Neoadjuvant camrelizumab, nab-paclitaxel, and carboplatin in patients with stage IB-IIIA non-small cell lung cancer (NANE-LC): a study protocol of prospective, single-arm, multicenter, phase II study
Introduction
Randomized controlled trials have shown that patients with non-small cell lung cancer (NSCLC) who receive neoadjuvant chemotherapy obtain enhanced survival benefits compared with patients treated with surgery alone (1-3). In the NATCH trial, NSCLC patients achieved 22.8% of major pathological response (MPR), and virtually no patients achieved pathological complete response (pCR) after neoadjuvant chemotherapy (2). Although neoadjuvant chemotherapy improves the survival outcome of resectable NSCLC, it is still insufficient, and new neoadjuvant therapy regimens are needed to improve treatment efficacy.
Immune checkpoint inhibitors (ICIs) that target the programmed cell death 1 (PD-1) axis have revolutionized the management of advanced NSCLC (4-8). These ICIs have been utilized for neoadjuvant therapy in patients with resectable NSCLC. The patients with resectable NSCLC who were enrolled in the CheckMate 159 trial (9) were treated with two cycles of neoadjuvant therapy using nivolumab monotherapy. This treatment significantly improved the MPR rate (45%) and the pCR rate (15%). However, the NEOSTAR (10) and the LCMC3 (11) trials, assessing patients under neoadjuvant ICI monotherapy, yielded an MPR rate of 17% and 19%, respectively. Thus, the benefit of neoadjuvant therapy based on ICI monotherapy in patients with resectable NSCLC still remains controversial.
To further improve the benefits for patients with resectable NSCLC, a new strategy of neoadjuvant therapy combining ICIs and chemotherapy has been implemented. The NADIM trial (12) was a phase II clinical trial that enrolled stage IIIA NSCLC patients who received three cycles of neoadjuvant therapy with nivolumab, paclitaxel, and carboplatin. This trial demonstrated that neoadjuvant ICIs combined with chemotherapy significantly improve patients’ MPR and pCR ratios (83% and 71%, respectively). Another phase II clinical trial (13) enrolled patients with stage IB-IIIA NSCLC who received four cycles of neoadjuvant therapy with atezolizumab, nab-paclitaxel, and carboplatin. In this trial, 50% of the patients achieved MPR, of which 21% achieved pCR. Although both trials demonstrated that neoadjuvant ICIs combined with platinum-based chemotherapy bring additional benefits for patients with NSCLC, high-level evidence-based medical data are still lacking to support this observation.
Moreover, current published studies have focused on finding appropriate biomarkers or strategies to predict the benefit for patients receiving ICIs. Among these, the expression of programmed cell death-ligand 1 (PD-L1), the tumor mutation burden (TMB), and high microsatellite instability or mismatch repair deficiency have been widely used for patients with advanced solid tumors (6,14-16). The combination of these biomarkers with other factors, such as sex, genetic mutations, or ctDNA maximum somatic allele frequency, seems to be a promising strategy for predicting the benefit for patients receiving ICIs (17-20). Regarding patients with resectable NSCLC, the Checkmate-159 trial indicated an absence of correlation between pathological response to ICIs and PD-L1 expression, while there was a linear correlation between pathological response and TMB (9).
Currently, some new strategies for predicting the efficacy of ICIs are being discovered and reported. The INSPIRE trial (21), a phase II trial, demonstrated that the changes in ctDNA before and after ICIs are strongly correlated with the benefit of ICIs in patients with incurable, locally advanced, or metastatic solid tumors. Importantly, the CheckMate 078 and CheckMate 870 (22) trials revealed a strong association between the gut microbiome diversity and patient’s response to ICIs, implying that gut microbiome diversity may be a predictive strategy for ICIs in patients with NSCLC. Furthermore, long non-coding RNAs (lncRNAs) were found to be closely related to immune dysfunction and response to ICIs. A novel multiomics algorithm model that included TMB, PD-L1 expression, cytotoxic T-lymphocyte infiltration, and lncRNA score displayed a strong correlation with the overall survival (OS) of patients treated with ICIs (23). Moreover, some studies have shown that artificial intelligence can be applied for the diagnosis and treatment of NSCLC patients, while radiographic characteristics might serve as noninvasive approaches for predicting the response to immunotherapy (24,25). However, it is still unclear whether these predictive biomarkers or strategies are effective in predicting the benefit to patients receiving neoadjuvant ICIs.
The aim of this study is to evaluate the efficacy and safety of neoadjuvant therapy with the PD-1 inhibitor camrelizumab, nab-paclitaxel, and carboplatin for patients with stage IB-IIIA NSCLC and to establish a multiomics artificial intelligence system to predict neoadjuvant therapy efficacy based on radiomics, metabolism, genetic, and clinical-pathological characteristics. In addition, the resistance mechanisms to neoadjuvant therapy that utilizes camrelizumab, nab-paclitaxel, and carboplatin will be explored.
Methods
Study design
This NANE-LC study is a prospective, single-arm, multicenter, phase II trial. A total of 40 patients will undergo surgery after three cycles of neoadjuvant therapy with camrelizumab, nab-paclitaxel, and carboplatin. Figure 1 shows the flowchart of the trial.
Eligibility criteria
The key inclusion and exclusion criteria of this study are shown in Figure 2.
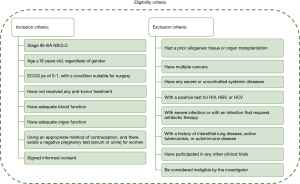
Treatment
The patients will receive three cycles (one cycle is defined as a period of 21±3 days) of neoadjuvant therapy with camrelizumab 200 mg, nab-paclitaxel 260 mg/m2, and carboplatin area under the curve (AUC) 5. This treatment will be followed by surgery.
Patient registration
After confirming eligibility and obtaining a signed informed consent form, each patient will be registered and will receive treatment. Patient recruitment began in August 2020. It is expected to continue for the next two years.
Specimen collection
During the trial, samples of tumor tissues will be collected, including biopsy samples and surgical tumor tissue samples. Blood samples and fecal samples will be collected before every cycle of neoadjuvant therapy and before surgery. The tumor tissues and blood samples will be used for whole genome sequencing, whole transcriptome sequencing, and single-cell sequencing. The fecal samples will be used for 16S rDNA sequencing and nontargeted metabolomics.
Dosages and treatment regimen
Adjustment of the dosage regimens in case of adverse events (AEs) occurring during the trial shall be based on the dose-adjustment regulations specified on the drug medication guides or relevant clinical decisions taken by the researchers.
Endpoint measures
The primary endpoint measure is the MPR rate. The secondary endpoint measures are the pCR rate, the complete resection (R0) rate, the disease-free survival (DFS), the OS, the objective response rate (ORR), AEs, serious AEs, and quality of life (QOL). The exploratory endpoint measure is the establishment of an artificial intelligence prediction system for neoadjuvant therapy effect prediction and exploration of drug resistance mechanisms. This system will be based on the multiomics data, including radiomics, metabolism, genetic, and pathological characteristics. A detailed description is provided in Figure 3.
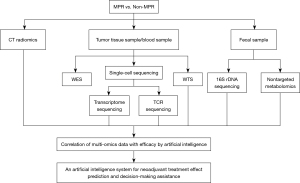
Response and safety evaluation
Pre-treatment evaluations of the patients are compulsory and include chest and abdomen-enhanced computed tomography (CT) scans, brain magnetic resonance imaging scans, bone scans or positron emission tomography scans, and electrocardiography. Patients will undergo a tumor evaluation using a chest-enhanced CT scan before the third cycle of neoadjuvant therapy and prior to surgery. The tumor response and/or radiologic disease progression will be evaluated based on version 1.1 of the Response Evaluation Criteria in Solid Tumors. AEs will be recorded using version 5.0 of the National Cancer Institute’s Common Terminology Criteria for Adverse Events. The QOL will be assessed using questionnaires of the Quality of Life Questionnaire-Core 30 of The European Organization for Research and Functional Assessment of Cancer Therapy-Lung before and after every cycle of neoadjuvant treatment.
Statistical analysis
Descriptive statistics will be used to summarize the demographic data and baseline characteristics of the patients, and a t-test, a chi-square test, or a rank sum test will be used to assess group equilibria. The number and percentage of MPR, pCR, the R0 excision rate, and the ORR will be calculated. The Kaplan-Meier method will be used to estimate the survival curve, median, and annual rate of DFS and OS. For the treatment sensitivity analysis of the biomarkers, the Cox univariate and multivariate analyses will be used to analyze the correlation between biomarker expression, clinical efficacy, and prognosis. Artificial intelligence algorithms will be used to establish a prediction system for the neoadjuvant therapy outcome based on the multiomics data. In the process of Artificial intelligence analysis, convolutional neural network (CNN)-based deep learning will be used to train images, and a variety of algorithms, including GoogLeNet, RetinaNet, ResNet, RCNN, fast-rCNN, fath-rCNN, and VGGNet, will be used for feature extraction, screening, modeling, and verification. Moreover, transfer learning algorithm will be used, which refers to the method of transferring the pre-trained CNN model to other datasets and relearning the characteristics of the target dataset. Data augmentation will be adopted to increase the size of the training dataset and expand the existing samples by iterations of random translational shift, rotation, and horizontal and vertical flips. The performance of the prediction system will be evaluated using the receiver operating characteristic curve, the corresponding AUC, the diagnostic accuracy, sensitivity, specificity, the positive predictive value, and the negative predictive value. The Clopper Pearson method will be used to calculate the 95% confidence interval. All statistical analyses will be performed with R software 4.0.5 and SPSS 24.0 software.
Sample size
The sample size was calculated using the Simon two-stage method. MPR rates of 22.8% and 17% were expected according to the NATCH (2) and the NEOSTAR (10) trials, respectively, and an MPR rate of 19% was expected according to the LCMC3 trial (11), in which patients received neoadjuvant ICI monotherapy. It is believed that patients treated with neoadjuvant ICI monotherapy could achieve MPR rates of about 20%. Therefore, an MPR rate of 42.8% was expected in this study, with a two-sided alpha value of 0.05 and a power of 0.8. The sample size for the first stage was 13. If more than three cases reach the MPR, the second stage will begin, in which 27 cases will be included. The results of this trial will be considered positive if more than 13 cases out of 40 effective cases reach MPR. Effective cases are defined as patients who received at least once cycle of neoadjuvant therapy and completed the first tumor assessment.
Ethical consideration and registration
This trial protocol was approved on July 23, 2020 by the ethics committee of Sun Yat-sen Memorial Hospital of Sun Yat-sen University (No. 2020-KY-061-001), Jiangxi Provincial Cancer Hospital (No. 2021ky074), and Third Affiliated Hospital of Sun Yat-sen University (No. [2021]02-205-01). All patients enrolled in the trial have signed or will sign informed consent forms prior to registration according to the Declaration of Helsinki (as revised in 2013). This protocol has been registered at ClinicalTrials.gov (identification number: NCT04541251). As of August 2021, three subjects have been enrolled and have signed the informed consent forms.
Discussion
ICIs, one of the most significant immunotherapies, have entered clinical practice and brought new hope to NSCLC patients. Although many ongoing trials focus on the identification of new biomarkers, such as PD-L1 and TMB, usable as potential surrogates for the prediction of clinical outcomes, current strategies and biomarkers for predicting ICI efficacy are still limited. In this study, we not only aim to evaluate the efficacy and safety of ICIs combined with chemotherapy as neoadjuvant therapy for NSCLC patients but also to explore the efficacy of new prediction strategies.
It is well known that NSCLC patients with epidermal growth factor receptor (EGFR) mutations are preferred for targeted therapy and benefit little from immunotherapy. Related studies have shown that ICIs combined with chemotherapy or target drug therapy might show improved efficacy for NSCLC patients with EGFR mutations (26-28). NSCLC patients with unknown EGFR status could benefit from ICIs alone (9) or combined with chemotherapy (11,13). Therefore, we did not use a particular EGFR status in the enrollment criteria, and we hope to discuss this point during the future data analysis.
In the meantime, a significant correlation between PD-L1 expression and smoking habits has been reported (29). Yet, recent subgroup analyses from the KEYNOTE-042 study (30) showed that there was no significant OS benefit for the never-smoking subgroup of patients with PD-L1 positive expression, regardless of PD-L1 TPS ≥1%, ≥20%, or ≥50%. In contrast, subgroup analyses in the KEYNOTE-189 study (4) showed better OS benefit for never-smoking NSCLC patients than for current or former smoking patients who received ICI combined with chemotherapy. In a recent retrospective study (31), the researchers analyzed the association between smoking and ICI activity in 315 NSCLC patients with PD-L1 TPS ≥50%. They found that a number of never smokers and light smokers significantly benefited from immunotherapy. Although higher TMB levels in heavy smokers might lead to more significant benefits from immunotherapy, smoking status alone did not fully explain the complexity of neoantigen production and presentation. The influence of patients’ smoking habits on ICIs’ efficacy is worthy of further subgroup analysis.
With the smooth completion of this study, we hope to deliver new evidence on patients with stage IB-IIIA NSCLC who received neoadjuvant camrelizumab, nab-paclitaxel, and carboplatin. Based on radiomics, metabolism, genetic, and clinical-pathological characteristics, our new multiomics artificial intelligence system may represent a new valuable strategy for neoadjuvant therapy efficacy prediction and decision-making. In addition, this new tool may provide potential directions for exploring the mechanisms of resistance to current cancer therapies.
Acknowledgments
The authors thank the patients, their families, and the participating investigators. In addition, we appreciate the assistance obtained from the Artificial Intelligence Lab and the Big Data Center of Sun Yat-sen Memorial Hospital, Sun Yat-sen University and Jiangsu Hengrui Pharmaceutical Co. Ltd.
Funding: This study was supported by grants from the National Science and Technology Major Project (No. 2020ZX09201021), the Medical Artificial Intelligence Project of Sun Yat-sen Memorial Hospital (No. YXRGZN201902), the National Natural Science Foundation of China (No. 81572596 and 81972471), the Natural Science Foundation of Guangdong Province (No. 2017A030313828 and 2021A1515010838), the Guangzhou Science and Technology Major Program (No. 201704020131 and 201903010028), the Guangdong Science and Technology Department (No. 2017B030314026), the Sun Yat-sen University Clinical Research 5010 Program (No. 2018007), the Sun Yat-sen Clinical Research Cultivating Program (No. SYS-C-201801), the Guangdong Medical Science and Technology Program (No. A2020558), the Tencent Charity Foundation (No. SYSU-81000-20200311-0001 and SYSU-05160-20200506-0001), the Guangdong Provincial People’s Hospital Intermural Program (No. KJ012019447 and KJ012020453), and the Beijing Xisike Clinical Oncology Research Foundation (No. Y-HS202102-0038).
Footnote
Data Sharing Statement: Available at https://dx.doi.org/10.21037/jtd-21-1022
Peer Review File: Available at https://dx.doi.org/10.21037/jtd-21-1022
Conflicts of Interest: All authors have completed the ICMJE uniform disclosure form (available at https://dx.doi.org/10.21037/jtd-21-1022). The authors have no conflicts of interest to declare.
Ethical Statement: The authors are accountable for all aspects of the work in ensuring that questions related to the accuracy or integrity of any part of the work are appropriately investigated and resolved. This trial protocol was approved on July 23, 2020. It was supervised and managed by the ethics committee of Sun Yat-sen Memorial Hospital of Sun Yat-sen University (No. 2020-KY-061-001), Jiangxi Provincial Cancer Hospital (No.2021ky074), and Third Affiliated Hospital of Sun Yat-sen University (No. [2021]02-205-01). All patients enrolled in the trial have signed or will sign informed consent forms prior to registration, in accordance with the Declaration of Helsinki (as revised in 2013). As of August 2021, three subjects have been enrolled and have signed the informed consent form.
Open Access Statement: This is an Open Access article distributed in accordance with the Creative Commons Attribution-NonCommercial-NoDerivs 4.0 International License (CC BY-NC-ND 4.0), which permits the non-commercial replication and distribution of the article with the strict proviso that no changes or edits are made and the original work is properly cited (including links to both the formal publication through the relevant DOI and the license). See: https://creativecommons.org/licenses/by-nc-nd/4.0/.
References
- Pisters KM, Vallières E, Crowley JJ, et al. Surgery with or without preoperative paclitaxel and carboplatin in early-stage non-small-cell lung cancer: Southwest Oncology Group Trial S9900, an intergroup, randomized, phase III trial. J Clin Oncol 2010;28:1843-9. [Crossref] [PubMed]
- Felip E, Rosell R, Maestre JA, et al. Preoperative chemotherapy plus surgery versus surgery plus adjuvant chemotherapy versus surgery alone in early-stage non-small-cell lung cancer. J Clin Oncol 2010;28:3138-45. [Crossref] [PubMed]
- Scagliotti GV, Pastorino U, Vansteenkiste JF, et al. Randomized phase III study of surgery alone or surgery plus preoperative cisplatin and gemcitabine in stages IB to IIIA non-small-cell lung cancer. J Clin Oncol 2012;30:172-8. [Crossref] [PubMed]
- Gandhi L, Rodríguez-Abreu D, Gadgeel S, et al. Pembrolizumab plus Chemotherapy in Metastatic Non-Small-Cell Lung Cancer. N Engl J Med 2018;378:2078-92. [Crossref] [PubMed]
- Paz-Ares L, Luft A, Vicente D, et al. Pembrolizumab plus Chemotherapy for Squamous Non-Small-Cell Lung Cancer. N Engl J Med 2018;379:2040-51. [Crossref] [PubMed]
- Reck M, Rodríguez-Abreu D, Robinson AG, et al. Pembrolizumab versus Chemotherapy for PD-L1-Positive Non-Small-Cell Lung Cancer. N Engl J Med 2016;375:1823-33. [Crossref] [PubMed]
- Huang C, Wu Y, Fan Y, et al. Camrelizumab umbrella trial based on PD-L1 expression: OS and PFS2 in pre-treated advanced NSCLC. J Thorac Oncol 2021;16:S638-9. [Crossref]
- Zhou C, Wang Y, Zhao J, et al. Efficacy and Biomarker Analysis of Camrelizumab in Combination with Apatinib in Patients with Advanced Nonsquamous NSCLC Previously Treated with Chemotherapy. Clin Cancer Res 2021;27:1296-304. [Crossref] [PubMed]
- Forde PM, Chaft JE, Smith KN, et al. Neoadjuvant PD-1 Blockade in Resectable Lung Cancer. N Engl J Med 2018;378:1976-86. [Crossref] [PubMed]
- Cascone T, William W, Weissferdt A, et al. Neoadjuvant nivolumab (N) or nivolumab plus ipilimumab (NI) for resectable non-small cell lung cancer (NSCLC): Clinical and correlative results from the NEOSTAR study. J Clin Oncol 2019;37:8504. [Crossref]
- Rusch V, Chaft J, Johnson B, et al. Neoadjuvant atezolizumab in resectable non-small cell lung cancer (NSCLC): interim analysis and biomarker data from a multicenter study (LCMC3). J Clin Oncol 2019;37:8503. [Crossref]
- Provencio M, Nadal E, Insa A, et al. Phase II study of neo-adjuvant chemo/immunotherapy for resectable stages IIIA non-small cell lung cancer- NADIM Study-SLCG. J Thorac Oncol 2018;13:abstract Oa01.05.
- Shu CA, Gainor JF, Awad MM, et al. Neoadjuvant atezolizumab and chemotherapy in patients with resectable non-small-cell lung cancer: an open-label, multicentre, single-arm, phase 2 trial. Lancet Oncol 2020;21:786-95. [Crossref] [PubMed]
- Marabelle A, Le DT, Ascierto PA, et al. Efficacy of Pembrolizumab in Patients With Noncolorectal High Microsatellite Instability/Mismatch Repair-Deficient Cancer: Results From the Phase II KEYNOTE-158 Study. J Clin Oncol 2020;38:1-10. [Crossref] [PubMed]
- Le DT, Kim TW, Van Cutsem E, et al. Phase II Open-Label Study of Pembrolizumab in Treatment-Refractory, Microsatellite Instability-High/Mismatch Repair-Deficient Metastatic Colorectal Cancer: KEYNOTE-164. J Clin Oncol 2020;38:11-9. [Crossref] [PubMed]
- Yu Y, Zeng D, Ou Q, et al. Association of Survival and Immune-Related Biomarkers With Immunotherapy in Patients With Non-Small Cell Lung Cancer: A Meta-analysis and Individual Patient-Level Analysis. JAMA Netw Open 2019;2:e196879 [Crossref] [PubMed]
- Li A, Chen Y, Zhang W, et al. Joint association of patients' sex and PD-L1 expression with overall survival benefits and tumor-immune microenvironment in immune checkpoint inhibitors for cancers. Clin Transl Med 2020;10:e92 [Crossref] [PubMed]
- He Z, Li A, Lin D, et al. Association of immune checkpoint inhibitor with survival in patients with cancers with protein tyrosine phosphatase receptor T mutation. Clin Transl Med 2020;10:e214 [Crossref] [PubMed]
- Yu Y, Lin D, Li A, et al. Association of Immune Checkpoint Inhibitor Therapy With Survival in Patients With Cancers With MUC16 Variants. JAMA Netw Open 2020;3:e205837 [Crossref] [PubMed]
- Yu Y, Chen Y, Li A, et al. Novel blood-based tumor mutation algorithm and nomogram predict survival of immune checkpoint inhibitor in non-small-cell lung cancer: Results from two multicenter, randomized clinical trials. Clin Transl Med 2020;10:e53 [Crossref] [PubMed]
- Clouthier DL, Lien SC, Yang SYC, et al. An interim report on the investigator-initiated phase 2 study of pembrolizumab immunological response evaluation (INSPIRE). J Immunother Cancer 2019;7:72. [Crossref] [PubMed]
- Jin Y, Dong H, Xia L, et al. The Diversity of Gut Microbiome is Associated With Favorable Responses to Anti-Programmed Death 1 Immunotherapy in Chinese Patients With NSCLC. J Thorac Oncol 2019;14:1378-89. [Crossref] [PubMed]
- Yu Y, Zhang W, Li A, et al. Association of Long Noncoding RNA Biomarkers With Clinical Immune Subtype and Prediction of Immunotherapy Response in Patients With Cancer. JAMA Netw Open 2020;3:e202149 [Crossref] [PubMed]
- Trebeschi S, Drago SG, Birkbak NJ, et al. Predicting response to cancer immunotherapy using noninvasive radiomic biomarkers. Ann Oncol 2019;30:998-1004. [Crossref] [PubMed]
- Khorrami M, Prasanna P, Gupta A, et al. Changes in CT Radiomic Features Associated with Lymphocyte Distribution Predict Overall Survival and Response to Immunotherapy in Non-Small Cell Lung Cancer. Cancer Immunol Res 2020;8:108-19. [Crossref] [PubMed]
- Garassino MC, Cho BC, Kim JH, et al. Durvalumab as third-line or later treatment for advanced non-small-cell lung cancer (ATLANTIC): an open-label, single-arm, phase 2 study. Lancet Oncol 2018;19:521-36. [Crossref] [PubMed]
- Reck M, Mok TSK, Nishio M, et al. Atezolizumab plus bevacizumab and chemotherapy in non-small-cell lung cancer (IMpower150): key subgroup analyses of patients with EGFR mutations or baseline liver metastases in a randomised, open-label phase 3 trial. Lancet Respir Med 2019;7:387-401. [Crossref] [PubMed]
- Oxnard GR, Yang JC, Yu H, et al. TATTON: a multi-arm, phase Ib trial of osimertinib combined with selumetinib, savolitinib, or durvalumab in EGFR-mutant lung cancer. Ann Oncol 2020;31:507-16. [Crossref] [PubMed]
- Wang GZ, Zhang L, Zhao XC, et al. The Aryl hydrocarbon receptor mediates tobacco-induced PD-L1 expression and is associated with response to immunotherapy. Nat Commun 2019;10:1125. [Crossref] [PubMed]
- Wu YL, Zhang L, Fan Y, et al. Randomized clinical trial of pembrolizumab vs chemotherapy for previously untreated Chinese patients with PD-L1-positive locally advanced or metastatic non-small-cell lung cancer: KEYNOTE-042 China Study. Int J Cancer 2021;148:2313-20. [Crossref] [PubMed]
- Gainor JF, Rizvi H, Jimenez Aguilar E, et al. Clinical activity of programmed cell death 1 (PD-1) blockade in never, light, and heavy smokers with non-small-cell lung cancer and PD-L1 expression ≥50. Ann Oncol 2020;31:404-11. [Crossref] [PubMed]