Artificial intelligence assisted display in thoracic surgery: development and possibilities
Introduction
Lung cancer has become the leading cause of cancer death globally (1), and lung cancer screening and treatment have always been the research focus. With the popularization of low-dose computed tomography (LDCT) screening, more and more solitary pulmonary nodules (SPNs) have been detected (2). SPNs are potentially malignant and heterogeneous. Therefore, the management of SNPs is particularly important (3-5), among which the accurate preoperative localization of pulmonary nodules is one of the most concerns in thoracic surgery. In recent years, sublobar resection, including segmentectomy, has become one of the main surgical options for SPNs. However, the great variations of bronchovascular anatomy of the lung segment make segmentectomy more difficult than lobectomy (6-9). Therefore, it is essential to clarify the adjacent anatomical relationship of potential malignant nodules (10).
For invasive lung cancer without metastasis, anatomic lobectomy and lymph node dissection based on minimal invasive video-assisted thoracoscopic surgery (VATS) has become the first choice for lung cancer treatment (11,12). However, many intraoperative accidents related to VATS have also been reported, including vascular injury and hemorrhage, adjacent structural damage, pleural adhesion (13-16), among which the intrathoracic vascular injury, especially pulmonary artery (PA) hemorrhage, is the most critical (17,18). Preoperatively localizing lung nodules precisely, intraoperatively identifying bronchovascular structures accurately, and anticipating complications in advance require a clear and visual display of individuals’ specific anatomy for surgical simulation and assistance. With the times, the surgical management for lung cancer needs to be improved, and the difficulties mentioned above might be overcome with the help of new emerging technology, artificial intelligence (AI) (19,20).
AI can be defined as utilizing computer programs to simulate independent and intelligent behavior comparable to a human (21). AI behaves with varying levels of autonomy. According to the definition, three-dimensional (3D) reconstruction, virtual reality (VR), augmented reality (AR), and mixed reality (MR) all could independently and automatically carry out construction or projection of surgical images and models based on medical imaging without artificial assistance were classified as general AI technology (22). Relatively, there are narrow AI techniques that cover various subfields. Machine learning (ML) focuses on how computers learn independently without additional programming. It comprises algorithms known as artificial neural network (ANN) that draws inspiration from the biology of the human brain and could be used for prediction after lots of training and validation. The ANN composed of many internal neural layers is also named deep learning (DL). Convolutional neural network (CNN) is one of DL architecture designed to recognize images by analyzing various features in the image, like shape or edges. Recurrent neuron network (RNN) is more suitable for temporal learning of text context. Incorporating RNN within CNN models is good at predicting time series tasks like identifying events sequence in the video. In addition, computer vision (CV) is built up based on a full CNN, which enables computers to learn and predict visual patterns in pictures and videos and is crucial for AI to understand the world (21,23).
In conclusion, the effectiveness of thoracic surgery should be further enhanced, and surgical safety should be ensured. Realizing AI is a promising tool, and we tried to determine the role it plays in thoracic surgical procedures assistance, particularly lung cancer surgery. The CT images and surgical videos are the common clinical data associated closely with thoracic surgery. Here, we focus on the AI-related display for surgical assistance in lung cancer surgery by reviewing AI’s integration with CT and anticipating its combination with surgical videos. This narrative review is based on research material obtained from PubMed up to September 2021. The search terms include “artificial intelligence”, “thoracic surgery”, “three-dimensional reconstruction”, “virtual reality”, “augmented reality”, “mixed reality”, “computer tomography”, “surgery”, and “videos”, etc. Those most relevant and interesting are fully reviewed. In particular, we used search strategy “(artificial intelligence [MeSH Terms]) AND (thoracic surgery [MeSH Terms])". In the last five years, we found that there has been no AI-based research in thoracic surgery videos except for one is based on laryngoscopy and bronchoscopy videos (24). Most surgical AI research focuses on laparoscopic surgery (25-28). The reason may be that the thoracic surgery videos are more difficult for traditional AI to apply than other surgery due to the higher complexity of the procedure. In this review, we illustrate the AI-assisted display technologies based on CT in lung cancer surgery and propose its possible development based on surgical videos in the future.
AI-assisted display based on CT
Chest thin-section CT is a standard technology for identifying lung nodules and their location, as well as individual pulmonary anatomy. However, the output of traditional CT is consecutive two-dimensional images, and it is challenging to visualize specific surgical anatomy. Recently, there are various emerging technologies with AI features that could transform and display CT images in a tridimensional and more intelligent form. As part of AI-assisted surgery, the application of three-dimensional (3D) reconstruction and 3D printed models is becoming popular in thoracic surgery, allowing surgeons to intuitively analyze lung lesions and adjacent anatomical structures, which is conducive to proper surgical planning and reduces surgical risks (29,30). For surgical assistance, the emergence of VR, AR, MR, and other cross-technologies of AI is seen as a disruptive revolution in the display of traditional medical imaging (31-33) (Table 1).
Table 1
Techniques | Descriptions | Applications |
---|---|---|
3D reconstruction/ 3D printing |
Converting anatomical information from thin-section CT to 3D models in virtual; using various materials to build reconstructed 3D models in physical form | Preoperative planning; intraoperative navigation; clinical teaching; doctor-patient communication |
VR | Generating an immersive artificial computer-simulated image and environment with real-time interaction | Surgical planning and simulation; intraoperative navigation; patient education; improving working condition; multi-center joint surgery virtual discussion |
AR | Enhanced version of the real physical world by superimposing a computer-generated image on the view of real life | |
MR | Encompassing both AR and VR, creating a mix of objects where digital and physical interact in real-time |
AI, artificial intelligence; CT, computed tomography; VR, virtual reality; AR, augmented reality; MR, mixed reality.
3D reconstruction /3D printing technology
3D reconstruction technology uses medical imaging data such as thin-section CT (Figure 1A-1C) to reconstruct the pulmonary vessels and bronchi so that the lung anatomy can be presented in a 3D manner (34). It provides powerful technical support for precise segmentectomy by helping locate the lesions, identify targeted blood vessels and bronchus, surgical margins, and reveal anatomical variations (10) (Figure 1D-1F). Prior studies reported that the accurate reconstruction rate of the PA could reach 95.2% (35,36), and the segmentation similarity of current AI models can reach a dice score above 0.80 (37).
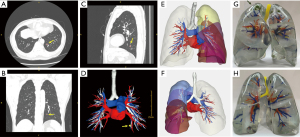
3D printing is an evolving manufacturing technology that uses special materials to build complex 3D structures layer by layer (38). It completed the transformation from virtual conformation to the physical and makes up for the shortcomings of virtual 3D images: lack of realism, unnatural light and shadow performance, poor display under strong light (Figure 1G,1H). Since 3D printing is an extension of 3D reconstruction, it also has the clinical applications of 3D reconstruction. A previous study used a 3D printed model to help identify small lung nodules with a success localization rate of 95.6% (39).
Furthermore, the combined application of 3D-VATS provides surgeons with a good sense of depth in the operative field, and intraoperative navigation by comparing the virtual lung anatomical structures with the real, reducing the operational difficulty, blood loss, and length of operation (30,39-43). In addition to preoperative planning and intraoperative navigation, 3D reconstruction/3D printing is helpful in doctor-patient communication, disease interpretation, and clinical teaching (44). However, high cost hinders the further popularization of this new technology (30).
VR technology
VR helps human generates an immersive, completely artificial computer-simulated image and the environment with real-time interaction. Previous studies demonstrated that VR is feasible for VATS training and assessment, making medical students understand anatomy more intuitively (31,45). The minimally invasive nature of VATS limits the surgical view and reduces tactile feedback. VR simulator allows novice surgeons to train their skills in a simulated, risk-free, and repeatable environment. Besides, it also provides surgeons opportunities to simulate the procedure and explore possible outcomes before surgery, which would help set accurate and safe surgical plans (46). What’s more, VR is also of great value in patient education, allowing patients to understand their anatomical conditions and surgical plans intuitively.
AR technology
In the field of simulation technology, AR is often confused with VR. VR is a computer-generated artificial simulation of a real-life environment or situation, while AR is a technology that superimposes a computer-generated image on the view of real life. The development of AR technology has brought positive possibilities for the reform of thoracic surgery. For example, during the traditional thoracoscopic surgery, surgeons need to look at the monitor in a specific direction and position, which would cause the surgeon to produce undesirable ergonomic effects and cause muscle fatigue, thereby affecting concentration. However, surgeons could receive the image information in a natural posture via AR glasses. Lim et al. (47) systematically compared the ergonomic differences between augmented reality glasses (ARG) and traditional monitors for surgeons. The results of questionnaire evaluation and electromyography test indicate that ARG can help correct surgeons’ poor posture during video-assisted surgery and reduced muscular fatigue of the upper body. More than this, AR performed a good value in the lesion-navigation system (32,48,49) and multi-center joint surgery virtual system (50).
MR technology
MR encompasses both AR and VR creating a mix where digital and physical objects interact in real-time (Figure 2). Due to the advancement in MR, cognition has brought the surgical simulator with enhanced immersion and interactivity to the next level (31,45,46). Wearing MR equipment, surgeons could control the holographic projection through gestures (Figure 2B,2C), which is beneficial for distinguishing the positional relationship between arteries, veins, and lesions (33). A prior study displayed that MR combined with a 3D printing navigational template can be feasible for precisely localizing pulmonary nodules (51,52). With this technology, the surgeon could remove the lesions under direct vision like the scientific movies show (Figure 2D-2F).
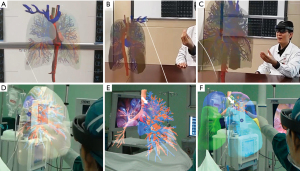
Under the technical background of VR, AR, and MR, the holographic and 3D presentation methods bridge the gap between the virtual world and the real world. It could be of great help to improve the surgeons’ spatial perception, thereby shortening the learning curve for surgeons and improving the efficiency of diagnosis and treatment (33,53).
AI-assisted display based on surgical video
In recent years, the accessibility of big data and the rise of DL potentially enable machines to understand clinical data, particularly surgical videos (23). At present, video-based surgical AI researches show relatively high accuracy in instruments, anatomical structures, and surgical phase recognition in other surgical fields like cataract surgery, gynecological surgery, and laparoscopic surgery (27,54-58) (Table 2), showing the potency of AI in the videos analysis of thoracic surgery. The thoracic procedures videos recorded routinely have accumulated as a large and informative data source. At the same time, little was explored in the qualitative or quantitative analysis regarding surgical context like bleeding in a crucial phase, significant anatomical structure injury, completeness of lymph node dissection, surgical steps and order, and operation duration, etc. (60). The intraoperative information itself remains as a black box for surgical application. And AI serves as a promising tool in facilitating this process (61).
Table 2
Study | Year | Operations | No. of video | Applications | Performance |
---|---|---|---|---|---|
Matava et al. (24) | 2020 | Laryngoscopy and bronchoscopy | 775 | Anatomy classification in real-time | Overall confidence of classification ranges 0.54 to 0.84 |
Kitaguchi et al. (26) | 2020 | Colorectal surgery | 300 | Surgical phase, action, and tool recognition | Accuracy of 81.0%, 83.2%, and 51.2% respectively |
Madad Zadeh et al. (27) | 2020 | Hysterectomy | 461 images | Anatomy detection | Accuracy of 24–97% |
Morita et al. (54) | 2019 | Cataract surgery | 303 | Surgical phase recognition | Mean correct response rate of 96.5% |
Bodenstedt et al. (55) | 2019 | Laparoscopic procedure | 80 | Surgical duration prediction | Overall average error of 37% |
Hashimoto et al. (56) | 2019 | Gastrectomy | 88 | Surgical phase recognition | Accuracy of 82% |
Korndorffer et al. (57) | 2020 | Cholecystectomy | 1,051 | CVS and intraoperative events evaluation | Accuracy of 75%, and 99% |
Mascagni et al. (59) | 2020 | Cholecystectomy | 100 | Formalization of video reporting of CVS | Kappa scores of inter-rater agreements by binary assessment is 0.75 |
Yamazaki et al. | 2020 | Gastrectomy | 52 | Surgical tool detection | Accuracy 86% accuracy |
AI, artificial intelligence; CVS, critical view of safety.
The first application is surgical education and training. Lobectomy and even segmentectomy are complex, difficult for thoracic surgeons in their early careers. AI-based commentary of surgical videos is one of the solutions. The second one is operation quality evaluation. Instead of being empirical to assess one expert’s surgical skill as we do in effect, AI could provide a more precise measurement of surgical quality by identifying how much blood loss, how much time spent in dealing with the bronchovascular stump, how much lymph nodes and stations were dissected, and so on. The third is intraoperative assistance. Though a qualified thoracic surgeon won’t need the help of AI to recognize anatomy, the comprehensive evaluation of disease severity and precise prediction of complications (e.g., bleeding or accidental injure) in real-time for quality assurance would be necessary. Last but not least, the postoperative analysis. Differ from the clinical models that merely consider perioperative variables for prognosis prediction (62), the surgical AI can decode the whole surgical video contexts in a measurable way to identify underlying intraoperative factors (e.g., time in dissecting lymph node or the sequence of different anatomical structure management) that affect prognosis. Quality evaluation of surgery is supposed to be associated with the recovery and mortality of patients. By reviewing previous video-based surgical AI research on other surgery, we propose the possible development of video-based AI-assisted display in thoracic surgery (Table 3).
Table 3
Potential process | Requirements | Applications |
---|---|---|
Construction of videos database | Thoracic surgeons’ experience and insight for clinical issues; consensus on the standardization of database establishment; efforts to construct an open, multi-center database | Surgical education and training; operation quality evaluation; intraoperative assistance; postoperative analysis |
Annotation of pretraining data for AI | Thoracic surgeons’ specific knowledge and involvement; time-consuming, labor-driven manual annotation; formulation of a set of annotation protocols for regulation; developing AI to help with the annotation in turn | |
Identification of instrument and anatomic structure; automated recognition of surgical phases | Training: a lot of annotated pictures was fed to AI program for learning; validation: developed AI is used for testing as compared to the real; a large amount of multi-dimensional image data for analysis; robust and generalized AI algorithms to support |
AI, artificial intelligence.
Construction of database
It is emphasized that AI is still underdeveloped across the overall surgical treatment of lung cancer. Surgeons are uniquely positioned to help drive innovations of AI with surgery rather than passively waiting for the technology to become mature (21). The priority that thoracic surgeons can directly participate in is the construction of clinical data sets developed for surgical AI development. Since the applications of thoracoscopy technology have enabled thoracic surgeons to record surgical videos easily, the videos routinely recorded have accumulated as a large and informative potential AI-training data source (60) for analysis of surgical cases, quality improvement, and education (63-65).
The thoracic surgeons’ experience and insight are demanded for effectively constructing a surgical videos database, from which valuable information could be applied to surgical problem-solving (20). Thoracic surgeons should also make a consensus on the standardization of videos database establishment regarding records, verification, storage, extraction, and use, to ensure the properties of data are compatible (Figure 3A). Most of the research teams conducted preliminary exploration of AI models verification based on their own relatively small-sized database with different metrics for reporting AI performance (25-27). Although those studies demonstrated the superior potential of AI in the surgical application, the data measured with differential criteria may lead to a high risk of bias when we make a decisive conclusion about the AI performance in surgical assistance (23).
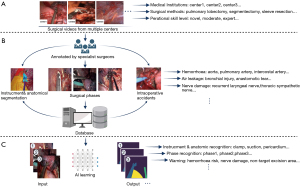
Using single-center data for AI model training can easily lead to overfitting, which reduces the generality and external accuracy of the model. Hashimoto et al. called to establish a large international database of surgical videos for AI training and verification, allowing standardized terms and guidelines for reporting techniques, methods, and results (56). What is more, that would provide researchers with an expansive dataset to test AI models that can explore the effects of intraoperative events, techniques, and postoperative complications. However, this entails massive efforts from thoracic surgeons altogether.
Annotation of pretraining data for AI
It is relatively difficult to make AI identify valuable information from a wide variety of surgical videos data. The first step for AI to exploit useful information to develop applicable models for surgical practice is to annotate the images from surgical videos, such as to label an operative image for classification or outline different kinds of objects and mark their names (Figure 3B). These annotated data are perceived as standard answers by AI, which needs to constantly learn a wide variety of surgical instruments, different anatomical structures, and varied surgical scenarios to improve recognition performance (28). So with the support of large data obtained by manual annotation, AI may eventually be truly intelligent.
Manual labeling of surgical pictures from videos is a time-consuming and laborious task that also requires specific knowledge and the involvement of thoracic surgeons. Thoracic surgeons also need to work together to formulate a set of annotation protocols for regulating this process, as various complicated situations would inevitably occur during surgery. The subjectivity of annotators can distort the data used for AI training, leading to systematic errors in surgical AI applications (21). Although most raw data require time-consuming, labor-driven annotation for being converted into AI-recognizable resources, AI could also help with the labeling process in saving time by improving manual annotation accuracy and speeding up labeling (66). Moreover, AI can also be used to standardize the documentation of surgical videos (59). However, incorrect annotation, variations in illumination, unfocused frames, blood, and smoke in the surgical field could further complicate AI analysis in surgical video and causes bias (23).
Detection and segmentation of instrument and anatomic structure
With the variety of instruments (ultrasonic scalpel, stapler, suction, etc.) and anatomical structure (aorta, pericardium, bronchi and pulmonary vessels, etc.) in VATS, whether AI can identify those different structures in the surgical video is a fundamental issue. The CNN in DL has made this idea a reality (20,21,28). The CNN typically comprises convolutional layers for regional feature extraction from images, pooled layers for degradation of large parameters, and a fully connected layer to output the results, which can be used for object detection and segmentation in the pictures (23,27,67). Instrument and anatomic structure recognition (Figure 3C) is a common application in surgical AI studies (23,25,27). The DL techniques usually follow two stages. The first is the training stage, during which a lot of annotated pictures (segmented from surgical video and its content are annotated) was fed to AI program for learning, and the second is the prediction stage that the developed AI model is used for identifying various objects from the imported video which is previously unseen by AI. The great performance of recognition of instruments and anatomical structures in lung cancer surgery requires a large amount of multi-dimensional image data and excellent AI algorithms to support. In the future, AI may be deployed to carry out more challenging works like real-time anatomical variations.
Automated recognition of surgical phases
Video study is an important subfield of CV that can process digital images and videos (21,56). Like cataract surgery, laparoscopic cholecystectomy, the surgical field has been initially explored for identifying the surgical phases from videos with Video study models and has high accuracy (56,58). Video study models generally include a visual model, CNN, and a temporal model, RNN, of which CNN is used for object feature detection in images (surgical content), and RNN is used to identify the frame sequence (surgical step sequence); the combination of the two can work better for automatic analysis of surgical phase in the videos. The general design flow of AI-recognition of surgical phases is firstly collecting surgical videos for frame segmenting and then mark each frame with the corresponding surgical phase according to the image context by surgeon reviewer. All labeled data is finally divided into a training set, verification set, and testing set for AI learning applications in general.
Lung cancer surgery is complicated and has long been considered an extensive surgery whose surgical phases include multiple steps like the dissection of lymph nodes, bronchi, pulmonary arteries, veins (68,69). This technique would also be expanded to lung cancer surgery in the future. AI will enable easier classification and retrieval of surgical video by indexing so that the unexploited surgical video can be reviewed and analyzed in batch, which offers more potential uses such as video-based surgical learning to improve resident surgical skills, video review-based quality evaluation (56).
Challenges of surgical AI in thoracic surgery
Although the preliminary results of AI applications in surgical fields are promising, it should be noted that some AI algorithms do not yet have perfect robustness and generalization, and the transparency and interpretability of the algorithms are also required to facilitate evaluation (67). In addition, we should also fully consider the particularities of VATS. For example, the anatomy of the lung segment is complex (36,70-72); the frequencies of some special variations are low, which may be neglected by AI due to less relevant training. Furthermore, there are many types of instruments in thoracoscopy, and their shapes are similar. Different centers may have differences in the same kind of devices they use, which increases the modeling difficulty of device separation and is not conducive to the wide application of AI models. Last but not least, there are many surgical procedures for lung cancer, and the surgical approach, sequence, technique, and duration used by different surgical experts may vary greatly. It is difficult for engineers to create a perfect algorithm for understanding all phases of surgical practice. When encountering special intraoperative situations such as blurring the lens, bleeding, smoke, etc., it may also lead to mistakes in AI recognition (56). In all, how to perfectly integrate AI with thoracic surgery is a very challenging topic.
Commentary and conclusions
Localizing lung nodules precisely, identifying bronchovascular structures accurately, and avoiding complications entail a visual display of individuals’ specific anatomy for surgical simulation and assistance. With the support of AI-assisted display technologies, including 3D reconstruction/3D printing, VR, AR, and MR, CT information in thoracic surgery has been fully utilized for realizing the transformation from 2D, static images to 3D, dynamic conformations. These broadly defined AI technologies become powerful assistants in surgical teaching, planning, and simulation. Surgeons will no longer be restricted by the venues and the lack of specimens for learning lung anatomy. They can repeatedly operate in the virtual system to improve learning efficiency. The visual anatomical models enable the surgeons to clarify the adjacent relationship between the lesion and the anatomical variations. It would become an essential basis for the delicate operation in the future.
AI-assisted display based on surgical videos is a new surgical application, which is noteworthy for potentially applying to thoracic surgical education, operation quality evaluation, intraoperative assistance, and postoperative analysis. AI-assisted display based on thoracic surgery videos is still in its infancy and needs to be promoted. By reviewing AI in other surgical fields, we propose the prospect of videos-based AI display for lung cancer surgery assistance and try to illustrate in a flow, including construction of videos database, annotation of surgical data, identification of instrument and anatomic structure, and automated recognition of surgical phases, etc. Lastly, thoracic surgeons should enhance cooperation with AI scientists and consider integrating AI and VATS in multi-dimensions to bring more intelligence into the surgical treatment of lung cancer.
Acknowledgments
Some part of Figure 3 was created with BioRender.com. Thanks for the support of the website.
Funding: None.
Footnote
Provenance and Peer Review: This article was commissioned by the editorial office, Journal of Thoracic Disease for the series “Artificial Intelligence in Thoracic Disease: From Bench to Bed”. The article has undergone external peer review.
Peer Review File: Available at https://dx.doi.org/10.21037/jtd-21-1240
Conflicts of Interest: The authors have completed the ICMJE uniform disclosure form (available at https://dx.doi.org/10.21037/jtd-21-1240). The series “Artificial Intelligence in Thoracic Disease: From Bench to Bed” was commissioned by the editorial office without any funding or sponsorship. JH served as the unpaid Guest Editor of the series and serves as an Executive Editor-in-Chief of Journal of Thoracic Disease. HL served as the unpaid Guest Editor of the series. The authors have no other conflicts of interest to declare.
Ethical Statement: The authors are accountable for all aspects of the work in ensuring that questions related to the accuracy or integrity of any part of the work are appropriately investigated and resolved.
Open Access Statement: This is an Open Access article distributed in accordance with the Creative Commons Attribution-NonCommercial-NoDerivs 4.0 International License (CC BY-NC-ND 4.0), which permits the non-commercial replication and distribution of the article with the strict proviso that no changes or edits are made and the original work is properly cited (including links to both the formal publication through the relevant DOI and the license). See: https://creativecommons.org/licenses/by-nc-nd/4.0/.
References
- Bray F, Ferlay J, Soerjomataram I, et al. Global cancer statistics 2018: GLOBOCAN estimates of incidence and mortality worldwide for 36 cancers in 185 countries. CA Cancer J Clin 2018;68:394-424. [Crossref] [PubMed]
- Arenberg D. Update on screening for lung cancer. Transl Lung Cancer Res 2019;8:S77-87. [Crossref] [PubMed]
- Godoy MC, Naidich DP. Subsolid pulmonary nodules and the spectrum of peripheral adenocarcinomas of the lung: recommended interim guidelines for assessment and management. Radiology 2009;253:606-22. [Crossref] [PubMed]
- Jia Y, Gong W, Zhang Z, et al. Comparing the diagnostic value of 18F-FDG-PET/CT versus CT for differentiating benign and malignant solitary pulmonary nodules: a meta-analysis. J Thorac Dis 2019;11:2082-98. [Crossref] [PubMed]
- National Lung Screening Trial Research Team. Reduced lung-cancer mortality with low-dose computed tomographic screening. N Engl J Med 2011;365:395-409. [Crossref] [PubMed]
- Akiba T, Marushima H, Kamiya N, et al. Thoracoscopic lobectomy for treating cancer in a patient with an unusual vein anomaly. Ann Thorac Cardiovasc Surg 2011;17:501-3. [Crossref] [PubMed]
- Nakamura T, Koide M, Nakamura H, et al. The common trunk of the left pulmonary vein injured incidentally during lung cancer surgery. Ann Thorac Surg 2009;87:954-5. [Crossref] [PubMed]
- Liu X, Zhao Y, Xuan Y, et al. Three-dimensional printing in the preoperative planning of thoracoscopic pulmonary segmentectomy. Transl Lung Cancer Res 2019;8:929-37. [Crossref] [PubMed]
- Zhang Y, Dai K, Chen C, et al. Subdivision and presentation of the pulmonary vasculature of the right upper lobe for anatomical segmentectomy with three-dimensional computed tomography reconstruction. Asian J Surg 2021;44:1023-5. [Crossref] [PubMed]
- Hagiwara M, Shimada Y, Kato Y, et al. High-quality 3-dimensional image simulation for pulmonary lobectomy and segmentectomy: results of preoperative assessment of pulmonary vessels and short-term surgical outcomes in consecutive patients undergoing video-assisted thoracic surgery†. Eur J Cardiothorac Surg 2014;46:e120-6. [Crossref] [PubMed]
- Dai C, Shen J, Ren Y, et al. Choice of Surgical Procedure for Patients With Non-Small-Cell Lung Cancer ≤ 1 cm or > 1 to 2 cm Among Lobectomy, Segmentectomy, and Wedge Resection: A Population-Based Study. J Clin Oncol 2016;34:3175-82. [Crossref] [PubMed]
- Nakazawa S, Shimizu K, Mogi A, et al. VATS segmentectomy: past, present, and future. Gen Thorac Cardiovasc Surg 2018;66:81-90. [Crossref] [PubMed]
- Gonzalez-Rivas D, Stupnik T, Fernandez R, et al. Intraoperative bleeding control by uniportal video-assisted thoracoscopic surgery†. Eur J Cardiothorac Surg 2016;49:i17-24. [PubMed]
- Byun CS, Lee S, Kim DJ, et al. Analysis of Unexpected Conversion to Thoracotomy During Thoracoscopic Lobectomy in Lung Cancer. Ann Thorac Surg 2015;100:968-73. [Crossref] [PubMed]
- Decaluwe H, Petersen RH, Hansen H, et al. Major intraoperative complications during video-assisted thoracoscopic anatomical lung resections: an intention-to-treat analysis. Eur J Cardiothorac Surg 2015;48:588-98; discussion 599. [Crossref] [PubMed]
- Puri V, Patel A, Majumder K, et al. Intraoperative conversion from video-assisted thoracoscopic surgery lobectomy to open thoracotomy: a study of causes and implications. J Thorac Cardiovasc Surg 2015;149:55-61, 62.e1.
- Sawada S, Komori E, Yamashita M. Evaluation of video-assisted thoracoscopic surgery lobectomy requiring emergency conversion to thoracotomy. Eur J Cardiothorac Surg 2009;36:487-90. [Crossref] [PubMed]
- Congregado M, Merchan RJ, Gallardo G, et al. Video-assisted thoracic surgery (VATS) lobectomy: 13 years' experience. Surg Endosc 2008;22:1852-7. [Crossref] [PubMed]
- Etienne H, Hamdi S, Le Roux M, et al. Artificial intelligence in thoracic surgery: past, present, perspective and limits. Eur Respir Rev 2020;29:200010. [Crossref] [PubMed]
- Ostberg NP, Zafar MA, Elefteriades JA. Machine learning: principles and applications for thoracic surgery. Eur J Cardiothorac Surg 2021;60:213-21. [Crossref] [PubMed]
- Hashimoto DA, Rosman G, Rus D, et al. Artificial Intelligence in Surgery: Promises and Perils. Ann Surg 2018;268:70-6. [Crossref] [PubMed]
- Zhou XY, Guo Y, Shen M, et al. Application of artificial intelligence in surgery. Front Med 2020;14:417-30. [Crossref] [PubMed]
- Anteby R, Horesh N, Soffer S, et al. Deep learning visual analysis in laparoscopic surgery: a systematic review and diagnostic test accuracy meta-analysis. Surg Endosc 2021;35:1521-33. [Crossref] [PubMed]
- Matava C, Pankiv E, Raisbeck S, et al. A Convolutional Neural Network for Real Time Classification, Identification, and Labelling of Vocal Cord and Tracheal Using Laryngoscopy and Bronchoscopy Video. J Med Syst 2020;44:44. [Crossref] [PubMed]
- Tokuyasu T, Iwashita Y, Matsunobu Y, et al. Development of an artificial intelligence system using deep learning to indicate anatomical landmarks during laparoscopic cholecystectomy. Surg Endosc 2021;35:1651-8. [Crossref] [PubMed]
- Kitaguchi D, Takeshita N, Matsuzaki H, et al. Automated laparoscopic colorectal surgery workflow recognition using artificial intelligence: Experimental research. Int J Surg 2020;79:88-94. [Crossref] [PubMed]
- Madad Zadeh S, Francois T, Calvet L, et al. SurgAI: deep learning for computerized laparoscopic image understanding in gynaecology. Surg Endosc 2020;34:5377-83. [Crossref] [PubMed]
- Twinanda AP, Shehata S, Mutter D, et al. EndoNet: A Deep Architecture for Recognition Tasks on Laparoscopic Videos. IEEE Trans Med Imaging 2017;36:86-97. [Crossref] [PubMed]
- Zhang Z, Lin J, Chai T, et al. Total superior vena cava reconstruction guided by preoperative three-dimensional (3D)-computed tomography bronchography and angiography. Transl Cancer Res 2020;9:5411-7. [Crossref]
- Kurenov SN, Ionita C, Sammons D, et al. Three-dimensional printing to facilitate anatomic study, device development, simulation, and planning in thoracic surgery. J Thorac Cardiovasc Surg 2015;149:973-9.e1. [Crossref] [PubMed]
- Jensen K, Bjerrum F, Hansen HJ, et al. Using virtual reality simulation to assess competence in video-assisted thoracoscopic surgery (VATS) lobectomy. Surg Endosc 2017;31:2520-8. [Crossref] [PubMed]
- Li C, Zheng B, Yu Q, et al. Augmented Reality and 3-Dimensional Printing Technologies for Guiding Complex Thoracoscopic Surgery. Ann Thorac Surg 2021;112:1624-31. [Crossref] [PubMed]
- Sauer IM, Queisner M, Tang P, et al. Mixed Reality in Visceral Surgery: Development of a Suitable Workflow and Evaluation of Intraoperative Use-cases. Ann Surg 2017;266:706-12. [Crossref] [PubMed]
- Iwano S, Yokoi K, Taniguchi T, et al. Planning of segmentectomy using three-dimensional computed tomography angiography with a virtual safety margin: technique and initial experience. Lung Cancer 2013;81:410-5. [Crossref] [PubMed]
- Fukuhara K, Akashi A, Nakane S, et al. Preoperative assessment of the pulmonary artery by three-dimensional computed tomography before video-assisted thoracic surgery lobectomy. Eur J Cardiothorac Surg 2008;34:875-7. [Crossref] [PubMed]
- Nagashima T, Shimizu K, Ohtaki Y, et al. An analysis of variations in the bronchovascular pattern of the right upper lobe using three-dimensional CT angiography and bronchography. Gen Thorac Cardiovasc Surg 2015;63:354-60. [Crossref] [PubMed]
- Guo W, Gu X, Fang Q, et al. Comparison of performances of conventional and deep learning-based methods in segmentation of lung vessels and registration of chest radiographs. Radiol Phys Technol 2021;14:6-15. [Crossref] [PubMed]
- Yeong WY, Chua CK, Leong KF, et al. Indirect fabrication of collagen scaffold based on inkjet printing technique. Rapid Prototyping Journal 2006;12:229-37. [Crossref]
- Fu R, Chai YF, Zhang JT, et al. Three-dimensional printed navigational template for localizing small pulmonary nodules: A case-controlled study. Thorac Cancer 2020;11:2690-7. [Crossref] [PubMed]
- Liu J, Cui F, Li J, et al. Development and clinical applications of glasses-free three-dimensional (3D) display technology for thoracoscopic surgery. Ann Transl Med 2018;6:214. [Crossref] [PubMed]
- She X, Gu Y, Xu C, et al. Combining 3D-CTBA and 3D-VATS Single-Operation-Hole to Anatomical Segmentectomy in the Treatment of Non-small Cell Lung Cancer. Zhongguo Fei Ai Za Zhi 2017;20:598-602. [PubMed]
- Zhang L, Wang L, Kadeer X, et al. Accuracy of a 3-Dimensionally Printed Navigational Template for Localizing Small Pulmonary Nodules: A Noninferiority Randomized Clinical Trial. JAMA Surg 2019;154:295-303. [Crossref] [PubMed]
- Qiu B, Ji Y, He H, et al. Three-dimensional reconstruction/personalized three-dimensional printed model for thoracoscopic anatomical partial-lobectomy in stage I lung cancer: a retrospective study. Transl Lung Cancer Res 2020;9:1235-46. [Crossref] [PubMed]
- Marro A, Bandukwala T, Mak W. Three-Dimensional Printing and Medical Imaging: A Review of the Methods and Applications. Curr Probl Diagn Radiol 2016;45:2-9. [Crossref] [PubMed]
- Jensen K, Hansen HJ, Petersen RH, et al. Evaluating competency in video-assisted thoracoscopic surgery (VATS) lobectomy performance using a novel assessment tool and virtual reality simulation. Surg Endosc 2019;33:1465-73. [Crossref] [PubMed]
- Ujiie H, Yamaguchi A, Gregor A, et al. Developing a virtual reality simulation system for preoperative planning of thoracoscopic thoracic surgery. J Thorac Dis 2021;13:778-83. [Crossref] [PubMed]
- Lim AK, Ryu J, Yoon HM, et al. Ergonomic effects of medical augmented reality glasses in video-assisted surgery. Surg Endosc 2021; [Epub ahead of print]. [Crossref] [PubMed]
- Grasso RF, Luppi G, Cazzato RL, et al. Percutaneous computed tomography-guided lung biopsies: preliminary results using an augmented reality navigation system. Tumori 2012;98:775-82. [Crossref] [PubMed]
- Grasso RF, Faiella E, Luppi G, et al. Percutaneous lung biopsy: comparison between an augmented reality CT navigation system and standard CT-guided technique. Int J Comput Assist Radiol Surg 2013;8:837-48. [Crossref] [PubMed]
- Khor WS, Baker B, Amin K, et al. Augmented and virtual reality in surgery-the digital surgical environment: applications, limitations and legal pitfalls. Ann Transl Med 2016;4:454. [Crossref] [PubMed]
- Perkins SL, Krajancich B, Yang CJ, et al. A Patient-Specific Mixed-Reality Visualization Tool for Thoracic Surgical Planning. Ann Thorac Surg 2020;110:290-5. [Crossref] [PubMed]
- Fu R, Zhang C, Zhang T, et al. A three-dimensional printing navigational template combined with mixed reality technique for localizing pulmonary nodules. Interact Cardiovasc Thorac Surg 2021;32:552-9. [Crossref] [PubMed]
- Andolfi C, Plana A, Kania P, et al. Usefulness of Three-Dimensional Modeling in Surgical Planning, Resident Training, and Patient Education. J Laparoendosc Adv Surg Tech A 2017;27:512-5. [Crossref] [PubMed]
- Morita S, Tabuchi H, Masumoto H, et al. Real-Time Extraction of Important Surgical Phases in Cataract Surgery Videos. Sci Rep 2019;9:16590. [Crossref] [PubMed]
- Bodenstedt S, Wagner M, Mündermann L, et al. Prediction of laparoscopic procedure duration using unlabeled, multimodal sensor data. Int J Comput Assist Radiol Surg 2019;14:1089-95. [Crossref] [PubMed]
- Hashimoto DA, Rosman G, Witkowski ER, et al. Computer Vision Analysis of Intraoperative Video: Automated Recognition of Operative Steps in Laparoscopic Sleeve Gastrectomy. Ann Surg 2019;270:414-21. [Crossref] [PubMed]
- Korndorffer JR Jr, Hawn MT, Spain DA, et al. Situating Artificial Intelligence in Surgery: A Focus on Disease Severity. Ann Surg 2020;272:523-8. [Crossref] [PubMed]
- Yu F, Silva Croso G, Kim TS, et al. Assessment of Automated Identification of Phases in Videos of Cataract Surgery Using Machine Learning and Deep Learning Techniques. JAMA Netw Open 2019;2:e191860. [Crossref] [PubMed]
- Mascagni P, Fiorillo C, Urade T, et al. Formalizing video documentation of the Critical View of Safety in laparoscopic cholecystectomy: a step towards artificial intelligence assistance to improve surgical safety. Surg Endosc 2020;34:2709-14. [Crossref] [PubMed]
- Hashimoto DA, Rosman G, Rus D, et al. Surgical Video in the Age of Big Data. Ann Surg 2018;268:e47-8. [Crossref] [PubMed]
- Liang H, Yan Z, Guo Y, et al. Comment on "Situating Artificial Intelligence in Surgery A Focus on Disease Severity". Ann Surg 2021;274:e924-5. [Crossref] [PubMed]
- Salati M, Migliorelli L, Moccia S, et al. A Machine Learning Approach for Postoperative Outcome Prediction: Surgical Data Science Application in a Thoracic Surgery Setting. World J Surg 2021;45:1585-94. [Crossref] [PubMed]
- Fard MJ, Ameri S, Darin Ellis R, et al. Automated robot-assisted surgical skill evaluation: Predictive analytics approach. Int J Med Robot 2018; [Crossref] [PubMed]
- Funke I, Mees ST, Weitz J, et al. Video-based surgical skill assessment using 3D convolutional neural networks. Int J Comput Assist Radiol Surg 2019;14:1217-25. [Crossref] [PubMed]
- Ward TM, Mascagni P, Madani A, et al. Surgical data science and artificial intelligence for surgical education. J Surg Oncol 2021;124:221-30. [Crossref] [PubMed]
- Lecuyer G, Ragot M, Martin N, et al. Assisted phase and step annotation for surgical videos. Int J Comput Assist Radiol Surg 2020;15:673-80. [Crossref] [PubMed]
- Roß T, Reinke A, Full PM, et al. Comparative validation of multi-instance instrument segmentation in endoscopy: Results of the ROBUST-MIS 2019 challenge. Med Image Anal 2021;70:101920. [Crossref] [PubMed]
- Bryan DS, Ferguson MK, Antonoff MB, et al. Consensus for Thoracoscopic Left Upper Lobectomy-Essential Components and Targets for Simulation. Ann Thorac Surg 2021;112:436-42. [Crossref] [PubMed]
- Ferguson MK, Bennett C. Identification of Essential Components of Thoracoscopic Lobectomy and Targets for Simulation. Ann Thorac Surg 2017;103:1322-9. [Crossref] [PubMed]
- Nagashima T, Shimizu K, Ohtaki Y, et al. Analysis of variation in bronchovascular pattern of the right middle and lower lobes of the lung using three-dimensional CT angiography and bronchography. Gen Thorac Cardiovasc Surg 2017;65:343-9. [Crossref] [PubMed]
- Zhang M, Mao N, Zhang K, et al. Analysis of the variation pattern in left upper division veins and establishment of simplified vein models for anatomical segmentectomy. Ann Transl Med 2020;8:1515. [Crossref] [PubMed]
- Maki R, Miyajima M, Ogura K, et al. Pulmonary vessels and bronchial anatomy of the left lower lobe. Surg Today 2020;50:1081-90. [Crossref] [PubMed]