Left ventricular assist device patient selection: do risk scores help?
Introduction
Mechanical circulatory support (MCS) and left ventricular assist device (LVAD) therapy is increasingly utilized in the advanced systolic heart failure (HF) population as a bridge to transplantation (BTT) or destination therapy (DT). As described in the 6th annual Interagency Registry for Mechanically Assisted Circulatory Support (INTERMACS) report in April 2014, over 12,000 durable implants have now been reported to this United States registry (1). A higher proportion of these are DT implants, increasing from 14.7% in 2006-2009, to 41.6% in 2010-2013. Furthermore, when stratified by INTERMACS profile (Table 1) at the time of implant, sicker patients are known to do less well with MCS (2), creating a shift toward implanting patients earlier in their advanced HF state (1-3). Impressively, actuarial survival of 80% at 1 year and 70% at 2 years has been maintained over the recent era (1).
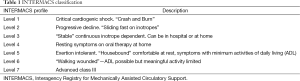
Full table
While INTERMACS classifications provide granularity for categorizing and risk stratifying advanced HF patients, several limitations to this scheme exist. These include, but are not restricted to: lack of input of individual patient features, clinical variability/sub-classifications within a profile, fluctuating levels during a particular patient’s pre-implant course, and subjective assessment by the person reporting the implant characteristics to INTERMACS. Due to the evolution and increasing utilization of MCS technology, and the importance of patient selection to outcomes, many classification schemes have been developed to provide a structure for medical decision making. As clinical experience grows, technology improves, and further favorable clinical characteristics are identified, it is incumbent upon the HF community to continually hone these instruments. The magnitude of such tools cannot be understated when it comes to aiding in the informed consent and shared-decision making process for patients, families, and the healthcare team.
Risk models, or a statistically derived framework to predict outcomes from robust datasets, can be particularly helpful in this process. A robust model will have the power to discriminate (i.e., correctly stratify patients by risk), calibrate (i.e., the degree of agreement between the predicted and observed risk), and will be broadly applicable, with minimal complexity and good external validation (4). In advanced HF, risk models are useful for predicting mortality from HF and for predicting survival after VAD therapy, thereby allowing one to better gauge when the risks of MCS therapy outweigh the risks of death from continued medical therapy of HF. Many of the models that have been applied to patients with advanced HF will be reviewed and referenced here, along with implications for clinical practice and potential future applications of these tools.
Seattle Heart Failure Model (SHFM) (5)
Existing risk models prior to the development and publication of the SHFM in 2006 had limited applicability. Many of these models relied upon invasive means of testing or data gathering (e.g., hemodynamics, peak VO2), hospitalization data, varying medical therapy, or were not well-validated in other databases or populations (5-8). The purpose behind the derivation of the SHFM model was to use easily obtainable clinical and laboratory data in the current era of HF medical and device interventions (excluding MCS).
The SHFM was developed using the cohort from the PRAISE1 study (the Prospective Randomized Amlodipine Survival Evaluation). PRAISE included 1,125 patients with an LVEF ≤30% with NYHA class IIIB-IB HF. Univariate and multivariate predictors of survival from PRAISE and Beta Coefficients (natural log of hazard ratios) from large trial and meta-analyses of medications and defibrillator/biventricular pacemaker therapy were then used to develop a risk score. Further details on these predictors are well-described (5). The mathematical formula for the SHFM is complex, and positive and negative scores can result. To improve ease and applicability, a web-based calculator (http://www.SeattleHeartFailureModel.org) is available that converts a given score to a mortality estimation. The calculator can also serve as an interactive tool to demonstrate the impact of adding or removing certain medications or devices from a patient’s regimen.
The SHFM has been internally and externally validated in a variety of HF populations. In the original study, the SHFM was applied to five other cohorts from varying ambulatory HF study cohorts. The discriminant ability was strong, as defined by the area under the receiver operator characteristic (AUC) of 0.73 (where a score of 0.5 is no better than chance).
Looking closely at the cohorts the SHFM were validated in, patients in the validation cohort were not necessarily reflective of an advanced HF population for whom MCS is being considered. The patients were mostly Caucasian clinical trial subjects from a wide range of New York Heart Association classes, ages, left ventricular ejection fractions (1/3 of patients in one validation cohort had an LVEF of >40%). Furthermore, the era in which the study cohorts were obtained did not necessarily include beta blockers, aldosterone antagonists, and biventricular pacemaker/defibrillators as standard of care. These limitations, as well as the lack of racial diversity in the original SHFM cohorts, may limit the applicability of SHFM to a more heterogeneous, advanced HF population (9).
The applicability of SHFM was tested in 445 patients referred for cardiac transplantation (9). In patients with advanced HF, the SHFM offered adequate discrimination, but underestimated absolute risk. This was particularly true for black patients and those who went on to receive MCS therapy. The authors theorized that this may have been due to the aforementioned limitations, and that the majority of the serious adverse events (LVAD/death/urgent transplantation) in the original SHFM study were driven by death. When restricting the outcome of the model in their population to death alone, the authors found that SHFM performed much better, pointing to judicious use of risk models when a combined endpoint is involved (9). Nevertheless, other analyses of the SHFM have shown its discriminatory ability to risk stratify outcomes in advanced HF patients (10,11). The model can be viewed to have high positive predictive value. However, the SHFM may miss patients with advanced HF who actually have higher than predicted mortality risk and therefore benefit from MCS therapy. The SHFM also does not predict survival after MCS therapy, which is largely influenced by operative risk and complications encountered during MCS.
Destination Therapy Risk Score (DTRS) (12)
LVAD support for DT was first investigated in the landmark Randomized Evaluation of Mechanical Assistance in the Treatment of Congestive Heart Failure (REMATCH) trial (13). In this trial, end-stage HF patients not deemed to be transplant candidates supported with LVAD therapy had significantly improved 1-year survival compared to patients receiving maximal medical therapy. The mortality in the LVAD group was driven by a high rate of post-operative mortality, especially in the first 90 days, potentially limiting the utilization of DT. To examine this phenomenon further, Lietz et al. set out to define preoperative predictors in the post-trial era that would identify in-hospital mortality, and then used these risk factors to devise the DTRS (12).
In this study, 222/280 patients consenting to be a part of the DT registry for the HeartMate XVE LVAD were deemed to have enough comprehensive, pertinent data to be included. Because the majority of in-hospital deaths occurred in the first 3 months after surgery, 90-day in-hospital mortality was chosen as the primary end point. Univariate and multivariate analyses then uncovered 9 variables that were used to derive a weighted risk score (ranging from 0 to 27), based on each variable’s odds ratio (Table 2). The predicted and observed 90-day in-hospital mortality was then calculated for each decile to create four operative risk categories with corresponding mortality rates (12). The authors report good discrimination (based on an AUC of 0.89) and good calibration (by the Hosemer-Lemeshow statistic χ2=4.5, P=0.81). However, they also acknowledged limitations to the risk score, and the potential lack of applicability to the newer generation, continuous-flow (CF) devices (12).
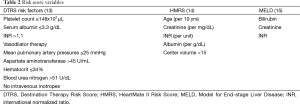
Full table
Over the next few years, several studies showed improved durability and better patient outcomes with CF support compared with that of pulsatile devices (16-20). In addition to improved durability, several factors are believed to account for improved outcomes on CF support, including better patient selection, enhanced surgical techniques, and improved pre- and post-operative care.
Given the evolution of technology, the factors discussed above, and the fact that the DTRS was derived in patients not considered for transplant, many began to question the applicability of the DTRS in the modern VAD era, and to those who were felt to be transplant candidates. A single center analysis by Schaffer et al. showed that the SHFM and the Acute Physiology and Chronic Health Evaluation II (APACHE II) scores had better predictive ability than the DTRS in predicting outcome after MCS (21). In a larger analysis, Teuteberg et al. examined risk prediction of the DTRS in over 1,100 patients enrolled in the HeartMate II (HMII) DT and BTT trials (16,18,22). Compared to the DTRS cohort, the CF trial cohorts clearly presented a shift toward implanting less sick patients. While the 90-day in-hospital mortality was well-delineated by the DTRS between high and low risk groups, the DTRS could not discriminate between the low and medium risk cohorts, or between risk groups of BTT patients (22). More specifically, the DTRS could only moderately predict survival for the BTT + DT and DT groups (AUC, 0.56). Additionally, the highest risk DT patients had a considerably better 1-year survival (62% vs. <30%) than in the original DTRS paper (12,22). These results obviated the need for an improved risk prediction model with better discriminatory capability more reflective of the current CF era.
HeartMate II Risk Score (HMRS) (14)
The HMRS was derived to address risk prediction in the CF era. Patients from both HMII BTT and DT trials were combined and randomly divided into two cohorts. Baseline clinical, pre-operative laboratory and cardiopulmonary hemodynamic measurements were obtained ≤48 hrs before implant. Clinically relevant markers of risk from other LVAD risk models (12,14,15,23-25) were also incorporated in the multivariate analysis. Further details on the derivation and validation of the HMRS are well-described (14).
Characteristics of the validation and derivation cohorts were similar. On multivariable analysis, correlates of 90-day mortality were age, serum creatinine, albumin, international normalized ratio (INR) (off warfarin), and implanting center volume. When compared to DTRS and Model for End-stage Liver Disease (MELD) scores, the HMRS had better discriminatory capability in the total HMII population (Figure 1), and when stratified by device indication (BTT vs. DT). The HMRS performed less well when the HMII validation cohort alone was evaluated (AUC, 0.64), and it was no better than the MELD score in this cohort. In retrospective data review, patients in the validation cohort had a higher frequency of death from VAD related complications (including infection and stroke) than the derivation cohort which may account for lower HMRS discrimination. The HMRS was also shown to be predictive of long-term survival after LVAD implant, but this was also largely driven by the ability of the model to predict operative survival. In summary, the HMRS provides a more contemporary risk model in the CF era, regardless of device intent, with a less complex set of variables (5 vs. 9) than the DTRS (Table 2).
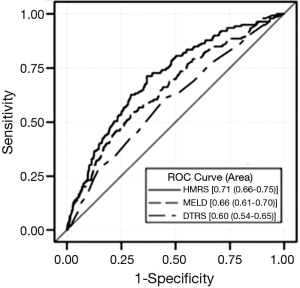
To further characterize its applicability to a non-clinical trial population, the Columbia University group applied the HMRS to its population of HMII LVADs implanted between 2004 and 2012 (26). Pre-operative data from 201 patients were analyzed, and HMRS did not discriminate mortality at this high volume center institution. Their mortality data with 85% 1-year survival was similar to that reported in the post-U.S. Food and Drug Administration Approval Study (27), and the control arm of the ADVANCE HeartWare HVAD trial (28). While the score appeared to discriminate survival in sicker patients (e.g., INTERMACS profiles 1-2), it performed less well in the less sick. The authors acknowledge that the high volume they experience, the non-clinical trial nature of patients, a large number of patients implanted off of temporary forms of support, and the shift to implanting less sick patients may account for the lack of discrimination in a score derived from clinical trial patients.
Model for End-stage Liver Disease (MELD) score (15)
The modified MELD has taken a role in risk prediction in MCS candidates. Originally derived for patients being considered for transhepatic portosystemic shunts and later for liver transplant candidate categorization, the modified MELD score [MELD =9.5 (Ln creatinine) +11.2 (Ln INR) +3.78 (Ln bilirubin) +6.43 with Cr upper limit of 4.0 and Bili/INR lower limit of 1.0] is comprised of makers of multisystem dysfunction commonly encountered in patients with advanced HF (Table 2). In a study at the University of Michigan, preoperative MELD scores were calculated in 211 MCS candidates and were shown to correlate with operative bleeding risk (each 5 unit increase in MELD score was associated with 15±3.8 units of total blood product exposure) following MCS implant (pulsatile and nonpulsatile devices). Further, when survival was examined in the University Michigan and INTERMACS (n=324) cohorts, each 5 unit increase in preoperative MELD score was associated with a 20% increase in mortality. For values ≥17, mortality in both cohorts was 2.5 times higher than patients with lower preoperative scores (15). Others have shown worse outcome with higher MELD scores in pure CF VAD cohorts (29,30). However, like the other risk models discussed above, the MELD score has limitations, and we can again anticipate these to be greatest in a “less ill cohort”. The score fails to take into account other known correlates of risk in MCS candidates, including age and INTERMACS profile, and serum creatinine in those who are malnourished can vastly underestimate renal dysfunction. Finally, all the models discussed herein use patient preoperative INR (a median of 1.1 in the HMRS cohort), obtained within 48 hours of device implant. Score risk predictions will only be accurate if the INR reflects a patient’s coagulopathic state upon operating room entry, and estimated INR values (e.g., trial medians) may need to be used if risk models are employed in the outpatient setting.
Predicting right ventricular (RV) failure
RV failure confers high morbidity and mortality after LVAD implant. In INTERMACS, patients requiring RVAD support had 2.5 higher early mortality than non-RVAD supported patients (1). RV failure is also associated with increased risks for bleeding, renal failure, and longer hospital lengths of stay (31). Presently, at least 6 risk scores devised to predict RV failure post-LVAD implantation have been developed and have been the subject of prior review (32). The various scores include clinical (requirement for inotrope, vasopressor, ventilator, and/or intra-aortic balloon pump support), laboratory (serum creatinine, bilirubin, hepatic transaminases), hemodynamic [right atrial pressure (RA) and RA/wedge pressure ratio, RV stroke work index], and/or echocardiographic (severe tricuspid regurgitation, severe RV dysfunction) data to assess a candidate’s risk for RV failure. However, risk discrimination of the various scores remain poor and no single risk score or echo parameter offers the sensitivity or specificity needed to confidently predict need for biventricular assist device support in the pre-VAD setting.
Discussion and future directions
As the prevalence of HF rises, so will the potential need for advanced HF therapies. In the relatively young field of MCS, advancing technology, improving surgical techniques, and increasing clinical experience have all led to dissemination of the therapy with improved outcomes. Regardless, the current era of MCS has demonstrated no recent change in actuarial survival (1). Until further developments are made in this continually evolving field, the need for appropriate patient selection is fueled by our continued knowledge that the less sick do better (1,2).
As outlined here, many risk models have attempted to address which groups of patients will be successful with MCS support. These models vary from using specific medicine doses and types of devices, extrapolated effects of particular devices, exact laboratory and cardiopulmonary hemodynamic data, and even antiquated LVADs that are no longer available for use. Other available CF LVADs on the market are just now being examined with regard to the extrapolation of these scores (33). While more contemporary risk models provide good discrimination between varying risk levels, they also often use clinical trial patients who may have only had purely advanced HF, often without the confounding variables of other significant comorbidities. For example, these models do not consider clinically relevant conditions such as frailty (34), chronic obstructive pulmonary disease, dementia, patient motivation, psychosocial parameters, or financial difficulties that may impact adherence. Promising work is being done using Bayesian analyses to help define both mortality and other outcomes (35). Yet even more importantly, these models to date focus on short term mortality and not long term survival or quality of life. In addition to adjusting to life with a driveline, batteries, and without a bath, the frequency of re-hospitalization after LVAD and other potential long-term complications such as bleeding, stroke, and RV failure must be contemplated. The advantages and limitations of the risk scores reviewed in this manuscript are summarized in Table 3.
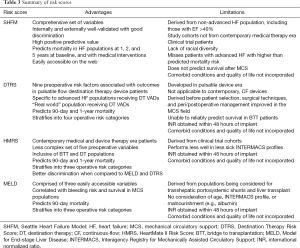
Full table
However, risk models can provide a starting point for discussion with patients, caregivers, and the healthcare team to participate in a patient-centered model of care. While many argue a risk prediction tool is not needed for clinicians who implant devices, these tools may be beneficial during the informed consent and education process of patients and families. Discussing estimated mortality risks for continued HF medical management vs. devices therapy using patient specific data may help patients understand why MCS therapy is or is not in their best interest. Taking all of this under consideration, models are never meant to replace clinical judgment, but to act merely as adjunctive tools in the decision-making process. With promising technologies on the horizon and emerging data indicating there may be a role for MCS therapy in even less sick patients (36-38), continually re-evaluating our tools for patient selection and shared-decision making with new medical, interventional, surgical, and device management therapies will be paramount to the future.
Acknowledgements
None.
Footnote
Conflicts of Interest: The authors have no conflicts of interest to declare.
References
- Kirklin JK, Naftel DC, Pagani FD, et al. INTERMACS Annual Report: A 10, 000-patient database. J Heart Lung Transplant 2014;33:555-64. [PubMed]
- Boyle AJ, Ascheim DD, Russo MJ, et al. Clinical outcomes for continuous-flow left ventricular assist device patients stratified by pre-operative INTERMACS classification. J Heart Lung Transplant 2011;30:402-7. [PubMed]
- Kirklin JK, Naftel DC, Stevenson LW, et al. INTERMACS Database for durable devices for circulatory support: first annual report. J Heart Lung Transplant 2008;27:1065-72. [PubMed]
- Moons KG, Kengne AP, Woodward M, et al. Risk prediction models: I. Development, internal validation, and assessing the incremental value of a new (bio)marker. Heart 2012;98:683-90. [PubMed]
- Levy WC, Mozaffarian D, Linker DT, et al. The Seattle Heart Failure Model: prediction of survival in heart failure. Circulation 2006;113:1424-33. [PubMed]
- Aaronson KD, Schwartz JS, Chen TM, et al. Development and prospective validation of a clinical index to predict survival in ambulatory patients referred for cardiac transplant evaluation. Circulation 1997;95:2660-7. [PubMed]
- Fonarow GC, Adams KF Jr, Abraham WT, et al. Risk stratification for in-hospital mortality in acutely decompensated heart failure: classification and regression tree analysis. JAMA 2005;293:572-80. [PubMed]
- Koelling TM, Joseph S, Aaronson KD. Heart failure survival score continues to predict clinical outcomes in patients with heart failure receiving beta-blockers. J Heart Lung Transplant 2004;23:1414-22. [PubMed]
- Kalogeropoulos AP, Georgiopoulou VV, Giamouzis G, et al. Utility of the Seattle Heart Failure Model in patients with advanced heart failure. J Am Coll Cardiol 2009;53:334-42. [PubMed]
- Ketchum ES, Moorman AJ, Fishbein DP, et al. Predictive value of the Seattle Heart Failure Model in patients undergoing left ventricular assist device placement. J Heart Lung Transplant 2010;29:1021-5. [PubMed]
- Levy WC, Mozaffarian D, Linker DT, et al. Can the Seattle heart failure model be used to risk-stratify heart failure patients for potential left ventricular assist device therapy? J Heart Lung Transplant 2009;28:231-6. [PubMed]
- Lietz K, Long JW, Kfoury AG, et al. Outcomes of left ventricular assist device implantation as destination therapy in the post-REMATCH era: Implications for patient selection. Circulation 2007;116:497-505. [PubMed]
- Rose EA, Gelijns AC, Moskowitz AJ, et al. Long-term mechanical left ventricular assistance for end-stage heart failure. N Engl J Med 2001;345:1435-43. [PubMed]
- Cowger J, Sundareswaran K, Rogers JG, et al. Predicting survival in patients receiving continuous flow left ventricular assist devices. J Am Coll Cardiol 2013;61:313-21. [PubMed]
- Matthews JC, Pagani FD, Haft JW, et al. Model for end-stage liver disease score predicts left ventricular assist device operative transfusion requirements, morbidity, and mortality. Circulation 2010;121:214-20. [PubMed]
- Slaughter MS, Rogers JG, Milano CA, et al. Advanced heart failure treated with continuous-flow left ventricular assist device. N Engl J Med 2009;361:2241-51. [PubMed]
- Kirklin JK, Naftel DC, Kormos RL, et al. Third INTERMACS annual report: the evolution of destination therapy in the United States. J Heart Lung Transplant 2011;30:115-23. [PubMed]
- Miller LW, Pagani FD, Russell SD, et al. Use of a continuous-flow device in patients awaiting heart transplantation. N Engl J Med 2007;357:885-96. [PubMed]
- Pagani FD, Miller LW, Russell SD. Extended mechanical circulatory support with a continuous-flow rotary left ventricular assist device. J Am Coll Cardiol 2009;54:312-21. [PubMed]
- John R, Naka Y, Smedira NG. Continuous flow left ventricular device outcomes in commercial use compared with the prior clinical trial. Ann Thorac Surg 2011;92:1406-13, discussion 1413. [PubMed]
- Schaffer JM, Allen JG, Weiss ES, et al. Evaluation of risk indices in continuous-flow left ventricular assist device patients. Ann Thorac Surg 2009;88:1889-96. [PubMed]
- Teuteberg JJ, Ewald GA, Adamson RM, et al. Risk assessment for continuous flow left ventricular assist devices: does the destination therapy risk score work? J Am Coll Cardiol 2012;60:44-51. [PubMed]
- Kirklin JK, Naftel DC, Kormos RL, et al. The Fourth INTERMACS annual report: 4,000 implants and counting. J Heart Lung Transplant 2012;31:117-26. [PubMed]
- Rao V, Oz MC, Flannery MA, et al. Revised screening scale to predict survival after insertion of a left ventricular assist device. J Thorac Cardiovasc Surg 2003;125:855-62. [PubMed]
- Lietz K, Long JW, Kfoury AG, et al. Impact of center volume on outcomes of left ventricular assist device implantation as destination therapy: analysis of the Thoratec HeartMate Registry, 1998 to 2005. Circ Heart Fail 2009;2:3-10. [PubMed]
- Thomas SS, Nahumi N, Han J, et al. Pre-operative mortality risk assessment in patients with continuous-flow left ventricular assist devices: Application of the HeartMate II risk score. J Heart Lung Transplant 2014;33:675-81. [PubMed]
- Starling RC, Naka Y, Boyle AJ, et al. Results of the post-U.S. Food and Drug Administration-approval study with a continuous-flow left ventricular assist device as a bridge to heart transplantation: a prospective study using the INTERMACS (Interagency Registry for Mechanically Assisted Circulatory Support). J Am Coll Cardiol 2011;57:1890-8. [PubMed]
- Aaronson KD, Slaughter MS, Miller LW, et al. Use of an intrapericardial, continuous-flow centrifugal pump in patients awaiting heart transplantation. Circulation 2012;125:3191-200. [PubMed]
- Yang JA, Kato TS, Shulman BP, et al. Liver dysfunction as a predictor of outcomes in patients with advanced heart failure requiring ventricular assist device support: Use of the Model of End-stage Liver Disease (MELD) and MELD eXcluding INR (MELD-XI) scoring system. J Heart Lung Transplant 2012;31:601-10. [PubMed]
- Eisen HJ. The MELD Scoring System and the Prediction of Outcomes in Heart Failure Patients: What we have learned from the hepatologists. J Am Coll Cardiol 2013;61:2262-3. [PubMed]
- Romano MA, Cowger J, Aaronson KD, et al. Diagnosis and Management of right sided heart failure in subjects supported with left ventricular assist devices. Curr Treat Options Cardiovasc Med 2010;12:420-30. [PubMed]
- Kalogeropoulos AP, Kelkar A, Weinberger JF, et al. Validation of clinical scores for right ventricular failure prediction after implantation of continuous-flow left ventricular assist devices. J Heart Lung Transplant 2015;34:1595-603. [PubMed]
- Castle L, Aaronson K, Slaughter M, et al. Risk Assessment for HeartWare HVAD Support as a Bridge to Transplant: Is the HeartMate II Risk Score Applicable? J Heart Lung Transplant 2015;34:S24.
- Dunlay SM, Park SJ, Joyce LD, et al. Frailty and outcomes following implantation of left ventricular assist device as destination therapy. J Heart Lung Transplant 2014;33:359-65. [PubMed]
- Loghmanpour NA, Kanwar MK, Druzdzel MJ, et al. A New Bayesian Network-Based Risk Stratification Model for Prediction of Short-Term and Long-Term LVAD mortality. ASAIO J 2015;61:313-23. [PubMed]
- Teuteberg JJ, Kittleson M, Cowger J, et al. Prediction of Events in Patients with Advanced Heart Failure: Application of the Seattle Heart Failure Model to the MEDAMACs Population. J Heart Lung Transplant 2012;31:S63.
- Early Implantation: Is It Too Soon? International Society for Heart and Lung Transplantation, Annual Meeting. Nice, France. April 2015.
- Estep JD, Starling RC, Horstmanshof DA, et al. Risk Assessment and Comparative Effectiveness of Left Ventricular Assist Device and Medical Management in Ambulatory Heart Failure Patients (ROADMAP). J Heart Lung Transplant 2015;34:S80. [PubMed]