A novel seven-gene risk profile in BALF to identify high-risk patients with idiopathic pulmonary fibrosis
Introduction
Idiopathic pulmonary fibrosis (IPF) is a progressive and fatal chronic fibrosing interstitial lung disease of unknown cause with an estimated median survival time of 3 years (1-3). Importantly, IPF is a highly heterogeneous disease showing a wide range of clinical behavior, from relatively slow progression and long-term survival to accelerated progressive disease course and shorter survival (4,5). Besides pirfenidone and nintedanib being approved to decrease the decline of lung function and disease progression (6), lung transplantation (LTx) is the only effective curative therapy for IPF patients (7). However, many patients expire before receiving LTx (8,9). Thus, predictive biomarkers to identify high-risk patients with inferior survival and determine the optimal timing of referral for transplantation are urgently needed.
The difficulty of predicting the prognosis of patients with IPF has prompted research into biomarkers. Recent studies have identified several prognostic systems for IPF based on clinical parameters (3,10,11) and peripheral blood biomarkers, including proteins and genes (12-16). Even so, very little literature is available on whether molecular events in the alveolar microenvironment could suggest novel potential prognostic biomarkers of IPF and provide some clues for molecular features of the disease with distinct clinical course phenotypes. Bronchoalveolar lavage fluid (BALF) is reflective of the local alveolar milieu and studies have proved altered molecular environment of alveoli in patients with IPF (17-20). The advent of ‘omics’ technologies (including transcriptomics) that produce a large amount of data and hold considerable promise for personalized care has accelerated the pace of biomarker discovery. Recently, four studies attempted to identify molecular biomarkers and constructed prognostic signatures by using the BALF transcriptome data of patients with IPF (21-24). However, the four prediction models were all hampered by limited predictive ability or only focusing on the analysis of specific genes. Therefore, improving outcome prediction over what is currently available may have significant implications on individualized prognosis prediction and optimal timing of referral for LTx.
In the present study, we applied a novel method to screen potential prognostic genes in BALF samples from IPF patients. It is found that there exists different gene expression profiles determining clinical outcomes in terms of survival in IPF patients with similar baseline lung function (5,25), and several genetic variants are associated with disease progression and survival (26). Therefore, to enhance the genetic difference and place greater emphasis on the detection of the specific genetic difference that results in poor clinical outcome, we categorized the patients with IPF into short-term survivors (STSs) who survived less than two years and long-term survivors (LTSs) who survived more than two years according to the survival time window for referral for LTx (27). We integrated the differences of mRNA expression between IPF patients and healthy donors (HDs) and between the IPF patients with different survival, which may not only predict prognosis but may also contribute to discovering molecular mechanisms involved in disease progression. The integrated differentially expressed genes (DEGs) were first screened in the derivation cohort, and these genes were narrowed down using univariate Cox regression analysis, least absolute shrinkage and selection operator (LASSO) regression, and multivariate Cox regression analysis until seven genes were identified to construct an innovative gene model for predicting the prognosis of IPF. As a further step, we comprehensively assessed the proposed model’s discrimination, calibration, and clinical practicability in the derivation and validation cohorts. In addition, we performed functional enrichment and immune status analyses for the risk model. Our results offer new insight into the role of BALF in the evaluation of patients with IPF and may offer clues to the development of progressive IPF. We present the following article following the TRIPOD reporting checklist (available at https://jtd.amegroups.com/article/view/10.21037/jtd-21-1830/rc).
Methods
Data acquisition and processing
The general idea and flow chart of the study is shown in Figure 1. We obtained the gene expression profiles of BALF and relevant clinical data of the study population from the Gene Expression Omnibus (GEO) (https://www.ncbi.nlm.nih.gov/geo/, GSE70866). The GSE70866 dataset (21) was based on two platforms, GPL14550 (Agilent-028004 SurePrint G3 Human GE 8x60K Microarray) and GPL17077 (Agilent-039494 SurePrint G3 Human GE v2 8x60K Microarray). The GPL14550 platform consists of 20 HDs from Freiburg and 112 patients with IPF (62 patients from Freiburg, and 50 patients from Siena), while the GPL17077 platform contains 64 patients from Leuven. BALF cells were harvested from 176 patients with IPF and 20 HDs at the time of diagnosis. Total RNA was extracted, labeled and hybridized to Agilent gene expression arrays. Our study assigned the individuals from the GPL14550 platform to the derivation cohort and the individuals from the GPL17077 platform to the validation cohort. IPF patients from the two cohorts were dichotomously categorized into STSs and LTSs, respectively. Clinical variables in this study included age, gender, GAP (gender, age, and two lung physiology variables), survival status, and survival time. The study was conducted in accordance with the Declaration of Helsinki (as revised in 2013). Since the data from the GEO database is publicly available, the present study was exempted from the approval of the local ethics committee and informed consent.
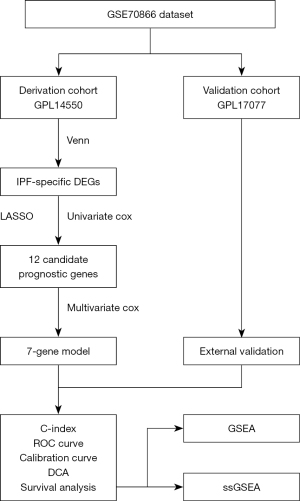
Screening of DEGs
Using the limma R package, we first screened the DEGs between IPF patients and HDs. DEGs between the two groups were estimated by fold-change (FC) filtering combined with the T-test. False discovery rate (FDR) was calculated to correct the P-value. Genes with an absolute value of FC ≥2.0 and an FDR <0.05 were considered significantly differentially expressed. Differential analysis was also applied between STS and LTS groups, and those with the same criterion were identified as DEGs. Finally, the overlapping datasets of the two group DEGs were retrieved for further analysis.
Identification of crucial prognostic genes
We first used univariate Cox regression analysis to identify crucial prognostic genes to determine the correlation between the DEGs and survival status in the derivation cohort. Next, DEGs with a P-value <0.05 were selected for subsequent analysis. Through this method, we identified all the 42 DEGs as candidate prognostic genes. Then, we applied a LASSO method (28,29) to determine the best predictive genes ideal for prognosis prediction.
Development of the gene model to predict prognosis
We fit a multivariate Cox regression model predictive of overall survival (OS) based on genes derived from the LASSO regression. The stepwise gene selection process was based on the Akaike information criterion (AIC) (30), and the model with minimum AIC value was determined as the final fitted model. A forest plot was used to show the result of the multivariate Cox regression. A nomogram was developed to predict the probability of 1-, 2-, and 3-year OS.
Assessment and validation of the gene model
The proposed model's predictive performance and clinical practicability were assessed by the C-statistic, calibration curve, and decision curve analysis (DCA) in derivation and validation cohorts. We used 1,000 bootstrap samples to conduct these activities. The time-dependent predictive value of the model was evaluated by using the time-dependent receiver operating characteristic (ROC) curve. Patients with IPF were stratified into high- and low-risk groups in the light of the median risk score (calculated by the total nomogram points). The Kaplan-Meier (KM) analysis estimated the probabilities of OS, with differences between groups assessed using the log-rank test.
Functional enrichment and immune activity analyses between different risk groups
We performed enrichment analysis between the high- and low-risk groups by using gene set enrichment analysis (GSEA) to investigate the potential biological characteristic of the predictive model. Then, the single sample GSEA (ssGSEA) was used to calculate the infiltrating immune cells' scores and evaluate the activity of immune-related pathways in different risk groups.
Statistical analysis
Participant baseline characteristics were summarized as median, range, mean ± standard deviation (SD), number, and percentages, as appropriate. We used the T-test or Mann-Whitney U test and χ2 test or Fisher’s exact test to compare continuous and categorical variables. All statistical analyses were performed using R-3.5.1 and the corresponding packages. A two-sided P<0.05 was considered statistically significant.
Results
Participant baseline characteristics
A total of one hundred seventy-six patients with IPF were included in the study, consisting of 112 patients in the derivation cohort (including 20 HDs) and 64 patients in the validation cohort. No differences between IPF subjects in the derivation cohort and that in the validation cohort were observed regarding the age, gender, and GAP stage (Table 1). Of 112 IPF patients in the derivation cohort, 70 were considered STSs and 42 LTSs, while 41 were considered STSs and 23 LTSs in the validation cohort. Patients in STS and LTS groups were also matched concerning age, gender, and GAP stage (Table S1).
Table 1
Variables | Derivation cohort, n=112 | Validation cohort, n=64 | P |
---|---|---|---|
Age, years, median (IQR) | 69.5 (62.0, 76.0) | 68.5 (63.75, 75.0) | 0.920 |
Gender, No. (%) | 0.726 | ||
Female | 19 (17.0) | 13 (20.3) | |
Male | 93 (83.0) | 51 (79.7) | |
GAP, No. (%) | 0.075 | ||
I | 31 (27.7) | 25 (39.1) | |
II | 52 (46.4) | 31 (48.4) | |
III | 29 (25.9) | 8 (12.5) |
Mann-Whitney U-test and χ2 test were used for comparison between derivation and validation cohorts for continuous and categorical data, respectively. IQR, interquartile range (25% and 75% percentiles); No., number; GAP, Gender, Age, and Physiology.
Identification of IPF-specific candidate prognostic genes
Firstly, differential expression analysis of the mRNA expression profiles was performed between the IPF patients and HDs in the derivation cohort, and 381 DEGs were identified. Then, of 112 IPF patients in the derivation cohort, mRNA expression profiles between STS and LTS groups were compared, and 144 DEGs were identified. Lastly, the DEGs between STSs and LTSs were further overlapped with the DEGs between IPF and HDs, and 42 DEGs were finally identified for further analysis (Figure 2A). The correlation network of the 42 DEGs was presented in Figure 2B.
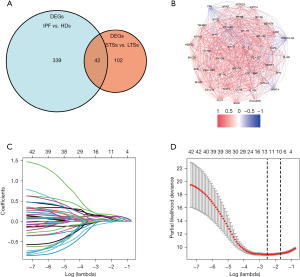
Univariate Cox regression was then applied for the 42 DEGs to identify genes significantly correlated with OS. Finally, all the 42 genes were entered in the LASSO regression for further shrinkage (all P<0.01; Figure S1). Upon the partial likelihood deviance reaching a minimum in the LASSO regression, 12 genes were identified as IPF-specific prognostic candidates (Figure 2C,2D).
Construction, assessment, and validation of the predictive model
After LASSO regression, 12 genes (CCL2, CCR3, CXCL14, DACH1, HS3ST1, MRVI1, NRAP, PDCD1LG2, SOD3, STAB1, TM4SF1, and TPST1) were selected as the potential predictors. In the multivariate analysis process, the optimal fitted prognostic model with the minimum AIC value consisted of 7 genes (CCR3, HS3ST1, MRVI1, NRAP, SOD3, STAB1, and TPST1). All the seven genes were associated with increased risk with hazard ratios (HRs) >1, and 3 genes (CCR3, NRAP, and SOD3) were independent predictors of shorter survival (Figure 3).
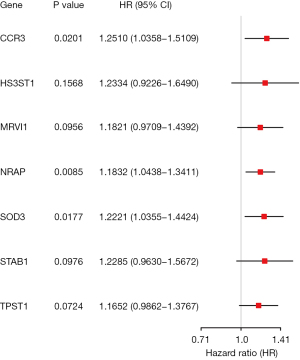
We then assessed the proposed model’s prediction capability. The C-index of the proposed model in the derivation cohort was 0.815 (95% CI: 0.769–0.861), significantly higher than the GAP staging system (0.617, 95% CI: 0.552–0.682; P<0.001). Similarly, the C-index of the model (0.812, 95% CI 0.703–0.921) was also higher than that of the GAP staging system (0.670, 95% CI: 0.575–0.765; P<0.01) in the validation cohort. We also plotted ROC curves to assess the prediction accuracy for 1-, 2-, and 3-year survival. The area under the curves (AUCs) of ROC curves for predicting 1-, 2- and 3-year survival in the derivation cohort were 0.857, 0.918, and 0.930, respectively (Figure 4A), and those in the validation cohort were 0.850, 0.880, and 0.925, respectively (Figure 4B). Besides the excellent discrimination at the three specific time points above, time-dependent ROC curves showed that our proposed model consistently outperformed the GAP staging system from 0.5- to 3-year mortality prediction in both the derivation and validation cohorts (Figure 4C,4D). Furthermore, calibration curves of our proposed model in the derivation and validation cohorts displayed good agreements between prediction and actual observation of 1-, 2-, and 3-year survival (Figure 5A,5B). Thus, the high discrimination and good calibration indicated that our proposed model demonstrated accurate prediction capability. In addition, the clinical usefulness of our proposed model was quantified by the DCA curve; it provided better clinical applicability in predicting 1-, 2-, and 3-year survival of the patients with IPF due to good net benefit with wide ranges of threshold probabilities compared with the GAP staging system (Figure 5C,5D). Eventually, a personalized scoring nomogram based on the proposed model was generated to predict the probability of 1-, 2-, and 3-year survival to quantify the risk assessment for an individual patient with IPF (Figure 6).
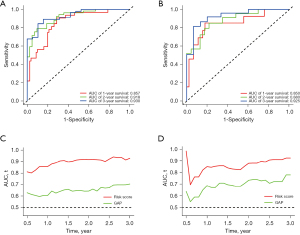
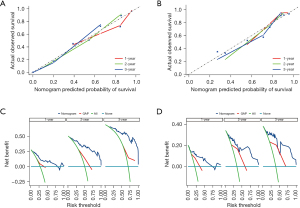
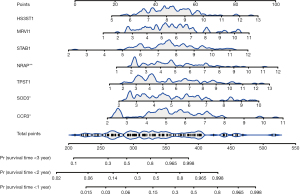
Nomogram model-based risk stratification
Patients in the derivation and validation cohorts were divided into two risk groups (high-risk vs. low-risk) based on the median score calculated by the total nomogram points, respectively (Figure 7A,7B). As the risk score increased, the patients’ risk of death increased, and the survival time decreased (Figure 7C,7D). Median OS for high-risk patients versus low-risk patients was 0.86 (95% CI: 0.67–1.29) years versus 3.21 [95% CI: 2.89–not reached (NR)] years (P<0.0001; Figure 7E) in the derivation cohort. Median OS was not reached for the low-risk patients versus 1.75 (95% CI: 0.96–NR) years for the high-risk patients in the validation cohort (P<0.0001; Figure 7F).
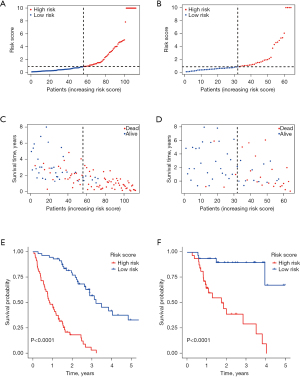
Heatmaps were used to visualize the difference of age, gender, GAP stage, survival status, and the seven gene expression profile between high-risk and low-risk patients in the derivation and validation cohorts. As illustrated in Figure 8A,8B, high-risk patients had higher GAP stage and more deaths than low-risk patients (P<0.01). There were no significant differences between high-risk and low-risk patients regarding age and gender (P>0.05). Figure 8A and split violin (Figure 8C) showed that all the seven genes were up-regulated in the high-risk group in the derivation cohort, and five of them were up-regulated in the validation cohort (Figure 8B,8D).
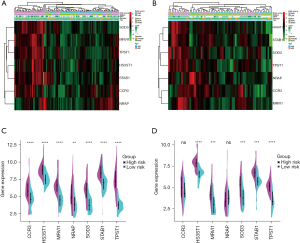
We performed univariate and multivariable Cox regression analyses to evaluate whether the risk score could be an independent prognostic predictor. On univariate analyses, the risk score was a prognostic factor for inferior survival in derivation and validation cohorts (Figure 9A,9B). Multivariate analyses showed that the risk score was an independent prognostic factor of poor survival after adjusting for other confounding factors (age, gender, GAP stage) in both cohorts (HR 5.243, 95% CI: 2.958–9.295; HR 7.130, 95% CI: 2.159–23.549; Figure 9C,9D).
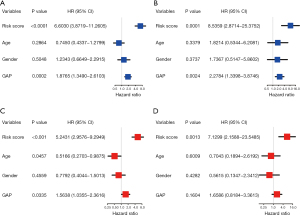
Functional enrichment and immune status analyses for the risk model
To further elucidate the biological features associated with the risk model, GSEA was performed to investigate the differences of hallmark gene sets between the high- and low-risk groups. GSEA analyses indicated that all the enriched pathways were activated in the high-risk group and were mainly associated with INFLAMMATORY_RESPONSE and TNFA_SIGNALING_VIA_NFKB in both the derivation and validation cohorts (Figure 10A,10B).
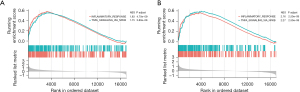
Considering that the risk profile was associated with inflammation, we further explored the relationship between risk scores and immune status using the ssGSEA (Figure 11A). As shown in Figure 11B, the risk score and corresponding genes were correlated with antigen processing and presentation contents, such as APC co-stimulation, CCR, DCs, and Macrophages. The scores of DCs, Macrophages, APC co-stimulation, CCR, and parainflammation in the high-risk group were significantly higher than those in the low-risk group in the derivation cohort (Figure 11C,11D). Similarly, the scores of Macrophages, APC co-stimulation, CCR, and parainflammation in the high-risk group were also significantly higher than those in the low-risk group in the validation cohort (Figure S2). These results implied that high-risk was associated with inflammatory process mediated by the immune response, which was consistent with the result of GSEA.
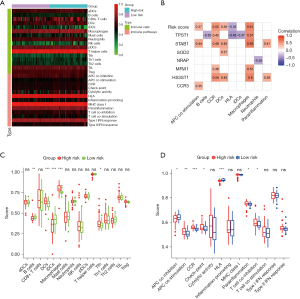
Discussion
IPF is a progressive and lethal interstitial lung disease with a highly variable clinical outcome. Due to the very heterogeneous clinical course, it remains a great challenge to accurately identify the high-risk patients for death and determine the optimal timing of referral for transplantation. In the present study, we used the transcriptome data of BALF to construct a novel predictive model with seven-gene signature for risk stratification and individual survival prediction in IPF patients. The model was derived in one cohort and validated in an independent cohort. Impressively, we examined the proposed model’s prognostic value through comprehensive methods, and further preliminarily explored the potential mechanism involved in the disease progression.
The notion that BALF reflecting the local alveolar milieu may be informative in IPF research has gained significant momentum in recent years (31). Based on the fact that the GSE70866 dataset is the only one linking the survival data to mRNA expression profiles in BALF of patients with IPF, there exists four prediction models with distinct gene signature (21-24). Prasse et al. firstly provided the comprehensive study of BAL gene expression patterns and generated a stable six-gene signature, performing better than the GAP index (C-index, 0.67 vs. 0.63) for predicting mortality in IPF (21). Xia et al. extracted two gene modules with WGCNA, and developed a four-gene signature model (C-index 0.72) (23). Besides these, two other studies have focused on the analysis of specific genes. Specifically, Li et al. recently found that both hypoxia and immune status were associated with the survival of patients with IPF, and established a nine-gene prognostic classifier with the AUCs of ROC curves for predicting 1-, 2- and 3-year survival of 0.789, 0.768, and 0.754, respectively (22). Li et al. investigated the relationship between ferroptosis and the prognosis of IPF, constructing a ferroptosis-related genes (FRGs) signature with the 1-, 2- and 3-year AUCs of 0.737, 0.772, and 0.731, respectively (24). While in our study, we used a novel method to screen prognostic genes, and derived a seven-gene risk profile presented by a personalized scoring nomogram. Our nomogram model revealed excellent predictive ability in both the derivation and validation cohorts. The C-index for the derivation and validation cohorts were 0.815 and 0.812, respectively, outperforming the GAP staging system, and were higher than those previously reported (21,23). The AUCs of our ROC analyses were also better than the ROC curves in previous studies (22,24). In addition, calibration curves demonstrated an optimal agreement between prediction and observation of 1-, 2- and 3-year survival probability. Furthermore, the results of the time-dependent ROC curves and DCAs showed the superiority of the nomogram model for clinical prediction and net benefits compared with the GAP staging system. All of the above attributes are important because accurate outcome prediction has efficient implications for IPF patients.
IPF is a heterogeneous disease in terms of survival (4,5). Our model could stratify patients into two different risk subgroups with significantly distinct prognosis following the median risk score. The risk score was proved to be an independent predictor of inferior survival, and as expected, high-risk patients had a worse prognosis than low-risk patients. The clinical implication of identifying high-risk patients are substantial. Since high-risk patients have a dismal prognosis with a median survival time of fewer than two years and the waiting time for LTx is approximately one year (9,32), these patients identified as high risk should receive an LTx evaluation urgently. Thus, incorporating our prognostic risk model in the assessment of patients with IPF may improve the accuracy of lung transplantation referral, allowing patients who need it to receive LTx in a more timely manner while delaying those who may not need it urgently.
Our proposed model comprised seven genes, CCR3, SOD3, HS3ST1, MRVI1, NRAP, STAB1, and TPST1. Almost all of the seven signature genes have been identified previously in multiple diseases including pulmonary fibrosis. Desai et al. measured the gene expression of molecular markers of inflammation and oxidative stress between IPF subjects and controls and found CCR3 to have increased mRNA levels in IPF patients (33). Huaux et al. proposed that CCR3 plays a novel role in granulocyte recruitment and bleomycin-induced lung fibrosis (34). In the current study, we found that CCR3 was upregulated in patients with IPF and as an independent risk factor of poor survival. SOD is one of the vital antioxidant enzymes preventing oxidant-mediated lung injury. Many lines of evidence support the protective role of SOD in pulmonary fibrosis (35-38). The overexpression of SOD3 in our study may indicate an attempt to compensate for increased oxidative stress in IPF. HS3ST1 is required for antithrombin’s anti-inflammatory activity and is associated with atherosclerosis (39). The up-regulation of MRVI1 gene is associated with decreased overall survival for stage III serous ovarian carcinoma patients with chemo-resistance, and it is a susceptibility gene for moyamoya syndrome in European patients with neurofibromatosis type 1 (40,41). The NRAP gene encodes the nebulin related anchoring protein, and upregulation of its expression was experimentally observed in dilated cardiomyopathy (DCM) mice models and human DCM patients (42,43). The protein encoded by STAB1 is a scavenger receptor in macrophages, involving in homeostatic balance and the resolution of inflammation (44,45). Our study elucidated that STAB1 has a strong positive correlation with macrophages. TPST-1 has been found aberrantly expressed in several cancers and is correlated with metastasis (46,47). In a word, all the seven genes were high-expressed in BALF of patients with IPF and were associated with inferior prognosis in our study. However, except for CCR3 and SOD3, the functions of the other five genes in IPF have not been elaborated, and further studies are needed.
Of mention, the finding that high-risk patients with IPF had shorter survival and a higher risk of mortality may indicate high-risk patients experiencing a progressive disease course. However, the mechanisms of disease progression are not fully elucidated. Our study could provide some clues for mechanistic research on IPF progression. On one hand, GSEA analyses depicted that enriched pathways in high-risk patients were mainly associated with inflammatory hallmarks; on the other hand, the results of ssGSEA indicated that the high-risk group is closely correlated with the immune process, particularly macrophages, DCs, APC co-stimulation, CCR, and parainflammation. These findings led to an increased understanding of the mechanisms associated with IPF progression that inflammation mediated by immune response might be involved in the disease progression. O'Dwyer’s study supported the idea that the altered lung microbiota generated molecular patterns engaging innate immune receptors and induced sustained inflammation, promoting disease progression (48). In addition, a recent review by Gibson et al. (49) concluded that compared with IPF, progressive fibrosing interstitial lung disease is characterized by the presence of identifiable antigen-driven immune response and more inflammatory infiltration, somewhat supporting our conclusion. However, this deduction of inflammation-mediated progressive fibrosis should be tested empirically in future work.
The study has several strengths. First, we determined the specificity of the seven-gene prognostic model to IPF by integrating the DEGs between IPF patients and HDs with those between IPF patients with short and extended survival. Compared with previously reported models constructed using the same dataset, the prediction performance of our model was better. Second, the present study had the advantage of comprehensively evaluating the robust performance of the model, and it had been validated through an independent cohort. We evaluated the model by reporting the discrimination, calibration, and clinical practicality simultaneously. Discrimination and calibration are cardinal characteristics in assessing model performance; however, they are underreported in the published medical literature (50). Reports on the clinical practicality of models are even fewer. We also acknowledge several limitations. First, though the derivation and validation cohorts come from two distinct platforms, further external validation using other datasets or real-world prospective clinical cohorts is necessary to verify the predictive value of the seven-gene model. Besides, the prediction of our model may be compromised since some clinical variables, such as high-resolution computed tomography (HRCT) fibrosis scores, pulmonary function tests, and treatment information, were unavailable. Furthermore, most of the enrolled genes in the model have not been elaborated except for the initial description of CCR3 and SOD3; future research is warranted to provide deep insight into the biological functions of these genes in IPF.
Conclusions
We derived and validated a novel prognostic gene model that performed well in risk stratification and individualized survival prediction for patients with IPF, facilitating personalized management of IPF patients, especially for high-risk patients in the choice of optimal timing of referral for transplantation. In addition, it deepened the understanding of the role inflammation played in IPF progression, which needs to be further studied.
Acknowledgments
We want to thank the GEO database and Prof. Antje Prasse for uploading the data to GEO. The first author also would like to thank his wife Shengjiao Wang and his parents for their dedication and love.
Funding: None.
Footnote
Reporting Checklist: The authors have completed the TRIPOD reporting checklist. Available at https://jtd.amegroups.com/article/view/10.21037/jtd-21-1830/rc
Peer Review File: Available at https://jtd.amegroups.com/article/view/10.21037/jtd-21-1830/prf
Conflicts of Interest: All authors have completed the ICMJE uniform disclosure form (available at https://jtd.amegroups.com/article/view/10.21037/jtd-21-1830/coif). The authors have no conflicts of interest to declare.
Ethical Statement: The authors are accountable for all aspects of the work in ensuring that questions related to the accuracy or integrity of any part of the work are appropriately investigated and resolved. The study was conducted in accordance with the Declaration of Helsinki (as revised in 2013). Since the data from the GEO database is publicly available, the present study was exempted from the approval of the local ethics committee and informed consent.
Open Access Statement: This is an Open Access article distributed in accordance with the Creative Commons Attribution-NonCommercial-NoDerivs 4.0 International License (CC BY-NC-ND 4.0), which permits the non-commercial replication and distribution of the article with the strict proviso that no changes or edits are made and the original work is properly cited (including links to both the formal publication through the relevant DOI and the license). See: https://creativecommons.org/licenses/by-nc-nd/4.0/.
References
- Strongman H, Kausar I, Maher TM. Incidence, Prevalence, and Survival of Patients with Idiopathic Pulmonary Fibrosis in the UK. Adv Ther 2018;35:724-36. [Crossref] [PubMed]
- Vancheri C, Failla M, Crimi N, et al. Idiopathic pulmonary fibrosis: a disease with similarities and links to cancer biology. Eur Respir J 2010;35:496-504. [Crossref] [PubMed]
- King TE Jr, Tooze JA, Schwarz MI, et al. Predicting survival in idiopathic pulmonary fibrosis: scoring system and survival model. Am J Respir Crit Care Med 2001;164:1171-81. [Crossref] [PubMed]
- Martinez FJ, Safrin S, Weycker D, et al. The clinical course of patients with idiopathic pulmonary fibrosis. Ann Intern Med 2005;142:963-7. [Crossref] [PubMed]
- Selman M, Carrillo G, Estrada A, et al. Accelerated variant of idiopathic pulmonary fibrosis: clinical behavior and gene expression pattern. PLoS One 2007;2:e482. [Crossref] [PubMed]
- Raghu G, Rochwerg B, Zhang Y, et al. An Official ATS/ERS/JRS/ALAT Clinical Practice Guideline: Treatment of Idiopathic Pulmonary Fibrosis. An Update of the 2011 Clinical Practice Guideline. Am J Respir Crit Care Med 2015;192:e3-19. [Crossref] [PubMed]
- Raghu G, Collard HR, Egan JJ, et al. An official ATS/ERS/JRS/ALAT statement: idiopathic pulmonary fibrosis: evidence-based guidelines for diagnosis and management. Am J Respir Crit Care Med 2011;183:788-824. [Crossref] [PubMed]
- Bennett D, Fossi A, Bargagli E, et al. Mortality on the Waiting List for Lung Transplantation in Patients with Idiopathic Pulmonary Fibrosis: A Single-Centre Experience. Lung 2015;193:677-81. [Crossref] [PubMed]
- Hosenpud JD, Bennett LE, Keck BM, et al. Effect of diagnosis on survival benefit of lung transplantation for end-stage lung disease. Lancet 1998;351:24-7. [Crossref] [PubMed]
- Ley B, Ryerson CJ, Vittinghoff E, et al. A multidimensional index and staging system for idiopathic pulmonary fibrosis. Ann Intern Med 2012;156:684-91. [Crossref] [PubMed]
- du Bois RM, Weycker D, Albera C, et al. Ascertainment of individual risk of mortality for patients with idiopathic pulmonary fibrosis. Am J Respir Crit Care Med 2011;184:459-66. [Crossref] [PubMed]
- Richards TJ, Kaminski N, Baribaud F, et al. Peripheral blood proteins predict mortality in idiopathic pulmonary fibrosis. Am J Respir Crit Care Med 2012;185:67-76. [Crossref] [PubMed]
- Herazo-Maya JD, Noth I, Duncan SR, et al. Peripheral blood mononuclear cell gene expression profiles predict poor outcome in idiopathic pulmonary fibrosis. Sci Transl Med 2013;5:205ra136. [Crossref] [PubMed]
- Herazo-Maya JD, Sun J, Molyneaux PL, et al. Validation of a 52-gene risk profile for outcome prediction in patients with idiopathic pulmonary fibrosis: an international, multicentre, cohort study. Lancet Respir Med 2017;5:857-68. [Crossref] [PubMed]
- Huang Y, Ma SF, Vij R, et al. A functional genomic model for predicting prognosis in idiopathic pulmonary fibrosis. BMC Pulm Med 2015;15:147. [Crossref] [PubMed]
- Lu Y, Chen J, Tang K, et al. Development and Validation of the Prognostic Index Based on Inflammation-Related Gene Analysis in Idiopathic Pulmonary Fibrosis. Front Mol Biosci 2021;8:667459. [Crossref] [PubMed]
- Prasse A, Pechkovsky DV, Toews GB, et al. A vicious circle of alveolar macrophages and fibroblasts perpetuates pulmonary fibrosis via CCL18. Am J Respir Crit Care Med 2006;173:781-92. [Crossref] [PubMed]
- Hara A, Sakamoto N, Ishimatsu Y, et al. S100A9 in BALF is a candidate biomarker of idiopathic pulmonary fibrosis. Respir Med 2012;106:571-80. [Crossref] [PubMed]
- Landi C, Bargagli E, Bianchi L, et al. Towards a functional proteomics approach to the comprehension of idiopathic pulmonary fibrosis, sarcoidosis, systemic sclerosis and pulmonary Langerhans cell histiocytosis. J Proteomics 2013;83:60-75. [Crossref] [PubMed]
- Landi C, Bargagli E, Carleo A, et al. A system biology study of BALF from patients affected by idiopathic pulmonary fibrosis (IPF) and healthy controls. Proteomics Clin Appl 2014;8:932-50. [Crossref] [PubMed]
- Prasse A, Binder H, Schupp JC, et al. BAL Cell Gene Expression Is Indicative of Outcome and Airway Basal Cell Involvement in Idiopathic Pulmonary Fibrosis. Am J Respir Crit Care Med 2019;199:622-30. [Crossref] [PubMed]
- Li X, Cai H, Cai Y, et al. Investigation of a Hypoxia-Immune-Related Microenvironment Gene Signature and Prediction Model for Idiopathic Pulmonary Fibrosis. Front Immunol 2021;12:629854. [Crossref] [PubMed]
- Xia Y, Lei C, Yang D, et al. Construction and validation of a bronchoalveolar lavage cell-associated gene signature for prognosis prediction in idiopathic pulmonary fibrosis. Int Immunopharmacol 2021;92:107369. [Crossref] [PubMed]
- Li M, Wang K, Zhang Y, et al. Ferroptosis-Related Genes in Bronchoalveolar Lavage Fluid Serves as Prognostic Biomarkers for Idiopathic Pulmonary Fibrosis. Front Med (Lausanne) 2021;8:693959. [Crossref] [PubMed]
- Boon K, Bailey NW, Yang J, et al. Molecular phenotypes distinguish patients with relatively stable from progressive idiopathic pulmonary fibrosis (IPF). PLoS One 2009;4:e5134. [Crossref] [PubMed]
- Mathai SK, Yang IV, Schwarz MI, et al. Incorporating genetics into the identification and treatment of Idiopathic Pulmonary Fibrosis. BMC Med 2015;13:191. [Crossref] [PubMed]
- Mogulkoc N, Brutsche MH, Bishop PW, et al. Pulmonary function in idiopathic pulmonary fibrosis and referral for lung transplantation. Am J Respir Crit Care Med 2001;164:103-8. [Crossref] [PubMed]
- Tibshirani R. Regression Shrinkage and Selection via the Lasso. J R Stat Soc Ser B- Stat Methodol 1996;58:267-88.
- Tibshirani R. The lasso method for variable selection in the Cox model. Stat Med 1997;16:385-95. [Crossref] [PubMed]
- Akaike H. A new look at the statistical model identification. IEEE Trans Automat Contr 1974;19:716-23. [Crossref]
- Inoue Y, Kaner RJ, Guiot J, et al. Diagnostic and Prognostic Biomarkers for Chronic Fibrosing Interstitial Lung Diseases With a Progressive Phenotype. Chest 2020;158:646-59. [Crossref] [PubMed]
- United Kingdom Transplant Support Service Authority. Cardiothoracic Organ Transplant Audit 1985–1995. Bristol, 1996:50-6.
- Desai B, Mattson J, Paintal H, et al. Differential expression of monocyte/macrophage- selective markers in human idiopathic pulmonary fibrosis. Exp Lung Res 2011;37:227-38. [Crossref] [PubMed]
- Huaux F, Gharaee-Kermani M, Liu T, et al. Role of Eotaxin-1 (CCL11) and CC chemokine receptor 3 (CCR3) in bleomycin-induced lung injury and fibrosis. Am J Pathol 2005;167:1485-96. [Crossref] [PubMed]
- Bowler RP, Nicks M, Warnick K, et al. Role of extracellular superoxide dismutase in bleomycin-induced pulmonary fibrosis. Am J Physiol Lung Cell Mol Physiol 2002;282:L719-26. [Crossref] [PubMed]
- Fattman CL, Chang LY, Termin TA, et al. Enhanced bleomycin-induced pulmonary damage in mice lacking extracellular superoxide dismutase. Free Radic Biol Med 2003;35:763-71. [Crossref] [PubMed]
- Fattman CL, Tan RJ, Tobolewski JM, et al. Increased sensitivity to asbestos-induced lung injury in mice lacking extracellular superoxide dismutase. Free Radic Biol Med 2006;40:601-7. [Crossref] [PubMed]
- Kliment CR, Englert JM, Gochuico BR, et al. Oxidative stress alters syndecan-1 distribution in lungs with pulmonary fibrosis. J Biol Chem 2009;284:3537-45. [Crossref] [PubMed]
- Smits NC, Kobayashi T, Srivastava PK, et al. HS3ST1 genotype regulates antithrombin's inflammomodulatory tone and associates with atherosclerosis. Matrix Biol 2017;63:69-90. [Crossref] [PubMed]
- Kim YS, Hwan JD, Bae S, et al. Identification of differentially expressed genes using an annealing control primer system in stage III serous ovarian carcinoma. BMC Cancer 2010;10:576. [Crossref] [PubMed]
- Santoro C, Giugliano T, Kraemer M, et al. Whole exome sequencing identifies MRVI1 as a susceptibility gene for moyamoya syndrome in neurofibromatosis type 1. PLoS One 2018;13:e0200446. [Crossref] [PubMed]
- Ehler E, Horowits R, Zuppinger C, et al. Alterations at the intercalated disk associated with the absence of muscle LIM protein. J Cell Biol 2001;153:763-72. [Crossref] [PubMed]
- Perriard JC, Hirschy A, Ehler E. Dilated cardiomyopathy: a disease of the intercalated disc? Trends Cardiovasc Med 2003;13:30-8. [Crossref] [PubMed]
- Krieger M, Stern DM. Series introduction: multiligand receptors and human disease. J Clin Invest 2001;108:645-7. [Crossref] [PubMed]
- Kzhyshkowska J, Krusell L. Cross-talk between endocytic clearance and secretion in macrophages. Immunobiology 2009;214:576-93. [Crossref] [PubMed]
- Xu J, Deng X, Tang M, et al. Tyrosylprotein sulfotransferase-1 and tyrosine sulfation of chemokine receptor 4 are induced by Epstein-Barr virus encoded latent membrane protein 1 and associated with the metastatic potential of human nasopharyngeal carcinoma. PLoS One 2013;8:e56114. [Crossref] [PubMed]
- Jiang Z, Zhu J, Ma Y, et al. Tyrosylprotein sulfotransferase 1 expression is negatively correlated with c Met and lymph node metastasis in human lung cancer. Mol Med Rep 2015;12:5217-22. [Crossref] [PubMed]
- O'Dwyer DN, Ashley SL, Gurczynski SJ, et al. Lung Microbiota Contribute to Pulmonary Inflammation and Disease Progression in Pulmonary Fibrosis. Am J Respir Crit Care Med 2019;199:1127-38. [Crossref] [PubMed]
- Gibson CD, Kugler MC, Deshwal H, et al. Advances in Targeted Therapy for Progressive Fibrosing Interstitial Lung Disease. Lung 2020;198:597-608. [Crossref] [PubMed]
- Bouwmeester W, Zuithoff NP, Mallett S, et al. Reporting and methods in clinical prediction research: a systematic review. PLoS Med 2012;9:1-12. [Crossref] [PubMed]