Association between cine CMR-based radiomics signature and microvascular obstruction in patients with ST-segment elevation myocardial infarction
Introduction
Emergency percutaneous coronary intervention (PCI) can quickly restore epicardial coronary blood flow in patients with ST-segment elevation myocardial infarction (STEMI), but research shows that microvascular perfusion cannot be restored to normal in nearly half of patients due to microvascular dysfunction or reperfusion injury (1). This non-reflux phenomenon of PCI is known as microvascular obstruction (MVO) and is associated with increased morbidity and mortality (2-4). Early diagnosis of MVO and its severity is conducive to clinical prognosis assessment and the formulation and implementation of treatment regimens (5). At present, there is still a lack of accurate biomarkers for non-invasive in vivo diagnosis and evaluation of MVO severity. Recently, cardiac magnetic resonance (CMR) has become as the gold standard technique for detecting extent of scar with acute myocardial infarction (AMI), MVO, and myocardial hemorrhage (6). Late gadolinium enhancement (LGE) of CMR can provide a good non-invasive evaluation of MVO (7), but this protocol is time consuming and the administration of gadolinium can arouse some adverse events, such as nephrogenic systemic fibrosis (NSF) (8,9). NSF is thought to occur exclusively in patients with kidney disease. Patients with coronary artery disease are often suffered diabetes, high blood pressure and arteriosclerosis simultaneously. These diseases can injury kidneys also. In the process of PCI, patients may receive large doses of the iodine contrast agent. Therefore, all these factors may expose patients to high risk of NSF if gadolinium agents were administrated. In order to overcome such drawbacks, researchers tried to seek contrast free CMR for characterizing myocardium (10,11). The aim of this study is to investigate the association between cine CMR-based radiomics and MVO, so as to develop a contrast free CMR technique to detect MVO in patients with STEMI. We present the following article in accordance with the STARD reporting checklist (available at https://jtd.amegroups.com/article/view/10.21037/jtd-21-1706/rc).
Methods
Patients
The study was conducted in accordance with the Declaration of Helsinki (as revised in 2013). The study was approved by Ethics Board of Jinan University (No. LL-KY-2021909) and individual consent for this analysis was waived due to its retrospective nature. One hundred and fifty-nine consecutive patients with STEMI who underwent CMR within 6 days after PCI were found in the electronica data base from October 2017 to May 2021. Inclusion criteria were: (I) PCI was performed within 24 hours of onset of myocardial infarction symptoms or clinical diagnosis of acute myocardial infarction, and occluded vessels were successfully opened; (II) CMR was performed within 6 days after PCI; and (III) the CMR examination was completed successfully and the images could be read from Picture Archiving and Communication Systems (PACS). Exclusion criteria were: (I) history of PCI before this time (n=16); (II) inadequate quality for image segmenting or incomplete clinical and imaging data (n=22); and (III) failure of extracting radiomics features (n=5). Patients’ general information and hematological indicators were collected, including gender, age, smoking history, diabetes, hypertension, culprit vessels and mean platelet volume (MPV), cardiac troponin (cTn), creatine phosphokinases (CPK), high sensitivity C-reactive protein (hs-CRP), N-terminal pro-brain natriuretic peptide (NT-ProBNP), urea, creatinine, low-density lipoprotein cholesterol (LDL-C), triglycerides (TG) and so on.
Cine CMR and LGE imaging acquisition
A 3.0T MR system (Magnetom Skyra; Siemens Healthcare) and 18-channel cardiac phased-control coil are used to acquire CMR images, equipped with respiratory gating and ECG vector gating. Steady-state precession fast sequence was used to capture standard left ventricular (LV) short axis, which completely encompassed the whole LV by contiguous section, for cine CMR before intravenous injection of contrast agent. The specific scan parameters are shown in Table 1.
Table 1
Sequences parameters | Cine | T1WI | T2WI | LGE |
---|---|---|---|---|
Acquisition plane | Short axis | Short axis | Short axis | Short axis |
TR (msec) | 3.8 | 680 | 2252 | 909.8 |
TE (msec) | 1.4 | 27 | 72 | 300 |
Slice thickness (mm) | 8 | 5 | 5 | 8 |
Slice spacing (mm) | 1.6 | 1.8 | 2 | 1.6 |
FOV (mm) | 91×38 | 92×42 | 92×42 | 88×39 |
Acquisition matrix | 138×208 | 220×320 | 230×230 | 220×300 |
Flip angle | 46 | 180 | 180 | 30 |
Contrast agent acquisition time (min) | – | – | – | 10–15 |
Dose of LGE (mmol/kg) | – | – | – | 0.1 |
MRI, magnetic resonance imaging; FOV, field of view; LGE, late gadolinium enhancement; TR, repetition time; TE, echo time; T1WI, T1-weighted imaging; T2WI, T2-weighted imaging.
Image preprocessing and radiomics features extraction
CMR images were analyzed by two expert diagnostic radiologists (12 and 10 years of experience) in concent. LGE, which is defined as 5 standard deviations above the mean signal intensity of normal myocardium, is used as the gold standard for evaluating myocardial infarction. The concentration of the contrast agent during delayed enhancement is higher than that of the normal myocardium because the intercellular space is widened of the infarcted myocardium. Therefore, the infarcted myocardium manifests as hyperintensity at the LGE images. In this study, each patient’s myocardial infarction was divided into with and without MVO (MVO was defined as hypointense areas within the hyperintense myocardium at the LGE images, while the hyperintense myocardium was described as areas of myocardial infarction). ITK-SNAP (http://www.itksnap.org/pmwiki/pmwiki.php) software was used to segment the myocardium. The slice on the end-diastolic phase images which corresponds to the maximum hyperintensity area at LGE was selected and region of interesting (ROI) was manually delineated around the boundary of the whole circle of the left myocardium by a radiologist with more 3-year-experience at interpreting CMR images. The open-source software Pyradiomics package (https://pyradiomics.readthedocs.io) was used to extract radiomic features. One hundred and five radiomics features were extracted, which included 12 shape-based features, 18 first order statistics features, 24 gray level co-occurrence matrix (GLCM) features, 16 gray level run length matrix (GLRLM) features, 16 gray level size zone matrix (GLSZM) features, 14 gray level dependence matrix (GLDM) features and 5 neighbouring gray tone difference matrix (NGTDM) features.
Statistical analysis
R software version 4.0.0 (http://www.r-project.org) and SPSS were used to analyze statistics. The difference is statistically significant when the P value is less than 0.05. Continuous variables were presented as mean ± standard deviation. The Mann-Whitney U test was used to compare differences in continuous variables between segments with MVO and without MVO if the distribution was not normal. The student t-test was used for normal distribution. The categorical variables were presented as frequency with percentage. Chi square test or Fisher’s exact test was used for categorical variables. The procession of radiomics feature selection and dimension reduction were following: first, Pearson correlation coefficient was used to remove those features with high correlation [if two features were high correlated (r>0.8), the one with lower area under the curve (AUC) of receiver operating characteristic (ROC) was removed]; second, Mann-Whitney U rank test was used for univariate analysis; third, the statistically significant features of univariate analysis are standardized by Z-score to eliminate the difference in scale between different features; at last, the least absolute shrinkage and selection operator (LASSO) regression with 5-fold cross-validation was employed to select the strongest features to construct a radiomics score through a linear combination. Binary logistic regression was used to assess the association of clinical characteristics and radiomics score with MVO. The flow diagram of radiomics features extraction process and radiomics score construction is displayed in Figure 1. AUC of ROC was calculated to evaluate the predictive performance of radiomics score for MVO.
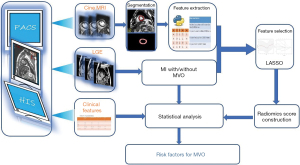
Results
Finally, 116 patients were included in this study (Figure 2). Among the 116 patients, MI without MVO was identified in 66 patients and MI with MVO was identified in 50 patients. The clinical characteristics at admission are listed in Table 2. Cardiac troponin (cTn), high sensitivity C-reactive protein (hs-CRP) and creatine phosphokinases (CPK) were associated with MVO statistically significant at univariable analysis. Univariable analysis revealed that 11 radiomics features were statistically associated with MVO (Table 3). LASSO regression selected five features to build radiomics score (Table 4). The radiomics score of MI with MVO was higher than that of MI without MVO (0.1500±0.77 vs. −0.72±0.90, P<0.001). Two typical cases were displayed in Figure 3 and Figure 4. Multivariable analysis demonstrated that hs-CRP, CPK and radiomics score were independent risk factors for MVO (Table 5). Radiomics score had the highest odds ratio (OR) 4.41 (95% CI: 2.26–9.93) with a ROC-AUC of 0.75 (95% CI: 0.68–0.85) to predict MVO. At an optimal cutoff value of −0.03 derived from ROC using Youden index, the sensitivity and specificity were 0.63 and 0.76 for identifying MVO, respectively.
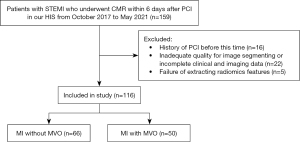
Table 2
Demographic characteristics | All patients (n=116) | Without MVO (n=66) | MVO (n=50) | P value |
---|---|---|---|---|
Sex | 0.755 | |||
Male | 105 [91] | 59 [89] | 46 [92] | |
Female | 11 [9] | 7 [11] | 4 [8] | |
Age, yrs | 51.86±11.26 | 52.61±11.93 | 50.88±10.35 | 0.407 |
Time to PCI (h) | 3 (2, 6.5) | 3 (1.62, 6.5) | 3 (2, 6) | 0.434 |
Systolic | 139.99±23.76 | 141.71±23.9 | 137.72±23.61 | 0.372 |
Diastolic | 88.72±16.77 | 89.03±15.72 | 88.3±18.23 | 0.821 |
Hypertension | 0.72 | |||
No | 50 [43] | 27 [41] | 23 [46] | |
Yes | 66 [57] | 39 [59] | 27 [54] | |
Hypertension stage | 0.266 | |||
0 | 51 [44] | 28 [42] | 23 [46] | |
1 | 13 [11] | 9 [14] | 4 [8] | |
2 | 18 [16] | 13 [20] | 5 [10] | |
3 | 34 [29] | 16 [24] | 18 [36] | |
Hypotensive drugs | 1 | |||
No | 74 [64] | 42 [64] | 32 [64] | |
Yes | 42 [36] | 24 [36] | 18 [36] | |
Culprit vessels | 0.473 | |||
LAD | 65 [56] | 39 [59] | 26 [52] | |
RCA | 26 [22] | 15 [23] | 11 [22] | |
LCX | 10 [9] | 4 [6] | 6 [12] | |
LAD + RCA | 3 [3] | 3 [5] | 0 [0] | |
LAD + LCX | 9 [8] | 4 [6] | 5 [10] | |
RCA + LCX | 3 [3] | 1 [2] | 2 [4] | |
Diabetes | 0.808 | |||
No | 86 [74] | 50 [76] | 36 [72] | |
Yes | 30 [26] | 16 [24] | 14 [28] | |
Current or previous smoking | 0.968 | |||
No | 45 [39] | 25 [38] | 20 [40] | |
Yes | 71 [61] | 41 [62] | 30 [60] | |
Drink | 1 | |||
No | 106 [91] | 60 [91] | 46 [92] | |
Yes | 10 [9] | 6 [9] | 4 [8] | |
Peak Troponin I | 9.41(1.07, 24.28) | 5.4 (0.65, 15.62) | 14.56 (5.8, 36.67) | 0.003 |
MYO | 61.43 (30.29, 156.6) | 53.61 (27.35, 125.05) | 67 (35.1, 235.75) | 0.143 |
CK-MB | 71.6 (16.08, 165.5) | 57.2 (6.89, 128) | 73.91 (27.79, 186.75) | 0.176 |
NT-proBNP | 683 (223.62, 1,565) | 601 (281.72, 1,525.25) | 898 (195.5, 1,631.75) | 0.562 |
MPV | 10.41±0.91 | 10.31±0.85 | 10.56±0.97 | 0.153 |
LDL | 2.89±1.09 | 3.02±1.17 | 2.71±0.97 | 0.115 |
TG | 1.52 (1.1, 2.59) | 1.66 (1.13, 2.52) | 1.44 (1.09, 2.6) | 0.406 |
hs-CRP | 5.18 (2, 13.19) | 4.46 (2.04, 7.81) | 6.39 (1.97, 15.01) | 0.246 |
S-CRP | 6.5 (2.61, 15.27) | 4.86 (2.63, 8.74) | 10.39 (2.58, 39.17) | 0.018 |
BUN | 4.9 (3.79, 5.8) | 4.9 (3.82, 6.15) | 4.85 (3.78, 5.7) | 0.987 |
Cr | 80.45 (70.95, 90) | 83 (72, 90.75) | 77.5 (70.85, 85) | 0.267 |
CPK | 1,021 (419.5, 1,933.25) | 554.5 (230.25, 1,054.75) | 1,542.5 (1,046, 2,972.5) | <0.001 |
Continuous variables are expressed as mean ± SD or median (confidence interval), and discrete variables are expressed as n [%]. MVO, microvascular obstruction; PCI, percutaneous coronary intervention; LAD, left anterior descending coronary artery; RCA, right coronary artery; LCX, left circumflex coronary artery; MYO, myohemoglobin, CK-MB, Creatine Kinase Isoenzyme; NT-proBNP, N-terminal pro-brain natriuretic peptide; MPV, mean platelet volume; LDL, low density lipoprotein, TG, triacylglycerol; hs-CRP, high sensitivity C-reactive protein; S-CRP, sensitivity C-reactive protein; BUN, blood urea nitrogen; CRP, C-reactive protein; Cr, creatinine; CPK, creatine phosphokinases.
Table 3
Radiomic features | Without MVO (n=66) | MVO (n=50) | P value |
---|---|---|---|
Shape maximum 2D diameter slice | 65.549±17.050 (−0.257±0.772) | 78.723±25.662 (0.339±1.162) | 0.005 |
Shape-surface area | 0.972±0.902 (0.266±1.185) | 0.502±0.390 (−0.351±0.513) | 0.017 |
First order mean | 33.773±15.505 (0.154±0.981) | 28.123±15.760 (−0.203± 0.998) | 0.030 |
Glrlm long run low gray level emphasis | 0.112±0.060 (0.173±1.075) | 0.090±0.476 (−0.228±0.849) | 0.026 |
Glszm gray level non-uniformity | 12.363±4.309 (−0.295±1.058) | 15.150±3.125 (−0.389±0.767) | <0.001 |
Glszm large area high gray level emphasis | 2,178.293±2,205.948 (−0.135±0.892) | 2,953.880±2,745.796 (0.178±1.111) | 0.049 |
Gldm dependence non-uniformity | 57.668±23.940 (−0.271±0.912) | 73.480±25.356 (0.358±1.001) | 0.002 |
Gldm gray level non-uniformity | 91.524±47.116 −0.176±1.036) | 110.054±41.393 (0.232±0.910) | 0.026 |
Gldm large dependence high gray level emphasis | 280.116±100.830 (−0.235±0.646) | 365.426±198.877 (0.311±1.273) | 0.001 |
Gldm small dependence low gray level emphasis | 0.024±0.012 (0.172±1.085) | 0.196±0.009 (−0.228±0.832) | 0.034 |
Ngtdm strength | 3.120±2.195 (0.131±1.018) | 2.464±2.069 (−0.173±0.959) | 0.028 |
In parentheses are the normalized values of the features. MVO, microvascular obstruction.
Table 4
Radiomic features | λ coefficient | Clinical meaning |
---|---|---|
Shape maximum 2D diameter slice | 0.02797425 | The largest pairwise Euclidean distance between tumor surface mesh vertices |
First order mean | −0.10906300 | The average gray level intensity within the ROI |
Gray level non-uniformity | 0.56528432 | GLN measures the variability of gray-level intensity values in the image, with a lower value indicating more homogeneity in intensity values |
Gldm large dependence high gray level emphasis | 0.56073542 | Measures the joint distribution of large dependence with higher gray-level values |
Ngtdm strength | −0.30037888 | Strength is a measure of the primitives in an image. Its value is high when the primitives are easily defined and visible, i.e., an image with slow change in intensity but more large coarse differences in gray level intensities |
ROI, region of interesting; GLN, gray level non-uniformity.
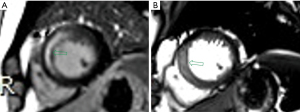
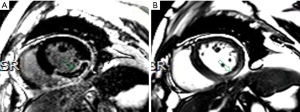
Table 5
Risk factors for MVO | Odds ratio | 95% CI | P value |
---|---|---|---|
cTn | – | – | 0.845 |
hs-CRP | 1.018 | 1.006–1.034 | 0.009 |
CPK | 1.0007 | 1.0004–1.0012 | 0.001 |
RS | 4.41 | 2.26–9.93 | <0.001 |
MVO, microvascular obstruction; CI, confidence interval; cTn, cardiac troponin; hs-CRP, high sensitivity C-reactive protein; CPK, creatine phosphokinases; RS, radiomics score.
Discussion
In this study, we explore the association between cine MR-based radiomics signature and MVO in patients with STEMI, and the result showed that radiomics signatures based on cine CMR was independent risk factor of MVO, which could achieve moderate predictive performance.
Although MVO can be visualized by LGE or T2* mapping, the former needs intravenous administration of contrast materials and the latter is still not commercially available broadly in clinical broadly in clinical centers (12). The delay scanning is not only time-consuming, but the use of contrast materials often brings some adverse events to patients with acute myocardial infarction who have chronic kidney disease. Therefore, contrast free CMR protocol is not time saving, but softer for these patients also. As generally speaking, T2 relaxation might be prolonged because of the increase in intercellular water in patients with myocardial infarction. Meanwhile, if the myocardium suffers from MVO at the same time, hemorrhage might occur (13). In hemorrhage, local accumulation of paramagnetic hemoglobin breakdown products led to shortening of T2-relaxation times and susceptible effect. Cine CMR imaging is one of the most common sequences to assess heart function (6). It bases on steady state free precession, which is a gradient recall echo sequence addressing T2 relaxation and being sensitive to magnetic susceptibility. Therefore, it can reflect T2 relaxation change and have the ability to detect changes due to hemorrhage. Recently, using a single-shot T2-prepared balanced SSFP sequence to obtain 3D T2 mapping of myocardium, Bustin and colleagues reported that focal areas of increased T2 values were well consistent with LGE findings in patients with myocarditis (14). To best of our knowledge, this is the first study to harness routine cine CMR-based radiomics signature to predict MVO in patients with STEMI after PCI. The radiomics score derived from five LASSO regression model selected features showed that the myocardium slice harboring MI with MVO had higher value. The feature with highest coefficient among the five selected features is the gray level non-uniformity, followed by the large dependence high gray level emphasis. Both belong to GLDM features. A higher value of gray level non-uniformity indicates high heterogeneity in intensity values. Large dependence high gray level emphasis measures the joint distribution of large dependence with higher gray level values, with higher value indicating more concentration of high intensity. Therefore, the higher radiomics score of the myocardium slice harboring MI with MVO means being more heterogeneous and more concentration of high intensity. Our primary study showed that myocardium slice harboring MI with MVO had some characteristics that could be distinguished from those without MVO on the contrast free cine CMR images. Unfortunately, these characteristics could not be perceived by naked eyes. Using radiomics, these characteristics could be extracted to identify presence of MVO at contrast free CMR images. Although the predictive performance was still moderate, this technique showed the potential that cine CMR-bases radiomics could predict MVO without administration of contrast agency.
Limitations of this study should be acknowledged. First, this study had a relatively small sample size with retrospective data. Therefore, no cohort was split to validate the performance of model. Second, although radiomics score archived the highest OR, it did not mean that radiomics score was superior to the other two clinical characteristics as OR is scaled by an arbitrary factor (15). Third, MRI is a multiparametric imaging modality, this study did not include multiply MR sequences and quantitative parameters, especially T1 mapping, T2 mapping and extracellular volume fraction, which had shown to be promising modalities for cardiomyopathy assessment. Last, this is a single-center and small-sample study, which may affect the accuracy of the results, therefore, follow-up studies will collect samples from other institutions for external verification in order to illustrate the feasibility of predicting MVO based on the radiomic signature of cine CMR.
In conclusion, radiomics signature based on cine CMR was an independent risk factor to predict MVO in patients with STEMI and its OR was higher than other clinical characteristics, and it is expected to be a biological marker to replace LGE in the diagnosis of MVO. As CMR is a multiparametric imaging modality, if radiomics signatures derived from multiparameter, especially those quantitative parameters, predictive performance could be improved. Therefore, further researches based on multiparametric CMR are warranted to construct high predictive performance model to stratify patients with STEMI and provide decision support for patient management making.
Acknowledgments
Funding: This work was supported by the Shenzhen Science and Technology Project (No. GJHZ20180928172002087).
Footnote
Reporting Checklist: The authors have completed the STARD reporting checklist. Available at https://jtd.amegroups.com/article/view/10.21037/jtd-21-1706/rc
Data Sharing Statement: Available at https://jtd.amegroups.com/article/view/10.21037/jtd-21-1706/dss
Conflicts of Interest: All authors have completed the ICMJE uniform disclosure form (available at https://jtd.amegroups.com/article/view/10.21037/jtd-21-1706/coif). YXJW serves as an unpaid editorial board member of Journal of Thoracic Disease. The other authors have no conflicts of interest to declare.
Ethical Statement: The authors are accountable for all aspects of the work in ensuring that questions related to the accuracy or integrity of any part of the work are appropriately investigated and resolved. The study was conducted in accordance with the Declaration of Helsinki (as revised in 2013). The study was approved by Ethics Board of Jinan University (No. LL-KY-2021909) and individual consent for this analysis was waived due to its retrospective nature.
Open Access Statement: This is an Open Access article distributed in accordance with the Creative Commons Attribution-NonCommercial-NoDerivs 4.0 International License (CC BY-NC-ND 4.0), which permits the non-commercial replication and distribution of the article with the strict proviso that no changes or edits are made and the original work is properly cited (including links to both the formal publication through the relevant DOI and the license). See: https://creativecommons.org/licenses/by-nc-nd/4.0/.
References
- Ito H, Maruyama A, Iwakura K, et al. Clinical implications of the 'no reflow' phenomenon. A predictor of complications and left ventricular remodeling in reperfused anterior wall myocardial infarction. Circulation 1996;93:223-8. [Crossref] [PubMed]
- Jeremy RW, Links JM, Becker LC. Progressive failure of coronary flow during reperfusion of myocardial infarction: documentation of the no reflow phenomenon with positron emission tomography. J Am Coll Cardiol 1990;16:695-704. [Crossref] [PubMed]
- Morishima I, Sone T, Okumura K, et al. Angiographic no-reflow phenomenon as a predictor of adverse long-term outcome in patients treated with percutaneous transluminal coronary angioplasty for first acute myocardial infarction. J Am Coll Cardiol 2000;36:1202-9. [Crossref] [PubMed]
- Reffelmann T, Kloner RA. The "no-reflow" phenomenon: basic science and clinical correlates. Heart 2002;87:162-8. [Crossref] [PubMed]
- Albert TS, Kim RJ, Judd RM. Assessment of no-reflow regions using cardiac MRI. Basic Res Cardiol 2006;101:383-90. [Crossref] [PubMed]
- Pontone G, Andreini D, Guaricci AI, et al. Association between haptoglobin phenotype and microvascular obstruction in patients with STEMI: a cardiac magnetic resonance study. JACC Cardiovasc Imaging 2019;12:1007-17. [Crossref] [PubMed]
- Robbers LF, Eerenberg ES, Teunissen PF, et al. Magnetic resonance imaging-defined areas of microvascular obstruction after acute myocardial infarction represent microvascular destruction and haemorrhage. Eur Heart J 2013;34:2346-53. [Crossref] [PubMed]
- Mathur M, Jones JR, Weinreb JC. Gadolinium Deposition and Nephrogenic Systemic Fibrosis: A Radiologist's Primer. Radiographics 2020;40:153-62. [Crossref] [PubMed]
- Mendoza FA, Artlett CM, Sandorfi N, et al. Description of 12 cases of nephrogenic fibrosing dermopathy and review of the literature. Semin Arthritis Rheum 2006;35:238-49. [Crossref] [PubMed]
- Tahir E, Sinn M, Bohnen S, et al. Acute versus Chronic Myocardial Infarction: Diagnostic Accuracy of Quantitative Native T1 and T2 Mapping versus Assessment of Edema on Standard T2-weighted Cardiovascular MR Images for Differentiation. Radiology 2017;285:83-91. [Crossref] [PubMed]
- Gottbrecht M, Kramer CM, Salerno M. Native T1 and Extracellular Volume Measurements by Cardiac MRI in Healthy Adults: A Meta-Analysis. Radiology 2019;290:317-26. [Crossref] [PubMed]
- Triadyaksa P, Oudkerk M, Sijens PE. Cardiac T2 * mapping: Techniques and clinical applications. J Magn Reson Imaging 2020;52:1340-51. [Crossref] [PubMed]
- Carrick D, Haig C, Ahmed N, et al. Comparative Prognostic Utility of Indexes of Microvascular Function Alone or in Combination in Patients With an Acute ST-Segment-Elevation Myocardial Infarction. Circulation 2016;134:1833-47. [Crossref] [PubMed]
- Bustin A, Hua A, Milotta G, et al. High-Spatial-Resolution 3D Whole-Heart MRI T2 Mapping for Assessment of Myocarditis. Radiology 2021;298:578-86. [Crossref] [PubMed]
- Norton EC, Dowd BE, Maciejewski ML. Odds Ratios-Current Best Practice and Use. JAMA 2018;320:84-5. [Crossref] [PubMed]