Native T1-mapping and diffusion-weighted imaging (DWI) can be used to identify lung cancer pathological types and their correlation with Ki-67 expression
Introduction
Lung cancer is one of the leading causes of cancer-related deaths worldwide (1). Although significant progress has been made in lung cancer treatment, including surgery, chemotherapy, and radiotherapy, further improvement in survival rates is needed. Therefore, obtaining accurate information on diagnosis, stage, histological subtype, degree of tumor differentiation, and molecular markers before treatment is crucial for the selection of clinical treatment strategies (2-4). Immunohistochemistry (IHC) analysis is able to add prognostic value to the current staging system through identifying markers of tumour aggressiveness. Ki-67 protein is a nuclear antigen associated with tumor proliferation and closely associated with lung cancer development, metastasis, and prognosis. Previous studies have shown that proliferative activity in dependence of histological tumour type and significant positive correlations were found between Ki-67 overexpression and poorer differentiation, larger tumor size and higher pathologic stages (5-7). Accurate preoperative assessment of the Ki-67 index in lung cancer facilitates prognosis prediction and provides helpful information for clinical decision-making. Histopathological examination is the gold standard for diagnosis of lung cancer and pathological classification. However, it has some contraindications, such as a higher risk of preoperative complications or interstitial lung disease in patients with poor cardiopulmonary function, and limitations in patients with poor compliance (8).
Magnetic resonance imaging (MRI) provides excellent soft-tissue contrast and multiparametric imaging compared to computed tomography (CT) or positron emission tomography (PET)/CT, while involving no exposure to ionizing radiation. The functional or metabolic information of imaging modalities reflects the biophysical properties of tissues. Magnetic resonance diffusion-weighted imaging (DWI) describes the microscopic Brownian motion of water molecules in biological tissues, reflecting changes of diffusion limitation to characterize the microstructure of tissues. Changes in diffusion limitation can be quantified using an apparent diffusion coefficient (ADC) (9), which is the best imaging parameter for in vivo quantification of the combined effects of capillary perfusion and conflation of spinning nuclei and diffusing molecules. DWI has attracted increasing attention in differentiating pathological types of tumors, distinguishing benign and malignant tumors, and evaluating therapeutic responses (10-12), as well as evaluating thoracic lymph nodes in lung cancer patients (13). Some studies have also suggested that ADC value can help distinguish between high and low expression of Ki-67 protein (14,15).
T1-mapping imaging technology is a highly sensitive and quantitative MRI measurement technology based on tissue specificity, which can reflect the inherent longitudinal relaxation time of tissues. It can be used for quantitative in vivo histology and to determine biological tissue characteristics. T1-mapping can reflect slight differences in tissue composition and water content and can be directly quantified into the T1 value. In the pathological condition of diseases, the tissue T1 value tends to change (16). T1-mapping has been mainly used in the field of cardiovascular imaging, where it has emerged as an important imaging tool for characterization of myocardial tissue (17,18), while the research on lung diseases has mainly focused on the functional evaluation of chronic obstructive pulmonary disease (19,20). In addition, T1-mapping has shown its potential ability to distinguish benign from malignant lung lesions due to the different water content of the lung lesions (21). However, in their study, the range of T1 values for malignant lung tumors was broad and it is unclear whether they are associated with different pathological types of lung cancer.
To the best of our knowledge, there have been no studies on DWI combined with T1-mapping to predict histological types and degree of tumor differentiation of lung cancer. Therefore, this study aimed to investigate the value of DWI and T1-mapping in predicting different pathological types and differentiation degrees of lung cancer and their correlation with Ki-67 protein expression. We present the following article in accordance with the STARD reporting checklist (available at https://jtd.amegroups.com/article/view/10.21037/jtd-22-77/rc).
Methods
Patient population
A total of 78 patients with pulmonary tumors were consecutively enrolled from May 2020 to June 2021 in this prospective study. Inclusion criteria were: (I) patients suspected of lung cancer on X-ray or CT, (II) patients with complete clinical data, (III) no treatment options received before the MRI examination, and (IV) lesion diameter ≥1.5 cm. Exclusion criteria were: (I) serious image artifacts; (II) patients with contraindication for MRI examination, such as those with metal implants; (III) patients with incomplete clinical data; and (IV) benign lesions, adenosquamous carcinoma, large cell carcinoma, and other rare tumors. In all, 64 lung cancer patients were included in our study. All patients underwent routine MRI scans and native T1-mapping imaging within 1 week before puncture or surgery. Figure 1 depicts the patient selection process.
The study was conducted in accordance with the Declaration of Helsinki (as revised in 2013). It was approved by the Ethics Committee of the First Affiliated Hospital of Soochow University (No. 2020-244) and informed consent was taken from all the patients. The complete study protocol is available from the Chinese Clinical Trial Registry. The Trial Registration number is: ChiCTR2100045624.
MRI acquisitions
MRI examinations were performed using a 3.0 T clinical magnetic resonance scanner (MAGNETOM Skyra, Siemens Healthineers, Erlangen, Germany) with a dedicated 18-channel body-phased array coil. Patients underwent respiratory training before the examination. Image acquisition included coronal T2 weighted imaging (T2WI), axial 3D-T1WI, T2WI, DWI, and respiratory-gated steady-state precession readout single-shot Modified Look-Locker Inversion Recovery (MOLLI) sequences. The Look-Locker sequence was selected for data acquisition at a given time in the cardiac cycle, and the image signal was collected several times after a flip pulse. All images were collected into data sets that could generate T1-mapping color maps immediately after data collection, and the signal strength of each pixel represented the T1 value (22). Table 1 lists the detailed parameters of the MRI examinations.
Table 1
Sequence | T2 haste | T2 BLADE | T1 VIBE-3d | DWI | MOLLI | T1 VIBE-3d c+ |
---|---|---|---|---|---|---|
Scan plane | Coronal | Axial | Axial | Axial | Axial | Axial |
Voxel size, mm | 1.3×1.3×5 | 1.3×1.3×5 | 1.3×1.3×3.5 | 1.6×1.6×5 | 1.9×1.9×5 | 1.3×1.3×3.5 |
Slice | 24 | 20 | 1 | 20 | 4 | 1 |
TR/TE, msec | 1,400/87 | 3,000/87 | 4.11/1.24 | 5,600/72 | 246.8/1.01 | 4.11/1.24 |
Averages | 1 | 1 | 1 | – | 1 | 1 |
Fov, mm | 400 | 400 | 420 | 400 | 360 | 420 |
Flip angle, degrees | 180 | 180 | 12 | – | 35 | 12 |
Matrix | 320 | 320 | 320 | 128 | 192 | 320 |
Fat saturation | None | Yes | None | Yes | None | None |
Parameter map type | – | – | – | – | T1-mapping | – |
No. inversions | – | – | – | – | 4 | – |
MOLLI TI start, msec | – | – | – | – | 180 | – |
MOLLI TI trigger delay, msec | – | – | – | – | 380 | – |
BLADE is the proprietary name for periodically rotated overlapping parallel lines with enhanced reconstruction (PROPELLER) in MR systems from Siemens Healthcare. MRI, magnetic resonance imaging; HASTE, half Fourier single-shot Turbo spin-Echo; VIBE-3D, 3-dimensional volumetric interpolated breath-hold examination; DWI, diffusion-weighted imaging; MOLLI, Modified Look-Locker Inversion Recovery; TR, repetition time; TE, echo time; FOV, field of view.
Image analysis
All acquired data were transferred to a vendor-provided workstation (syngo.via, Siemens Healthineers). Two radiologists (Dr. A and B, with 8 and 10 years of experience in CT and MRI diagnosis of thoracic tumors, respectively), who were blinded to pathology results and clinical data, independently assessed ADC and T1-mapping images in randomized order. A region of interest (ROI) was placed on the solid component with the largest and most uniform signal of the whole tumor at the level of maximum transverse diameter. With the T2WI images and enhanced T1WI images used as reference, a ROI was placed to avoid nonenhanced areas such as necrosis, cystic change, and hemorrhage as much as possible, and to exclude the outer edge of the tumor to reduce part of the volume effects. The ROI size ranged from 2.1 to 8.8 cm2. The automatically generated T1 and ADC values within the ROI were recorded, and the values measured by the 2 observers were averaged.
Pathological analysis
Specimen treatment was as follows: the specimens were cut into serial 1-mm-thick sections by referring to the axial radiological images, after which they were fixed in 10% neutral formaldehyde solution for 24–72 hours using conventional materials, dehydration, and paraffin embedding. The tumor specimens were examined through immunohistochemical and hematoxylin and eosin staining for histological observation and analyzed by immunohistochemistry using antibodies against Ki-67 (1:200, Dako). Ki-67-positive tumour cells were counted by focusing on representative areas avoiding obvious hot spots as well as areas with specifically low indices and areas with necrosis or haemorrhage. Ki-67 score was measured by determining the percentage of cells with positive nuclei in >1,000 tumor cells in >4 fields.
Statistical methods
SPSS 25.0 (SPSS Inc., Chicago, IL, USA) and GraphPad Prism 8.0 software were used for statistical analysis. The intraclass correlation coefficient (ICC) and Bland-Altman plots were generated to assess the interobserver agreement of the T1 and ADC mean values in lesions. An ICC of 1.0 was considered to represent perfect agreement; 0.81–0.99, almost perfect agreement; 0.61–0.80, substantial agreement; 0.41–0.60, moderate agreement; 0.21–0.40, fair agreement; and 0.20 or less, slight agreement (23). The Kolmogorov-Smirnov method was used to test the normal distribution of measurement data. Differences between groups for quantitative variables conforming to normal distribution were analyzed by t-test or ANOVA. Categorical variables were expressed as frequencies (percentages), and differences between groups were compared using chi-square tests. Receiver operating characteristic (ROC) curves were used to analyze the differential diagnostic efficacy of ADC values and T1 values between the moderately and highly differentiated group and the poorly differentiated group. The correlation between ADC value, T1 value, and Ki-67 protein expression index was determined. Pearson correlation analysis was used for data with normal distribution; otherwise, Spearman correlation analysis was used. Two-tailed P value <0.05 was considered statistically significant.
Results
Clinical characteristics
A total of 64 patients, each with 1 lesion, were included in this study (including 49 males and 15 females, average age 62.1±8.1 years, range 39–75 years). The average lesion size was 4.2±2.0 cm (range, 1.8–10 cm). The lesions were confirmed as adenocarcinoma (AD, n=33), squamous cell carcinoma (SQ, n=19), and small cell lung cancer (SCLC, n=12). Among the 64 lesions, 30 were in the poorly differentiated group and 34 were in the moderately and highly differentiated group. There were statistically significant differences in tumor size and pathological type between the moderately and highly differentiated group and the poorly differentiated group (P<0.05), but there were no statistically significant differences in age, gender, smoking history, or clinical stage (P>0.05). Due to the high degree of malignancy, all small cell carcinomas were classified as poorly differentiated carcinomas (Table 2).
Table 2
Characteristics | Total (n=64) | Poorly differentiated group (n=30) | Moderately and highly differentiated group (n=34) | t/χ2 | P |
---|---|---|---|---|---|
Age, mean ± SD, years | 62.1±8.1* | 61.9±8.2* | 62.2±8.1* | −0.15a | 0.883 |
Size, mean ± SD, cm | 4.2±2.0* | 5.16±2.36* | 3.52±1.29* | 3.38a | 0.002 |
Gender, n (%) | 3.21b | 0.073 | |||
Male | 49 (76.56) | 26 (86.67) | 23 (67.65) | ||
Female | 15 (23.44) | 4 (13.33) | 11 (32.35) | ||
Smoking history, n (%) | 2.49b | 0.115 | |||
Smoker | 36 (56.25) | 20 (66.67) | 16 (47.06) | ||
Never | 28 (43.75) | 10 (33.33) | 18 (52.94) | ||
Clinical stage, n (%) | 2.54b | 0.111 | |||
I, II | 19 (29.69) | 6 (20.00) | 13 (38.24) | ||
III, IV | 45 (70.31) | 24 (80.00) | 21 (61.76) | ||
Histologic type, n (%) | 26.53b | <0.001 | |||
Adenocarcinoma | 33 (51.56) | 6 (20.00) | 27(79.41) | ||
Squamous cell carcinoma | 19 (29.69) | 12 (40.00) | 7(20.59) | ||
Small cell carcinoma | 12 (18.75) | 12 (40.00) | 0 (0.00) |
*, parameter values showed a normal distribution, expressed as mean ± standard deviation. a, an independent sample t-test was used to compare the numerical variables (age, size); statistically significant differences were observed in mass size between the moderately and highly differentiated group and the poorly differentiated group (P<0.05). b, the chi-square test was used to compare the categorical variables (gender, smoking history, clinical stage, and histological type); statistically significant differences were observed in histological type between the moderately and highly differentiated group and the poorly differentiated group (P<0.05). Fisher’s exact test was used when the expected count was <5.
ICC of ADC and T1 values
There were no significant differences in the T1 and ADC values measured by Dr. A and B (both P>0.05) (Table 3). Interobserver agreements for ADC and T1 value were almost perfect [T1 value ICC =0.942, 95% confidence interval (CI): 0.900–0.963; ADC value ICC =0.820, 95% CI: 0.719–0.890] (Figure 2). The average ADC and T1 values were then calculated.
Table 3
Measurement index | Observer A | Observer B | t | P | ICC (95% CI) |
---|---|---|---|---|---|
T1 value (×10−3 msec) | 1,525.81±151.03* | 1,523.82±138.54* | 0.31 | 0.755 | 0.94 (0.900–0.963) |
ADC value (×10−6 mm2/s) | 1,116.07±266.42* | 1,118.28±270.05* | −0.11 | 0.912 | 0.82 (0.719–0.890) |
*, parameter values showed a normal distribution, paired sample t-test was used. ICC, intraclass correlation coefficient; ADC, apparent diffusion coefficient; 95% CI, 95% confidence interval.
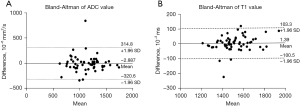
Comparison among lesions of different histological types
The ADC values of SCLC were significantly different from those of SQ (P<0.05) and AD (P<0.001); however, there were no significant differences between those of AD and SQ (P>0.05). On the other hand, the T1 value of AD was significantly different from that of SQ (P<0.001) and SCLC (P<0.001); yet, there were no significant differences between SCLC and SQ (P>0.05). There were statistically significant differences in the average Ki-67 expression index among lung tumors of different pathological types (P<0.05) (Table 4). Two typical cases are shown in Figures 3,4.
Table 4
AD (n=33) | SQ (n=19) | SCLC (n=12) | F | P | |
---|---|---|---|---|---|
ADC value (×10−6 mm2/s), mean ± SD | 1,219.6±201.3* | 1,114.3±281.2* | 840.15±115.39* | 13.48 | <0.001a |
AD vs. SQ | – | – | – | – | 0.097b |
AD vs. SCLC | – | – | – | – | <0.001b |
SQ vs. SCLC | – | – | – | – | 0.001b |
T1 value (×10−3 msec), mean ± SD | 1,446.5±109.7* | 1,589.3±136.1* | 1,638.35±107.9* | 15.72 | <0.001a |
AD vs. SQ | – | – | – | – | <0.001b |
AD vs. SCLC | – | – | – | – | <0.001b |
SQ vs. SCLC | – | – | – | – | 0.263b |
Ki-67 (%), mean ± SD | 31.7±23.3* | 66.5±16.7* | 87.92±8.65* | 35.43 | <0.001a |
AD vs. SQ | – | – | – | – | <0.001b |
AD vs. SCLC | – | – | – | – | <0.001b |
SQ vs. SCLC | – | – | – | – | 0.003b |
*, parameter values showed a normal distribution, expressed as mean ± standard deviation. a, P<0.05, the difference was statistically significant (one-way ANOVA); b, P<0.05, the difference was statistically significant (one-way ANOVA and LSD test). ADC, apparent diffusion coefficient; AD, adenocarcinoma; SQ, squamous cell carcinoma; SCLC, small cell carcinoma; LSD, least significant difference.
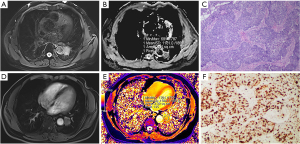
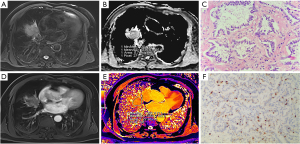
Comparison of groups with different degrees of differentiation
There were statistically significant differences in ADC value and T1 value between the poorly differentiated group and the moderately and highly differentiated group (P<0.001) (Table 5). The ROC curve constructed with T1 values showed an area under the curve (AUC) value of 0.827, an optimal cutoff value of 1,498.5×10−3 msec, a sensitivity of 0.765, and a specificity of 0.846. The ROC curve constructed with ADC values showed an AUC value of 0.839, an optimal cutoff value of 1,059.5×10−6 mm2/s, a sensitivity of 0.882, and a specificity of 0.769. The ROC curve constructed by combining T1 and ADC values showed an AUC value of 0.912, a sensitivity of 0.912 and a specificity of 0.769 (Figure 5).
Table 5
Measurement index | Poorly differentiated group (n=30) | Moderately and highly differentiated group (n=34) | t | P |
---|---|---|---|---|
ADC value (×10−6 mm2/s) | 953.6±208.2 | 1,261.5±203.4 | −5.976 | <0.001 |
T1 value (×10−3 msec) | 1,605.7±121.1 | 1,453.5±121.6 | 5.009 | <0.001 |
Independent sample t-test was used.
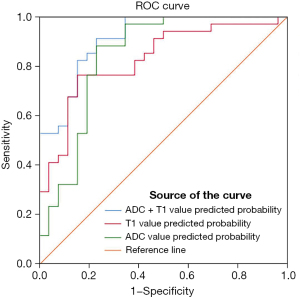
Correlation analysis of the expression index of Ki-67 in lung cancer with ADC and T1 values
Pearson correlation analysis showed that the native T1 value had a significant positive correlation with the expression index of Ki-67 (r=0.66, P<0.001), while ADC value had a significant negative correlation with the expression index of Ki-67 (r=−0.45, P<0.01) (Figure 6).
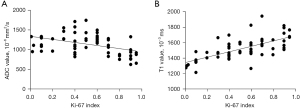
Discussion
In this study, we confirmed that DWI and native T1 value could be used to noninvasively identify the pathological type and differentiation degree of lung cancer. In addition, the combination of the two sequences improves the diagnostic efficacy of the degree of differentiation. Both ADC and T1 values showed almost perfect interobserver agreement, which is consistent with previous research (24-26), thus providing further support for the clinical applicability of this approach. Further, our results also revealed a good correlation between T1 and ADC values with Ki-67 index, providing a new perspective for predicting the malignant degree of lung cancer.
Recently, growing evidence has shown that ADC and T1-mapping are independent imaging biomarkers for characterizing histological cancer type and biological features. However, due to low lung proton density, B0 inhomogeneity and physiological movement of heart and great vessels, differential diagnosis based on ADC in lung tumors has always been a challenge in research. It is therefore uncertain whether ADC can be used as a reliable indicator to differentiate malignancy from other anomalies, as in other organs. With the development of magnetic resonance technology, DW rapid imaging of lung MRI has become possible, which has minimized the effect of gross physiologic motion from respiration and cardiac movement (27). ADC values were significantly reduced in small cell carcinoma compared with adenocarcinoma and squamous cell carcinoma in our study, which is consistent with recent meta-analyses (28). This may be due to the high density of SCLC cells containing large nuclei and almost complete lack of cytoplasm, limiting the diffusion of water molecules and thus reducing the ADC value (28). However, the ADC values of AD and SQ overlapped greatly, and the differences between them were not statistically significant, making it hard to distinguish lung cancer subtypes, especially non-small cell carcinoma subtypes, based on ADC values alone. It has also been suggested that ADC values are useful for distinguishing the degree of cell differentiation of lung cancers (29). The diffusion of water molecules in poorly differentiated tumors is more restricted and their ADC values are lower due to increased cell density and membrane structure (30). The results of our study were consistent with these reports, and ADC values might reflect the characteristics of lung tumours.
In this study, the mean T1 value in patients with lung cancer was in the range of 1,446–1,638 msec, which was slightly higher than that reported previously (1,471–1,603 msec) using the B1-corrected T1-mapping at 3 T MRI (31). We believe that different T1-mapping sequences can lead to variability in measurements. B1-corrected T1-mapping can reduce the effects of magnetic field inhomogeneity but cannot guarantee the correction of potential errors, including phase coding caused by cardiac and vascular motion (32). The Look-Locker method is less affected by the magnetic field inhomogeneity of B1 and has high repeatability. Tirkes et al. (33) found that the MOLLI sequence could be used to obtain more accurate T1 values than the variable flip angle (VFA) sequence compared to T1 relaxation measurements in the pancreas and liver.
The main disadvantages of conventional MR examination currently in use are that they are performed on a qualitative non-parametric MR sequence, allowing only semi-quantitative/qualitative analysis, and that they rely on technical factors inherent in MR signal acquisition, including coil, slice thickness, and repetition time selection, and are subject to observer subjective factors. By contrast, T1 longitudinal relaxation times can be used for quantitative analysis of in vivo histology to determine the characteristics of biological tissues. The T1 sequence used in this study can be performed in a single breath hold and can easily be incorporated into routine MR examinations. It has been shown (21) that the native T1 values of malignant lung tumors or pulmonary tuberculosis are lower than those of non-tuberculous benign lesions, probably because benign lesions contain more water. In our study, we found higher T1 values in SQ and SCLC compared to AD, possibly due to a combination of high cell density and more extracellular matrix (ECM) micronecrotic areas, resulting in higher T1 values in the tissues (34,35). In addition, Peng et al. (36) revealed that the T1-mapping technique could be used to identify the degree of differentiation of hepatocellular carcinoma. Our results indicated that the combination of ADC value and T1 value showed a good ability to differentiate poorly differentiated tumors. Future microscopic and precise comparative analysis of tissue sections and DWI and T1-mapping images is needed to clarify the factors influencing ADC and T1 values in lung cancer. Furthermore, our findings suggest a high interobserver agreement on native T1 values for lung cancer, and we therefore speculate that it may serve as a stable longitudinal parameter to describe and monitor lung cancer.
The invasive nature of tissue biopsy is a factor in the tendency of many centers to avoid routine preoperative biopsy for evaluation of Ki-67 antigen expression (37). In this study, T1 values were significantly and positively correlated with Ki-67 antigen expression index, which may be an option to optimize individualized treatment. Higher Ki-67 index is associated with more active tumor cells, more metabolism, and faster multiplication (38), all of which lead to changes in the tumor cell microenvironment and thus affect the T1 relaxation time. Therefore, quantification of tumor T1 value may be a potential indicator for evaluating disease progression in lung cancer patients. In addition, high expression of Ki-67 is associated with a high proliferation rate of tumor cells (39), where the increased density of tumor cells allows for more restricted diffusion of water molecules and correspondingly lower ADC values. This is consistent with the significant negative correlation between ADC value and Ki-67 index observed in the present study. Further, a moderate to high negative correlation between ADC values and Ki-67 index was also observed in other oncology studies (39-41).
The present study had several limitations. Firstly, heterogeneity within tumor tissue may lead to bias in measurement of ADC and T1 values. Secondly, although larger areas of necrosis were excluded, smaller necrotic regions may have been included in the ROI areas, affecting T1 and ADC measurements. Thirdly, the number of cases in this study was small and the pathologic types involved were limited. Finally, imaging was performed with a single scanner and T1-mapping sequence, and reproducibility across different scanners and field strengths was not tested. In the future, a larger sample size, more types of lung tumors, and different MRI devices will be needed to confirm our results.
In conclusion, our study demonstrated the value of DWI and T1-mapping in distinguishing the pathological type and the degree of tumor differentiation in lung cancer as well as their correlation with Ki-67 index.
Acknowledgments
Funding: This work was supported by the Gusu Medical Talent of Suzhou City Program (grant No. GSWS2020009), the Translational Research Grant of NCRCH (grant No. 2020WSB06), the National Natural Science Foundation of China (grant No. 81671743), the Clinical Key Diseases Diagnosis and Therapy Special Project of Health and Family Planning Commission of Suzhou (grant No. LCZX201801), the Advanced Talents within Six Industries of Jiangsu Province Program (grant No.WSW-057), and the High-level Health Personnel ‘Six-One’ Project of Jiangsu Province in China (grant No. LGY2016035).
Footnote
Reporting Checklist: The authors have completed the STARD reporting checklist. Available at https://jtd.amegroups.com/article/view/10.21037/jtd-22-77/rc
Data Sharing Statement: Available at https://jtd.amegroups.com/article/view/10.21037/jtd-22-77/dss
Conflicts of Interest: All authors have completed the ICMJE uniform disclosure form (available at https://jtd.amegroups.com/article/view/10.21037/jtd-22-77/coif). The authors have no conflicts of interest to declare.
Ethical Statement: The authors are accountable for all aspects of the work in ensuring that questions related to the accuracy or integrity of any part of the work are appropriately investigated and resolved. The study was conducted in accordance with the Declaration of Helsinki (as revised in 2013). It was approved by the Ethics Committee of the First Affiliated Hospital of Soochow University (No. 2020-244) and informed consent was taken from all the patients.
Open Access Statement: This is an Open Access article distributed in accordance with the Creative Commons Attribution-NonCommercial-NoDerivs 4.0 International License (CC BY-NC-ND 4.0), which permits the non-commercial replication and distribution of the article with the strict proviso that no changes or edits are made and the original work is properly cited (including links to both the formal publication through the relevant DOI and the license). See: https://creativecommons.org/licenses/by-nc-nd/4.0/.
References
- Siegel RL, Miller KD, Jemal A. Cancer statistics, 2016. CA Cancer J Clin 2016;66:7-30. [Crossref] [PubMed]
- Stupp R, Monnerat C, Turrisi AT 3rd, et al. Small cell lung cancer: state of the art and future perspectives. Lung Cancer 2004;45:105-17. [Crossref] [PubMed]
- Kim HS, Lee KS, Ohno Y, et al. PET/CT versus MRI for diagnosis, staging, and follow-up of lung cancer. J Magn Reson Imaging 2015;42:247-60. [Crossref] [PubMed]
- Suda K, Tomizawa K, Mitsudomi T. Biological and clinical significance of KRAS mutations in lung cancer: an oncogenic driver that contrasts with EGFR mutation. Cancer Metastasis Rev 2010;29:49-60. [Crossref] [PubMed]
- Warth A, Cortis J, Soltermann A, et al. Tumour cell proliferation (Ki-67) in non-small cell lung cancer: a critical reappraisal of its prognostic role. Br J Cancer 2014;111:1222-9. [Crossref] [PubMed]
- Wei DM, Chen WJ, Meng RM, et al. Augmented expression of Ki-67 is correlated with clinicopathological characteristics and prognosis for lung cancer patients: an up-dated systematic review and meta-analysis with 108 studies and 14,732 patients. Respir Res 2018;19:150. [Crossref] [PubMed]
- Martin B, Paesmans M, Mascaux C, et al. Ki-67 expression and patients survival in lung cancer: systematic review of the literature with meta-analysis. Br J Cancer 2004;91:2018-25. [Crossref] [PubMed]
- Uto T, Takehara Y, Nakamura Y, et al. Higher sensitivity and specificity for diffusion-weighted imaging of malignant lung lesions without apparent diffusion coefficient quantification. Radiology 2009;252:247-54. [Crossref] [PubMed]
- Le Bihan D, Breton E, Lallemand D, et al. Separation of diffusion and perfusion in intravoxel incoherent motion MR imaging. Radiology 1988;168:497-505. [Crossref] [PubMed]
- Chen L, Zhang J, Chen Y, et al. Relationship between apparent diffusion coefficient and tumour cellularity in lung cancer. PLoS One 2014;9:e99865. [Crossref] [PubMed]
- Yabuuchi H, Hatakenaka M, Takayama K, et al. Non-small cell lung cancer: detection of early response to chemotherapy by using contrast-enhanced dynamic and diffusion-weighted MR imaging. Radiology 2011;261:598-604. [Crossref] [PubMed]
- Chang Q, Wu N, Ouyang H, et al. Diffusion-weighted magnetic resonance imaging of lung cancer at 3.0 T: a preliminary study on monitoring diffusion changes during chemoradiation therapy. Clin Imaging 2012;36:98-103. [Crossref] [PubMed]
- Pauls S, Schmidt SA, Juchems MS, et al. Diffusion-weighted MR imaging in comparison to integrated [18F]-FDG PET/CT for N-staging in patients with lung cancer. Eur J Radiol 2012;81:178-82. [Crossref] [PubMed]
- Choi SY, Chang YW, Park HJ, et al. Correlation of the apparent diffusion coefficiency values on diffusion-weighted imaging with prognostic factors for breast cancer. Br J Radiol 2012;85:e474-9. [Crossref] [PubMed]
- Surov A, Ginat DT, Sanverdi E, et al. Use of Diffusion Weighted Imaging in Differentiating Between Maligant and Benign Meningiomas. A Multicenter Analysis. World Neurosurg 2016;88:598-602. [Crossref] [PubMed]
- Haaf P, Garg P, Messroghli DR, et al. Cardiac T1 Mapping and Extracellular Volume (ECV) in clinical practice: a comprehensive review. J Cardiovasc Magn Reson 2016;18:89. [Crossref] [PubMed]
- Germain P, El Ghannudi S, Jeung MY, et al. Native T1 mapping of the heart - a pictorial review. Clin Med Insights Cardiol 2014;8:1-11. [Crossref] [PubMed]
- Jellis CL, Kwon DH. Myocardial T1 mapping: modalities and clinical applications. Cardiovasc Diagn Ther 2014;4:126-37. [PubMed]
- Alamidi DF, Morgan AR, Hubbard Cristinacce PL, et al. COPD Patients Have Short Lung Magnetic Resonance T1 Relaxation Time. COPD 2016;13:153-9. [Crossref] [PubMed]
- Morgan AR, Parker GJ, Roberts C, et al. Feasibility assessment of using oxygen-enhanced magnetic resonance imaging for evaluating the effect of pharmacological treatment in COPD. Eur J Radiol 2014;83:2093-101. [Crossref] [PubMed]
- Yang S, Shan F, Yan Q, et al. A pilot study of native T1-mapping for focal pulmonary lesions in 3.0 T magnetic resonance imaging: size estimation and differential diagnosis. J Thorac Dis 2020;12:2517-28. [Crossref] [PubMed]
- Messroghli DR, Radjenovic A, Kozerke S, et al. Modified Look-Locker inversion recovery (MOLLI) for high-resolution T1 mapping of the heart. Magn Reson Med 2004;52:141-6. [Crossref] [PubMed]
- Landis JR, Koch GG. The measurement of observer agreement for categorical data. Biometrics 1977;33:159-74. [Crossref] [PubMed]
- Alamidi DF, Smailagic A, Bidar AW, et al. Variable flip angle 3D ultrashort echo time (UTE) T1 mapping of mouse lung: A repeatability assessment. J Magn Reson Imaging 2018; [Epub ahead of print]. [Crossref] [PubMed]
- Triphan SM, Jobst BJ, Anjorin A, et al. Reproducibility and comparison of oxygen-enhanced T1 quantification in COPD and asthma patients. PLoS One 2017;12:e0172479. [Crossref] [PubMed]
- Weller A, Papoutsaki MV, Waterton JC, et al. Diffusion-weighted (DW) MRI in lung cancers: ADC test-retest repeatability. Eur Radiol 2017;27:4552-62. [Crossref] [PubMed]
- Keogan MT, Edelman RR. Technologic advances in abdominal MR imaging. Radiology 2001;220:310-20. [Crossref] [PubMed]
- Shen G, Jia Z, Deng H. Apparent diffusion coefficient values of diffusion-weighted imaging for distinguishing focal pulmonary lesions and characterizing the subtype of lung cancer: a meta-analysis. Eur Radiol 2016;26:556-66. [Crossref] [PubMed]
- Matoba M, Tonami H, Kondou T, et al. Lung carcinoma: diffusion-weighted mr imaging--preliminary evaluation with apparent diffusion coefficient. Radiology 2007;243:570-7. [Crossref] [PubMed]
- Winfield JM, Orton MR, Collins DJ, et al. Separation of type and grade in cervical tumours using non-mono-exponential models of diffusion-weighted MRI. Eur Radiol 2017;27:627-36. [Crossref] [PubMed]
- Jiang J, Cui L, Xiao Y, et al. B1 -Corrected T1 Mapping in Lung Cancer: Repeatability, Reproducibility, and Identification of Histological Types. J Magn Reson Imaging 2021;54:1529-40. [Crossref] [PubMed]
- Yoon JH, Lee JM, Kim E, et al. Quantitative Liver Function Analysis: Volumetric T1 Mapping with Fast Multisection B1 Inhomogeneity Correction in Hepatocyte-specific Contrast-enhanced Liver MR Imaging. Radiology 2017;282:408-17. [Crossref] [PubMed]
- Tirkes T, Zhao X, Lin C, et al. Evaluation of variable flip angle, MOLLI, SASHA, and IR-SNAPSHOT pulse sequences for T1 relaxometry and extracellular volume imaging of the pancreas and liver. MAGMA 2019;32:559-66. [Crossref] [PubMed]
- Adams LC, Ralla B, Jurmeister P, et al. Native T1 Mapping as an In vivo Biomarker for the Identification of Higher-Grade Renal Cell Carcinoma: Correlation With Histopathological Findings. Invest Radiol 2019;54:118-28. [Crossref] [PubMed]
- Travis WD, Brambilla E, Nicholson AG, et al. The 2015 World Health Organization Classification of Lung Tumors: Impact of Genetic, Clinical and Radiologic Advances Since the 2004 Classification. J Thorac Oncol 2015;10:1243-60. [Crossref] [PubMed]
- Peng Z, Jiang M, Cai H, et al. Gd-EOB-DTPA-enhanced magnetic resonance imaging combined with T1 mapping predicts the degree of differentiation in hepatocellular carcinoma. BMC Cancer 2016;16:625. [Crossref] [PubMed]
- Tomiyama N, Yasuhara Y, Nakajima Y, et al. CT-guided needle biopsy of lung lesions: a survey of severe complication based on 9783 biopsies in Japan. Eur J Radiol 2006;59:60-4. [Crossref] [PubMed]
- Sun Y, Tong T, Cai S, et al. Apparent Diffusion Coefficient (ADC) value: a potential imaging biomarker that reflects the biological features of rectal cancer. PLoS One 2014;9:e109371. [Crossref] [PubMed]
- Surov A, Caysa H, Wienke A, et al. Correlation Between Different ADC Fractions, Cell Count, Ki-67, Total Nucleic Areas and Average Nucleic Areas in Meningothelial Meningiomas. Anticancer Res 2015;35:6841-6. [PubMed]
- Zhang XY, Sun YS, Tang L, et al. Correlation of diffusion-weighted imaging data with apoptotic and proliferation indexes in CT26 colorectal tumor homografts in balb/c mouse. J Magn Reson Imaging 2011;33:1171-6. [Crossref] [PubMed]
- Mori N, Ota H, Mugikura S, et al. Luminal-type breast cancer: correlation of apparent diffusion coefficients with the Ki-67 labeling index. Radiology 2015;274:66-73. [Crossref] [PubMed]
(English Language Editor: A. Muijlwijk)