Molecular clustering based on gene set expression and its relationship with prognosis in patients with lung adenocarcinoma
Introduction
Lung cancer is one of the most prevalent malignant tumors worldwide, with high morbidity and mortality (1,2). Non-small cell lung carcinoma (NSCLC) and small cell carcinoma are the 2 main types of lung cancer, accounting for 85% of all cases (3). Lung adenocarcinoma (LUAD) makes up 40% of NSCLC (4) and is highly invasive and metastatic, yielding a 5-year survival rate of merely 19.5% (5). Despite improvements in computed tomography (CT) imaging, bronchoscopy, sputum cytology, and major surgical, radiotherapy, and chemotherapy treatments with improved sensitivity and accuracy (6), the prognosis of patients with LUAD remains poor. Therefore, it is necessary to explore the pathophysiological and molecular mechanisms of LUAD to develop novel diagnostic and therapeutic strategies.
With the rapid development of molecular biology in recent years, there are large amounts of data available in public databases for statistical analysis. LUAD subtypes based on proteome, methylation (7), gene expression (8,9), complementary RNA (cRNA) (10), DNA repair-based gene expression signatures (11), and immune-related signature (12) have been reported, but studies in this field focusing on gene set-based expression (GSE) typing are limited (13,14). GSE utilizes the gene expression profiles of functionally related gene sets in Gene Ontology (GO) categories or priori-defined biological classes to assess the significance of gene sets associated with clinical outcomes or phenotypes (14). Furthermore, GSE can reflect the interactions between tumor and immune cells and provide clues for finding predictive biomarkers and new targets (15). The potentially relevant gene expression signatures between specific subgroups are commonly identified using Gene Set Enrichment Analysis (GSEA), which can calculate the enrichment score for a set of genes associated with survival and prognosis in LUAD. A published study in this field identified novel 19-gene prognostic signature based on gene expression in LUAD patients (16). Single-sample GSEA is specially used to calculate the score for the enrichment of a set of genes regulating the DNA damage repair (DDR) pathway (17). Gene sets contain more genetic information, which may be more effective in clarifying the molecular mechanism of LUAD and predicting prognosis than single-gene studies.
This present study demonstrated that LUAD can be classified into 3 clinically relevant subtypes with distinct survival patterns based on gene set expression. The findings were validated using an independent dataset (GSE68465). Furthermore, the common differential gene sets were identified, and functional clustering and pathway analyses were performed on upregulated gene sets to identify the hub genes. We present the following article in accordance with the STREGA reporting checklist (available at https://jtd.amegroups.com/article/view/10.21037/jtd-22-557/rc).
Methods
Patients and gene expression microarray data acquisition
High-throughput RNA sequencing (RNA-seq) and clinical data were downloaded from The Cancer Genome Atlas (TCGA) database (https://tcga-data.nci.nih.gov/tcga/). The criteria for screening cases and files in the TCGA databases were detailed in Table 1. The data was not limited by age at diagnosis, days to death, nor race and ethnicity. Finally, 490 cases and 551 files were obtained. Clinical data were exported as XML files and 486 clinical files were collated. The study was conducted in accordance with the Declaration of Helsinki (as revised in 2013).
Table 1
Options | Choice | Options | Choice |
---|---|---|---|
Primary site | Bronchus and lung | Data category | Gene expression quantification |
Program | TCGA | Experimental strategy | RNA-seq |
Project | TCGA-LUAD | Workflow type | *HTSeq-FPKM |
Disease | Adenomas and adenocarcinomas |
*, HTSeq is a Python software used for gene Count expression analysis. TCGA, The Cancer Genome Atlas; LUAD, lung adenocarcinoma; RNA-seq, RNA sequencing; FPKM, Fragments per Kilobase of transcript per Million mapped reads.
The TCGA-LUAD samples were pooled into an integral meta-dataset using the Perl software and batch effects were removed by applying the ComBat function in the SVA package of the R software. In the TCGA cohort, 551 samples, including 54 non-tumor samples and 497 tumor samples, were selected. c7.immunesigdb-HALLMARK.gmt was downloaded from GSEA, which was used to realize gene set clustering analysis (GSCA).
Unsupervised cluster analysis
Molecular cancer subtypes from multi-omics data were identified, validated, and visualized using the R package CancerSubtypes (18). The algorithm for feature selection was based on a multivariate Cox regression model (features included gene expression, overall survival time, status, and cutoff <0.05) and was applied to the TCGA dataset. Different gene subtypes were identified using the clustering method [consensus nonnegative matrix factorization (NMF)] which is an unsupervised learning method for pattern recognition on gene set expression profiling and cell composition classifying genes into clusters. The default times of the run was set at 30 to ensure computation of a consensus matrix for selection of the best possible results. The accuracy and fit of the clustering assignment were assessed by the silhouette width index. The values of the silhouette width ranges from −1 to 1 and are positively associated with the degree of cohesion and separation.
The heatmaps were drawn using the “pHeatmap” package. The patient survival probability among clusters was analyzed using Kaplan-Meier survival curves with log-rank tests. A P value <0.05 was considered statistically significant.
Analysis of different gene sets among the different subtypes
To identify the different gene sets between different subtypes, the “venndiagram” package was used. The conditions were set to FDR <0.05 and |logFC | >0.02.
Establishment and verification of a survival risk scoring model
For the common differential gene sets obtained above, the univariate Cox regression and Kaplan-Meier method in the “survival” package were used to classify the gene sets into a high-risk group and a low-risk group. The survival rates were compared between the two groups. Gene sets related to prognosis were identified, and Lasso regression was performed using the glmnet package to obtain the model and formula. The high-risk group and the low-risk group were determined by the cut-off value of the mean risk score of each sample in the training group and the validation group. The cross validation was performed using the GSE68465 dataset, and the validation group was similarly processed.
Functional and pathway enrichment analyses
Gene Ontology (GO) analyses and KEGG (Kyoto Encyclopedia of Genes and Genomes) analyses were performed on the gene sets identified in the Lasso regression model to understand the biological characteristics of these genes. Protein-protein interaction (PPI) network analysis is a powerful tool for understanding the biological responses in various lung cancer subtypes. In the PPI network, a protein is defined as a node, while the interaction between two nodes is defined as an edge. The size of the node correlates to the degree: the larger the size, the higher the degree. The thickness of an edge indicates the degree of correlation: the thicker the edge, the higher the correlation degree (19). The online database STRING (https://string-db.org/) was used to construct a PPI network of the genes and analyze the functional interactions between proteins. A confidence score ≥0.400 was considered significant. The hub genes were analyzed using Cytoscape, which are important nodes with many interactions visualizing the PPI networks. The modules of the PPI network identified were screened using the Cytoscape plug-in molecular complex detection (MCODE). The default cutoff values of MCODE, node score, K core, and the maximum depth were set at 2, 0.2, 2, and 100, respectively. The topological algorithm and the PPI network were constructed using the STRING software to determine the top 10 hub genes (20).
Statistical analysis
The diagnostic performance of the genes was assessed using area under the receiver operating characteristic (ROC) curve (AUC). The distribution of the differentially expressed genes was shown by heatmaps. Survival rates among different LUAD subtypes were analyzed by using Kaplan-Meier survival curve with Log-rank test. A two-tailed P value <0.05 was considered as statistical significance. All the statistical analyses were performed by using R software (Version 4.1.1).
Results
Identification of three LUAD subtypes using unsupervised hierarchical cluster analysis
The study design is shown in the flow chart in Figure 1.
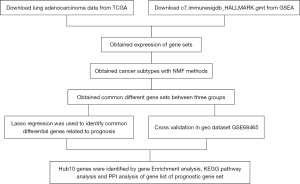
The TCGA-LUAD dataset and the c7.immunesigdb-Hallmark.gmt from GSEA were merged to obtain the gene set expression data, and the differences in gene set expression between the healthy group and the malignant group were compared (Figure 2). According to the total within sum of square (Figure 3A), the samples were divided into three subtypes (Figure 3B). The contour width of the three types were 0.95, 0.96, and 0.99 respectively, and the overall mean value was 0.96 (Figure 3C). The survival rates of the three subtypes varied significantly (P<0.05), with type 1 showing the lowest survival probability (Figure 3D). The differential gene set expression in the three subtypes are shown in Figure 3E. Detailed clinical data of the three subtypes are shown in Table 2.
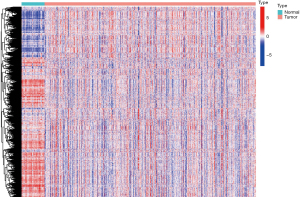
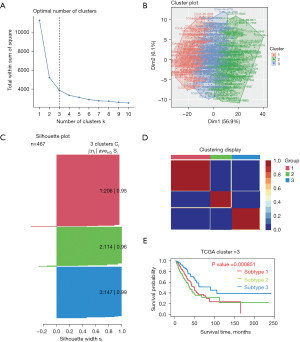
Table 2
Covariates | Cluster | Total | C1 | C2 | C3 | P value |
---|---|---|---|---|---|---|
Age (years) | ≤65 | 224 (47.97%) | 107 (51.94%) | 60 (52.63%) | 57 (38.78%) | 0.02 |
>65 | 233 (49.89%) | 98 (47.57%) | 49 (42.98%) | 86 (58.5%) | ||
Unknown | 10 (2.14%) | 1 (0.49%) | 5 (4.39%) | 4 (2.72%) | ||
Gender | Female | 254 (54.39%) | 107 (51.94%) | 54 (47.37%) | 93 (63.27%) | 0.02 |
Male | 213 (45.61%) | 99 (48.06%) | 60 (52.63%) | 54 (36.73%) | ||
Stage | Stage I | 253 (54.18%) | 101 (49.03%) | 50 (43.86%) | 102 (69.39%) | <0.01 |
Stage II | 107 (22.91%) | 54 (26.21%) | 28 (24.56%) | 25 (17.01%) | ||
Stage III | 74 (15.85%) | 39 (18.93%) | 24 (21.05%) | 11 (7.48%) | ||
Stage IV | 25 (5.35%) | 10 (4.85%) | 10 (8.77%) | 5 (3.4%) | ||
Unknown | 8 (1.71%) | 2 (0.97%) | 2 (1.75%) | 4 (2.72%) | ||
T | T1 | 159 (34.05%) | 58 (28.16%) | 25 (21.93%) | 76 (51.7%) | <0.01 |
T2 | 248 (53.1%) | 122 (59.22%) | 71 (62.28%) | 55 (37.41%) | ||
T3 | 39 (8.35%) | 17 (8.25%) | 13 (11.4%) | 9 (6.12%) | ||
T4 | 18 (3.85%) | 9 (4.37%) | 4 (3.51%) | 5 (3.4%) | ||
Unknown | 3 (0.64%) | 0 (0%) | 1 (0.88%) | 2 (1.36%) | ||
M | M0 | 314 (67.24%) | 138 (66.99%) | 79 (69.3%) | 97 (65.99%) | 0.15 |
M1 | 24 (5.14%) | 10 (4.85%) | 10 (8.77%) | 4 (2.72%) | ||
Unknown | 129 (27.62%) | 58 (28.16%) | 25 (21.93%) | 46 (31.29%) | ||
N | N0 | 302 (64.67%) | 121 (58.74%) | 68 (59.65%) | 113 (76.87%) | <0.01 |
N1 | 86 (18.42%) | 43 (20.87%) | 24 (21.05%) | 19 (12.93%) | ||
N2 | 65 (13.92%) | 38 (18.45%) | 21 (18.42%) | 6 (4.08%) | ||
N3 | 2 (0.43%) | 2 (0.97%) | 0 (0%) | 0 (0%) | ||
Unknown | 12 (2.57%) | 2 (0.97%) | 1 (0.88%) | 9 (6.12%) |
C1, Cluster 1; C2, Cluster 2; C3, Cluster 3.
Analysis of the common gene sets among the different subtypes
Pairwise comparisons were made among the three tumor subtypes to obtain the differential gene sets among the three groups, namely, C2–C1, C3–C2, and C3–C1 (available online: https://cdn.amegroups.cn/static/public/jtd-22-557-1-3.zip). The intersection of the three differential gene sets was taken as the common differential gene set, as represented by the Venn diagram (Figure 4A). The association between the common differential gene sets and the clinical data was examined (Figure 4B).
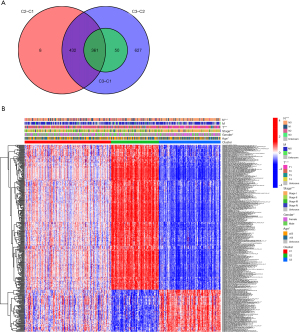
Establishment and verification of a survival risk scoring model based on the common differential gene sets
Univariate Cox regression analysis was performed on the common differential gene sets and the survival data to obtain the prognosis-related gene sets (available online: https://cdn.amegroups.cn/static/public/jtd-22-557-4.xls). Lasso regression was performed to obtain the risk score model as follows:
6.88*GSE45365-HEALTHY-VS-MCMV-INFECTION-BCELL-IFNAR-KO-UP-3.96*GSE24634-NAIVE-CD4-TCELL-VS-DAY5-IL4-CONV-TREG-UP-4.60*GSE36476-YOUNG-VS-OLD-DONOR-MEMORY-CD4-TCELL-72H-TSST-ACT-DN. The Lasso regression diagram is shown in Figure 5A. Using the GSE68465 dataset, there were significant differences in survival rates among the three subtypes (P<0.05; Figure 5B). The contour widths of the three subtypes were determined to be 0.98, 0.98, and 0.93, with an average contour width of 0.96 (Figure 5C), and all subtypes performed well (Figure 5D). The risk score of the training group was calculated based on the risk model and was divided into a high-risk group and a low-risk group using the cut-off value. Kaplan-Meier survival curves demonstrated that there was a significant difference between the two groups (Figure 6A) and the training group (Figure 6B). The mean expression of the three gene sets was the cut-off value, which was divided into a high-expression group and a low-expression group. The results showed that the expression of GSE45365-HEALTHY-VS-MCMV-INFECTION-BCELL-IFNAR-KO-UP was positive, with better prognosis. The opposite expression was observed in the other two gene sets (Figure 7A-7C).
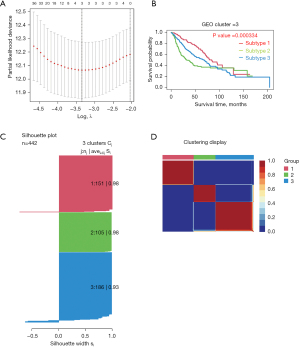
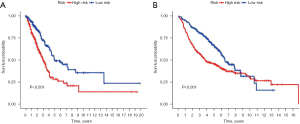
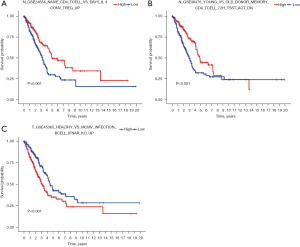
Functional and pathway enrichment analyses
Functional and pathway cluster analyses were performed on the gene list using the R software packages “enrichplot” and “clusterprofiler”. GO analysis indicated that the main biological processes (BP) were regulating cell cycle, regulating mitotic cell cycle phase transition, and catabolic procedure. The main cellular components (CC) were spindle, chromosomal region, and midbody. The related molecular functions (MF) were mainly ubiquitin-like protein ligase binding, translation regulator activity, and oxidation activity (Figure 8A). Kyoto Encyclopedia of Genes and Genomes (KEGG) analysis revealed that Epstein-Barr virus (EBV) infection was the most significantly enriched KEGG pathway for key module genes, the p53 signaling pathway, and the proteome pathway (Figure 8B). Furthermore, the PPI network was analyzed using STRING and Cytospace 3.0, and the top 9 hub genes identified were KIF2C, DLGAP5, KIF20A, PSMC1, PSMD1, PSMB7, SNAI2, FGF13, and BMP2 (Figure 9).
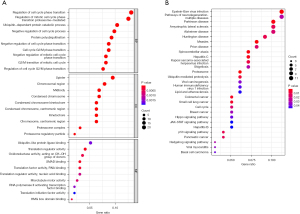
Discussion
The management of lung cancer is challenging in clinical practice because even patients with early-stage adenocarcinoma who receive complete surgical resection are at considerable risk of recurrence and mortality. Therefore, research on the molecular mechanisms of LUAD is of great significance for the treatment and diagnosis of lung cancer (21,22). The target of microRNAs (miRNAs) or methylation is the mRNA, therefore the expression of the target mRNA is crucial (23). Gene expression profiling techniques, such as microarrays or RNA-seq, have been widely used to generate a wealth of transcriptomic profiles in many cancer types (24,25). In this study, we combined data from the TCGA database with GMT files to identify a set of differentially expressed genes. This gene set was combined with clinical information and three subtypes of LUAD were identified.
This present study demonstrated that LUAD could be classified into three clinically relevant subtypes with different survival patterns. The common differential gene sets of the three subtypes were analyzed, and 361 common differential gene sets were identified. Among the 361 differential gene sets, 3 gene sets were related to prognosis.
The down- or up-regulation of these 3 related gene sets could be explained as “genes upregulated during primary acute viral infection: B lymphocytes versus CD8 T cells”. Functional aggregation and pathway analysis showed that the differentially expressed genes were mainly involved with virus infections, measles, and other infection diseases, suggesting that the poor prognosis associated with LUAD may be related to immunity and infection. This agrees with many previous studies (26-28). Inflammatory molecules have been shown to be associated with the development, transformation, and survival of lung cancer, including tumor necrosis factor (TNF)-α (29), transforming growth factor (TGF)-β (30), and interleukin (IL)-10 (30). Therefore, cancer may be treated by inhibiting immune responses, which is also the basis of immunotherapy, which has become a significant treatment strategy, along with surgery and chemotherapy (8). The PPI network analysis identified 9 hub genes which may be potential targets for immunotherapy.
In summary, based on unsupervised learning and expression of gene sets, we successfully stratified LUAD into three clinically relevant subtypes with different survival patterns. Furthermore, we identified a common prognosis-related gene set and identified hub genes of the three subtypes.
Acknowledgments
Funding: None.
Footnote
Reporting Checklist: The authors have completed the STREGA reporting checklist. Available at https://jtd.amegroups.com/article/view/10.21037/jtd-22-557/rc
Conflicts of Interest: All authors have completed the ICMJE uniform disclosure form (available at https://jtd.amegroups.com/article/view/10.21037/jtd-22-557/coif). The authors have no conflicts of interest to declare.
Ethical Statement: The authors are accountable for all aspects of the work in ensuring that questions related to the accuracy or integrity of any part of the work are appropriately investigated and resolved. The study was conducted in accordance with the Declaration of Helsinki (as revised in 2013).
Open Access Statement: This is an Open Access article distributed in accordance with the Creative Commons Attribution-NonCommercial-NoDerivs 4.0 International License (CC BY-NC-ND 4.0), which permits the non-commercial replication and distribution of the article with the strict proviso that no changes or edits are made and the original work is properly cited (including links to both the formal publication through the relevant DOI and the license). See: https://creativecommons.org/licenses/by-nc-nd/4.0/.
References
- Thai AA, Solomon BJ, Sequist LV, et al. Lung cancer. Lancet 2021;398:535-54. [Crossref] [PubMed]
- Bade BC, Dela Cruz CS. Lung Cancer 2020: Epidemiology, Etiology, and Prevention. Clin Chest Med 2020;41:1-24. [Crossref] [PubMed]
- Ricotti A, Sciannameo V, Balzi W, et al. Incidence and Prevalence Analysis of Non-Small-Cell and Small-Cell Lung Cancer Using Administrative Data. Int J Environ Res Public Health 2021;18:9076. [Crossref] [PubMed]
- Ettinger DS, Wood DE, Aisner DL, et al. NCCN Guidelines Insights: Non-Small Cell Lung Cancer, Version 2.2021. J Natl Compr Canc Netw 2021;19:254-66. [Crossref] [PubMed]
- Siegel RL, Miller KD, Jemal A. Cancer statistics, 2020. CA Cancer J Clin 2020;70:7-30. [Crossref] [PubMed]
- Doll KM, Rademaker A, Sosa JA. Practical Guide to Surgical Data Sets: Surveillance, Epidemiology, and End Results (SEER) Database. JAMA Surg 2018;153:588-9. [Crossref] [PubMed]
- Li XS, Nie KC, Zheng ZH, et al. Molecular subtypes based on DNA methylation predict prognosis in lung squamous cell carcinoma. BMC Cancer 2021;21:96. [Crossref] [PubMed]
- Boldrini L, Giordano M, Melfi F, et al. Distinct Angiogenic microRNA-mRNA Expression Profiles Among Subtypes of Lung Adenocarcinoma. Pathol Oncol Res 2020;26:1089-96. [Crossref] [PubMed]
- Hu F, Zhou Y, Wang Q, et al. Gene Expression Classification of Lung Adenocarcinoma into Molecular Subtypes. IEEE/ACM Trans Comput Biol Bioinform 2020;17:1187-97.
- Qin N, Ma Z, Wang C, et al. Comprehensive characterization of functional eRNAs in lung adenocarcinoma reveals novel regulators and a prognosis-related molecular subtype. Theranostics 2020;10:11264-77. [Crossref] [PubMed]
- Hu B, Liu D, Liu Y, et al. DNA Repair-Based Gene Expression Signature and Distinct Molecular Subtypes for Prediction of Clinical Outcomes in Lung Adenocarcinoma. Front Med (Lausanne) 2020;7:615981. [Crossref] [PubMed]
- Sun S, Guo W, Wang Z, et al. Development and validation of an immune-related prognostic signature in lung adenocarcinoma. Cancer Med 2020;9:5960-75. [Crossref] [PubMed]
- Guo G, Yang L, Wen Y, et al. Analysis of the tumor immune environment identifies an immune gene set-based prognostic signature in non-small cell lung cancer. Ann Transl Med 2022;10:15. [Crossref] [PubMed]
- Hsueh HM, Zhou DW, Tsai CA. Random forests-based differential analysis of gene sets for gene expression data. Gene 2013;518:179-86. [Crossref] [PubMed]
- Gibellini L, De Biasi S, Porta C, et al. Single-Cell Approaches to Profile the Response to Immune Checkpoint Inhibitors. Front Immunol 2020;11:490. [Crossref] [PubMed]
- Zhao J, Guo C, Ma Z, et al. Identification of a novel gene expression signature associated with overall survival in patients with lung adenocarcinoma: A comprehensive analysis based on TCGA and GEO databases. Lung Cancer 2020;149:90-6. [Crossref] [PubMed]
- Sun H, Liu SY, Zhou JY, et al. Specific TP53 subtype as biomarker for immune checkpoint inhibitors in lung adenocarcinoma. EBioMedicine. 2020;60:102990. [Crossref] [PubMed]
- Xu T, Le TD, Liu L, et al. CancerSubtypes: an R/Bioconductor package for molecular cancer subtype identification, validation and visualization. Bioinformatics 2017;33:3131-3. [Crossref] [PubMed]
- Kohl M, Wiese S, Warscheid B. Cytoscape: software for visualization and analysis of biological networks. Methods Mol Biol 2011;696:291-303. [Crossref] [PubMed]
- Chin CH, Chen SH, Wu HH, et al. cytoHubba: identifying hub objects and sub-networks from complex interactome. BMC Syst Biol 2014;8:S11. [Crossref] [PubMed]
- Conde E, Rojo F, Gómez J, et al. Molecular diagnosis in non-small-cell lung cancer: expert opinion on ALK and ROS1 testing. J Clin Pathol 2022;75:145-53. [Crossref] [PubMed]
- Jonna S, Subramaniam DS. Molecular diagnostics and targeted therapies in non-small cell lung cancer (NSCLC): an update. Discov Med 2019;27:167-70. [PubMed]
- Cao J, Gong J, Li X, et al. Unsupervised Hierarchical Clustering Identifies Immune Gene Subtypes in Gastric Cancer. Front Pharmacol 2021;12:692454. [Crossref] [PubMed]
- Chen Z, Zhao M, Li M, et al. Identification of differentially expressed genes in lung adenocarcinoma cells using single-cell RNA sequencing not detected using traditional RNA sequencing and microarray. Lab Invest 2020;100:1318-29. [Crossref] [PubMed]
- Wang J, Dean DC, Hornicek FJ, et al. RNA sequencing (RNA-Seq) and its application in ovarian cancer. Gynecol Oncol 2019;152:194-201. [Crossref] [PubMed]
- McFarland DC, Breitbart W, Miller AH, et al. Depression and Inflammation in Patients With Lung Cancer: A Comparative Analysis of Acute Phase Reactant Inflammatory Markers. Psychosomatics 2020;61:527-37. [Crossref] [PubMed]
- Conway EM, Pikor LA, Kung SH, et al. Macrophages, Inflammation, and Lung Cancer. Am J Respir Crit Care Med 2016;193:116-30. [Crossref] [PubMed]
- Zhang J, Veeramachaneni N. Targeting interleukin-1β and inflammation in lung cancer. Biomark Res 2022;10:5. [Crossref] [PubMed]
- Li CQ, Ma QY, Gao XZ, et al. Research Progress in Anti-Inflammatory Bioactive Substances Derived from Marine Microorganisms, Sponges, Algae, and Corals. Mar Drugs 2021;19:572. [Crossref] [PubMed]
- Shapouri-Moghaddam A, Mohammadian S, Vazini H, et al. Macrophage plasticity, polarization, and function in health and disease. J Cell Physiol 2018;233:6425-40. [Crossref] [PubMed]