Co-infecting pathogens can contribute to inflammatory responses and severe symptoms in COVID-19
Introduction
A newly emerged infectious disease, COVID-19, caused by a novel coronavirus (SARS-CoV-2), was first reported in December 2019. The pneumonia cases infected with SARS-CoV-2 over 253 million and 5 million deaths as of November 2021 (1-3). While most (>80%) cases were characterized by mild symptoms, such as fever, cough, myalgia or fatigue, ground-glass opacity, and hyposmia (2), between 16–19% of cases developed a severe form of the disease, or acute respiratory distress syndrome (ARDS) (4,5), with manifestations such as pulmonary edema, diffuse alveolar injury with the formation of hyaline membranes, fibrinous exudates, hypercytokinemia, severe lymphopenia, and recruitment of multinucleated giant cells (6-8). Patients with severe forms of COVID-19 may develop multiple organ failure or injuries, and prolonged critical illness, which may lead to death (9).
The most studied model of COVID-19 aggravation features a “cytokine storm syndrome” (10), reflecting an over-activation of the immune system that leads to high levels of inflammatory mediators (i.e., cytokines and chemokines) being released into the circulation, resulting in multiple organ damage and failure (11). Severely or critically ill patients with COVID-19 have significantly increased serum levels of inflammatory mediators, including interleukin (IL)-1β, IL-2, IL-6, IL-8, IL-10, granulocyte-macrophage colony-stimulating factor, chemokines (CCL2, CCL3, CCL5), interferon (IFN)-γ-induced protein 10 (IP-10), tumor necrosis factor-α (TNF-α) and IFN-γ (12-14). Among these, IL-6 and IL-10 have been the most stable biomarkers of disease severity. In addition to serum, up-regulated cytokine and chemokine levels were also observed in the respiratory system as a primary pathology (15), in which IL-6, IL-8, IL-1β, and a number of chemokines had significantly elevated levels in bronchoalveolar lavage fluid (BALF) or macrophages from cases with severe COVID-19 (16,17).
While most studies have focused on COVID-19 induced mobility and mortality, co-infection and secondary infection with other pathogens may also impact mobility and mortality. For example, co-infection or lysis of bacteria in the setting of viral pneumonia can generate inflammation and acute lung injury (18), as demonstrated for influenza viruses (19,20), SARS-CoV (21-23), and MERS-CoV infections (23,24). In the case of COVID-19, several viral, bacterial, and fungal pathogens have been identified alongside SARS-CoV-2, including Streptococcus pneumoniae, Klebsiella pneumoniae, Aspergillus fumigatus (A. fumigatus), Acinetobacter baumannii (A. baumannii), Candida albicans, Mycoplasma, cytomegalovirus (CMV), and influenza A/B viruses (25-27). While the estimated rate of co-infection/superinfection varies substantially (2.1–20.7%) among different studies (23,27,28), a higher proportion was observed in patients with COVID-19 in intensive care unit (ICU) compared with those not in ICU (26,29). Patients with COVID-19 experiencing co-infection/superinfection were likely to experience worse clinical outcomes (30). An early study involving 191 inpatients from Wuhan found that 50% of non-survivors had secondary bacterial infections (6).
Despite the enormous research effort to determine the mechanisms underlying the severe manifestation of COVID-19, our understanding remains incomplete. It is unclear how SARS-CoV-2 interacts with other co-infecting pathogens and how this may impact the host immune response. To address this issue, we investigated (I) the presence and abundance of all pathogens (defined here as the total “infectome” within patients) from upper and lower respiratory tract (URT and LRT) samples, and (II) serum cytokine and chemokine levels throughout the course of the disease in four cases with severe or critical COVID-19 in an ICU in China. We employed a total RNA sequencing (i.e., meta-transcriptomics) approach that can simultaneously reveal the transcriptome of virus, host, and other microbes within a sample. Using this approach we identified, for the first time and at high resolution, a complex interaction between microbial pathogens during the development of COVID-19. We present the following article in accordance with the STROBE reporting checklist (available at https://jtd.amegroups.com/article/view/10.21037/jtd-21-1284/rc).
Methods
Sample collection
Four cases with COVID-19 were included in this study, all of which had laboratory-confirmed SARS-CoV-2 infection and were admitted to the ICU at Dongguan People’s Hospital. The target conditions were severe or critical COVID-19 (Table 1) (31). The dates of admission, age, clinical history, clinical scores, intervention measures, microbiological investigations, and clinical outcomes were collected at the earliest possible time points upon hospitalization. Day 1 of clinical onset was defined as the first day of the appearance of clinical symptoms. Three patients were in a critical condition and one was classified as severe according to the guidelines of SARS-CoV-2 infection from the World Health Organization (Table 1) (31). Sample collection began February 7th and ended August 8th 2020 (until discharged or death). Samples were collected by different clinicians who were provided with the same collection guidelines once every 2 days. The sampling types included throat swab, BALF, sputum, and whole blood, most of which were collected for routine diagnostic purposes. The corresponding healthy control and reagent samples were collected from healthy volunteers and sample collection medium from the hospital. Additional selection criteria for cases involved in the downstream experiments included the availability, timing, and condition of samples. The study was conducted in accordance with the Declaration of Helsinki (as revised in 2013). The study was approved by ethics committee of the First Affiliated Hospital of Guangzhou Medical University (No. 2020-85) and Dongguan People’s Hospital (No. KYKT2020-005-A1) approved the sampling procedure and the use of patient samples for this study. Informed consent was obtained from each patient.
Table 1
Classification | Severity definitions |
---|---|
Critical COVID-19 | (I) The criteria for ARDS, sepsis, septic shock, or other conditions that would normally require the provision of life-sustaining therapies such as mechanical ventilation (invasive or non-invasive) or vasopressor therapy |
Severe COVID-19 | (I) Oxygen saturation <90% on room air |
(II) Respiratory rate >30 breaths/min in adults and children >5 years old; ≥60 breaths/min in children <2 months old; ≥50 in children 2–11 months old; and ≥40 in children 1–5 years old | |
(III) Signs of severe respiratory distress (accessory muscle use, inability to complete full sentences, and, in children, very severe chest wall indrawing, grunting, central cyanosis, or presence of any other general danger signs) | |
Non-severe COVID-19 | (I) Absence of any criteria for severe or critical COVID-19 |
The table is from the reference (31). It is a derivative of “WHO severity definitions” from WHO et al., WHO Therapeutics and COVID-19: living guideline, WHO/2019-nCoV/therapeutics/2021.3, used under CC BY-NC-SA 3.0 IGO license. ARDS, acute respiratory distress syndrome.
RNA purification and quantitative reverse transcription PCR (qRT-PCR) detection of SARS-CoV-2
The peripheral BALF, sputum, throat swab, and whole blood samples were collected from the patients at various time points after hospitalization. Samples were collected by different clinicians who were provided with the same collection guidelines. Viral RNAs were extracted from samples using the QIAamp RNA Viral Kit (Qiagen, Heiden, Germany), in accordance with the manufacturer’s instructions in a biosafety II laboratory after sample inactivation. The qRT-PCR assay was performed using the 2019-nCoV Nucleic Acid Detection Kit (GENEODX, Shanghai, China).
Meta-transcriptomic sequencing and data analyses
For each sample, total RNA was quantified using the Qubit RNA HS Assay Kit (Thermo Fisher Scientific, Waltham, MA, USA) and then reverse transcribed and amplified using an Ovation Trio RNA Seq Library Preparation Kit (NuGEN, CA, USA). The subsequent libraries were sequenced with an Illumina Novaseq platform at Novagene (Beijing, China). For each library, poor quality reads, adaptor sequences, non-complex reads, and duplicated reads were removed using the BBmap software package. Ribosomal RNA (rRNA) reads from human and other organisms were similarly removed by mapping against a comprehensive collection of large and small subunit rRNA sequences downloaded from the SILVA database (32). The remaining reads were incorporated into a pathogen discovery pipeline. Virus identification was performed by directly comparing reads against reference virus genome databases using the blastn program and against the non-redundant protein (nr) database using the diamond blastx program (33). Bacteria and fungi were first identified using MetaPhlAn2 program (34) and then confirmed by inspecting the mapped reads. The abundance level of each pathogen was estimated by mapping reads against the corresponding genomes (mitochondrial in the case of fungi) and measured using reads per million (RPM); total viral reads/total non-redundant reads × 1 million. A “positive” hit for the presence of a microbe was noted if the abundance level was larger than 1 RPM. To prevent false-positives resulting from index hopping during sequencing, we used a threshold of 0.1% of total read abundance for samples sharing the same sequencing lane. Host transcriptomes were characterized using a HISAT-StringTie protocol (35).
Measurement of cytokines and chemokines
Serum cytokine and chemokine levels were quantified by the Bio-Plex method using the BioPlex 2200 Multiplex Testing System (Bio-Rad, Hercules, CA, USA) in accordance with the manufacturer’s instructions. The capture sandwich immunoassay format was designed on magnetic beads. The capture antibody-coupled beads were first incubated with antigen standards, samples, or controls, followed by incubation with biotinylated detection antibodies and reporter streptavidin-phycoerythrin conjugate. Beads were then passed through the Bio-Plex 200 suspension array reader, which was equipped with lasers that measure the fluorescence of the beads and bound streptavidin-phycoerythrin. A high-speed digital processor managed the data output, and the Bio-Plex Manager™ 6.0 software presented the concentration results in pg/mL. The total amount of cytokines in individual samples was then recalculated based on their protein content measured by the Lowry method. This allowed quantitative measurements for 48 different chemokines, cytokines, growth factors, and immune mediators, including IL-1α, IL-1β, IL-1Ra, IL-6, IL-8, IL-10, IL-18, TNF-α, IFN-γ, IP-10, CCL2 (MCP-1), CCL3 (MIP-1α), and CCL5 (RANTES) in 25-µL samples.
Statistical analysis
For cases 1 and 2 who experienced an extended disease course, the strength of correlations among clinical data [i.e., Murray score, Clinical Pulmonary Infection Score (CPIS)], pathogen abundance (including both SARS-CoV-2 and co-infected pathogens), cytokine levels (estimated by meta-transcriptomics and Bio-Plex), and convalescent plasma therapy was evaluated using Pearson correlations or Point-Biserial correlations. We also examined the correlation between SARS-CoV-2 abundance levels estimated by meta-transcriptomics and those estimated by qRT-PCR. The levels of serum inflammatory mediators were evaluated using one-way analysis of variance among healthy controls, acute-phase patients, and the recovery phase of cases with COVID-19. Throughout the text, figures, and legends, the following terminology is used to show statistical significance: *, P<0.05; **, P<0.01; ***, P<0.001.
Data availability
All non-human reads were deposited in the sequence read archive (SRA) databases under the SRA submission: SUB8556497.
Results
Clinical characteristics and primary interventions of four patients with COVID-19 in ICU
Four patients with COVID-19 were enrolled in this study, with SARS-CoV-2 infection confirmed by Dongguan Center for Disease Control and Prevention. All patients had a history of exposure to a confirmed case of COVID-19 or traveled to Hubei Province prior to the onset of disease, and three had pre-existing conditions; chronic kidney disease (case 1), hypertension (cases 2 and 3), diabetes and Parkinson’s disease (case 3). All cases developed severe or critical COVID-19 and were admitted to ICU, where invasive mechanical ventilation (all cases) and extracorporeal membrane oxygenation (ECMO) (cases 1 and 4) were administered. To inhibit viral infection and mitigate inflammatory responses, antiviral therapy (all cases), small dosage and short-term glucocorticoids (cases 1, 2, and 3), and convalescent plasma therapies (cases 1 and 2) were administered (Figure 1, Table 2). To help prevent secondary infections, all patients were given antibacterial therapy before admission to the ICU, and three (cases 1, 2, and 4) had antifungal therapy at different stages during treatment (Figure 1, Table 1). After treatment, case 3 recovered after 27 days in hospital, while cases 1 and 2 showed prolonged infection and were transferred from isolated ICU to ICU on the 52nd day after onset. Case 2 eventually recovered and was discharged on the 64th day after onset of disease, while cases 1 and 4 died after the 192nd and 25th day in hospital, respectively.
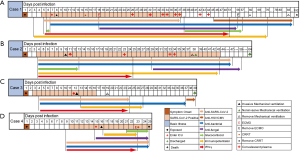
Table 2
Characteristics | Case | |||
---|---|---|---|---|
1 | 2 | 3 | 4 | |
Clinical symptoms | ||||
Fever | Yes | Yes | Yes | Yes |
Maximal temperature (°C) | 39.2 | 38.5 | 37.5 | 38.8 |
Cough | Yes | Yes | Yes | No |
Dyspnoea or tachypnoea | Yes | Yes | Yes | Yes |
Headache | No | No | No | No |
Fatigue | No | No | No | No |
Sputum production | Yes | Yes | Yes | No |
Diarrhea or vomiting | Yes | Yes | No | No |
Complications | ||||
Pneumonia | Yes | Yes | Yes | Yes |
ARDS | Yes | Yes | Yes | Yes |
Acute kidney injury | Yes | No | No | No |
Shock | No | No | No | Yes |
Other virus co-infection | CMV (mNGS) | HSV (mNGS) | HSV (mNGS) | No |
HSV (NGS) | ||||
Bacteria coinfection | M. odoratus (mNGS) | No | No | A. baumannii (culture) |
Fungus coinfection | A. fumigatus (mNGS) | Candida tropicalis (mNGS) | A. fumigatus (mNGS) | No |
Candida tropicalis (mNGS) | Candida tropicalis (mNGS) | |||
Others | No | M. orale (mNGS) | No | No |
Treatment | ||||
Anti-viral | Arbidol | Arbidol | Arbidol | Arbidol |
Lopinavir-Ritonavir | Lopinavir-Ritonavir | Lopinavir-Ritonavir | Lopinavir-Ritonavir | |
Oseltamivir | Oseltamivir | XueBiJing | Oseltamivir | |
Interferon | Interferon | Interferon | Interferon | |
Ganciclovir | Hydroxychloroquine | |||
Acyclovir | ||||
Famciclovir | ||||
Valacyclovir | ||||
Anti-bacterial | Sulperazon | Sulperazon | Sulperazon | Sulperazon |
Linezolid | Linezolid | Linezolid | Linezolid | |
Meropenem | Meropenem | Meropenem | Meropenem | |
Tigecycline | Levofloxacin | Moxifloxacin | Moxifloxacin | |
Polymyxin | Ceftazidime | 4-Quinolones | Vancomycin | |
Tazocin | Tigecycline | Cefuroxim | Tigecycline | |
Ceftazidime | ||||
Vancomycin | ||||
Fortum | ||||
Piperacillin | ||||
Levofloxacin | ||||
Tazobactam | ||||
Anti-fungal | Amphotericin B | Amphotericin B | No | Cancidas |
Cancidas | Cancidas | Caspofun | ||
Voriconazole | Voriconazole | |||
Glucocorticoid | Methylprednisolone | Norepinephrine | No | Methylprednisolone |
Hydrocortisone | ||||
Noradrenaline | ||||
Convalescent plasma | Yes | Yes | No | No |
Oxygen inhalation | Yes | Yes | Yes | Yes |
Mechanical ventilation | Yes | Yes | Yes | Yes |
ECMO | Yes | No | No | Yes |
CRRT | Yes | Yes | No | Yes |
Intravenous immune globulin | Yes | Yes | Yes | Yes |
Duration of hospitalization, days | 192 | 48 | 39 | 25 |
Clinical outcome | Death (192 days after onset) | Discharged | Discharged | Death |
ARDS, acute respiratory distress syndrome; CMV, cytomegalovirus; HSV, herpes simplex virus; mNGS, meta-transcriptomics next generation sequence; ECMO, extracorporeal membrane oxygenation; CRRT, continuous renal replacement therapy; M. odoratus, Myroides odoratus; A. fumigatus, Aspergillus fumigatus; M. orale, Mycoplasma orale.
For cases 1 and 2, we closely followed both the lung injury status and viral/microbial infection status, measured by Murray score and CPIS, and C-reactive protein (CRP), respectively, throughout the entire course of the disease (36,37). The highest Murray scores were observed at the early phase of ICU treatment (Figure 2A), implying severe acute lung injury/ARDS. Murray scores steadily dropped from a plateau (day 22 for case 1 and day 25 for case 2) and remained at relatively low levels (<2). Conversely, CPIS and CRP curves fluctuated in cases 1 and 2 (Figure 2). Indeed, CPIS had at least three major peaks in case 1 (Figure 2A), suggesting continually changing pathogen diversity or abundance during the entire course of COVID-19.
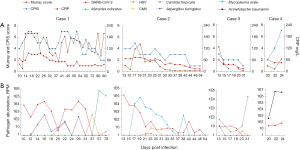
Total infectome in patients with severe/critical COVID-19
We closely monitored the dynamics of infectious pathogens within COVID-19 patients by studying the abundance level of SARS-CoV-2 and other respiratory pathogens using meta-transcriptomic analyses of respiratory and blood samples. The co-infecting pathogens detected were herpes simplex virus (HSV), CMV, Myroides odoratus (M. odoratus), A. fumigatus, Mycoplasma orale (M. orale), A. baumannii and Candida tropicalis (Figure 2B). Importantly, the abundance level of SARS-CoV-2, measured as RPM, showed a high correlation with those estimated by an RT-PCR assay [SARS-CoV-2 cycle threshold (Ct) value], and therefore provided a reliable means to quantify pathogen activity (r=−0.907; P<0.001; Figure S1). These co-pathogens appeared and proliferated at different course stages of COVID-19 and some persisted even after SARS-CoV-2 had become undetectable (Figure 2). For example, in case 1, early infection (before day 31) was characterized by a high abundance of SARS-CoV-2 (up to 3×104 RPM) and relatively high abundance of A. fumigatus (up to 5×102 RPM), with the two pathogens exhibiting broadly synchronous dynamics. Following the decline and disappearance of SARS-CoV-2 at day 38, case 1 remained critically ill and experienced a surge of CMV (2 RPM) at day 38, followed by proliferation of the opportunistic pathogen M. odoratus (>105 RPM) at a later stage of hospitalization (from day 52; Figure 2B). In case 2, the levels of SARS-CoV-2 steadily declined from day 13, and remained low from day 25, although the virus was never completely cleared from the system until the week before release. In addition, case 2 was infected with M. orale, and its abundance peaked at day 19, by which time it was the dominant pathogen in the URT (< day 13; Figure 2B). In addition to M. orale, case 2 also experienced HSV co-infection between days 21 and 27. In case 3, high levels of Candida tropicalis were detected at a later stage of infection (day 31; Figure 2B). In case 4, high levels of multidrug-resistant A. baumannii were detected in the respiratory system before death (days 22 and 24; Figure 2B). In cases 3 and 4, the levels of SARS-CoV-2 remained low (<100 RPM) during the entire course of our study.
We also compared the trend in Murray score, which measures lung injury status, with the changing dynamics of the infectome. This comparison revealed an association between clinical manifestation in the lungs and the timing and intensity of some pathogens (e.g., SARS-CoV-2, A. fumigatus, M. orale) but not others (e.g., Candida tropicalis). Conversely, CPIS and CRP levels, that monitor infections in general, showed a resemblance to the dynamics of the total infectome rather than individual pathogens (Figure 2).
Host responses in patients with severe/critical COVID-19 during disease progression
We followed the levels of inflammatory mediators in serum (protein levels) as well as the URT and LRT (mRNA levels) during the entire course of severe COVID-19. This analysis revealed dynamic changes in certain mediators in response to SARS-CoV-2 and other pathogens in the host. The general pattern of levels of inflammatory mediators reflected the observed pathogen dynamics. In cases 1 and 2, higher levels of inflammatory mediators were observed in blood at the early stage of ICU treatment when SARS-CoV-2 abundance was high, although some lasted for only a brief period (4–8 days) (Figure 3). In addition, the peak in abundance was comparable between cases 1 and 2, except for IL-6; the peak for IL-6 in case 1 (705.27 pg/mL) was 10 times higher than that in case 2 (69.25 pg/mL) (Figure S2).
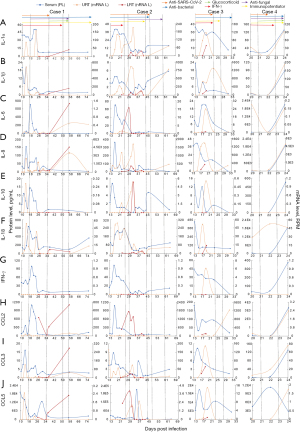
However, SARS-CoV-2 was not alone in exhibiting high levels in parallel with mediators of inflammatory responses (Figure 4). In addition to SARS-CoV-2, the proliferation of other pathogens, such as A. baumannii and M. odoratus, was associated with elevated inflammatory responses in other patients (Figure 3). In case 1, the rising levels of M. odoratus (>105 RPM) at day 56 (Figure 2B) were associated with a significant increase in IL-1β, IL-8, CCL2, and CCL3 mRNA levels in the LRT, although the levels of these mediators remained low in the blood (Figures 2A,3A,3D,3H,3I). In case 4, very high levels of many inflammatory mediators, including IL-1α, IL-1β, IL-6, IL-8, IL-10, IFN-γ, CCL2, and CCL3, were found in blood and the URT at day 24, 2 days before the patient’s death. This was seemingly associated with intense replication of A. baumannii (>106 RPM, or 23.4% of total RNA) in the respiratory tract (Figures 2B,3).
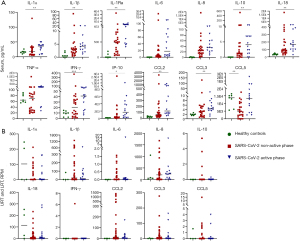
Interestingly, there were marked differences in some inflammatory mediators between blood and the URT and LRT. For example, blood levels of IL-6 and IFN-γ peaked at the early phase of infection in cases 1 (days 14–26) and 2 (days 15–21), although mRNA expression levels in the respiratory tract remained undetectable in both patients during the same period (Figures 2,3C,3G). Indeed, there was a general lack of association between inflammatory mediators in the blood and URT (Figure S3). Furthermore, differences in mediator levels between the URT and LRT were also observed. In case 2, the LRT experienced peak levels of IL-6 (up to 15 RPM) and CCL2 (up to 103 RPM) between days 22 and 33 (Figure 3), but this was not observed in the URT. The HSV appeared during this period but was only detected between days 23 to 31 in the URT and at relatively low levels (<10 RPM).
To demonstrate that SARS-CoV-2 may not be the only microbial factor that results in high levels of inflammatory mediators, samples from the four patients were divided into SARS-CoV-2 (I) non-active and (II) active groups, defined based on SARS-CoV-2 RPM levels (< or >102 RPM). Interestingly, high levels of inflammatory mediators appeared in both active and non-active groups (Figure 4A), and similar observations were made for URT and LRT samples (mRNA levels; Figure 4B). However, it is important to note that the extremely high levels of IL-6 and IL-10 were most likely associated with A. baumannii infections (Figure S4).
Correlations among clinical score, total infectome, and cytokine levels
To determine if pulmonary injury was related to pathogen abundance and inflammatory mediator levels, correlations among the Murray score/CPIS, the total infectome (including SARS-CoV-2 and other pathogens), and inflammatory mediator levels were examined for all four patients. In cases 1 and 2, the Murray score was significantly correlated with CPIS and several inflammatory mediators in the blood (i.e., IL-1Ra, IL-6, IL-18, TNF-α, and IP-10) (Figure 5), suggesting that pathogens and inflammatory responses are critical in causing severe symptoms in the lungs. Conversely, SARS-CoV-2 was significantly correlated with the Murray score in case 1 (r=0.636; P<0.05) but not case 2 (r=0.485; P=0.067). For case 2, M. orale had significant correlations with the Murray score (r=0.631; P<0.05) and many inflammatory mediators in the blood (i.e., IL-1α, IL-1Ra, IL-6, IL-8, IL-18, TNF-α, IFN-γ, IP-10, and CCL2) and respiratory tract (i.e., IL-1α, IL-8, and IL-18), whereas SARS-CoV-2 had significant positive correlations with IL-10, TNF-α, IP-10, and CCL3 (Figure 5B). In addition to “infectomes”, the levels of inflammatory mediators were also likely to reflect clinical intervention. For example, a negative correlation was identified between the use of convalescent plasma and IL-1β, TNF-α, and IL-10 (Figure 5B).
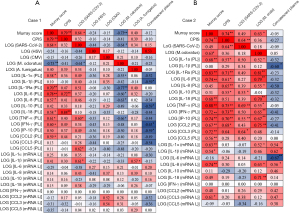
Discussion
Our study used an expansive meta-transcriptomics approach to characterize the total infectome and host responses in patients experiencing severe COVID-19. By revealing the changing abundance levels of a diverse array of pathogens (including SARS-CoV-2) in the context of an ever-changing host immune response, we show that the cytokine storms associated with acute lung injury and other severe clinical outcomes are not exclusively linked to the ongoing presence of SARS-CoV-2, especially toward the later course of the disease. These results suggest the need to consider the complex nature of pathogen co-infections and host interactions within each patient and to trace their changing dynamics throughout the course of the disease, especially for those experiencing prolonged periods of hospitalization. Consequently, these results indicate the need for a precision medicine approach to investigate both the infection and the host response, optimally and daily.
The meta-transcriptomics analysis also revealed at least eight pathogens in the four cases studied, with each patient containing 1–5 co-infecting pathogens. This is a higher frequency of co-infection than previously observed (i.e., 2.1–20.7%) (23,27). However, most previous studies have been based on diagnostic reports from hospitals or qPCR assays for a fixed panel of pathogens, and have been restricted by the selection of target pathogens, pathogen discovery methods, and often a single time point analysis. In contrast, the current meta-transcriptomics approach, although more expensive and time-consuming in practice, revealed all potential pathogens actively expressing RNA molecules within the host throughout the course of the disease (27,38,39). Using this strategy, we identified an opportunistic pathogen (M. odoratus) in case 1 that is not typically included in screening panels for respiratory pathogens, and it reached alarmingly high levels (>105 RPM, or 20.87% of total RNA). The patient in question eventually died, long after SARS-CoV-2 was cleared by the immune system.
Our results confirmed that cytokine storms contributed to acute lung injury, and occurring in both the peripheral circulation and, to a lesser extent, in the respiratory track. However, substantial differences exist in the timing of cytokine release and target pathogens. Importantly, the data generated here suggest that SARS-CoV-2 may not be the only pathogen contributing to the strong chemotactic and inflammatory responses (Figures 4,5), although it is most likely responsible for the initial surge in the levels of various inflammatory mediators (Figures 3,4) (14). Indeed, animal models with influenza virus infection demonstrate significantly higher morbidity and mortality during bacterial superinfection in comparisons to single viral infections (18). In the case of SARS-CoV-2, bacterial and fungal co-infection was associated with a 2.5-fold increase in the risk of death in cases with SARS-CoV-2, suggesting that the immunomodulation induced or enhanced by these pathogens was not trivial (40).
Based on the results for patients in the current study, we propose two possible models for co-pathogen induced immunomodulation. In the first model, the cytokine storm is largely induced by the co-infecting pathogens. This was potentially observed in case 4, where a much stronger cytokine storm was observed with the infection of A. baumannii, a common hospital-acquired pathogen, after SARS-CoV-2 infection was almost cleared from the system. Severe infections caused by hospital-acquired A. baumannii are commonplace and believed to trigger lipopolysaccharide-Toll-like receptor 4 (TLR4)-mediated sepsis (41). In the second model, a combination of SARS-CoV-2 and co-infecting pathogens is involved in triggering a cytokine storm, potentially with a synergistic effect. In our study, A. fumigatus and M. orale co-appeared with SARS-CoV-2 in cases 1 and 2, respectively, and M. orale had significant correlations with multiple inflammatory mediators and the Murray score. Although the mechanism for the later correlations remains unclear, it has been suggested that heat shock protein production by M. orale may activate macrophages to secrete pro-inflammatory cytokines, thus activating immunomodulation (42,43). Furthermore, it is important to note that the dynamics of inflammatory mediators varied substantially among different cases, even for those showing a similar disease course. Because these patients had distinct “infectomes”, it is highly likely that such differences contribute to the distinct inflammatory responses.
In addition to bacteria and fungi, the current data also revealed frequent co-infection with human herpesviruses while the patient’s received treatment for COVID-19. Indeed, HSV was detected in three of the four cases, and CMV was detected in the blood of case 1. The timing of the proliferation of herpesviruses followed the cytokine storm and disorders in immunity, although herpesvirus infection has been reported following the immunomodulatory therapies to dampen the cytokine storm (44). Importantly, reactivation of herpesvirus under immunosuppression conditions can have dire clinical consequences, such as liver impairment or failure (44,45), which may further aggravate COVID-19. Furthermore, many herpesvirus infections may lack overt symptoms in the early stages of infection. Among the cases positive for herpesviruses, only case 1 developed symptoms of disseminated skin herpes, and despite the detection of viruses as early as day 31, related symptoms did not appear until day 43, prompting treatment with Ganciclovir, Famciclovir, Valacyclovir, and Bactroban (Mecobalamin with adjuvant therapy), with the condition beginning to improve on day 47 (44,45). Therefore, we suggest that herpesvirus infection be actively monitored throughout the entire course of COVID-19.
Our results have revealed substantial differences between serum levels of inflammatory mediators and mRNA levels of these mediators in the URT and LRT. One possibility is that these discrepancies are caused by differences in local versus systemic immune responses (46,47). Indeed, several mediators are highly expressed in the URT and/or LRT, but are absent in the blood, as expected with many locally confined infections. Nevertheless, in the current study, respiratory tract measurements were largely associated with the URT, with most data for LRT missing because of sparse sampling. Therefore, the current respiratory tract data are incomplete and hence cannot be used for conclusive comparisons. Another potential explanation is that discrepancies in measurements of inflammatory mediators reflect differences in the measurement approach used. Inflammatory mediators in the serum were measured with a protein assay, while those in the URT and LRT were measured at the mRNA level. The latter approach may not have sufficient resolution for differential expression comparisons if the overall expression level of the protein (e.g., IL6, IL-10, and IFN-γ) is low. Therefore, while our data suggest potential differences between inflammatory mediators in the blood and respiratory systems, further studies with more complete data points and a consistent analytical method are required to confirm this.
Despite the importance of the current observations, we note several limitations of our study. First, only four severely or critically ill patients with COVID-19 were included, so the sample size is too small to draw generalized conclusions. Second, samples were collected after patients were admitted to the ICU, and there was no information on the early phase of the disease. As a result, we cannot determine whether these cases were experiencing nosocomial infections. Third, this study lacks a substantial number of lower respiratory samples, such as sputum or BALF, throughout the course of COVID-19, limiting our ability to characterize the total infectome and host responses at these locations.
In summary, our study was able to demonstrate the complexity of the COVID-19 infectome, the potential interactions of pathogens with the host, and their potential roles in disease progression in severe and critically ill patients.
Acknowledgments
Funding: This work was supported by the National Natural Science Foundation of China (grant number 81761128014), Guangdong Province “Pearl River Talent Plan” Innovation and Entrepreneurship Team Project (grant number 2019ZT08Y464), Open Project of State Key Laboratory of Respiratory Disease (grant number SKLRD-OP-202001), Zhongnanshan Medical Foundation of Guangdong Province (grant number ZNSA-2020012), Guangdong Basic and Applied Basic Research Fund Project (grant number 2020B1515120045), Special Project for Scientific and Technological Development and Emergency Response in COVID-19 Prevention and Control of Guangdong Province (grant number 2020A111129028), Special Project for Research and Promotion of Prevention and Control Techniques of COVID-19 and Emergency Response in Dongguan City (grant number 202071715001114), Macao Science and Technology Development Fund (grant number 0042/2020/A), Guangzhou Institute of Respiratory Health Open Project (Funds provided by China Evergrande Group) (grant number 2020GIRHHMS01), and ARC Australian Laureate Fellowship (grant number FL170100022).
Footnote
Reporting Checklist: The authors have completed the STROBE reporting checklist. Available at https://jtd.amegroups.com/article/view/10.21037/jtd-21-1284/rc
Data Sharing Statement: Available at https://jtd.amegroups.com/article/view/10.21037/jtd-21-1284/dss
Peer Review File: Available at https://jtd.amegroups.com/article/view/10.21037/jtd-21-1284/prf
Conflicts of Interest: All authors have completed the ICMJE uniform disclosure form (available at https://jtd.amegroups.com/article/view/10.21037/jtd-21-1284/coif). CL and XB were employed by the medical company Novogene Bioinformatics Technology Co., Ltd. and responsible for completing the library sequencing. The other authors have no conflicts of interests to declare.
Ethical Statement: The authors are accountable for all aspects of the work in ensuring that questions related to the accuracy or integrity of any part of the work are appropriately investigated and resolved. The study was conducted in accordance with the Declaration of Helsinki (as revised in 2013). The study was approved by ethics committee of the First Affiliated Hospital of Guangzhou Medical University (No. 2020-85) and Dongguan People’s Hospital (No. KYKT2020-005-A1) approved the sampling procedure and the use of patient samples for this study. Informed consent was obtained from each patient.
Open Access Statement: This is an Open Access article distributed in accordance with the Creative Commons Attribution-NonCommercial-NoDerivs 4.0 International License (CC BY-NC-ND 4.0), which permits the non-commercial replication and distribution of the article with the strict proviso that no changes or edits are made and the original work is properly cited (including links to both the formal publication through the relevant DOI and the license). See: https://creativecommons.org/licenses/by-nc-nd/4.0/.
References
- World Health Organization. WHO Coronavirus (COVID-19) Dashboard. Available online: https://covid19.who.int/ (Accessed on 17 Nov 2021).
- Guan WJ, Ni ZY, Hu Y, et al. Clinical Characteristics of Coronavirus Disease 2019 in China. N Engl J Med 2020;382:1708-20. [Crossref] [PubMed]
- Chen L, Liu W, Zhang Q, et al. RNA based mNGS approach identifies a novel human coronavirus from two individual pneumonia cases in 2019 Wuhan outbreak. Emerg Microbes Infect 2020;9:313-9. [Crossref] [PubMed]
- Wu Z, McGoogan JM. Characteristics of and Important Lessons From the Coronavirus Disease 2019 (COVID-19) Outbreak in China: Summary of a Report of 72 314 Cases From the Chinese Center for Disease Control and Prevention. JAMA 2020;323:1239-42. [Crossref] [PubMed]
- Liang WH, Guan WJ, Li CC, et al. Clinical characteristics and outcomes of hospitalised patients with COVID-19 treated in Hubei (epicentre) and outside Hubei (non-epicentre): a nationwide analysis of China. Eur Respir J 2020;55:2000562. [Crossref] [PubMed]
- Zhou F, Yu T, Du R, et al. Clinical course and risk factors for mortality of adult inpatients with COVID-19 in Wuhan, China: a retrospective cohort study. Lancet 2020;395:1054-62. [Crossref] [PubMed]
- Zhang W, Zhao Y, Zhang F, et al. The use of anti-inflammatory drugs in the treatment of people with severe coronavirus disease 2019 (COVID-19): The Perspectives of clinical immunologists from China. Clin Immunol 2020;214:108393. [Crossref] [PubMed]
- Chung M, Bernheim A, Mei X, et al. CT Imaging Features of 2019 Novel Coronavirus (2019-nCoV). Radiology 2020;295:202-7. [Crossref] [PubMed]
- Berlin DA, Gulick RM, Martinez FJ. Severe Covid-19. N Engl J Med 2020;383:2451-60. [Crossref] [PubMed]
- Mehta P, McAuley DF, Brown M, et al. COVID-19: consider cytokine storm syndromes and immunosuppression. Lancet 2020;395:1033-4. [Crossref] [PubMed]
- Chousterman BG, Swirski FK, Weber GF. Cytokine storm and sepsis disease pathogenesis. Semin Immunopathol 2017;39:517-28. [Crossref] [PubMed]
- Huang C, Wang Y, Li X, et al. Clinical features of patients infected with 2019 novel coronavirus in Wuhan, China. Lancet 2020;395:497-506. [Crossref] [PubMed]
- Chen G, Wu D, Guo W, et al. Clinical and immunological features of severe and moderate coronavirus disease 2019. J Clin Invest 2020;130:2620-9. [Crossref] [PubMed]
- Liu J, Li S, Liu J, et al. Longitudinal characteristics of lymphocyte responses and cytokine profiles in the peripheral blood of SARS-CoV-2 infected patients. EBioMedicine 2020;55:102763. [Crossref] [PubMed]
- Buszko M, Park JH, Verthelyi D, et al. The dynamic changes in cytokine responses in COVID-19: a snapshot of the current state of knowledge. Nat Immunol 2020;21:1146-51. [Crossref] [PubMed]
- Zhou Z, Ren L, Zhang L, et al. Heightened Innate Immune Responses in the Respiratory Tract of COVID-19 Patients. Cell Host Microbe 2020;27:883-890.e2. [Crossref] [PubMed]
- Liao M, Liu Y, Yuan J, et al. Single-cell landscape of bronchoalveolar immune cells in patients with COVID-19. Nat Med 2020;26:842-4. [Crossref] [PubMed]
- McCullers JA. The co-pathogenesis of influenza viruses with bacteria in the lung. Nat Rev Microbiol 2014;12:252-62. [Crossref] [PubMed]
- Klein EY, Monteforte B, Gupta A, et al. The frequency of influenza and bacterial coinfection: a systematic review and meta-analysis. Influenza Other Respir Viruses 2016;10:394-403. [Crossref] [PubMed]
- Mermond S, Berlioz-Arthaud A, Estivals M, et al. Aetiology of community-acquired pneumonia in hospitalized adult patients in New Caledonia. Trop Med Int Health 2010;15:1517-24. [Crossref] [PubMed]
- Yap FH, Gomersall CD, Fung KS, et al. Increase in methicillin-resistant Staphylococcus aureus acquisition rate and change in pathogen pattern associated with an outbreak of severe acute respiratory syndrome. Clin Infect Dis 2004;39:511-6. [Crossref] [PubMed]
- Jang TN, Yeh DY, Shen SH, et al. Severe acute respiratory syndrome in Taiwan: analysis of epidemiological characteristics in 29 cases. J Infect 2004;48:23-31. [Crossref] [PubMed]
- Rawson TM, Moore LSP, Zhu N, et al. Bacterial and Fungal Coinfection in Individuals With Coronavirus: A Rapid Review To Support COVID-19 Antimicrobial Prescribing. Clin Infect Dis 2020;71:2459-68. [Crossref] [PubMed]
- Arabi YM, Deeb AM, Al-Hameed F, et al. Macrolides in critically ill patients with Middle East Respiratory Syndrome. Int J Infect Dis 2019;81:184-90. [Crossref] [PubMed]
- Yang X, Yu Y, Xu J, et al. Clinical course and outcomes of critically ill patients with SARS-CoV-2 pneumonia in Wuhan, China: a single-centered, retrospective, observational study. Lancet Respir Med 2020;8:475-81. [Crossref] [PubMed]
- Zhu X, Ge Y, Wu T, et al. Co-infection with respiratory pathogens among COVID-2019 cases. Virus Res 2020;285:198005. [Crossref] [PubMed]
- Kim D, Quinn J, Pinsky B, et al. Rates of Co-infection Between SARS-CoV-2 and Other Respiratory Pathogens. JAMA 2020;323:2085-6. [Crossref] [PubMed]
- Richardson S, Hirsch JS, Narasimhan M, et al. Presenting Characteristics, Comorbidities, and Outcomes Among 5700 Patients Hospitalized With COVID-19 in the New York City Area. JAMA 2020;323:2052-9. [Crossref] [PubMed]
- Lansbury L, Lim B, Baskaran V, et al. Co-infections in people with COVID-19: a systematic review and meta-analysis. J Infect 2020;81:266-75. [Crossref] [PubMed]
- Garcia-Vidal C, Sanjuan G, Moreno-García E, et al. Incidence of co-infections and superinfections in hospitalized patients with COVID-19: a retrospective cohort study. Clin Microbiol Infect 2021;27:83-8. [Crossref] [PubMed]
- World Health Organization. Therapeutics and COVID-19. 2021. Available online: https://apps.who.int/iris/bitstream/handle/10665/342368/WHO-2019-nCoV-therapeutics-2021.2-eng.pdf (Accessed on 6 July 2021).
- Quast C, Pruesse E, Yilmaz P, et al. The SILVA ribosomal RNA gene database project: improved data processing and web-based tools. Nucleic Acids Res 2013;41:D590-6. [Crossref] [PubMed]
- Buchfink B, Xie C, Huson DH. Fast and sensitive protein alignment using DIAMOND. Nat Methods 2015;12:59-60. [Crossref] [PubMed]
- Truong DT, Franzosa EA, Tickle TL, et al. MetaPhlAn2 for enhanced metagenomic taxonomic profiling. Nat Methods 2015;12:902-3. [Crossref] [PubMed]
- Pertea M, Kim D, Pertea GM, et al. Transcript-level expression analysis of RNA-seq experiments with HISAT, StringTie and Ballgown. Nat Protoc 2016;11:1650-67. [Crossref] [PubMed]
- Murray JF, Matthay MA, Luce JM, et al. An expanded definition of the adult respiratory distress syndrome. Am Rev Respir Dis 1988;138:720-3. [Crossref] [PubMed]
- Schurink CAM, Nieuwenhoven CAV, Jacobs JA, et al. Clinical pulmonary infection score for ventilator-associated pneumonia: accuracy and inter-observer variability. Intensive Care Med 2004;30:217-24. [Crossref] [PubMed]
- Li CX, Li W, Zhou J, et al. High resolution metagenomic characterization of complex infectomes in paediatric acute respiratory infection. Sci Rep 2020;10:3963. [Crossref] [PubMed]
- Shi M, White VL, Schlub T, et al. No detectable effect of Wolbachia wMel on the prevalence and abundance of the RNA virome of Drosophila melanogaster. Proc Biol Sci 2018;285:20181165. [Crossref] [PubMed]
- Martins-Filho PR, Tavares CSS, Santos VS. Factors associated with mortality in patients with COVID-19. A quantitative evidence synthesis of clinical and laboratory data. Eur J Intern Med 2020;76:97-9. [Crossref] [PubMed]
- Wong D, Nielsen TB, Bonomo RA, et al. Clinical and Pathophysiological Overview of Acinetobacter Infections: a Century of Challenges. Clin Microbiol Rev 2017;30:409-47. [Crossref] [PubMed]
- Chattin-Kacouris BR, Ishihara K, Miura T, et al. Heat shock protein of Mycoplasma salivarium and Mycoplasma orale strains isolated from HIV-seropositive patients. Bull Tokyo Dent Coll 2002;43:231-6. [Crossref] [PubMed]
- Har-Noy M, Or R. Allo-priming as a universal anti-viral vaccine: protecting elderly from current COVID-19 and any future unknown viral outbreak. J Transl Med 2020;18:196. [Crossref] [PubMed]
- Busani S, Bedini A, Biagioni E, et al. Two Fatal Cases of Acute Liver Failure Due to HSV-1 Infection in COVID-19 Patients Following Immunomodulatory Therapies. Clin Infect Dis 2021;73:e252-5. [Crossref] [PubMed]
- Xu R, Zhou Y, Cai L, et al. Co-reactivation of the human herpesvirus alpha subfamily (herpes simplex virus-1 and varicella zoster virus) in a critically ill patient with COVID-19. Br J Dermatol 2020;183:1145-7. Erratum in: Br J Dermatol 2021;185:685. [Crossref] [PubMed]
- Jha A, Dunning J, Tunstall T, et al. Patterns of systemic and local inflammation in patients with asthma hospitalised with influenza. Eur Respir J 2019;54:1900949. [Crossref] [PubMed]
- Gutierrez-Reyes G, Lopez-Ortal P, Sixtos S, et al. Effect of pentoxifylline on levels of pro-inflammatory cytokines during chronic hepatitis C. Scand J Immunol 2006;63:461-7. [Crossref] [PubMed]