A narrative review of artificial intelligence-assisted histopathologic diagnosis and decision-making for non-small cell lung cancer: achievements and limitations
Introduction
Lung cancer is one of the leading causes of cancer-related deaths worldwide (1). Non-small cell lung cancer (NSCLC) is the most frequent type of lung cancer, among which adenocarcinoma (ADC) and squamous cell carcinoma (SCC) account for nearly 85% (2). With the widespread use and technical progress in low-dose computed tomography in screening high-risk populations susceptible to lung cancer, the mortality rate of lung cancer has decreased by 20% (3,4). Notably, although the histopathologic analysis by experienced pathologists is still the gold standard for diagnosing NSCLC and identifying histologic subtypes, it is sometimes difficult to precisely distinguish poorly-differentiated ADC and SCC due to similar morphologic characteristics (5). Subjective or erroneous evaluation of histopathologic images may lead to inappropriate treatment planning, and a corresponding decreased survival in NSCLC patients (6). Moreover, it is time-consuming and challenging for a pathologist to interpret highly complex pathologic images via morphologic evaluation of tissue sections, thus causing histopathologic diagnostics and stratification bias (7,8). Thus, highly sensitive and automatic artificial intelligence (AI) might be implemented to help oncologists make more precise diagnoses of NSCLC and provide more robust evidence for decision-making, which requires limited human intervention.
AI technology is a series of autonomous learning and complex algorithms for recognition, analysis, and predictive results. In the histopathologic diagnosis of digital imaging slides, AI technology provides a new method with which to process medical data that is able to discover high-dimension information, reflecting the underlying pathophysiology that may not be visible to the unaided eye (9). The quantitative features extracted from medical data (10) by AI can improve the objective accurate discrimination of lung cancer subtypes, contributing to the determination of optimal therapeutic strategies in personalized drug treatment and surgical procedures (11). Thus far, several studies have investigated the various AI models based on training sets, and have validated the classification performance of those models, some of which exhibited a performance level similar to experienced pathologists (12). In addition, AI has been shown to be capable of learning the highly complex associations between tumor-related risks and individual prognosis, which will give rise to individualized survival prediction (13).
Therefore, we conducted this systematic review in an attempt to summarize the application, advantages, and limitations of AI in NSCLC diagnosis and decision-making. The aforementioned studies were identified by searching databases, including PubMed, EMBASE, Web of Science, and Cochrane Library, up to February 2021 without language restrictions.
We present the following article in accordance with the Narrative Review reporting checklist (available at https://dx.doi.org/10.21037/jtd-21-806).
The current convolutional neural network (CNN) of AI
AI in healthcare, including machine learning or more advanced deep learning (DL), may be a reality in future clinical practice. Specifically, machine learning approaches have been shown to improve the accuracy and automation of histopathologic image analysis (14), whereas CNNs are currently the state-of-the-art AI architecture for pathologic classification of NSCLC on digital slides (15). The CNN scheme used for image classification consists of several convolutional layers, each followed by a pooling layer and a series of fully connected layers (16,17), as shown in Figure 1. Through inputting images of the entire histopathologic digital slide, the convolutional layers convolve those images and convert them into feature maps (18). Subsequently, the pooling layers are used to down-sample the underlying computation and to reduce the dimensions of the image data (19). Finally, the fully connected layers analyze the output data of convolutional and pooling layers and obtain the classified consequence of the images (18). DL proposes an end-to-end CNN model to automatically learn high-level features from the training set instead of the handcrafting descriptors in machine learning scheme (19), indicating that the DL scheme might be more precise than a conventional machine learning model (20,21).
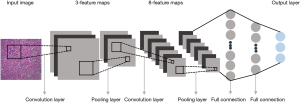
Diagnosing histologic subtypes of NSCLCs based on digital histopathologic slides and gene profiles
Morphologic evaluation of tissue sections remains the basis of histopathologic diagnostics and directs the application of additional analyses (22). Although tumor cell morphology between ADCs and SCCs may be very different, some cannot be easily identified by visual inspection, and most misclassifications are found between the two main histologic types, thus requiring confirmatory immunohistochemistry (IHC) staining (11,23). In contrast, AI based on quantitative image features is a useful tool for reducing misclassification (22,24).
Several studies have determined the ability of AIs to objectively identify NSCLC subtypes, as shown in Table 1 (11,15,25-27). Yu and his colleagues from Harvard Medical School collected 1,600 whole-slide images of ADCs, SCCs, or adjacent normal tissues from The Cancer Genome Atlas (TCGA) and the International Cancer Genome Consortium (ICGC) cohorts to develop and validate the CNN models (15). After the training process, VGGNet and GoogLeNet for distinguishing ADCs from SCCs yielded receiver-operating characteristic (ROC) curves (AUCs) of approximately 0.877–0.927 in both training and test datasets, exhibiting slightly better performance than ResNet and AlexNet (15). The robustness of the aforementioned CNN models was also validated in the ICGC set with 100 image tiles regarded as the largest deviation between the ground truth and the model output (15). The researchers also trained a set of machine learning algorithms for predicting subtypes of NSCLCs, demonstrating that all machine learning models were 13–25% inferior to the CNN classifications in the TCGA test set (15). Additionally, the ability of various architectures of CNN algorithms on histopathologic images across various configurations was evaluated by the Elemento O team using the 4009 IHC or hematoxylin and eosin (H&E)-stained images of ADCs and SCCs (26). After fine-tuning with hundreds of entire-slide histopathologic images as the training data, the performance of differentiating ADCs from SCCs showed that Inception-Fine tune architectures (V1 and V3) provided accuracy, precision, and sensitivity >90% in the Stanford Tissue Microarray Dataset (TMAD), which was significantly superior to other configurations of CNN algorithms (26). The recall, precision and AUC of the Inception-Fine tune architectures (V1 and V3) maintained the superiorities in the TCGA dataset (26). Furthermore, Coudray et al. (11) constructed the robust Inception V3 for distinguishing ADCs, SCCs, and normal tissues with similar results using 2075 whole-slide images from two datasets. The CNN algorithm not only achieved a slightly higher AUC, sensitivity, and specificity comparable to pathologists, but also obtained an AUC of 0.886 for the biopsies (11). Therefore, the AI algorithm offers a powerful translational strategy to differentiate subtypes of NSCLC based on cytology specimens or small biopsies of unresectable tumors. A study conducted by Teramoto et al. (25) included 298 image slides from 76 bronchoscopy or small biopsy samples to train and validate a CNN algorithm. This CNN model for discriminating ADCs and SCCs achieved pathologist-level performance with a classification accuracy of 71.1%. Machine learning algorithms for subtyping NSCLCs were developed in another study (27) from 400 small biopsy specimens using a 3-marker IHC panel (TTF-1, Napsin A, and p40). Of the biopsies, 82.8% were successfully classified as ADCs or SCCs. Notably, although the performance of the machine learning algorithm was superior over the 298-image-based CNN model, more human interactions and a larger sample size were thought to be two major factors for the improved accuracy, which spawned more workload for pathologists.
Table 1
Authors | Publication year | Number of datasets | Number of cases | Number of images | Subtypes (images number) | Training set (images) | Validation set (images) | Test set (images) | Independent test datasets (images) | Classifier | Results | Conclusion | |||
---|---|---|---|---|---|---|---|---|---|---|---|---|---|---|---|
ACC | AUC | SN | SP | ||||||||||||
Coudray et al. (11) | 2018 | 2 | NR | 2075 | TCGA: ADC (n=567), SCC (n=609), normal (n=459); independent test dataset: NR |
1,145 | 245 | 245 | 340 | Inception v3 | Normal: 0.984; ADC: 0.969; SCC: 0.966. |
0.968 | 89% | 93% | CNN model could be a useful tool for classification of ADCs and SCCs, depending on whole-slide images and mutational gene status of NSCLCs |
Teramoto et al. (25) | 2017 | 1 | 76 | 298 | ADC (n=82), SCC (n=125), SCLC (n=91) |
96 | 98 | 104 | 0 | CNN | ADC: 89.0%; SCC: 60.0%; SCLC: 70.3%; total: 71.1%. |
NR | NR | NR | Approximately 71% of the images were classified correctly, which was on par with the accuracy of cytotechnologists and pathologists |
Yu et al. (15) | 2020 | 2 | 1009 | 1600 | TCGA: ADC (n=427), SCC (n=457), normal (n=514); ICGC: ADC (n=87), SCC (n=38), normal (n=77) | 1,280 | 0 | 320 | 202 | AlexNet, GoogLeNet, VGGNet-16, and ResNet | NR | VGGNet: 0.891; ResNet: 0.795; GoogLeNet: 0.863; AlexNet: 0.838 |
NR | NR | The utility of CNNs in classifying the histopathology images of the major types of NSCLCs obtained promising performance |
Khosravi et al. (26) | 2017 | 2 | NR | 4009 | TCGA: ADC (n=1,606), SCC (n=1,543); TMAD: ADC (n=637), SCC (n=223) | 1,629 | 0 | 1,520 | 860 | CNN-basic, Inception V3, Inception V1, Inception-ResNet V2 | CNN-basic: 73%; V3-4000: 80%; V3-12000: 78%; V1-Fine tune: 92%; V2: 75%; V3-Fine tune: 90% |
CNN-basic: 64%; V3-4000: 79%; V3-12000: 76%; V1-Fine tune: 89%; V2: 68%; V3-Fine tune: 87% |
CNN-basic: 73%; V3-4000: 80%; V3-12000: 78%; V1-Fine tune: 92%; V2: 75%; V3-Fine tune: 90% |
NR | Fine-tuned inception architectures provided promising accuracies for distinguishing ADCs and SCCs in both datasets, significantly superior to the other four CNNs with various configurations |
Koh et al. (27) | 2014 | 2 | 400 | 400 | SNUH 1: ADC (n=108), SCC (n=59), other (n=17); SNUH 2: NR; SNUH 3 and SNUBH: NR | 184 | 186 | 30 | 0 | DT and SVM | DT: 72.2%; SVM: 80% |
NR | ADC: 83.3%; SCC: 83.3% |
ADC: 83.3%; SCC: 91.7% |
Machine learning algorithms were effective for subtyping NSCLCs in small biopsies using p63 and/or CK5/6 in addition to the 3-marker IHC panel |
NR, not reported; AI, artificial intelligence; CNN, convolutional neural networks; SVM, Support Vector Machine; DT, Decision Tree; ADC, adenocarcinoma; SCC, squamous-cell carcinoma; NSCLC, non-small cell lung cancer; SCLC, small cell lung cancer; TCGA, The Cancer Genome Atlas cohort; IGCG, the International Cancer Genome Consortium; TMAD, the Stanford Tissue Microarray Dataset; SNUH, Seoul National University Hospital; SNUBH, Seoul National University Bundang Hospital; IHC, immunohistochemistry; ACC, accuracy; SN, sensitivity; SP, specificity.
According to the gene mutation data provided by the Catalogue of Somatic Mutations in Cancer (COSMIC), ADCs and SCCs express significantly different frequencies of recurrent mutant genes, such as epidermal growth factor receptor (EGFR), TP53, KRAS, LRP1B, NFE2L2, and CDKN2A (28-30). A previous study demonstrated that machine learning algorithms for analyzing ambiguous histologic findings in small biopsies using p63 and/or CK5/6, in addition to the 3-marker IHC panel, are more effective for subtyping NSCLCs compared to the 3-marker IHC (27). Predicting NSCLC subtypes by identifying specific genotypes is a potentially powerful method that can be recommended as a special marker for IHC-stained slides. Therefore, several references determined the sensitivity and specificity of genetic markers to discriminate the subtypes of NSCLCs, as shown in Table 2 (31-37). A retrospective study selected the genetic features from 77 ADCs and 73 SCCs to construct the support vector machine (SVM) and random forest (RF) classifier (32). Compared with the RF classifier, which consisted of 260 features, the SVM algorithm had 1,100 features for classifying lung ADC and SCC samples at the transcriptomic level and achieved higher accuracy than the corresponding measurements yielded by the optimal RF classifier. Combining the SVM and RF classifiers with the most important 43 features, the Repeated Incremental Pruning to Produce Error Reduction (RIPPER) algorithm was used to construct the classification rules for discriminating ADCs and SCCs, with near-equal accuracy compared to the optimal SVM/RF classifier. In addition, Podolsky et al. (33) involved 480 NSCLCs from three institutions to evaluate the effectiveness of machine learning algorithms in the task of NSCLC classification at the gene expression level. SVM showed the best results in all datasets, which was regarded as the most appropriate auxiliary tool in predicting NSCLC subsets. Moreover, the current DL-based multi-model method was estimated by Xiao et al. (31) using 162 ADCs from TCGA. This model yielded satisfactory results, achieving an accuracy of 99.20% for distinguishing ADCs from normal, which was significantly superior to single classical classifiers in ADC prediction. Similarly, based on RNA-sequencing data from 180 normal and 673 early-stage ADC tissues, Li et al. (35) identified a gene module that represents the distinguishing characteristics of adenocarcinoma in situ (AIS) as AIS-specific genes in the machine learning pipeline. Machine learning models with 20 selected early ADC signature genes were robust for early ADC diagnosis from normal with approximately 98% accuracy.
Table 2
Authors | Publication year | Number of datasets | Number of cases | Number of genes (total) | Subtypes (cases) | Training set (cases) | Validation set (cases) | Test set (cases) | Independent test datasets (cases) | Classifier | Results | Conclusion | ||||
---|---|---|---|---|---|---|---|---|---|---|---|---|---|---|---|---|
ACC | SN | SP | AUC | Precision | ||||||||||||
Xiao et al. (31) | 2017 | 1 | 162 | 1,385 | TCGA: ADC (n=162) | NR | NR | NR | 0 | DL-based multi-model (KNN, SVM, DT, RF, GBDT) | KNN: 88.00%; SVM: 97.20%; DT: 96.80%; RF: 93.20%; GBDT: 96.80%; majority voting: 97.20%; DL-based method: 99.20% | DT: 97.37% | NR | NR | DT: 98.46% | The DL-based multi-model algorithm could obtain more information to achieve the accuracy of 99.20% for distinguishing ADCs from normal |
Yuan et al. (32) | 2020 | 1 | 150 | 1,100, 260, 43 (n=20,502) | GEO: ADC (n=77), SCC (n=73) | NR | NR | NR | 0 | SVM, RF, RIPPER | SVM: 0.867; RF: 0.880; RIPPER: 0.867 | SVM: 0.987; RF: 0.974; RIPPER: 0.867 | SVM: 0.740; RF: 0.781; RIPPER: 0.872 | NR | SVM: 0.800; RF: 0.772; RIPPER: 0.877 | Analyzing the gene expression dataset of NSCLC subtypes, the RIPPER algorithm yielded the almost equal performance of subtyping NSCLCs compared with the SVM/RF classifier |
Podolsky et al. (33) | 2016 | 3 | 480 | NR | DFCI: ADC (n=139), SCC (n=21), other (n=26), normal (n=17); UMD: ADC (n=86), normal (n=10); BWHD: ADC (n=150), other (n=31) | 235 | 96 | 149 | 0 | KNN, NB, SVM, DT | NR | NR | NR | KNN, k=1: 0.87; KNN, k=5: 0.96; KNN, k=10: 0.97; NB_normal: 0.85; NB_histogram: 0.84; SVM: 0.91; C4.5 DT: 0.92 | NR | Compared with other machine learning algorithms, SVM was the optimal tool in NSCLC morphology classification based on gene expression level evaluation |
Cai et al. (34) | 2015 | 2 | 1,099 | 16 (n=45) | TCGC: ADC (n=126), SCC (n=134); GEO: SCLC (n=28); TCGA: ADC (n=452), SCC (n=359) | 288 | 0 | 811 | 0 | RF and multi-SVMs | Training datasets: 86.54%; Independent datasets: 84.60% | Training datasets: 84.37%; Independent datasets: 85.52% | NR | NR | Training datasets: 66.79%; Independent datasets: 85.94% | The accuracies of multi-SVM model with such 16 top features for diagnosing NSCLC subtypes were 86.54% and 84.6% in the training and test set, respectively |
Li et al. (35) | 2018 | 2 | 853 | 20 (n=107) | TCGA: ADC (n=286), normal (n=59); GEO: ADC (n=387), normal (n=121) | 2/3 of each dataset | 0 | 1/3 of each dataset | 0 | RF, SVM, and ANN | TCGA: 98.68%; GSE68465: 99.51%; GSE10072: 97.91%. | TCGA: 99.28%; GSE68465: 99.95%; GSE10072: 98.05% | TCGA: 95.68%; GSE68465: 92.83%; GSE10072: 97.75% | NR | NR | Machine learning models with twenty ADC signature genes were robust for early ADC diagnosis |
Dong et al. (36) | 2019 | 1 | 369 | 699 | TCGA: ADC (n=369) | NR | NR | NR | 0 | SVM, KNN, LR, RF, gcForest and the ensemble MLW-gcForest | Methylation: 0.751; RNA: 0.689; CNV: 0.645; multi-modal: 0.908 | Methylation: 0.763; RNA: 0.679; CNV: 0.677; Multi-modal: 0.882 | NR | Multi-model: 0.96 | Methylation: 0.771; RNA: 0.659; CNV: 0.675; Multi-modal: 0.896 | MLW-gcForest algorithm had an AUC of 0.96 and an accuracy of 0.908 for ADC staging, better than those achieved by traditional machine learning algorithms |
Yang et al. (37) | 2020 | 2 | 600 | 42, 26, 16 (n=528) | TCGA: ADC (n=470); GSE62182: ADC (n=94); GSE83527: ADC (n=36) | 376 | 94 | 0 | 130 | SVM | NR | NR | NR | TCGA: 0.62; GSE62182: 0.66; GSE83527: 0.63 | NR | The 16‑miRNA signature analyzed by LIBSVM algorithm showed a similar ability to classify ADC pathological stages to that of the combinations of 42 or 26 miRNAs |
NR, not reported; AI, artificial intelligence; DL, deep learning; SVM, Support Vector Machine; KNN, K-nearest neighbors; GBDT, gradient boosting decision trees; LR, logistic regression; RF, Random Forest; DT, Decision Tree; ANN, artificial neural networks; NB, Naive Bayes; RIPPER, Repeated Incremental Pruning to Produce Error Reduction algorithm; ADC, adenocarcinoma; SCC, squamous-cell carcinoma; NSCLC, non-small cell lung cancer; SCLC, small cell lung cancer; TCGA, The Cancer Genome Atlas; GEO, Gene Expression Omnibus; DFCI, Dana-Farber Cancer Institute; UMD, University of Michigan Dataset; BWHD, Brigham and Women’s Hospital Dataset; CNV, copy number variation; AUC, Receiver-operating characteristic (ROC) curve; ACC, accuracy; SN, sensitivity; SP, specificity.
Generally, a number of studies selected large datasets of whole-slide images and gene profiles of NSCLC to train DL models or machine learning models to build robust AI schemes, which were subsequently validated in another independent dataset (15,11,26). Compared with the machine learning algorithms, the CNN algorithms with ever-increasing power might be more suitable to discriminate the heterogeneity of NSCLCs, especially for digital whole-slides. Quantitative images and genetic features by processing technology for diagnosis of NSCLCs had value in improving efficiency, accuracy, and consistency in histopathologic evaluations. Thus, the present findings demonstrate that AI technology serves as a promising diagnostic tool for pathologic subtypes of ADCs in both histologic images and gene profiles.
Predicting pathologic stages of NSCLCs based on gene profiles
Gene expression variants have been reported to have critical roles in tumor progression and metastasis, thus suggesting the feasibility of genetic biomarkers for the detection and classification of NSCLC subsets (37). Although the gene profiles have been identified as predictors of clinical diagnosis or NSCLC outcomes, whether gene profiles can be used as pathologic staging marker has not been established. There have been two studies exploring the sensibility and specificity of genetic markers to discriminate the stages of NSCLCs, as shown in Table 2. Yang and his colleagues (37) selected 16 miRNAs from 600 ADCs to evaluate machine learning algorithms. The resulting classification model demonstrated the ability of machine learning algorithms to accurately differentiate ADC pathologic stages. The SVM with 16 miRNAs had a similar ability to classify ADC pathologic stages to combinations of 26 or 42 miRNAs (37). Furthermore, with the RNA-seq, methylation data, and copy number variation (CNV) of 369 ADCs from TCGA, Dong et al. (36) developed the MLW-gcForest model, a machine learning-based ensemble algorithm, which achieved better classification performance in ADC staging (accuracy, 0.908; precision, 0.896; recall, 0.882; F1, 0.889) incorporating multi-modal data compared with single-modal data. Dong et al. (36) indicated that MLW-gcForest integrating multi-modal genetic data effectively improved the accuracy of ADC staging, which was significantly superior to the traditional machine learning algorithms. Generally, gene mutations play a critical role in tumor progression and tumor phenotypes. Although the expression profiles of early lung cancer signature genes identified in the above-mentioned studies have the ability to predict accurate and robust NSCLC stages, AI technologies should be verified by additional studies compared with conventional clinical variables.
Accuracy in assessing histologic growth patterns of ADCs
The main histologic growth patterns of non-mucinous ADCs were defined as follows (23): lepidic; acinar; papillary; micropapillary; and solid patterns. An increasing body of evidence indicates that ADCs comprise a heterogeneous group of growth patterns, and tumor growth patterns in the excised tumor specimen impact clinical prognosis (38,39). For example, compared with acinar and papillary patterns, micropapillary and solid patterns are associated with worse prognoses (40). Furthermore, it is sometimes difficult to identify the predominant and minor histologic subtypes (38). Thus far, there have been two studies in which AI algorithms were applied to accurate and objective classification of ADC growth patterns (7,12,38,40-43) (Table 3).
Table 3
Authors | Publication year | Number of datasets | Number of cases | Number of images | Subtypes (images) | Training set (images/cells) | Validation set (images/cells) | Test set (images/cells) | Independent test datasets (images/cells) |
Classifier | Results | Conclusion | ||||
---|---|---|---|---|---|---|---|---|---|---|---|---|---|---|---|---|
ACC | SN | SP | AUC | Precision | ||||||||||||
Gertych et al. (38) | 2019 | 3 | 110 | 206 | CSMC: ADC (n=91); MIMW: ADC (n=88); TCGA: ADC (n=27) |
78 | 19 | 109 | 0 | GoogLeNet, ResNet-50 and AlexNet | FT-AlexNet:75.3%; DN-AlexNet-1: 89.90%; GoogLeNet-2: 85.84%; Resnet-50-3: 87.64% |
NR | NR | NR | NR | One of the DN-AlexNets obtained the best performance than other CNNs, with the accuracies of 89.90% for classification involving the five tissue classes in test set |
Wei et al. (12) | 2019 | 1 | NR | 422 | DHMC: ADC (n=422) | 245 | 34 | 143 | 0 | ResNet | NR | NR | NR | Lepidic: 0.988; acinar: 0.970; papillary: 0.993; micropapillary: 0.981; solid: 0.997; benign: 0.988 |
NR | CNN could improve classification accuracy of ADC patterns by automatically pre-screening, superior to pathologists |
Wang et al. (7) | 2020 | 2 | 507 | 639 | TCGA: ADC (n=208); NLST: ADC (n=431) |
12,000 cell nuclei | 1,227 cell nuclei | 1,086 cell nuclei | 0 | Mask R-CNN, Cox proportional hazard prognostic model | 88% in the validation set; 90% in the testing set. | NR | NR | NR | NR | Mask R-CNN extracted and identified 48 cell spatial features, which could predict high-risk group, significantly worse survival than the low-risk group |
AbdulJabbar et al. (41) | 2020 | 2 | 1,070 | 4,599 | TRACERx: NSCLC (n=275); LATTICe-A: ADC (n=4,324) |
16790 H&E cells and 9333 IHC cells | 4219 H&E cells | 5951 H&E cells and 5028 IHC cells | 5082 H&E cells | SCCNN | Lymphocyte: 0.942; tumor: 0.933; other: 0.917; stromal: 0.936 |
Lymphocyte: 0.902; tumor: 0.936; other: 0.853; stromal: 0.898 |
Lymphocyte: 0.982; tumor: 0.930; other: 0.981; stromal: 0.973 |
NR | NR | SCCNN for NSCLCs exhibited high accuracy of single-cell classification in H&E digital slides and T-cell identification in the IHC image slides, respectively |
Wang et al. (40) | 2019 | 3 | NR | 159 | TCGA and NLST: ADC (n=29); SPORE: ADC (n=130) |
29 | 130 | 0 | 0 | DL-based ConvPath software | Lymphocytes: 99.3%; stromal cells: 87.9%; tumor cells: 91.6%; overall: 92.9% |
NR | NR | NR | NR | The overall classification accuracies of the CNN in both datasets were 99.3% for lymphocytes, 87.9% for stromal cells, and 91.6% for tumor cells, respectively |
Teramoto et al. (42) |
2020 | 1 | 60 | 793 | Normal (n=25); malignant (n=35) |
NR | 173 | NR | 0 | PGGAN, DCGAN, ImageNet | ImageNet: 0.810; DCGAN: 0.795; PGGAN: 0.853 |
ImageNet: 0.850; DCGAN: 0.793; PGGAN: 0.854 |
ImageNet: 0.768; DCGAN: 0.797; PGGAN: 0.853 |
NR | NR | PGGAN for cytological specimens improved the classification specificity by 8.5% and the total classification accuracy by approximately 4.3% compared to a CNN model |
Saha et al. (43) | 2021 | 1 | 712 | 712 | TCGA: ADC (n=356); SCC (n=356) |
356 | 160 | 160 | 0 | TilGAN | 0.98 | 0.96 | NR | NR | 0.98 | TilGAN generated the high quality of synthetic pathology images could efficiently classify real TIL and non-TIL patches with improved accuracy |
NR, not reported; CNN, convolutional neural networks; DL, deep learning; SCCNN, sensitive convolutional neural networks; ADC, adenocarcinoma; NSCLC, non-small cell lung cancer; TCGA, The Cancer Genome Atlas; CSMC, Cedars-Sinai Medical Center; MIMW, the Military Institute of Medicine in Warsaw; DHMC, the Dartmouth-Hitchcock Medical Center; NLST, the National Lung Screening Trial project; SPORE, the University of Texas Special Program of Research Excellence; H&E, hematoxylin and eosin; IHC, immunohistochemistry; ACC, accuracy; SN, sensitivity; SP, specificity; GAN, generative adversarial network; PGGAN, progressive growing of GAN; DCGAN, deep convolutional generative adversarial network; TIL, tumor-infiltrating lymphocyte.
To evaluate the CNN algorithms for assessing growth patterns, Gertych et al. (38) used 206 H&E-stained slides diagnosed as primary ADCs from three institutions. One training session with FT-AlexNet, four with DN-AlexNet, and three with GoogLeNet and ResNet-50 were constructed to convert growth patterns from the image slides into the qualitative features, which were all used to recognize five tumor component classifications (acinar, micropapillary, solid, cribriform, and non-tumor areas) in the validation and test datasets. One of the DN-AlexNets (accuracy =89.9%) performed better than the best models of GoogLeNet and Resnet-50 CNNs, which yielded accuracies of 85.84% and 87.64%, respectively (38). Additionally, the accuracies of DN-AlexNet and FT-AlexNet in 5-class classification tasks were 89.9% and 75.3% (38), respectively, thus achieving a pathologist-level performance. Moreover, Wei et al. (12) compared CNN models and pathologists, by randomly partitioning 245 entire-slide images for training, 34 images for developing, and another 143 images for testing from one cohort. ResNet with the deep residual network was used to classify five growth patterns (lepidic, acinar, papillary, micropapillary, and solid) and normal tissues. Interestingly, compared with the best corresponding measurements of 3 pathologists (average kappa score, 0.515; average agreement, 64.8%; robust agreement, 75.4%) with various levels of experience, the CNN model achieved a modestly better performance (average kappa score, 0.525; average agreement, 66.6%; robust agreement, 76.7%) in assessing growth patterns in the 143 testing images. Thus, the ResNet model performed at the pathologist-level classification of histologic patterns on resected ADC slides that was superior to inexperienced pathologists (12). Although semi-quantitative evaluation of histopathologic patterns and the best-characterized histopathologic features in the CNN schemes exhibited the capability to assist in precise decisions regarding oncologic therapy (6), additional proof is needed to prove the robustness and feasibility of AI models due to insufficient evidence.
Discriminating types of stromal cells in the tumor microenvironment (TME)
The cell spatial organization in tumor tissues provides important insight into tumor progression and metastasis, and reveals important information on the TME, including cell growth patterns and the spatial interactions among different types of cells (7). For example, expression of the relevant genes for extracellular matrix organization, which are mainly derived from fibroblasts, is associated with stromal cell density in the tumor tissues (7). Therefore, AI models were applied to automatically convert the entire digital pathologic image to a TME map across the entire slide, in which the features of tumor region and lymphocyte infiltration areas were quantified and extracted to identify TME cells and to predict the pathologic diagnosis (7).
The characteristics and results of the included studies for evaluating AI algorithms for identifying TME cells are shown in Table 3. To determine the ability of the CNN scheme for recognizing pathologic images, Wang et al. (40) manually labeled 11,988 tumors, stroma, and lymphocyte image patches centered at cell nuclei centroids from the region of interest (ROI) boundaries of 29 H&E-stained slides of ADCs in TCGA. Subsequently, the cell nuclei were detected by ConvPath software incorporating the image segmentation, DL, and feature extraction algorithms (40), which were classified into three categories (tumor cell, stromal cell, and lymphocyte) in the National Lung Screening Trial project (NLST) dataset. The overall classification accuracies of the CNN model in two datasets were 99.3% for lymphocytes, 87.9% for stromal cells, and 91.6% for tumor cells. This model was subsequently tested in the University of Texas Special Program of Research Excellence (SPORE) dataset, and yielded similar accuracies (40). Additionally, the data revealed that higher lymphocyte abundance mixed with different types of cells detected by the CNN algorithm was associated with a worse prognosis (40). Similarly, the Mask Regional Convolutional Neural Network (Mask R-CNN) architecture was developed based on >12,000 cell nuclei from 39 ROIs of ADCs in the NLST and TCGA dataset in another study using similar methods (7). The output layer for the Mask R-CNN model classified cell nuclei of the inputting images into six categories (tumor cell, stromal cell, lymphocyte, macrophage, red blood cell, and karyorrhexis), showing that the accuracies for tumor nuclei classification were 88% and 90% in the 1,227 nuclei validation set and the 1086 nuclei testing set, respectively (7). Furthermore, AbdulJabbar et al. (41) developed a sensitive convolutional neural network (SCNN) to spatially profile immune infiltration and discover tumor topologic determinants of immunosuppression in the digital pathology of NSCLCs. This pipeline facilitated spatial mapping of cancer cells, lymphocytes, stromal cells, and other cell types in 375 H&E-stained images to discriminate T cell subsets in 100 CD4/CD8/FOXP3 IHC images for pathologic tumor-infiltrating lymphocyte (TIL) estimates. The data indicated that ADCs with a high number of immune cold regions were at a significantly increased risk of relapse than ADCs with a low number, independent of the number of total regions sampled and the immune phenotypes of other regions. Recently, the classification capability of a new DL architecture for detecting the cytologic pathology images was evaluated by Teramoto et al. (42) and Saha et al. (43), which generated high-quality synthetic images for improving classification performance. The progressive growth of GAN (PGGAN) was proposed by Teramoto et al. (42) and assessed in a 60-patient dataset with 620 cytopathologic images. The overall classification accuracy of the CNN pretrained using PGGAN-generated images for identifying lung tumor cells was 85.3%, which was better than the CNNs pretrained using ImageNet or with a deep convolutional generative adversarial network (DCGAN). The proposed PGGAN for converting cytologic specimens has improved the classification specificity by 8.5% and the accuracy by nearly 4.3%. In a similar study, TilGAN, an efficient generative adversarial network to generate high-quality synthetic pathologic images followed by classification of TIL and non-TIL regions, was structured by Saha et al. (43) to analyze data from 356 ADCs and 356 SCCs. TilGAN for discriminating TIL and non-TIL regions on the entire-slide pathology images achieved an average classification accuracy of 97.83%, a precision of 98.34%, and a recall of 96.49%, showing the usefulness and effectiveness of the proposed GAN.
Therefore, the AI algorithm for nuclei segmentation and cell classification is an effective tool to study the tumor morphologic microenvironment and tumor growth patterns. Quantifying interactions between tumor and stromal cells or lymphocytes could potentially pave a way for predicting immune phenotypes and the immunotherapy response (40). Meanwhile, the capability of AI algorithms for recognizing distinct cells must be further verified, especially for identifying subtypes of T cells in the digital slides.
Prognostic prediction models of AI based on image slides
After surgical treatment for early-stage ADC, patients with stage IB or more advanced disease usually receive adjuvant chemotherapy, increasing the survival rate 5%-10% (44). However, nearly one-half of ADCs had relapses and subsequent disease progression (44,45). Based on a computational approach of AI, the ability to quantify relevant prognostic markers may identify the candidates for adjuvant therapy after pulmonary resection (6). Additionally, several morphometric features from H&E-stained images were significantly associated with pathologic diagnoses and prognoses and not easily identified by human evaluators, but could be detected using AI methods (46).
In a previous study, the ConvPath model automatically learned to identify different nuclei based on the topologic feature maps of TME, including the nucleus centroid, nuclear boundary, or non-nuclei (46). Based on those features, an image feature-based prognostic model was used to divide the patients into high- and low-risk subgroups. After adjusting for clinical variables, including age, gender, smoking status, and stage, the high-risk subgroup was associated with worse survival in both independent cohorts (40). As shown in Table 4 (6,8,9,46-48), a series of studies constructed a prognosis prediction model based on the AI algorithms or the features extracted by the AI pipeline. Yu et al. (6) obtained 2480 H&E-stained slides of 1,311 ADCs and SCCs from two datasets. Seven machine learning models were constructed to distinguish malignancy from normal adjacent tissue based on 15 relevant quantitative image features. Those features were selected to train Net-Cox proportional hazards models for predicting high-risk patients, which were superior to pathologists. Additionally, Luo et al. (9) developed an RF prediction model with 1,034 NSCLCs from the TCGA cohort. This model not only selected the 18 most important features from 943 extracted morphologic features, but also predicted high- and low-risk groups based on the 18 selected features. Moreover, Wang et al. (8) trained a CNN model to automatically extract histopathologic features, reducing the manually labeled and segmented ROIs of digital slides in the testing process. Subsequently, 22 features were used to discriminate ADCs from benign adjacent tissues and to construct a univariate Cox proportional hazard model, which was considered as an objective prognostic model of ADCs superior to other clinical variables. The AI scheme not only successfully visualized the tumor-related features in pathology images, but also could be applied to developing a model for predicting recurrence. Wang et al. (46) used a retrospective cohort, including 70 H&E-stained slides of early-stage NSCLCs to train three machine learning schemes [quadratic discriminant analysis (QDA), linear discriminant analysis (LDA), and SVM], involving the most predictive features associated with disease recurrence (46). The top seven discriminative features were ultimately determined by QDA from 2,242 total corresponding features. Moreover, QDA was also developed as a classifier regarded as the best of three classification models according to the best AUCs for predicting the postoperative recurrence in all cohorts. In addition, QDA yielded accuracies of 81%, 82%, and 75% for prediction of recurrence in cohorts 1, 2, and 3, respectively (46). However, any single features could not be used to successfully predict recurrence or death (49) and these three models were just machine learning algorithms, not the state-of-the-art DL algorithms. Therefore, a more reliable AI scheme with the ability to quantify risks by combining significant clinical characteristics and entire-slide histopathologic image data, is in need to predict clinical outcomes and to provide optimal therapeutic decisions for patients with NSCLC.
Table 4
Authors | Publication year | Number of datasets | Number of cases | Number of images | Features/genes (total) | Subtypes (cases) | Training set (cases) | Validation set (cases) | Test set (cases) | Independent test datasets (cases) | Classifier | Results | Conclusion | |||
---|---|---|---|---|---|---|---|---|---|---|---|---|---|---|---|---|
High vs. low risk | ACC | AUC | SN | |||||||||||||
Wang et al. (8) | 2018 | 2 | 539 | 824 | 22 F (n=22) | NLST: ADC (n=150); TCGA: ADC (n=389) | 150 | 0 | 0 | 389 | Inception (V3), univariate Cox proportional hazard model | 2.25 (1.34–3.77) | Tumor: 88.1%; non-malignant: 93.5%; overall: 89.8% |
NR | NR | Prognostic prediction model with 22 shape features extracted by CNN were considered as an objective prognostic model of ADCs superior to clinical variables |
Yu et al. (6) | 2016 | 2 | 1,311 | 2,480 | 240, 15 F (n=9,879) | TCGA: ADC (n=515), SCC (n=502); TMAD: ADC (n=227), SCC (n=67) | 70% of TCGA | 0 | 30% of TCGA | 294 | NB, SVM, BT, RF; net-Cox proportional hazards models | NR | NR | Bagging: 0.74; Naive bayes: 0.63; RF: 0.75; RF with CITs: 0.73; SVMs with gaussian kernel: 0.75; SVMs with linear kernel: 0.70; SVMs with polynomial kernel: 0.74 | NR | Histopathological classifiers could successfully predict survival outcomes of NSCLCs, superior to pathologists |
Luo et al. (9) | 2017 | 1 | 1,034 | 3,186 | 18 F (n=943) | TCGA: ADC (n=523), SCC (n=511) | 2/3 of TCGA | 0 | 1/3 of TCGA | 0 | RF prediction model | ADC: 2.34 (1.12–4.91); SCC: 2.22 (1.15–4.27) |
NR | NR | NR | The RF model with morphological features of digital slides showed the ability to predict prognosis in NSCLCs |
Wang et al. (46) | 2017 | 3 | 305 | NR | 7 F (n=242) | Cohort 1: ADC (n=17), SCC (n=44), Other (n=9); Cohort 2: ADC (n=51), SCC (n=21), Other (n=47); Cohort 3: ADC (n=54), SCC (n=20), Other (n=41) | 70 | 119 | 0 | 116 | QDA, LDA, SVM | NR | Cohort 1: 81%; Cohort 2: 82%; Batch 1: 75%; Batch 2: 75% |
Cohort 2: 0.84; Batch 1: 0.74; Batch 2: 0.77 |
NR | QDA with nuclear feature of digitized slides of NSCLC biopsies yielded an accuracy of 81%, 82% and 75% for recurrence prediction in cohort 1, 2 and 3 respectively |
Li et al. (47) | 2019 | 2 | 1,463 | – | 16 G (n=2,472) | TCGA: ADC (n=492); GEO: ADC (n=971) | 492 | 232 | 347 | 386 | LASSO; Cox regression | 3.32 (2.11–5.21) | NR | 1-year: 0.822; 2-year: 0.714; 3-year: 0.753. |
NR | The 16-gene-based LASSO model for ADC prognosis prediction was served as a practical and reliable prognosis predictive tool for ADCs |
Yu et al. (48) | 2019 | 1 | 371 | – | 28, 85 G | TCGA: ADC (n=371) | 297 | 0 | 74 | 0 | SVM | NR | EBT_0.10: 73.6%; EBT_0.15: 76.0%; EBT_0.20: 80.0% |
EBT_0.10: 0.710; EBT_0.15: 0.810; EBT_0.20: 0.896 |
EBT_0.10: 93.8%; EBT_0.15: 90.7%; EBT_0.20: 98.5% |
SVM model with the genetic features could well predict the ADC prognosis, much better than the conventional TNM staging system |
NR, not reported; AI, artificial intelligence; LASSO, least absolute shrinkage and selection operator; NB, Naive Bayes; RF, random forest; BT, bagging for classification trees; QDA, Quadratic discriminant analysis; LDA, linear discriminant analysis; SVM, support vector machine; ADC, adenocarcinoma; SCC, squamous-cell carcinoma; NSCLC, non-small cell lung cancer; SCLC, small cell lung cancer; TCGA, The Cancer Genome Atlas; NLST, the National Lung Screening Trial project; TMAD, the Stanford Tissue Microarray dataset; GEO, Gene Expression Omnibus; TNM, tumor, nodes, and metastasis; ACC, accuracy; SN, sensitivity; SP, specificity.
The treatment recommendation of the Watson for Oncology (WFO) system
With the rapid development of medicine in oncology, physicians might be unable to provide the latest therapeutic strategies for patients according to the new research findings and guidelines (50). Therefore, after accurate diagnosis based on AI technologies as mentioned above, the WFO system of AI, a cognitive computing system, was developed for assisting clinicians in providing precise treatment regimens based on the latest evidence and guidelines (51). The WFO system of AI can quickly identify key information in individual medical records, and in surface relevant evidence as well, which might affect patient management from the date of diagnosis to follow-up (51).
Through manually inputting of tumor-related information, WFO can conduct statistical analyses to predict the survival probability and output a personalized treatment recommendation for specific patients (52). A total of 149 patients with primary lung cancer were selected in a retrospective study, and Liu et al. (53) evaluated the consistency between the recommendations of WFO and the actual treatment provided by the multidisciplinary team (MDT). The general consistency was 65.8%, which was significantly affected by two major reasons, including pathologic subtypes and stages. The concordance rates of stage and subtypes are listed (51-54) (Table 5). Compared to patients with stage I–III NSCLCs, patients with stage IV NSCLCs obtained a higher concordance rate of 89% with respect to regimens. Another similar study by You et al. (52) evaluated the concordance rate between the treatment recommendations of 310 NSCLCs by WFO and actual regimens, in which the overall rate for both “recommended (34.52%)” and “for consideration (50.64%)” reached 85.16%. The concordance rates were 87.56% and 79.12% for ADCs and SCCs, respectively, indicating that WFO might still have moderate space to be improved. Furthermore, Yao et al. (54) collected 165 NSCLCs to evaluate the WFO system, achieving an overall consistency rate of 73.30%. The WFO system, studied by Kim et al. (51) using 405 cases with lung cancer, reached a higher overall concordance rate of 92.40%. Additionally, the agreement rates of ADCs and SCCs between MDT and WFO regimens were 94.90% and 90.20%, respectively (54), which were also similar to the Yao study with consistency rates of 90.5% and 90.7% for ADCs and SCCs, respectively. Although WFO yielded excellent performance in these studies, the patient’s physical findings, complications, and finances should be taken into account and WFO must be improved to adapt to the real clinical practice in different countries (55). For example, some targeted drugs recommended by the WFO using the National Comprehensive Cancer Network (NCCN) Clinical Practice guideline were not accessible for 15.27% of the patients in China during the study period of the You study (52). Thus, the WFO is considered to be a counseling adviser for patients with lung cancer and a reference tool for oncologists, the power of which should be further verified in larger cohorts (55).
Table 5
Authors | Publication year | Country | Number of cases (M/F) | Median age (range), years | Subtypes (cases) | Stage I NSCLC | Stage II NSCLC | Stage III NSCLC | Stage IV NSCLC | ADC | SCC | SCLC | Overall |
---|---|---|---|---|---|---|---|---|---|---|---|---|---|
Kim et al. (51) | 2020 | Korea | 405 (340/65) | 71 (37–88) | ADC (n=157); SCC (n=132); SCLC (n=94); Other (n=22) |
NR | NR | NR | NR | 94.90% | 90.20% | 97.90% | 92.40% |
You et al. (52) | 2020 | China | 310 (215/95) | NR | ADC (n=217); SCC (n=91); LC (n=2) |
NR | NR | NR | NR | 87.56% | 79.12% | – | 85.16% |
Yao et al. (54) | 2020 | China | 165 (109/56) | NR | ADC (n=121); SCC (n=43); ASC (n=1) |
Stage ≤III: 77.8% | 93.50% | 90.50% | 90.70% | – | 73.30% | ||
Liu et al. (53) | 2018 | China | 149 (124/25) | 60 (26–83) | ADC (n=61); SCC (n=61); SCLC (n=23); LC (n=1); ASC (n=3) |
83% | 59% | 42% | 89% | NSCLC: 61.1% | 83% | 81.90% |
NR, not reported; MDT, the multidisciplinary team; WFO, Watson for Oncology; ADC, adenocarcinoma; SCC, squamous-cell carcinoma; NSCLC, non-small cell lung cancer; SCLC, small cell lung cancer; ASC, adenosquamous carcinoma; LC, large cell lung cancer.
Comments
The AI algorithms with expert-level performance have been applied in several clinical fields, including classifying NSCLC subtypes on entire-slide images, analyzing the gene profiles of lung tumors, identifying cancer cells in the TME, and making clinical decisions. More importantly, after training with a large dataset, AI technology provides oncologists with an end-to-end analysis tool to use without requiring complex computational knowledge (26). Compared with a machine learning model, the more advanced DL pipeline with minimal human interaction is effective for automatic segmentation instead of manual segmentation. For extracting the medical features, the CNN model also reduces the necessities of hand-craft feature engineering with the help of end-to-end unsupervised DL (11).
Likewise, AI has been extended to find out the cell-free DNA, circulating tumor cells, and platelet RNA for diagnosing NSCLC via detecting the liquid biopsy samples (56-58). Nevertheless, the incorporation of both intensity and proportion of stained tumor cells in the liquid biopsies of NSCLC limited the improving diagnostic accuracy of AI technologies (27). Heretofore, only few studies have investigated the feasibility of applying AI technology to liquid biopsy samples. More robust evidences are warranted to confirm its promising prospect in diagnosing NSCLC.
Of note, AI still struggles with many challenges due to complex clinical situations and technical variables, such as pathologic image parameters, varieties of CNN architecture, patient comorbidities, and tumor heterogeneity. In the CNN scheme, image tiles from thick tissue cuts, regions with uneven slide thicknesses, and out-of-focus image tiles were considered as the major factors resulting in misclassification (15). The reproducibility of the AI models must be taken into considerations and must be further confirmed before applied into clinical daily activities. Although the risk of overfitting of AI models could be reduced by a large amount of data for training (25), most of the aforementioned studies involved inadequate samples, resulting in the presence of overfitting and instability of the AI models. For the WFO system, localization factors and individual elements were considered as the main bias risk for discordance between the actual therapies and treatment recommendations of the WFO (55). For example, regional differences in guidelines and available drugs led to distinct therapeutic experiences, which led to remarkable differences between the eastern and western countries (55). Notably, the performance of AI in the medical domains has been improved persistently to produce accurate and reliable results with more sophisticated designs.
Conclusions
In summary, AI tools have made substantial strides in recent years to interpret massive amounts of data in clinical domains, which are applied to diagnosis, treatment, and prognosis prediction of NSCLCs. Notably, more large-scale randomized controlled studies are warranted to confirm the accuracy, sensitivity and specificity of the AI algorithms, and to compare AI with experienced pathologists due to the complex clinical practices. Undeniably, the application of AI technologies in the field of lung cancer has a promising future.
Acknowledgments
Funding: The study was supported by National Natural Science Foundation of China (82172076); Jiangsu Key Research and Development Plan (Social Development) Project (BE2020653); Suzhou Key Discipline for Medicine (SZXK201803); Suzhou Key Laboratory of Thoracic Oncology (SZS201907); Municipal Program of People’s Livelihood Science and Technology in Suzhou (SS2019061); Discipline construction project of the Second Affiliated Hospital of Soochow University (XKTJ-XK202004); Scientific Program of Suzhou Municipal Health and Health Committee (LCZX202004).
Footnote
Provenance and Peer Review: This article was commissioned by the Guest Editors (Jianxing He and Hengrui Liang) for the series “Artificial Intelligence in Thoracic Disease: From Bench to Bed” published in Journal of Thoracic Disease. The article has undergone external peer review.
Reporting Checklist: The authors have completed the Narrative Review reporting checklist. Available at https://dx.doi.org/10.21037/jtd-21-806
Conflicts of Interest: The authors have completed the ICMJE uniform disclosure form (available at https://dx.doi.org/10.21037/jtd-21-806). The series “Artificial Intelligence in Thoracic Disease: From Bench to Bed” was commissioned by the editorial office without any funding or sponsorship. The authors have no other conflicts of interest to declare.
Ethical Statement: The authors are accountable for all aspects of the work in ensuring that questions related to the accuracy or integrity of any part of the work are appropriately investigated and resolved.
Open Access Statement: This is an Open Access article distributed in accordance with the Creative Commons Attribution-NonCommercial-NoDerivs 4.0 International License (CC BY-NC-ND 4.0), which permits the non-commercial replication and distribution of the article with the strict proviso that no changes or edits are made and the original work is properly cited (including links to both the formal publication through the relevant DOI and the license). See: https://creativecommons.org/licenses/by-nc-nd/4.0/.
References
- Sung H, Ferlay J, Siegel RL, et al. Global Cancer Statistics 2020: GLOBOCAN Estimates of Incidence and Mortality Worldwide for 36 Cancers in 185 Countries. CA Cancer J Clin 2021;71:209-49. [Crossref] [PubMed]
- Shi JF, Wang L, Wu N, et al. Clinical characteristics and medical service utilization of lung cancer in China, 2005-2014: Overall design and results from a multicenter retrospective epidemiologic survey. Lung Cancer 2019;128:91-100. [Crossref] [PubMed]
- National Lung Screening Trial Research Team. Reduced lung-cancer mortality with low-dose computed tomographic screening. N Engl J Med 2011;365:395-409. [Crossref] [PubMed]
- de Koning HJ, van der Aalst CM, de Jong PA, et al. Reduced Lung-Cancer Mortality with Volume CT Screening in a Randomized Trial. N Engl J Med 2020;382:503-13. [Crossref] [PubMed]
- Travis WD, Brambilla E, Riely GJ. New pathologic classification of lung cancer: relevance for clinical practice and clinical trials. J Clin Oncol 2013;31:992-1001. [Crossref] [PubMed]
- Yu KH, Zhang C, Berry GJ, et al. Predicting non-small cell lung cancer prognosis by fully automated microscopic pathology image features. Nat Commun 2016;7:12474. [Crossref] [PubMed]
- Wang S, Rong R, Yang DM, et al. Computational Staining of Pathology Images to Study the Tumor Microenvironment in Lung Cancer. Cancer Res 2020;80:2056-66. [Crossref] [PubMed]
- Wang S, Chen A, Yang L, et al. Comprehensive analysis of lung cancer pathology images to discover tumor shape and boundary features that predict survival outcome. Sci Rep 2018;8:10393. [Crossref] [PubMed]
- Luo X, Zang X, Yang L, et al. Comprehensive Computational Pathological Image Analysis Predicts Lung Cancer Prognosis. J Thorac Oncol 2017;12:501-9. [Crossref] [PubMed]
- Yu KH, Berry GJ, Rubin DL, et al. Association of Omics Features with Histopathology Patterns in Lung Adenocarcinoma. Cell Syst 2017;5:620-627.e3. [Crossref] [PubMed]
- Coudray N, Ocampo PS, Sakellaropoulos T, et al. Classification and mutation prediction from non-small cell lung cancer histopathology images using deep learning. Nat Med 2018;24:1559-67. [Crossref] [PubMed]
- Wei JW, Tafe LJ, Linnik YA, et al. Pathologist-level classification of histologic patterns on resected lung adenocarcinoma slides with deep neural networks. Sci Rep 2019;9:3358. [Crossref] [PubMed]
- She Y, Jin Z, Wu J, et al. Development and Validation of a Deep Learning Model for Non-Small Cell Lung Cancer Survival. JAMA Netw Open 2020;3:e205842. [Crossref] [PubMed]
- Litjens G, Sánchez CI, Timofeeva N, et al. Deep learning as a tool for increased accuracy and efficiency of histopathological diagnosis. Sci Rep 2016;6:26286. [Crossref] [PubMed]
- Yu KH, Wang F, Berry GJ, et al. Classifying non-small cell lung cancer types and transcriptomic subtypes using convolutional neural networks. J Am Med Inform Assoc 2020;27:757-69. [Crossref] [PubMed]
- Gong J, Liu JY, Sun XW, et al. Computer-aided diagnosis of lung cancer: the effect of training data sets on classification accuracy of lung nodules. Phys Med Biol 2018;63:035036. [Crossref] [PubMed]
- Gong J, Liu JY, Jiang YJ, et al. Fusion of quantitative imaging features and serum biomarkers to improve performance of computer-aided diagnosis scheme for lung cancer: A preliminary study. Med Phys 2018;45:5472-81. [Crossref] [PubMed]
- Zhao W, Yang J, Sun Y, et al. 3D Deep Learning from CT Scans Predicts Tumor Invasiveness of Subcentimeter Pulmonary Adenocarcinomas. Cancer Res 2018;78:6881-9. [Crossref] [PubMed]
- Wang J, Chen X, Lu H, et al. Feature-shared adaptive-boost deep learning for invasiveness classification of pulmonary subsolid nodules in CT images. Med Phys 2020;47:1738-49. [Crossref] [PubMed]
- Baldominos A, Cervantes A, Saez Y, et al. A Comparison of Machine Learning and Deep Learning Techniques for Activity Recognition using Mobile Devices. Sensors (Basel) 2019;19:521. [Crossref] [PubMed]
- Qian Y, Qiu Y, Li CC, et al. A novel diagnostic method for pituitary adenoma based on magnetic resonance imaging using a convolutional neural network. Pituitary 2020;23:246-52. [Crossref] [PubMed]
- Kriegsmann M, Haag C, Weis CA, et al. Deep Learning for the Classification of Small-Cell and Non-Small-Cell Lung Cancer. Cancers (Basel) 2020;12:1604. [Crossref] [PubMed]
- Hung YP, Chirieac LR. How should molecular findings be integrated in the classification for lung cancer? Transl Lung Cancer Res 2020;9:2245-54. [Crossref] [PubMed]
- Liu H, Jing B, Han W, et al. A Comparative Texture Analysis Based on NECT and CECT Images to Differentiate Lung Adenocarcinoma from Squamous Cell Carcinoma. J Med Syst 2019;43:59. [Crossref] [PubMed]
- Teramoto A, Tsukamoto T, Kiriyama Y, et al. Automated Classification of Lung Cancer Types from Cytological Images Using Deep Convolutional Neural Networks. Biomed Res Int 2017;2017:4067832. [Crossref] [PubMed]
- Khosravi P, Kazemi E, Imielinski M, et al. Deep Convolutional Neural Networks Enable Discrimination of Heterogeneous Digital Pathology Images. EBioMedicine 2018;27:317-28. [Crossref] [PubMed]
- Koh J, Go H, Kim MY, et al. A comprehensive immunohistochemistry algorithm for the histological subtyping of small biopsies obtained from non-small cell lung cancers. Histopathology 2014;65:868-78. [Crossref] [PubMed]
- Forbes SA, Beare D, Gunasekaran P, et al. COSMIC: exploring the world's knowledge of somatic mutations in human cancer. Nucleic Acids Res 2015;43:D805-11. [Crossref] [PubMed]
- Cancer Genome Atlas Research Network. Comprehensive molecular profiling of lung adenocarcinoma. Nature 2014;511:543-50. [Crossref] [PubMed]
- Zhao W, Yang J, Ni B, et al. Toward automatic prediction of EGFR mutation status in pulmonary adenocarcinoma with 3D deep learning. Cancer Med 2019;8:3532-43. [Crossref] [PubMed]
- Xiao Y, Wu J, Lin Z, et al. A deep learning-based multi-model ensemble method for cancer prediction. Comput Methods Programs Biomed 2018;153:1-9. [Crossref] [PubMed]
- Yuan F, Lu L, Zou Q. Analysis of gene expression profiles of lung cancer subtypes with machine learning algorithms. Biochim Biophys Acta Mol Basis Dis 2020;1866:165822. [Crossref] [PubMed]
- Podolsky MD, Barchuk AA, Kuznetcov VI, et al. Evaluation of Machine Learning Algorithm Utilization for Lung Cancer Classification Based on Gene Expression Levels. Asian Pac J Cancer Prev 2016;17:835-8. [Crossref] [PubMed]
- Cai Z, Xu D, Zhang Q, et al. Classification of lung cancer using ensemble-based feature selection and machine learning methods. Mol Biosyst 2015;11:791-800. [Crossref] [PubMed]
- Li D, Yang W, Zhang Y, et al. Genomic analyses based on pulmonary adenocarcinoma in situ reveal early lung cancer signature. BMC Med Genomics 2018;11:106. [Crossref] [PubMed]
- Dong Y, Yang W, Wang J, et al. MLW-gcForest: a multi-weighted gcForest model towards the staging of lung adenocarcinoma based on multi-modal genetic data. BMC Bioinformatics 2019;20:578. [Crossref] [PubMed]
- Yang Z, Yin H, Shi L, et al. A novel microRNA signature for pathological grading in lung adenocarcinoma based on TCGA and GEO data. Int J Mol Med 2020;45:1397-408. [Crossref] [PubMed]
- Gertych A, Swiderska-Chadaj Z, Ma Z, et al. Convolutional neural networks can accurately distinguish four histologic growth patterns of lung adenocarcinoma in digital slides. Sci Rep 2019;9:1483. [Crossref] [PubMed]
- Tsao MS, Marguet S, Le Teuff G, et al. Subtype Classification of Lung Adenocarcinoma Predicts Benefit From Adjuvant Chemotherapy in Patients Undergoing Complete Resection. J Clin Oncol 2015;33:3439-46. [Crossref] [PubMed]
- Wang S, Wang T, Yang L, et al. ConvPath: A software tool for lung adenocarcinoma digital pathological image analysis aided by a convolutional neural network. EBioMedicine 2019;50:103-10. [Crossref] [PubMed]
- AbdulJabbar K. Geospatial immune variability illuminates differential evolution of lung adenocarcinoma. Nat Med 2020;26:1054-62. [Crossref] [PubMed]
- Teramoto A, Tsukamoto T, Yamada A, et al. Deep learning approach to classification of lung cytological images: Two-step training using actual and synthesized images by progressive growing of generative adversarial networks. PLoS One 2020;15:e0229951. [Crossref] [PubMed]
- Saha M, Guo X, Sharma A. TilGAN: GAN for Facilitating Tumor-Infiltrating Lymphocyte Pathology Image Synthesis With Improved Image Classification. IEEE Access 2021;9:79829-40.
- Liang Y, Wakelee HA. Adjuvant chemotherapy of completely resected early stage non-small cell lung cancer (NSCLC). Transl Lung Cancer Res 2013;2:403-10. [PubMed]
- Crinò L, Weder W, van Meerbeeck J, et al. Early stage and locally advanced (non-metastatic) non-small-cell lung cancer: ESMO Clinical Practice Guidelines for diagnosis, treatment and follow-up. Ann Oncol 2010;21:v103-15. [Crossref] [PubMed]
- Wang X, Janowczyk A, Zhou Y, et al. Prediction of recurrence in early stage non-small cell lung cancer using computer extracted nuclear features from digital H&E images. Sci Rep 2017;7:13543. [Crossref] [PubMed]
- Li Y, Ge D, Gu J, et al. A large cohort study identifying a novel prognosis prediction model for lung adenocarcinoma through machine learning strategies. BMC Cancer 2019;19:886. [Crossref] [PubMed]
- Yu J, Hu Y, Xu Y, et al. LUADpp: an effective prediction model on prognosis of lung adenocarcinomas based on somatic mutational features. BMC Cancer 2019;19:263. [Crossref] [PubMed]
- Yuan M, Zhang YD, Pu XH, et al. Comparison of a radiomic biomarker with volumetric analysis for decoding tumour phenotypes of lung adenocarcinoma with different disease-specific survival. Eur Radiol 2017;27:4857-65. [Crossref] [PubMed]
- Doyle-Lindrud S. Watson will see you now: a supercomputer to help clinicians make informed treatment decisions. Clin J Oncol Nurs 2015;19:31-2. [Crossref] [PubMed]
- Kim MS, Park HY, Kho BG, et al. Artificial intelligence and lung cancer treatment decision: agreement with recommendation of multidisciplinary tumor board. Transl Lung Cancer Res 2020;9:507-14. [Crossref] [PubMed]
- You HS, Gao CX, Wang HB, et al. Concordance of Treatment Recommendations for Metastatic Non-Small-Cell Lung Cancer Between Watson for Oncology System and Medical Team. Cancer Manag Res 2020;12:1947-58. [Crossref] [PubMed]
- Liu C, Liu X, Wu F, et al. Using Artificial Intelligence (Watson for Oncology) for Treatment Recommendations Amongst Chinese Patients with Lung Cancer: Feasibility Study. J Med Internet Res 2018;20:e11087. [Crossref] [PubMed]
- Yao S, Wang R, Qian K, et al. Real world study for the concordance between IBM Watson for Oncology and clinical practice in advanced non-small cell lung cancer patients at a lung cancer center in China. Thorac Cancer 2020;11:1265-70. [Crossref] [PubMed]
- Zou FW, Tang YF, Liu CY, et al. Concordance Study Between IBM Watson for Oncology and Real Clinical Practice for Cervical Cancer Patients in China: A Retrospective Analysis. Front Genet 2020;11:200. [Crossref] [PubMed]
- Best MG, Sol N, In 't Veld SGJG, et al. Swarm Intelligence-Enhanced Detection of Non-Small-Cell Lung Cancer Using Tumor-Educated Platelets. Cancer Cell 2017;32:238-252.e9.
- Qi J, Hong B, Tao R, et al. Prediction model for malignant pulmonary nodules based on cfMeDIP-seq and machine learning. Cancer Sci 2021;112:3918-23. [Crossref] [PubMed]
- Shin H, Oh S, Hong S, et al. Early-Stage Lung Cancer Diagnosis by Deep Learning-Based Spectroscopic Analysis of Circulating Exosomes. ACS Nano 2020;14:5435-44. [Crossref] [PubMed]