liang) for the series “Artificial Intelligence in Thoracic Disease: From Bench to Bed” published in Journal of Thoracic Disease. The article has undergone external peer review.
Reporting Checklist: The authors have completed the Narrative Review reporting checklist. Available at: https://dx.doi.org/10.21037/jtd-21-761
Peer Review File: Available at https://dx.doi.org/10.21037/jtd-21-761
Conflicts of Interest: The authors have completed the ICMJE uniform disclosure form (available at https://dx.doi.org/10.21037/jtd-21-761). The series “Artificial Intelligence in Thoracic Disease: From Bench to Bed” was commissioned by the editorial office without any funding or sponsorship. The authors have no other conflicts of interest to declare.
Ethical Statement: The authors are accountable for all aspects of the work in ensuring that questions related to the accuracy or integrity of any part of the work are appropriately investigated and resolved.
Open Access Statement: This is an Open Access article distributed in accordance with the Creative Commons Attribution-NonCommercial-NoDerivs 4.0 International License (CC BY-NC-ND 4.0), which permits the non-commercial replication and distribution of the article with the strict proviso that no changes or edits are made and the original work is properly cited (including links to both the formal publication through the relevant DOI and the license). See: https://creativecommons.org/licenses/by-nc-nd/4.0/.
References
- Goodfellow I, Bengio B, Courville A. Deep Learning. MIT Press 2016.
- Accenture. Artificial Intelligence: Healthcare’s New Nervous System. Accenture Report, 2017. Available online: https://www.accenture.com/_acnmedia/PDF-49/Accenture-Health-Artificial-Intelligence.pdf
- Jiang F, Jiang Y, Zhi H, et al. Artificial intelligence in healthcare: past, present and future. Stroke Vasc Neurol 2017;2:230-43. [Crossref] [PubMed]
- He J, Baxter SL, Xu J, et al. The practical implementation of artificial intelligence technologies in medicine. Nat Med 2019;25:30-6. [Crossref] [PubMed]
- Murdoch TB, Detsky AS. The inevitable application of big data to health care. JAMA 2013;309:1351-2. [Crossref] [PubMed]
- Murff HJ, FitzHenry F, Matheny ME, et al. Automated identification of postoperative complications within an electronic medical record using natural language processing. JAMA 2011;306:848-55. [Crossref] [PubMed]
- Beam AL, Kohane IS. Big Data and Machine Learning in Health Care. JAMA 2018;319:1317-8. [Crossref] [PubMed]
- Sidey-Gibbons JAM, Sidey-Gibbons CJ. Machine learning in medicine: a practical introduction. BMC Med Res Methodol 2019;19:64. [Crossref] [PubMed]
- Breiman L. Random Forests. Machine Learning 2001;45:5-32. [Crossref]
- Shah SJ, Katz DH, Selvaraj S, et al. Phenomapping for novel classification of heart failure with preserved ejection fraction. Circulation 2015;131:269-79. [Crossref] [PubMed]
- Ostberg NP, Zafar MA, Elefteriades JA. Machine learning: principles and applications for thoracic surgery. Eur J Cardiothorac Surg 2021;60:213-21. [Crossref] [PubMed]
- Abraham J. Reduced lung cancer mortality with low-dose computed tomographic screening. Community Oncology 2011;8:441-4. [Crossref]
- Armato SG 3rd, Altman MB, La Rivière PJ. Automated detection of lung nodules in CT scans: effect of image reconstruction algorithm. Med Phys 2003;30:461-72. [Crossref] [PubMed]
- Li Q, Li F, Suzuki K, et al. Computer-aided diagnosis in thoracic CT. Semin Ultrasound CT MR 2005;26:357-63. [Crossref] [PubMed]
- Roos JE, Paik D, Olsen D, et al. Computer-aided detection (CAD) of lung nodules in CT scans: radiologist performance and reading time with incremental CAD assistance. Eur Radiol 2010;20:549-57. [Crossref] [PubMed]
- Murphy A, Skalski M, Gaillard F. The utilisation of convolutional neural networks in detecting pulmonary nodules: a review. Br J Radiol 2018;91:20180028. [Crossref] [PubMed]
- Yang Y, Feng X, Chi W, et al. Deep learning aided decision support for pulmonary nodules diagnosing: a review. J Thorac Dis 2018;10:S867-75. [Crossref] [PubMed]
- Krizhevsky A, Sutskever I, Hinton GE. ImageNet classification with deep convolutional neural networks. Communications of the ACM 2017;60:84-90. [Crossref]
- Kadir T, Gleeson F. Lung cancer prediction using machine learning and advanced imaging techniques. Transl Lung Cancer Res 2018;7:304-12. [Crossref] [PubMed]
- Ciompi F, Chung K, van Riel SJ, et al. Towards automatic pulmonary nodule management in lung cancer screening with deep learning. Sci Rep 2017;7:46479. [Crossref] [PubMed]
- Hussein S, Gillies R, Cao K, et al. TumorNet: Lung nodule characterization using multi-view Convolutional Neural Network with Gaussian Process. 2017 IEEE 14th International Symposium on Biomedical Imaging (ISBI 2017), 2017, pp. 1007-1010.
- Nam JG, Park S, Hwang EJ, et al. Development and Validation of Deep Learning-based Automatic Detection Algorithm for Malignant Pulmonary Nodules on Chest Radiographs. Radiology 2019;290:218-28. [Crossref] [PubMed]
- Li W, Cao P, Zhao D, et al. Pulmonary Nodule Classification with Deep Convolutional Neural Networks on Computed Tomography Images. Comput Math Methods Med 2016;2016:6215085. [Crossref] [PubMed]
- Nibali A, He Z, Wollersheim D. Pulmonary nodule classification with deep residual networks. Int J Comput Assist Radiol Surg 2017;12:1799-808. [Crossref] [PubMed]
- Eppenhof KAJ, Pluim JPW, Pulmonary CT. Registration Through Supervised Learning With Convolutional Neural Networks. IEEE Trans Med Imaging 2019;38:1097-105. [Crossref] [PubMed]
- da Silva GLF, Valente TLA, Silva AC, et al. Convolutional neural network-based PSO for lung nodule false positive reduction on CT images. Comput Methods Programs Biomed 2018;162:109-18. [Crossref] [PubMed]
- Ather S, Kadir T, Gleeson F. Artificial intelligence and radiomics in pulmonary nodule management: current status and future applications. Clin Radiol 2020;75:13-9. [Crossref] [PubMed]
- Talwar A, Willaime JMY, Pickup LC, et al. Pulmonary nodules: Assessing the imaging biomarkers of malignancy in a "coffee-break". Eur J Radiol 2018;101:82-6. [Crossref] [PubMed]
- Yang Y, Jin G, Pang Y, et al. The diagnostic accuracy of artificial intelligence in thoracic diseases: A protocol for systematic review and meta-analysis. Medicine (Baltimore) 2020;99:e19114. [Crossref] [PubMed]
- Naqi SM, Sharif M, Yasmin M. Multistage segmentation model and SVM-ensemble for precise lung nodule detection. Int J Comput Assist Radiol Surg 2018;13:1083-95. [Crossref] [PubMed]
- Choi W, Oh JH, Riyahi S, et al. Radiomics analysis of pulmonary nodules in low-dose CT for early detection of lung cancer. Med Phys 2018;45:1537-49. [Crossref] [PubMed]
- Bashir U, Kawa B, Siddique M, et al. Non-invasive classification of non-small cell lung cancer: a comparison between random forest models utilising radiomic and semantic features. Br J Radiol 2019;92:20190159. [Crossref] [PubMed]
- Horie Y, Yoshio T, Aoyama K, et al. Diagnostic outcomes of esophageal cancer by artificial intelligence using convolutional neural networks. Gastrointest Endosc 2019;89:25-32. [Crossref] [PubMed]
- Cai SL, Li B, Tan WM, et al. Using a deep learning system in endoscopy for screening of early esophageal squamous cell carcinoma (with video). Gastrointest Endosc 2019;90:745-753.e2. [Crossref] [PubMed]
- Guo L, Xiao X, Wu C, et al. Real-time automated diagnosis of precancerous lesions and early esophageal squamous cell carcinoma using a deep learning model (with videos). Gastrointest Endosc 2020;91:41-51. [Crossref] [PubMed]
- Hu J, Zhao Y, Li M, et al. Machine-learning-based computed tomography radiomic analysis for histologic subtype classification of thymic epithelial tumours. Eur J Radiol 2020;126:108929. [Crossref] [PubMed]
- Lee CK, Hofer I, Gabel E, et al. Development and Validation of a Deep Neural Network Model for Prediction of Postoperative In-hospital Mortality. Anesthesiology 2018;129:649-62. [Crossref] [PubMed]
- Bihorac A, Ozrazgat-Baslanti T, Ebadi A, et al. MySurgeryRisk: Development and Validation of a Machine-learning Risk Algorithm for Major Complications and Death After Surgery. Ann Surg 2019;269:652-62. [Crossref] [PubMed]
- Brunelli A, Salati M, Rocco G, et al. European risk models for morbidity (EuroLung1) and mortality (EuroLung2) to predict outcome following anatomic lung resections: an analysis from the European Society of Thoracic Surgeons database. Eur J Cardiothorac Surg 2017;51:490-7. [PubMed]
- Etienne H, Hamdi S, Le Roux M, et al. Artificial intelligence in thoracic surgery: past, present, perspective and limits. Eur Respir Rev 2020;29:200010. [Crossref] [PubMed]
- Esteva H, Marchevsky A, Núñez T, et al. Neural networks as a prognostic tool of surgical risk in lung resections. Ann Thorac Surg 2002;73:1576-81. [Crossref] [PubMed]
- Santos-García G, Varela G, Novoa N, et al. Prediction of postoperative morbidity after lung resection using an artificial neural network ensemble. Artif Intell Med 2004;30:61-9. [Crossref] [PubMed]
- Bolourani S, Wang P, Patel VM, et al. Predicting respiratory failure after pulmonary lobectomy using machine learning techniques. Surgery 2020;168:743-52. Erratum in: Surgery 2021;169:1001. [Crossref] [PubMed]
- Salati M, Migliorelli L, Moccia S, et al. A Machine Learning Approach for Postoperative Outcome Prediction: Surgical Data Science Application in a Thoracic Surgery Setting. World J Surg 2021;45:1585-94. [Crossref] [PubMed]
- Chang YJ, Hung KC, Wang LK, et al. A Real-Time Artificial Intelligence-Assisted System to Predict Weaning from Ventilator Immediately after Lung Resection Surgery. Int J Environ Res Public Health 2021;18:2713. [Crossref] [PubMed]
- Birkhoff DC, van Dalen ASHM, Schijven MP. A Review on the Current Applications of Artificial Intelligence in the Operating Room. Surg Innov 2021;28:611-9. [Crossref] [PubMed]
- Andras I, Mazzone E, van Leeuwen FWB, et al. Artificial intelligence and robotics: a combination that is changing the operating room. World J Urol 2020;38:2359-66. [Crossref] [PubMed]
- Mack MJ. Minimally invasive and robotic surgery. JAMA 2001;285:568-72. [Crossref] [PubMed]
- Dai Y, Abiri A, Pensa J, et al. Biaxial sensing suture breakage warning system for robotic surgery. Biomed Microdevices 2019;21:10. [Crossref] [PubMed]
- Shademan A, Decker RS, Opfermann JD, et al. Supervised autonomous robotic soft tissue surgery. Sci Transl Med 2016;8:337ra64. [Crossref] [PubMed]
- Cho Y, Lee A, Park J, et al. Enhancement of gesture recognition for contactless interface using a personalized classifier in the operating room. Comput Methods Programs Biomed 2018;161:39-44. [Crossref] [PubMed]
- Wang Z, Majewicz Fey A. Deep learning with convolutional neural network for objective skill evaluation in robot-assisted surgery. Int J Comput Assist Radiol Surg 2018;13:1959-70. [Crossref] [PubMed]
- Fard MJ, Ameri S, Darin Ellis R, et al. Automated robot-assisted surgical skill evaluation: Predictive analytics approach. Int J Med Robot 2018; [Crossref] [PubMed]
- Ershad M, Rege R, Majewicz Fey A. Automatic and near real-time stylistic behavior assessment in robotic surgery. Int J Comput Assist Radiol Surg 2019;14:635-43. [Crossref] [PubMed]
- Khorgami Z, Li WT, Jackson TN, et al. The cost of robotics: an analysis of the added costs of robotic-assisted versus laparoscopic surgery using the National Inpatient Sample. Surg Endosc 2019;33:2217-21. [Crossref] [PubMed]
- Cao C, Manganas C, Ang SC, et al. A systematic review and meta-analysis on pulmonary resections by robotic video-assisted thoracic surgery. Ann Cardiothorac Surg 2012;1:3-10. [PubMed]
- Luo L, Zhang F, Yao Y, et al. Machine learning for identification of surgeries with high risks of cancellation. Health Informatics J 2020;26:141-55. [Crossref] [PubMed]
- Zhao B, Waterman RS, Urman RD, et al. A Machine Learning Approach to Predicting Case Duration for Robot-Assisted Surgery. J Med Syst 2019;43:32. [Crossref] [PubMed]
- Rozario N, Rozario D. Can machine learning optimize the efficiency of the operating room in the era of COVID-19? Can J Surg 2020;63:E527-9. [Crossref] [PubMed]
- Bellini V, Guzzon M, Bigliardi B, et al. Artificial Intelligence: A New Tool in Operating Room Management. Role of Machine Learning Models in Operating Room Optimization. J Med Syst 2019;44:20. [Crossref] [PubMed]
- Yu KH, Zhang C, Berry GJ, et al. Predicting non-small cell lung cancer prognosis by fully automated microscopic pathology image features. Nat Commun 2016;7:12474. [Crossref] [PubMed]
- Coudray N, Ocampo PS, Sakellaropoulos T, et al. Classification and mutation prediction from non-small cell lung cancer histopathology images using deep learning. Nat Med 2018;24:1559-67. [Crossref] [PubMed]
- Wei JW, Tafe LJ, Linnik YA, et al. Pathologist-level classification of histologic patterns on resected lung adenocarcinoma slides with deep neural networks. Sci Rep 2019;9:3358. [Crossref] [PubMed]
- Gertych A, Swiderska-Chadaj Z, Ma Z, et al. Convolutional neural networks can accurately distinguish four histologic growth patterns of lung adenocarcinoma in digital slides. Sci Rep 2019;9:1483. [Crossref] [PubMed]
- Kanavati F, Toyokawa G, Momosaki S, et al. Weakly-supervised learning for lung carcinoma classification using deep learning. Sci Rep 2020;10:9297. [Crossref] [PubMed]
- Aydin N, Çelik Ö, Aslan AF, et al. Detection Of Lung Cancer On Computed Tomography Using Artificial Intelligence Applications Developed By Deep Learning Methods And The Contribution Of Deep Learning To The Classification Of Lung Carcinoma. Curr Med Imaging 2021. [Epub ahead of print]. doi:
10.2174/1573405617666210204210500 .10.2174/1573405617666210204210500 - Hyun SH, Ahn MS, Koh YW, et al. A Machine-Learning Approach Using PET-Based Radiomics to Predict the Histological Subtypes of Lung Cancer. Clin Nucl Med 2019;44:956-60. [Crossref] [PubMed]
- Park C, Na KJ, Choi H, et al. Tumor immune profiles noninvasively estimated by FDG PET with deep learning correlate with immunotherapy response in lung adenocarcinoma. Theranostics 2020;10:10838-48. [Crossref] [PubMed]
- Trebeschi S, Drago SG, Birkbak NJ, et al. Predicting response to cancer immunotherapy using noninvasive radiomic biomarkers. Ann Oncol 2019;30:998-1004. [Crossref] [PubMed]
- Rahman SA, Walker RC, Lloyd MA, et al. Machine learning to predict early recurrence after oesophageal cancer surgery. Br J Surg 2020;107:1042-52. [Crossref] [PubMed]
- Koike Y, Aokage K, Ikeda K, et al. Machine learning-based histological classification that predicts recurrence of peripheral lung squamous cell carcinoma. Lung Cancer 2020;147:252-8. [Crossref] [PubMed]
- Brunelle F, Brunelle P. Artificial intelligence and medical imaging: Definition, state of the art and perspectives. Bull Acad Natl Med 2019;203:8-9. [Crossref]
- MMatheny M, Israni ST, Ahmed M, et al. Artificial Intelligence in Health Care: The Hope, the Hype, the Promise, the Peril. 2019. Available online: https://nam.edu/artificial-intelligence-special-publication/
- I (Legislative acts) REGULATIONS REGULATION (EU) 2016/679 OF THE EUROPEAN PARLIAMENT AND OF THE COUNCIL of 27 April 2016 on the protection of natural persons with regard to the processing of personal data and on the free movement of such data, and repealing Directive 95/46/EC (General Data Protection Regulation) (Text with EEA relevance). Available online: https://gdpr-info.eu/
- Nick W, Castro D. The Impact of the EU’s New Data Protection Regulation on AI. Available online: https://datainnovation.org/2018/03/the-impact-of-the-eus-new-data-protection-regulation-on-ai/
- Price WN 2nd, Cohen IG. Privacy in the age of medical big data. Nat Med 2019;25:37-43. [Crossref] [PubMed]
- Kayaalp M. Patient Privacy in the Era of Big Data. Balkan Med J 2018;35:8-17. [Crossref] [PubMed]
- Char DS, Shah NH, Magnus D. Implementing Machine Learning in Health Care - Addressing Ethical Challenges. N Engl J Med 2018;378:981-3. [Crossref] [PubMed]
- CONSORT-AI and SPIRIT-AI Steering Group. Reporting guidelines for clinical trials evaluating artificial intelligence interventions are needed. Nat Med 2019;25:1467-8. Erratum in: Nat Med 2019;25:1948. [Crossref] [PubMed]
- Bignami E, Bellini V. Do We Need Specific Certification to Use Anesthesia Information Management Systems? Anesth Analg 2019;128:e30-1. [Crossref] [PubMed]
- Rampton V, Mittelman M, Goldhahn J. Implications of artificial intelligence for medical education. Lancet Digit Health 2020;2:e111-2. [Crossref] [PubMed]