Using clinical data to predict obstructive sleep apnea
Introduction
Obstructive sleep apnea (OSA) is a common disorder characterized by repetitive episodes of upper airway collapse during sleep, resulting in abnormal ventilation, intermittent oxygen desaturation, hypercapnia, oxidative stress, and sleep fragmentation (1-3). OSA is an original disease due to its close relationship with coronary artery disease, heart failure, stroke, arrhythmias, hypertension, and metabolic dysfunction (4,5). An estimated 1 billion adults worldwide may have OSA, which poses significant health and economic burdens (6).
Although polysomnography (PSG) is widely accepted as the gold standard diagnostic tool for OSA and co-existing sleep disorders (7), it is a time-consuming and labor-intensive procedure. Hence, a wide range of risk factors, such as habitual snoring, nocturnal choking, daytime sleepiness, enlarged tonsils, and body size parameters, have been used to establish various clinical prediction algorithms, questionnaires, and clinical tools for prospectively identifying individuals who are at increased risk for OSA (8-13). Risk factors associated with OSA and predictive accuracy have varied across studies (14,15). Thus, it is necessary to take advantage of comprehensive clinical data from patients with and without OSA to construct appropriate prediction models for determining the presence and severity of OSA. We hypothesized that efficient models to investigate the likelihood of OSA can be established by combining all significant clinical parameters.
We present the following article in accordance with the TRIPOD reporting checklist (available at https://jtd.amegroups.com/article/view/10.21037/jtd-20-3139/rc).
Methods
The study was conducted in accordance with the Declaration of Helsinki (as revised in 2013). The present study was approved by the Institutional Review Board of Beijing Tongren Hospital (Beijing, China; TRECKY2019-049), and written consent was obtained from all subjects.
Subjects
Subjects 18 to 70 years of age, who were referred for PSG to the Sleep Medicine Center of Beijing Tongren Hospital, were recruited in the experimental group consecutively for the development of the model from June to October 2019. The validation group was obtained from November to December 2019. Individuals with OSA-related surgical history (e.g., tonsillectomy, uvulopalatopharyngoplasty, and nasoseptoplasty), psychiatric disorders, and pregnancy were excluded.
Clinical data collection
Clinical assessment of all subjects was performed on the same day as PSG including the following.
General information
Sex, age, history of alcohol consumption, history of smoking, comorbidities (diabetes mellitus, hyperlipidemia, cardiovascular disease, asthma, and hypertension) were confirmed, either by self-report or associated test results, or medication taken by the subjects.
OSA-related symptoms
Selection of risk factors was based on previous studies (16), including snoring, witnessed nocturnal apnea, nocturia, struggling for breath upon awakening, insomnia, dry mouth in the morning, morning headaches, non-restorative sleep, excessive daytime sleepiness [estimated using the Epworth Sleepiness Scale (ESS)] (9), diurnal fatigue, cognitive function impairment (subjective memory or attention deficits), and rhinitis symptoms. Details regarding these symptoms were obtained by self-report or bed-fellow reporting. The definitions of symptoms are summarized in Table 1.
Table 1
Symptom | Definition |
---|---|
Snoring | ≥3 times/week |
Non-restorative sleep-awakening with unrefreshing feel | ≥3 times/week |
Dry mouth in the morning | ≥3 times/week |
Witnessed nocturnal apnea | ≥3 times/week |
Struggling for breath upon awakening | ≥3 times/week |
Diurnal fatigue-a subjective sense of weariness | ≥3 times/week |
Excessive daytime sleepiness | ESS score range from 0–24 |
Nocturia-getting up to urinate | ≥2 times/night |
Cognitive function impairment | subjective memory or attention deficits during the past 12 months |
Rhinitis symptoms-watery nasal discharge, sneezing, nasal obstruction and nasal itching | a history of the typical symptoms during the past 12 months |
Insomnia-attempting to fall asleep more than 30 minutes or waking up during the night and falling asleep again more than 30 minutes | ≥5–15 times/month |
Morning headaches | ≥3 times/week |
OSA, obstructive sleep apnea; ESS, Epworth Sleepiness Scale.
Questionnaires
All subjects with suspected OSA were required to complete three different questionnaires. The STOP-BANG questionnaire (≥3 positive items were considered to be at high risk for OSA) (10), Berlin Questionnaire (≥2 positive catalogs were regarded as a higher OSA risk group) (11), the ESS (score ranging from 0 to 24, with higher scores indicating more daytime sleepiness) (9).
Physical examination
Body measurements were completed with the subjects wearing light clothing and in the standing position including body height(m) and weight(kg), body mass index (BMI, expressed as kg/m2), BMI grade (normal <24 kg/m2; 24 kg/m2≤ overweight ≤28 kg/m2; obese >28 kg/m2), neck circumference (measured at the level of the thyroid cartilage), waist circumference (measured from a linear distance around the middle between the 12th rib and the top of the iliac crest at the end of expiration), and central obesity (defined as waist circumference >90 cm for men and 85 cm for women) (17). Evaluation of the upper airway structures is shown in Figure 1. Measurement of tonsillar size was performed according to Brodsky grading scale (grade 1, tonsils occupy ≤25% of the oropharyngeal airway; grade 2, tonsils occupy 25% < oropharyngeal airway ≤50%; grade 3, tonsils occupy 50% < oropharyngeal airway ≤75%; grade 4, tonsils occupy >75% of the oropharyngeal airway) (18). The determination of pharyngeal grade was dependent on the location of the intersection between the tongue and palatopharyngeal arch, relative to the tongue diameter: grade 1, palatopharyngeal arch intersects at the edge of the tongue; grade 2, palatopharyngeal arch intersects at ≥25% of the tongue diameter; grade 3, palatopharyngeal arch intersects at ≥50% of the tongue diameter; grade 4, palatopharyngeal arch intersects at ≥75% of the tongue diameter) (12). The procedure was performed without protruding the tongue and using a tongue depressor if necessary. Lateral narrowing was considered when visible lateral wall tissue impinged the posterior oropharyngeal space (19). Nasal examination was performed to determine the presence of a deviated nasal septum and/or hypertrophic inferior turbinates, using an endoscope with the patient seated and the head slightly back.
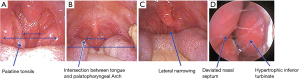
Thirty subjects’ examination was performed by three otolaryngologists and kappa statistical analysis was used to evaluate the degree of interexaminer agreement (20).
PSG
One night of PSG (Alice 5 or Bond) was performed after clinical assessment. Electroencephalogram (C3/A2, C4/A1, O1/A2, and O2/A1), electrooculogram, and submental electromyogram were recorded using surface electrodes. Moreover, electrocardiography, nasal airflow (measured using nasal cannulae with a pressure transducer), oral airflow (measured using a thermistor), chest wall, and abdominal movements (recorded by inductance plethysmography) pulse oximetry, video and audio recordings, and body position were also included. Definitions of respiratory events were based on American Academic Sleep Medicine (AASM) criteria, performed by experienced accredited sleep technologists blinded to the clinical assessment results. Apnea was defined as a drop in the peak signal excursion by ≥90% of pre-event baseline and the duration of the drop was ≥10 s. Hypopnea was defined as a drop in the peak signal excursion ≥30% and the duration ≥10 s and ≥3% oxygen desaturation from pre-event baseline or the event is associated with an arousal (21). The apnea-hypopnea index (AHI) was calculated as the total number of apneas and hypopneas per hour of sleep time. All eligible subjects were assigned to either the control group (AHI <15 events/h) or the OSA group (AHI ≥15 events/h).
Statistical analysis
Continuous variables are expressed as mean and standard deviation or median [inter quartile range (IQR)], where appropriate, and ordinal variables are expressed as percentages. The Mann-Whitney U test was used to compare non-normally distributed continuous. The Chi-squared test or Fisher’s exact test was used to compare categorical variables. Differences with P<0.05 (only two-tailed) were considered to be statistically significant. Significant variables identified in the primary case control analysis were entered into logistic and linear regression analyses to develop predictive models for OSA status and AHI. Finally, the diagnostic accuracy between the prediction model and other sleep questionnaires was examined using receiver operating characteristic (ROC) curve analysis. Two operating points were selected from the ROC curve: one was selected for the maximum diagnostic efficiency—was based on the best fitting sensitivity, specificity; one was selected for the other for high (90%) specificity. Statistical analysis was performed using SPSS version 26.0 (IBM Corporation, Armonk, NY, USA).
Results
Ultimately, 202 eligible subjects were included in the experimental group for development of the models, of whom 77.7% were male. The median age was 39.5 years (range, 18–68 years) and the median BMI was 26.9 kg/m2 (IQR, 24.5–29.4 kg/m2). The entire group had a large neck circumference [median neck circumference 40 cm (IQR 37.9–42.3 cm)]. The proportion of patients with OSA was 62.3%. The median AHI of the entire group was 26.6 events/h (IQR, 9.6–54.8 events/h). One hundred and one eligible subjects were included in the validation group. There was no difference in age, sex, BMI, AHI, and ESS between groups (Table 2).
Table 2
Characteristics | Experimental group | Validation group | P value |
---|---|---|---|
Sex | (Male) 77.7% | (Male) 83.2% | 0.27 |
Age | 39.5 (31.8, 48.3) | 43 (33.0, 52.0) | 0.51 |
BMI | 26.9 (24.5, 29.4) | 26.5 (24.3, 29.2) | 0.28 |
Neck circumference | 40 (37.9, 42.3) | 40.8 (38.1, 42.5) | 0.84 |
AHI | 26.6 (9.6, 54.8) | 23 (8.5, 47.3) | 0.16 |
ESS | 8 (5.8, 13.0) | 8 (5.0, 12.0) | 0.87 |
Data are expressed as median (interquartile range) or percentage (%). ESS, Epworth Sleepiness Scale; BMI, body mass index; AHI, apnea-hypopnea index.
Subject clinical characteristics
Demographic information and clinical characteristics of the subjects are summarized in Table 3. Patients with OSA were more likely to be male and older. A higher prevalence of a history of smoking and alcohol consumption was observed in the OSA group than in the control group. However, because the data were also analyzed according to sex, which was assumed to be a confounding factor, alcohol consumption in males was the only significant difference between subjects with OSA and controls. There was significant difference in hyperlipidemia between the OSA and control groups; however, there were no statistically significant differences in other comorbidities.
Table 3
Variable | Control group | OSA group | P value |
---|---|---|---|
Snoring | 81.3% | 98.4% | <0.001 |
Non-restorative sleep | 36.0% | 37.8% | 0.81 |
Dry mouth in the morning | 54.7% | 69.3% | 0.037 |
Witnessed nocturnal apnea | 21.3% | 69.3% | <0.001 |
Struggling for breath upon awakening | 28.0% | 44.1% | 0.038 |
Diurnal fatigue | 40.0% | 43.3% | 0.52 |
Excessive daytime sleepiness (ESS) | 7 [4, 11] | 10 [6, 14] | 0.008 |
Nocturia | 21.3% | 33.9% | 0.025 |
Cognitive function impairment | 74.7% | 64.6% | 0.066 |
Rhinitis symptoms | 61.3% | 56.7% | 0.519 |
Insomnia | 16.5% | 20.0% | 0.535 |
Morning headaches | 6.7% | 8.7% | 0.843 |
Sex | (Male) 66.7% | (Male) 84.3% | 0.003 |
Age | 37 [31, 44] | 42 [33, 51] | 0.01 |
Alcohol consumption | (Male) 46.0% | (Male) 71.0% | (Male) 0.003 |
(Female) 16% | (Female) 0.0% | (Female) 0.064 | |
History of smoking | (Male) 38.0% | (Male) 51.4% | (Male) 0.118 |
(Female) 4.0% | (Female) 5.0% | (Female) 0.873 | |
Diabetes mellitus | 4.0% | 6.3% | 0.654 |
Hyperlipidemia | 26.7% | 42.5% | 0.021 |
Cardiovascular disease | 2.7% | 4.7% | 0.465 |
Asthma | 4% | 4% | 0.807 |
Hypertension | 26.7% | 37.8% | 0.086 |
BMI | 25.9 (23.6, 28.7) | 27.4 (25.6, 30.1) | 0.003 |
BMI Grade | Normal (32.0%) | Normal (11.8%) | 0.021 |
Overweight (37.3%) | Overweight (48.8%) | ||
Obesity (30.7%) | Obesity (39.4%) | ||
Neck circumference | 39.5 (35.6, 41.1) | 41.1 (39.0, 42.5) | <0.001 |
Central obesity | 56.0% | 83.5% | <0.001 |
Tonsillar size | Grade 1 (66.7%) | Grade 1 (46.5%) | 0.01 |
Grade 2 (22.6%) | Grade 2 (31.5%) | ||
Grade 3 (8.0%) | Grade 3 (11.8%) | ||
Grade 4 (2.7%) | Grade 4 (10.2%) | ||
Pharyngeal grade | Grade 1 (9.3%) | Grade 1 (3.2%) | <0.001 |
Grade 2 (26.3%) | Grade 2 (5.6%) | ||
Grade 3 (44.7%) | Grade 3 (46.8%) | ||
Grade 4 (19.7%) | Grade 4 (44.4%) | ||
Lateral narrowing | 12% | 45.2% | <0.001 |
Deviated nasal septum | 57.3% | 74.8% | 0.01 |
Hypertrophic inferior turbinates | 37.3% | 34.7% | 0.701 |
Data are expressed as median (interquartile range) or percentage (%). OSA, obstructive sleep apnea; ESS, Epworth Sleepiness Scale; BMI, body mass index.
Significant differences were observed with regard to typical and atypical complaints, including snoring, witnessed nocturnal apnea, struggling for breath upon awakening, excessive daytime sleepiness, nocturia, and dry mouth in the morning. With regard to physical examination, all indicators of obesity (BMI, BMI grade, central obesity, and neck circumference) were significantly greater in the OSA group than in the control group. Furthermore, a higher proportion of tonsillar enlargement, deviated nasal septum, lateral narrowing, and pharyngeal narrowing were observed in the OSA group. Additionally, kappa value for tonsillar size (0.84), pharyngeal grade (0.77), lateral narrowing (0.81), deviated nasal septum (0.76) and hypertrophic inferior turbinates (0.64) represented good agreement.
Logistic and linear regression analysis
All important clinical variables identified in the univariate analysis were entered into the regression model. Finally, a logistic regression model consisting of 4 predictors was established (Table 4). Snoring yielded the most significant contribution, followed by witnessed nocturnal apnea. OSA status was defined using the following Eq. [1]:
Table 4
Variable | Coefficient(B) | SE | P value | OR |
---|---|---|---|---|
Snoring | 2.05 | 0.89 | 0.021 | 7.66 |
Witnessed nocturnal apnea | 1.80 | 0.38 | <0.001 | 6.33 |
Central obesity | 1.00 | 0.39 | 0.011 | 3.09 |
Lateral narrowing | 1.73 | 0.47 | <0.001 | 5.53 |
OSA, obstructive sleep apnea.
in which 1= presence of snoring, witnessed nocturnal apnea, central obesity or lateral narrowing and 0= absence of snoring, witnessed nocturnal apnea, central obesity, or lateral narrowing. The optimal cut-off value on the ROC was 0.61, yielding a sensitivity of 81.1% (95% CI: 73.2–87.5%) and a specificity of 76.0% (95% CI: 64.7–85.1%). The LR+ and LR– ratios were 3.4 (95% CI: 2.2–5.1) and 0.3 (95% CI: 0.2–0.4), respectively. The operating point with a high specificity (90%) was 0.82, yielding a sensitivity of 56.7% (95% CI: 47.6–65.5%), and a specificity of 90% (95% CI: 81.7–96.2%). The LR+ and LR– ratios were 6.1 (3.0–12.5) and 0.5 (0.4–0.6), respectively.
A comparison of ROC curves between the predictive model and other questionnaires is shown in Figure 2. The area under the ROC curve (AUC) was 83.7% (95% CI: 77.9–88.5%) for the predictive algorithm. The AUC of the new predictive model was significantly higher than that of the Berlin Questionnaire [AUC 53.5% (95% CI: 45.2–61.8%); P<0.01], ESS [AUC 61.1% (95% CI: 53.3–68.9%); P<0.01], and STOP-BANG questionnaire [AUC 73.8% (95% CI: 66.9–80.8%); P<0.01]. The linear regression model indicated that AHI was a function of 4 variables [2]:
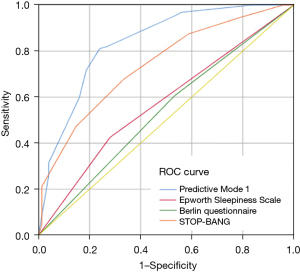
The variance inflation factor of the variables demonstrated no substantial indicators of multicollinearity (Table 5).
Table 5
Variable | Coefficient(B) | VIF | 95% CI | P value |
---|---|---|---|---|
Witnessed nocturnal apnea | 17.1 | 1.370 | 10.2 to 24.0 | <0.001 |
Tonsillar size | 6.2 | 1.088 | 2.9 to 9.5 | <0.001 |
Central obesity | 12.5 | 1.132 | 5.6 to 19.3 | <0.001 |
Lateral narrowing | 9.0 | 1.180 | 2.2 to 15.8 | 0.01 |
Intercept | −19.7 | −39.5 to 0.0 | 0.05 |
VIF, variance inflation factor; AHI, apnea-hypopnea index; CI, confidence interval.
Validation
We compared the results of the two predictive models with PSG in the validation group. The logistic regression model would classify subjects with and without OSA and achieved an accuracy of 0.812, a sensitivity of 81.3%, a specificity of 81.1%. The LR+ and LR– ratios were 4.3 and 0.8, respectively. The liner regression model would output a predictive AHI and the subjects would be divided into normal, mild, moderate, and severe group (normal <5 events/h; 5≤ mild <15 events/h; 15≤ moderate <30 events/h; severe ≥30 events/h) and achieved an accuracy of 0.416.
Model comparisons
Given that the estimation of lateral narrowing is a qualitative parameter and BMI is a common predictor for OSA, we presented the performance (AUC-ROC, sensitivity, specificity) of the models with and without these variables. Additionally, we present the performance of a model based on BMI, neck circumference, and oral cavity measurement (without symptoms) for the prediction of OSA. The results showed that explanatory variables in our model had the best predictive performance (Table 6).
Table 6
Models | AUC (%) | Sensitivity (%) (95% CI) | Specificity (%) (95% CI) | LR+ (95% CI) | LR– (95% CI) |
---|---|---|---|---|---|
Predictive model | 83.7 | 81.1 (73.2–87.5) | 76.0 (64.6–85.1) | 3.4 (2.2–5.1) | 0.3 (0.2–0.4) |
Model 1 | 76.3 | 70.1 (60.5–77.2) | 76.0 (67.7–87.3) | 2.9 (2.1–5.1) | 0.4 (0.3–0.5) |
Model 2 | 79.9 | 69.3 (60.5–77.2) | 78.7 (67.7–87.3) | 3.3 (2.1–5.1) | 0.4 (0.3–0.5) |
Model 3 | 78.4 | 75.4 (66.9–82.6) | 77.3 (66.2–86.2) | 3.3 (2.2–5.1) | 0.3 (0.2–0.4) |
Predictive model: Snoring, Witnessed nocturnal apnea, Central obesity, Lateral narrowing. Model 1: Snoring, Witnessed nocturnal apnea, BMI, Lateral narrowing. Model 2: Snoring, Witnessed nocturnal apnea, Central obesity. Model 3: Neck circumference, BMI, Tonsillar size, Lateral narrowing, Pharyngeal grade. LR, likelihood ratio; CI, confidence interval; AUC, area under the ROC curve.
Discussion
OSA is characterized by repeated episodes of upper airway collapse, which is considered to be the predominant condition exhibited in sleep-disordered breathing. Deemed a risk factor for motor vehicle accidents (22) and reduced quality of life (23), OSA has become a public health issue and, accordingly, has received more attention. The present study used clinical parameters to establish regression models to determine the presence and severity of OSA. In predicting an AHI ≥15 events/h, our predictive model achieved an accuracy of 0.812, a sensitivity of 81.3%, and a specificity of 81.1% in the validation group.
Habitual snoring and witnessed sleep apnea, which reflect narrowing of the upper airway, were predictors of OSA in our model. Although subjects with snoring were common in both groups, the study population was not from the community, and the OSA group had a higher proportion of such individuals. Results demonstrated that snoring and witnessed apnea were more important predictors than other symptoms because self-reported snoring and breath holding were associated with the presence of sleep apnea, even within single persons, persons living alone, and those customarily sleeping alone, of both sexes (24). There was little doubt that these nocturnal symptoms were sensitive indicators of OSA. A systematic review based on population-based studies reported that the sensitivity and specificity of snoring and reported apnea were 90% and 19%, and 80% and 42%, respectively, at the level of AHI >10 or 15 events/h (15). A combination of BMI and subjective snoring severity scale, which assesses snoring loudness, frequency, and duration, yielded a high sensitivity (97.4%) and but a low specificity (40%) (25). We only collected data regarding frequency of snoring, rather than loudness and duration, because of its accessibility in practice.
Obtaining accurate history is often problematic. Symptoms occur during sleep, exaggerating or disregarding symptoms are obstacle to obtain good history. The collection of subjective history from the subjects is usually insufficient; as such, it is important to examine anatomical abnormalities of the upper airway. Enlargement of soft tissue structures lead to narrowing of the upper airway and increased collapsibility, especially in Far-East Asians with relatively small cranial base dimensions (14). The Mallampati score was initially used to predict difficulty of endotracheal intubation based on the visibility of oral structures with the tongue protruding from the widely open mouth. It was also an independent predictor of both the presence and severity of OSA. For every increase in the Mallampati score, the odds of having OSA increased 2-fold, and the AHI increased by 5 events/h (26,27). Evaluation of oropharyngeal structures revealed that lateral narrowing increased the probability of OSA even after controlling for BMI and neck circumference. Moreover, tonsillar size has been strongly associated with AHI (19,28). Tonsillar size and lateral narrowing were independent predictors in our models, which was consistent with previous studies. The presence of lateral narrowing increase 5-fold of the odds of having OSA and every increase in tonsillar size was associated with an increase in AHI of 6 events/h. Asking patients to open their mouth to inspect redundant pharyngeal tissue was an essential step in estimating the likelihood of OSA. Obesity is regarded not only as a disease but also a strong risk factor for OSA that cannot be ignored. Waist circumference, BMI, and neck circumference are obesity indices. The cross-sectional study showed that waist circumference was a better predictor than general obesity indices or visceral adiposity indicators (29). Central obesity appears to play a more important role in OSA than other forms of obesity, and AHI has been reported to be significantly correlated with intra-abdominal and subcutaneous abdominal fat (30,31). Results from the present study also suggested that central obesity was a more important predictor included in the model than BMI or neck circumference. Patients with OSA, especially Far-East Asians, may have a low BMI (14). As such, BMI, as an indicator of obesity, could degrade the predictive performance of this risk factor.
A study prospectively studied the utility of four clinical prediction models for predicting the presence of OSA in the referral-based population. These models comprised snoring, witnessed apneas, hypertension, sex, age, BMI, and circumference. Using the best cutoff points, the sensitivities ranged from 76–96%, specificities from 13–54% (13). These models do not incorporate oral cavity measurements and suffer from poor specificities. Guideline paper suggested that symptoms and physical examination, such as choking at night, snoring, BMI, the Mallampati score, and lateral narrowing, were both essential for possible diagnosis of OSA (16). The predictors in our model, including history, physical examination and obesity, resulted in a more comprehensive inspection of subjects. Ultimately, the external validity of predictive models was performed and the experimental results proved our model is effective in predicting the presence of OSA. We compared our predictive model with other three models. The results demonstrated that removing or replacing original explanatory variables would degrade the predictive performance. Friedman et al. use snoring, Friedman tongue position, tonsil size, BMI and sleep questionnaires to construct predictive equation and the sensitivity and specificity of the model were 82% and 83.4%. The prediction improved when incorporating subjective and objective metrics into a single equation (32).
We compared our predictive model with three other questionnaires using ROC analysis. The Berlin Questionnaire, STOP-BANG questionnaire, and ESS are frequently used in our clinical practice to assess OSA status. Our predictive model demonstrated the best accuracy in detecting OSA. The accuracy of the STOP-BANG questionnaire was suboptimal, whereas the accuracy of the other two questionnaires was unsatisfactory. This result was supported by a meta-analysis that investigated the diagnostic accuracy of various questionnaires (33). It concluded that the STOP-BANG questionnaire was a superior tool for detecting OSA compared with the Berlin Questionnaire and ESS. As discussed, we believe that the relatively lower BMI of Asian patients could directly result in the misclassification of some of patients with OSA.
The predictive performance of clinical models varies significantly. A summary of 4 trials based on combinations of history and physical findings from physicians’ perspectives yielded an overall sensitivity of 58% and a specificity of 67%. A six-month prospective study based on the referral population surprisingly obtained a model with a sensitivity of 97.6% and a specificity of 100% according to BMI, neck circumference, and oral cavity measurements (15,34). Discrepancies, however, may be explained by differences in the study populations and method(s) of clinical assessment. In general, clinical prediction algorithms are not sufficient to substitute for PSG or home sleep apnea testing, but could be useful in prioritizing patients for testing or allowing starting continuous positive airway pressure without a sleep study (13,35). Using the operating point with a high specificity (90%), the sensitivity, specificity, LR+ and LR– ratios were 56.7%, 90%, 6.1, and 0.5. When the probability cutoff is chosen that achieve a high specificity, we could minimize the number of false positive tests. In other words, physicians could use the model to minimize the number of patients with an AHI <15 events/h placed into a definitive testing. The performance of predicting OSA severity was less satisfactory, suggesting clinical data was not efficient enough to predict OSA severity or the prediction of OSA severity needed to be divided into subgroups.
There were some limitations to the present study, the first of which was that the external validity of predictive models in different populations was limited. Second, our linear regression model might be less reliable, and establishing predictive model for the severity of OSA in different subgroups in a larger sample size was necessary. Third, referral-based controls in the analysis were supposed to be different from those without apnea in the community. Finally, patients with mild OSA should be included among the controls when defining OSA status at a cut-off of AHI 15 events/h. Thus, more reliable and more reproducible predictive models will be obtained with a larger sample size and validation of our prediction model in different populations will be necessary to overcome some of these limitations in the future.
Conclusions
Snoring, witnessed nocturnal apnea, tonsillar size, lateral narrowing, and central obesity were used to predict the presence and severity of OSA. The results suggest that it could potentially be useful in clinical applications used to identify patients who are at increased risk for OSA.
Acknowledgments
The authors thank the help of the study participants, otolaryngologists, and technologists at the Department of Otolaryngology Head and Neck Surgery, Bejing Tongren hospital.
Funding: This research was supported by the National Key Research & Development Program of China (2018YFC0116800), the National Natural Science Foundation of China (81970866), and Beijing Municipal Administration of Hospitals’ Youth Programme, Code: QMS20190202, and the payments were not made to their institution.
Footnote
Provenance and Peer Review: This article was a standard submission to the Sleep Section in the journal. The article has undergone external peer review.
Reporting Checklist: The authors have completed the TRIPOD reporting checklist Available at https://jtd.amegroups.com/article/view/10.21037/jtd-20-3139/rc
Data Sharing Statement: Available at https://jtd.amegroups.com/article/view/10.21037/jtd-20-3139/dss
Conflicts of Interest: All authors have completed the ICMJE uniform disclosure form (available at https://jtd.amegroups.com/article/view/10.21037/jtd-20-3139/coif). All authors report that this research was supported by the National Key Research & Development Program of China (2018YFC0116800), the National Natural Science Foundation of China (81970866), and Beijing Municipal Administration of Hospitals’ Youth Programme, Code: QMS20190202, and the payments were not made to their institution. The authors have no other conflicts of interest to declare.
Ethical Statement: The authors are accountable for all aspects of the work in ensuring that questions related to the accuracy or integrity of any part of the work are appropriately investigated and resolved. The study was conducted in accordance with the Declaration of Helsinki (as revised in 2013). The present study was approved by the Institutional Review Board of Beijing Tongren Hospital (Beijing, China; TRECKY2019-049), and written consent was obtained from all subjects.
Open Access Statement: This is an Open Access article distributed in accordance with the Creative Commons Attribution-NonCommercial-NoDerivs 4.0 International License (CC BY-NC-ND 4.0), which permits the non-commercial replication and distribution of the article with the strict proviso that no changes or edits are made and the original work is properly cited (including links to both the formal publication through the relevant DOI and the license). See: https://creativecommons.org/licenses/by-nc-nd/4.0/.
References
- Lévy P, Kohler M, McNicholas WT, et al. Obstructive sleep apnoea syndrome. Nat Rev Dis Primers 2015;1:15015. [Crossref] [PubMed]
- Lavie L. Obstructive sleep apnoea syndrome--an oxidative stress disorder. Sleep Med Rev 2003;7:35-51. [Crossref] [PubMed]
- Lévy P, Pépin JL, Arnaud C, et al. Intermittent hypoxia and sleep-disordered breathing: current concepts and perspectives. Eur Respir J 2008;32:1082-95. [Crossref] [PubMed]
- Sánchez-de-la-Torre M, Campos-Rodriguez F, Barbé F. Obstructive sleep apnoea and cardiovascular disease. Lancet Respir Med 2013;1:61-72. [Crossref] [PubMed]
- Veasey SC, Rosen IM. Obstructive Sleep Apnea in Adults. N Engl J Med 2019;380:1442-9. [Crossref] [PubMed]
- Benjafield AV, Ayas NT, Eastwood PR, et al. Estimation of the global prevalence and burden of obstructive sleep apnoea: a literature-based analysis. Lancet Respir Med 2019;7:687-98. [Crossref] [PubMed]
- Kapur VK, Auckley DH, Chowdhuri S, et al. Clinical Practice Guideline for Diagnostic Testing for Adult Obstructive Sleep Apnea: An American Academy of Sleep Medicine Clinical Practice Guideline. J Clin Sleep Med 2017;13:479-504. [Crossref] [PubMed]
- Young T, Skatrud J, Peppard PE. Risk factors for obstructive sleep apnea in adults. JAMA 2004;291:2013-6. [Crossref] [PubMed]
- Johns MW. A new method for measuring daytime sleepiness: the Epworth sleepiness scale. Sleep 1991;14:540-5. [Crossref] [PubMed]
- Chung F, Yegneswaran B, Liao P, et al. STOP questionnaire: a tool to screen patients for obstructive sleep apnea. Anesthesiology 2008;108:812-21. [Crossref] [PubMed]
- Netzer NC, Stoohs RA, Netzer CM, et al. Using the Berlin Questionnaire to identify patients at risk for the sleep apnea syndrome. Ann Intern Med 1999;131:485-91. [Crossref] [PubMed]
- Tsai WH, Remmers JE, Brant R, et al. A decision rule for diagnostic testing in obstructive sleep apnea. Am J Respir Crit Care Med 2003;167:1427-32. [Crossref] [PubMed]
- Rowley JA, Aboussouan LS, Badr MS. The use of clinical prediction formulas in the evaluation of obstructive sleep apnea. Sleep 2000;23:929-38. [Crossref] [PubMed]
- Li KK, Kushida C, Powell NB, et al. Obstructive sleep apnea syndrome: a comparison between Far-East Asian and white men. Laryngoscope 2000;110:1689-93. [Crossref] [PubMed]
- Myers KA, Mrkobrada M, Simel DL. Does this patient have obstructive sleep apnea?: The Rational Clinical Examination systematic review. JAMA 2013;310:731-41. [Crossref] [PubMed]
- Epstein LJ, Kristo D, Strollo PJ Jr, et al. Clinical guideline for the evaluation, management and long-term care of obstructive sleep apnea in adults. J Clin Sleep Med 2009;5:263-76. [Crossref] [PubMed]
- Bao Y, Lu J, Wang C, et al. Optimal waist circumference cutoffs for abdominal obesity in Chinese. Atherosclerosis 2008;201:378-84. [Crossref] [PubMed]
- Brodsky L. Modern assessment of tonsils and adenoids. Pediatr Clin North Am 1989;36:1551-69. [Crossref] [PubMed]
- Schellenberg JB, Maislin G, Schwab RJ. Physical findings and the risk for obstructive sleep apnea. The importance of oropharyngeal structures. Am J Respir Crit Care Med 2000;162:740-8. [Crossref] [PubMed]
- Byrt T. How good is that agreement? Epidemiology 1996;7:561. [Crossref] [PubMed]
- Berry RB, Budhiraja R, Gottlieb DJ, et al. Rules for scoring respiratory events in sleep: update of the 2007 AASM Manual for the Scoring of Sleep and Associated Events. Deliberations of the Sleep Apnea Definitions Task Force of the American Academy of Sleep Medicine. J Clin Sleep Med 2012;8:597-619. [Crossref] [PubMed]
- Mulgrew AT, Nasvadi G, Butt A, et al. Risk and severity of motor vehicle crashes in patients with obstructive sleep apnoea/hypopnoea. Thorax 2008;63:536-41. [Crossref] [PubMed]
- Campos-Rodriguez F, Queipo-Corona C, Carmona-Bernal C, et al. Continuous Positive Airway Pressure Improves Quality of Life in Women with Obstructive Sleep Apnea. A Randomized Controlled Trial. Am J Respir Crit Care Med 2016;194:1286-94. [Crossref] [PubMed]
- Bliwise DL, Nekich JC, Dement WC. Relative validity of self-reported snoring as a symptom of sleep apnea in a sleep clinic population. Chest 1991;99:600-8. [Crossref] [PubMed]
- Morris LG, Kleinberger A, Lee KC, et al. Rapid risk stratification for obstructive sleep apnea, based on snoring severity and body mass index. Otolaryngol Head Neck Surg 2008;139:615-8. [Crossref] [PubMed]
- Mallampati SR, Gatt SP, Gugino LD, et al. A clinical sign to predict difficult tracheal intubation: a prospective study. Can Anaesth Soc J 1985;32:429-34. [Crossref] [PubMed]
- Nuckton TJ, Glidden DV, Browner WS, et al. Physical examination: Mallampati score as an independent predictor of obstructive sleep apnea. Sleep 2006;29:903-8. [Crossref] [PubMed]
- Jara SM, Weaver EM. Association of palatine tonsil size and obstructive sleep apnea in adults. Laryngoscope 2018;128:1002-6. [Crossref] [PubMed]
- Zhao X, Xu H, Qian Y, et al. Abdominal Obesity Is More Strongly Correlated with Obstructive Sleep Apnea than General Obesity in China: Results from Two Separated Observational and Longitudinal Studies. Obes Surg 2019;29:2535-47. [Crossref] [PubMed]
- Schäfer H, Pauleit D, Sudhop T, et al. Body fat distribution, serum leptin, and cardiovascular risk factors in men with obstructive sleep apnea. Chest 2002;122:829-39. [Crossref] [PubMed]
- Grunstein R, Wilcox I, Yang TS, et al. Snoring and sleep apnoea in men: association with central obesity and hypertension. Int J Obes Relat Metab Disord 1993;17:533-40. [PubMed]
- Friedman M, Wilson MN, Pulver T, et al. Screening for obstructive sleep apnea/hypopnea syndrome: subjective and objective factors. Otolaryngol Head Neck Surg 2010;142:531-5. [Crossref] [PubMed]
- Chiu HY, Chen PY, Chuang LP, et al. Diagnostic accuracy of the Berlin questionnaire, STOP-BANG, STOP, and Epworth sleepiness scale in detecting obstructive sleep apnea: A bivariate meta-analysis. Sleep Med Rev 2017;36:57-70. [Crossref] [PubMed]
- Kushida CA, Efron B, Guilleminault C. A predictive morphometric model for the obstructive sleep apnea syndrome. Ann Intern Med 1997;127:581-7. [Crossref] [PubMed]
- Nigro CA, Borsini E, Dibur E, et al. Indication of CPAP without a sleep study in patients with high pretest probability of obstructive sleep apnea. Sleep Breath 2020;24:1043-50. [Crossref] [PubMed]